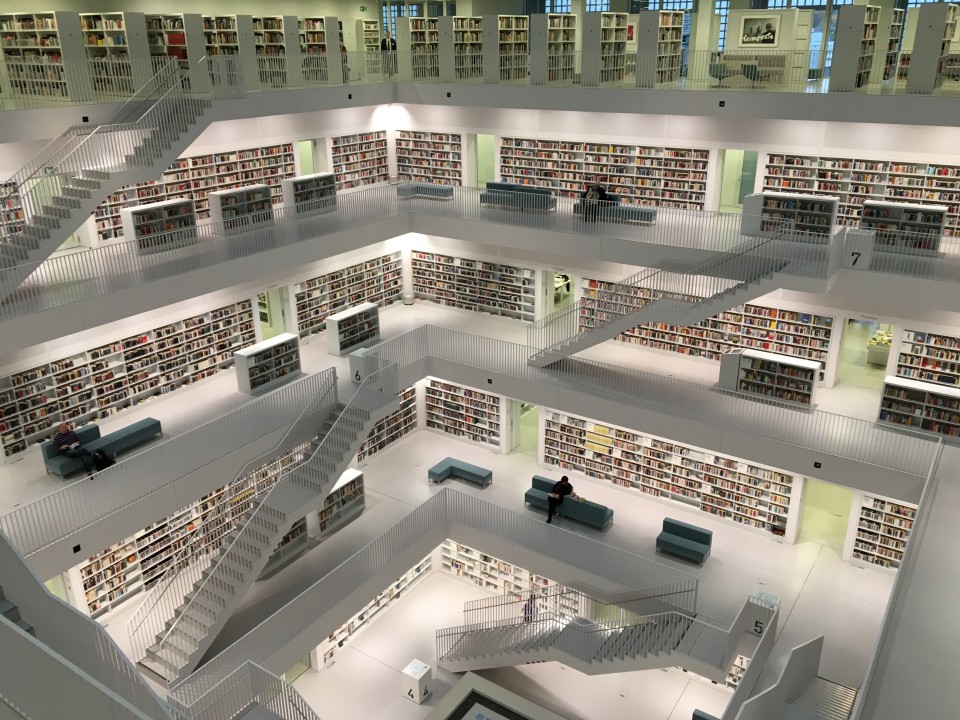
Enterprise Data Engineering Strategy: A must have for New Age organizations
Add Your Heading Text Here
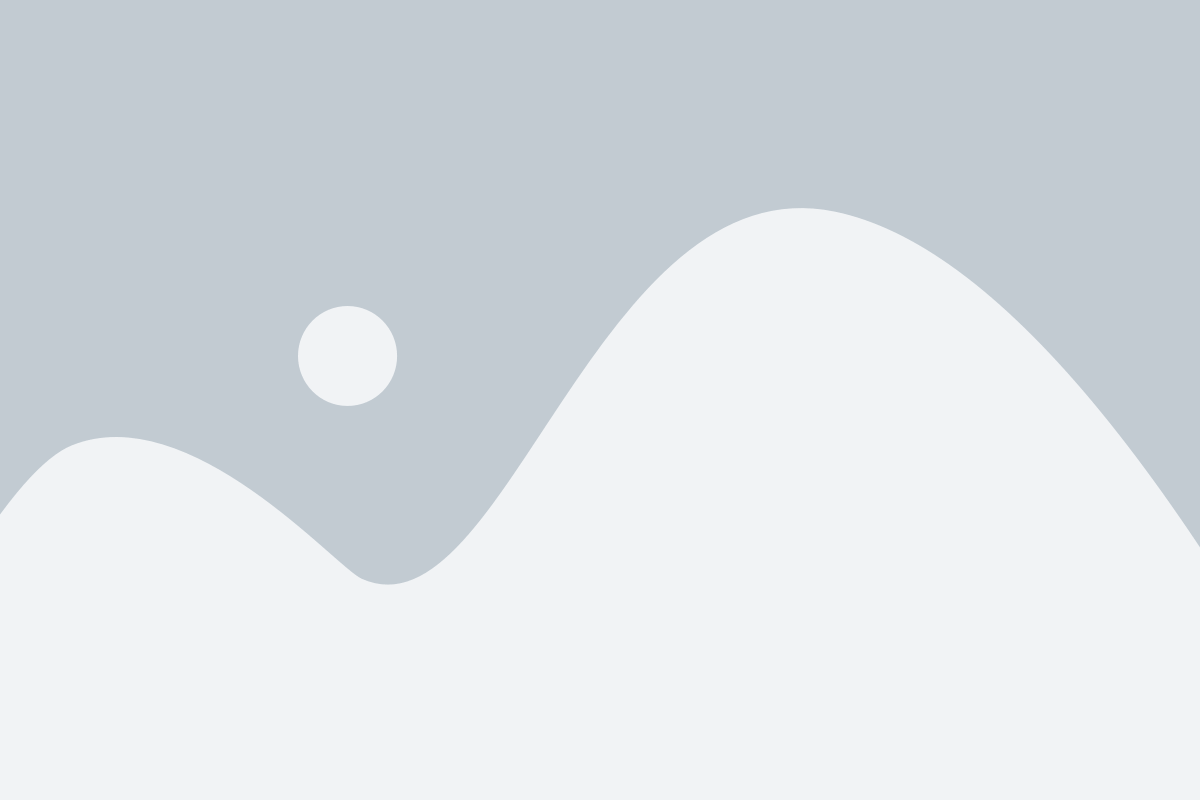
“Enterprise Data Engineering” may sound dated and not so cutting-edge buzzword now. But in this age of several disruptive ideas, concepts and technologies, Enterprise Data Engineering has caught-up with all the necessary advancements and capabilities equally if not better. With organizations becoming more and more keen to be data driven and embrace AI, there is heightened necessity of robust Enterprise Data Engineering Strategy (EDE Strategy). Organizations that do not have efficient EDE Strategy laid out would be ignoring or mismanaging their data asset. Inefficient use of data asset and insights would weaken the competitive edge and their competitors will leap ahead multi-fold in no-time.
EDE Strategy being the master plan of enterprise wide data infrastructure, is a foundational component for any meaningful corporate initiative in recent years. “Explosive growth of data” is being identified as a challenge, an opportunity, a new trend, a significant asset, a source of immense insights, etc. Social media, connected devices and detailed transaction logging are generating huge stream of data which simply can’t be ignored. In simple terms, a panoramic view and wholesome control of a data setup is essential to make data a powerhouse for organizational strategic growth.
Global IP traffic annual run rate is projected to reach 3.3 zettabytes by 2021 (TV & Smartphones together will be accounted for more than 60% of this IP traffic) -CISCO
Along with changing landscape of data, associated disciplines are now aligning to the new demands. EDE Strategy ensures that, these alignments are in line with the roadmap and caters to business users and their requirements.
EDE Strategy encompasses multiple strategy elements that are seeking increased attention now-a-days due to multiple advancements in & around them. Let me introduce some of those strategy elements here:
Automated Data Ingestion & Enrichment
Data Ingestion has now become seriously challenging due to variety & number of sources (social media, new devices, IoT, etc.), new types of data (text stream, video, images, voice, etc), volume of data and the increasing demand for immediately consumable data. Data Ingestion & Enrichment are almost coming together in several scenarios. Due to this, Data Ingestion is almost going off the ETL tools and is fast adopting Pythons and Sparks. Streamed data is not just about absorbing the data as it happens at the source. It is also about enabling AI within the ingestion pipeline to perform data enrichment by bringing together needed data sets (internal & external) and making the Ingestion-to-Insight transformation automatically in seconds/minutes.
ML/AI Models and Algorithms
ML/AI infuses smartness in building data objects, tables, views and models to oversee the data flow across data infrastructure. It uses intelligence in identifying data types/keys/join paths, find & fix data quality issues, identify relationships, identify required data sets to be imported, derive insights, etc. So, the advent of ML/AI in data engineering infuses intelligence into learning, adjusting, alerting and recommending by leaving complex tasks & administration to humans.
Cloud Strategy
The most significant shift seen in the digital world recently is the amount of data being generated and transported. Studies say, 90% of the data existing today were generated in just last 2 years. This is going to increase multi-fold in the coming years. The on-prem based infrastructure and provisioning processes aren’t agile enough to scale rapidly on demand. Even if this is managed, the associated overhead of buying, managing and securing the infrastructure becomes highly expensive and error prone. So, it is essential for organizations to opt for highly efficient and intelligent data platforms on cloud. Cloud offers several advantages across cost, speed, scale, performance, reliability and security. It is also maturing away from initial IaaS into newer services and players. But it doesn’t mean organizations simply initiate the cloud migration and get it done at the press of a button. There should be a carefully drafted Cloud strategy & execution roadmap for adopting cloud in alignment to organization requirements and constraints. Data and information on cloud has the potential to give organizations the flexibility, scalability and ability to discover powerful insights. Cloud also enables applying ML/AI for discovering dark data, monetizing opportunities and disruptive business insights.
Data Lake
Among the data-management technologies most significant space is of data lake. Data Lake is not a specific technology but a concept of housing “one source of truth” data for an organization. When implemented, data lakes can hold and process both structured and unstructured data. Though name indicates huge infrastructure, data lakes are less costly to operate if on cloud. It doesn’t require data to be indexed or prepared to fit specific storage requirements. Instead it holds data in their native formats. Data is then accessed, formatted or reconfigured when needed. Though data lakes are easy to initiate due to easily accessible and affordable cloud offerings, it requires careful planning and incremental adoption model for large scale implementations. In addition, the ever-changing data regulatory & compliance standards add to challenges of implementing and managing data lakes.
Master Data Management (MDM)
Though there is ongoing debate on whether MDM is needed where data lake is the central theme. Schema-on-Write, Schema-on-Read, unstructured data, cloud, etc., are the main contention points in these debates. No matter who wins these debates, it is important for us to know more about MDM while discussing on data engineering. Because, MDM is very essential for organizations to serve their customers/clients near real-time and with better efficiency. As Master Data is the key reference for transactions, typically all independent applications maintain them locally. This leads to redundancy, inconsistency and inefficiency when these data are brought together. It becomes a big challenge while integrating and processing these data due to complexity, chances for errors and increased cost. So, it is important to address MDM element in the EDE strategy carefully by considering organization objectives. Multiple models are practiced for implementing MDM like, registry, hybrid, hub, repository, coexistence, consolidation, etc., which would be discussed in my next articles.
Visualization
Visualization is an exercise that helps in understanding the data in a visual context like patterns, trends, relations, etc. This sounds like an external element or a client to the data engineering. Then, why is Virtualization an important element in EDE Strategy?
Gone are the days of graphs and charts for human analysis. Especially due to data deluge, fitting so much information in a graph or chart is almost impossible for human-beings. They need help in building meaningful representations by consuming huge amount of data. ML/AI is the answer to this wherein its models/algorithms show patterns and correlations by studying huge data-sets in no-time. So, to enable AI to process data, it is important to arrange and label the data suiting it the best. Hence, Visualization is an important aspect to be considered while constructing an Enterprise wide Data Engineering Strategy.
Conclusion
In this new era of data being the new oil, every interest required is being taken to improve the way data is received, cleansed, enriched, assembled and transformed. EDE Strategy ensures establishing effective deployment/management guidelines and continuously improves them because data environments are living organisms. A solid EDE Strategy is highly essential to cater to the demands of this new age. All organizations must have EDE Strategy for realizing their digital vision. Ignoring to have a well laid Enterprise Data Engineering Strategy is as good as regressing in this competitive world.
Related Posts
AIQRATIONS
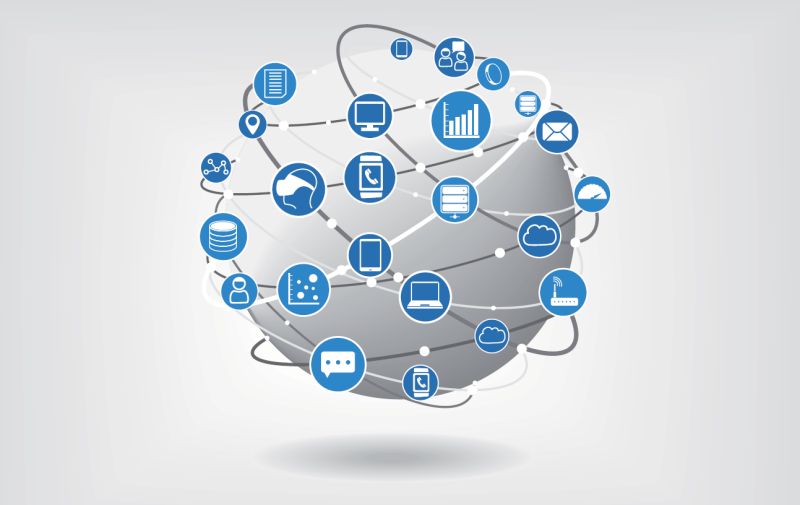
THE BEST PRACTICES FOR INTERNET OF THINGS ANALYTICS
Add Your Heading Text Here
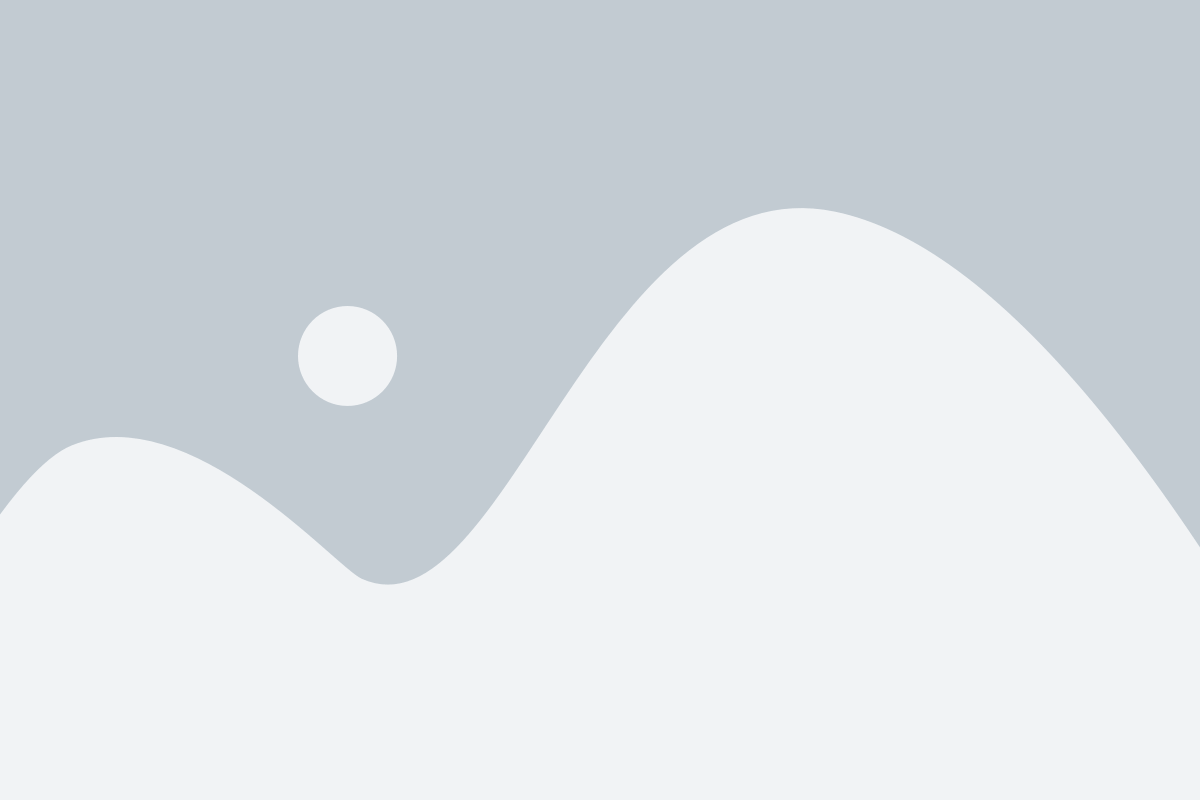
In most ways, Internet of Things analytics are like any other analytics. However, the need to distribute some IoT analytics to edge sites, and to use some technologies not commonly employed elsewhere, requires business intelligence and analytics leaders to adopt new best practices and software.
There are certain prominent challenges that Analytics Vendors are facing in venturing into building a capability. IoT analytics use most of the same algorithms and tools as other kinds of advanced analytics. However, a few techniques occur much more often in IoT analytics, and many analytics professionals have limited or no expertise in these. Analytics leaders are struggling to understand where to start with Internet of Things (IoT) analytics. They are not even sure what technologies are needed.
Also, the advent of IoT also leads to collection of raw data in a massive scale. IoT analytics that run in the cloud or in corporate data centers are the most similar to other analytics practices. Where major differences appear is at the “edge” — in factories, connected vehicles, connected homes and other distributed sites. The staple inputs for IoT analytics are streams of sensor data from machines, medical devices, environmental sensors and other physical entities. Processing this data in an efficient and timely manner sometimes requires event stream processing platforms, time series database management systems and specialized analytical algorithms. It also requires attention to security, communication, data storage, application integration, governance and other considerations beyond analytics. Hence it is imperative to evolve into edge analytics and distribute the data processing load all across.
Hence, some IoT analytics applications have to be distributed to “edge” sites, which makes them harder to deploy, manage and maintain. Many analytics and Data Science practitioners lack expertise in the streaming analytics, time series data management and other technologies used in IoT analytics.
Some visions of the IoT describe a simplistic scenario in which devices and gateways at the edge send all sensor data to the cloud, where the analytic processing is executed, and there are further indirect connections to traditional back-end enterprise applications. However, this describes only some IoT scenarios. In many others, analytical applications in servers, gateways, smart routers and devices process the sensor data near where it is generated — in factories, power plants, oil platforms, airplanes, ships, homes and so on. In these cases, only subsets of conditioned sensor data, or intermediate results (such as complex events) calculated from sensor data, are uploaded to the cloud or corporate data centers for processing by centralized analytics and other applications.
The design and development of IoT analytics — the model building — should generally be done in the cloud or in corporate data centers. However, analytics leaders need to distribute runtime analytics that serve local needs to edge sites. For certain IoT analytical applications, they will need to acquire, and learn how to use, new software tools that provide features not previously required by their analytics programs. These scenarios consequently give us the following best practices to be kept in mind:
Develop Most Analytical Models in the Cloud or at a Centralized Corporate Site
When analytics are applied to operational decision making, as in most IoT applications, they are usually implemented in a two-stage process – In the first stage, data scientists study the business problem and evaluate historical data to build analytical models, prepare data discovery applications or specify report templates. The work is interactive and iterative.
A second stage occurs after models are deployed into operational parts of the business. New data from sensors, business applications or other sources is fed into the models on a recurring basis. If it is a reporting application, a new report is generated, perhaps every night or every week (or every hour, month or quarter). If it is a data discovery application, the new data is made available to decision makers, along with formatted displays and predefined key performance indicators and measures. If it is a predictive or prescriptive analytic application, new data is run through a scoring service or other model to generate information for decision making.
The first stage is almost always implemented centrally, because Model building typically requires data from multiple locations for training and testing purposes. It is easier, and usually less expensive, to consolidate and store all this data centrally. Also, It is less expensive to provision advanced analytics and BI platforms in the cloud or at one or two central corporate sites than to license them for multiple distributed locations.
The second stage — calculating information for operational decision making — may run either at the edge or centrally in the cloud or a corporate data center. Analytics are run centrally if they support strategic, tactical or operational activities that will be carried out at corporate headquarters, at another edge location, or at a business partner’s or customer’s site.
Distribute the Runtime Portion of Locally Focused IoT Analytics to Edge Sites
Some IoT analytics applications need to be distributed, so that processing can take place in devices, control systems, servers or smart routers at the sites where sensor data is generated. This makes sure the edge location stays in operation even when the corporate cloud service is down. Also, wide-area communication is generally too slow for analytics that support time-sensitive industrial control systems.
Thirdly, transmitting all sensor data to a corporate or cloud data center may be impractical or impossible if the volume of data is high or if reliable, high-bandwidth networks are unavailable. It is more practical to filter, condition and do analytic processing partly or entirely at the site where the data is generated.
Train Analytics Staff and Acquire Software Tools to Address Gaps in IoT-Related Analytics Capabilities
Most IoT analytical applications use the same advanced analytics platforms, data discovery tools as other kinds of business application. The principles and algorithms are largely similar. Graphical dashboards, tabular reports, data discovery, regression, neural networks, optimization algorithms and many other techniques found in marketing, finance, customer relationship management and advanced analytics applications also provide most aspects of IoT analytics.
However, a few aspects of analytics occur much more often in the IoT than elsewhere, and many analytics professionals have limited or no expertise in these. For example, some IoT applications use event stream processing platforms to process sensor data in near real time. Event streams are time series data, so they are stored most efficiently in databases (typically column stores) that are designed especially for this purpose, in contrast to the relational databases that dominate traditional analytics. Some IoT analytics are also used to support decision automation scenarios in which an IoT application generates control signals that trigger actuators in physical devices — a concept outside the realm of traditional analytics.
In many cases, companies will need to acquire new software tools to handle these requirements. Business analytics teams need to monitor and manage their edge analytics to ensure they are running properly and determine when analytic models should be tuned or replaced.
Increased Growth, if not Competitive Advantage
The huge volume and velocity of data in IoT will undoubtedly put new levels of strain on networks. The increasing number of real-time IoT apps will create performance and latency issues. It is important to reduce the end-to-end latency among machine-to-machine interactions to single-digit milliseconds. Following the best practices of implementing IoT analytics will ensure judo strategy of increased effeciency output at reduced economy. It may not be suffecient to define a competitive strategy, but as more and more players adopt IoT as a mainstream, the race would be to scale and grow as quickly as possible.