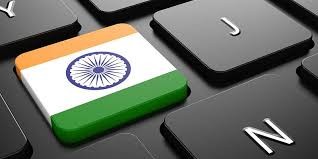
Personal Data Sharing & Protection: Strategic relevance from India’s context
Add Your Heading Text Here
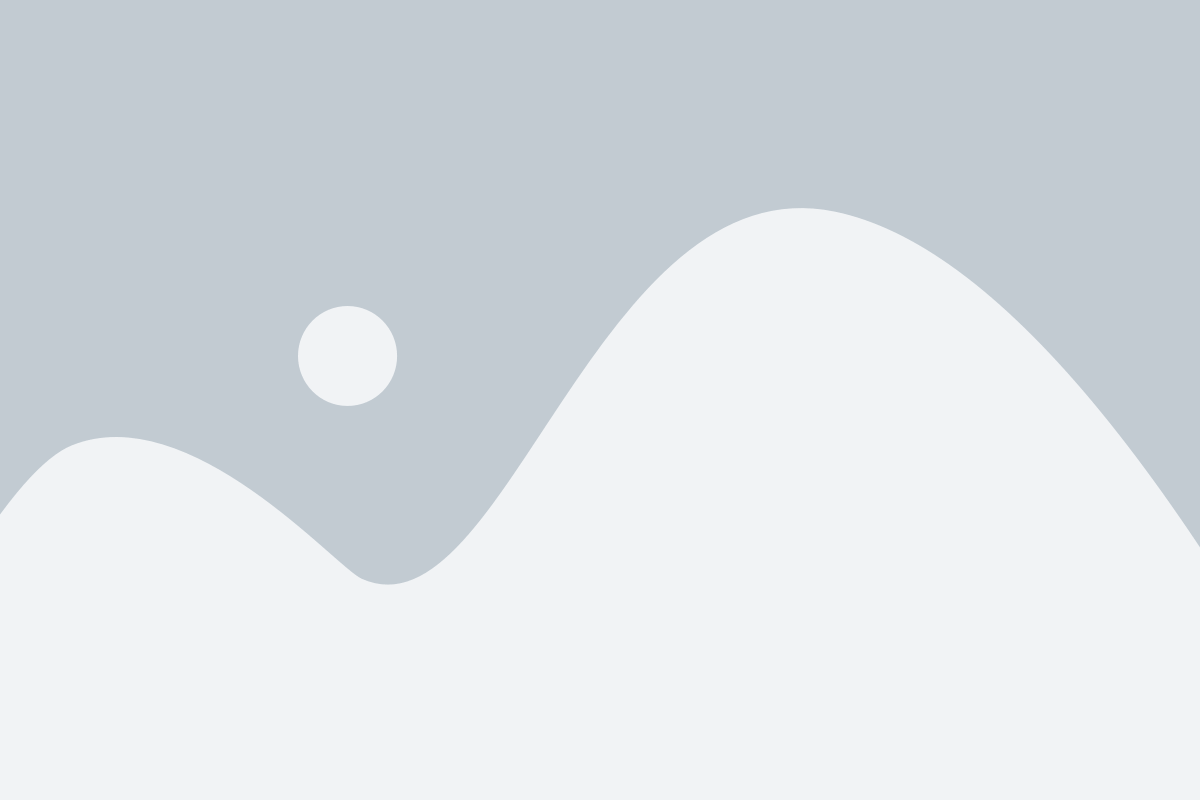
India’s Investments in the digital financial infrastructure—known as “India Stack”—have sped up the large-scale digitization of people’s financial lives. As more and more people begin to conduct transactions online, questions have emerged about how to provide millions of customers adequate data protection and privacy while allowing their data to flow throughout the financial system. Data-sharing among financial services providers (FSPs) can enable providers to more efficiently offer a wider range of financial products better tailored to the needs of customers, including low-income customers.
However, it is important to ensure customers understand and consent to how their data are being used. India’s solution to this challenge is account aggregators (AAs). The Reserve Bank of India (RBI) created AAs in 2018 to simplify the consent process for customers. In most open banking regimes, financial information providers (FIPs) and financial information users (FIUs) directly exchange data. This direct model of data exchange—such as between a bank and a credit bureau—offers customers limited control and visibility into what data are being shared and to what end. AAs have been designed to sit between FIPs and FIUs to facilitate data exchange more transparently. Despite their name, AAs are barred from seeing, storing, analyzing, or using customer data. As trusted, impartial intermediaries, they simply manage consent and serve as the pipes through which data flow among FSPs. When a customer gives consent to a provider via the AA, the AA fetches the relevant information from the customer’s financial accounts and sends it via secure channels to the requesting institution. implementation of its policies for consensual data-sharing, including the establishment and operation of AAs. It provides a set of guiding design principles, outlines the technical format of data requests, and specifies the parameters governing the terms of use of requested data. It also specifies how to log consent and data flows.
There are several operational and coordination challenges across these three types of entities: FIPs, FIUs, and AAs. There are also questions around the data-sharing business model of AAs. Since AAs are additional players, they generate costs that must be offset by efficiency gains in the system to mitigate overall cost increases to customers. It remains an open question whether AAs will advance financial inclusion, how they will navigate issues around digital literacy and smartphone access, how the limits of a consent-based model of data protection and privacy play out, what capacity issues will be encountered among regulators and providers, and whether a competitive market of AAs will emerge given that regulations and interoperability arrangements largely define the business.
Account Aggregators (AA’s):
ACCOUNT AGGREGATORS (AAs) is one of the new categories of non banking financial companies (NBFCs) to figure into India Stack—India’s interconnected set of public and nonprofit infrastructure that supports financial services. India Stack has scaled considerably since its creation in 2009, marked by rapid digitization and parallel growth in mobile networks, reliable data connectivity, falling data costs, and continuously increasing smartphone use. Consequently, the creation, storage, use, and analyses of personal data have become increasingly relevant. Following an “open banking “approach, the Reserve Bank of India (RBI) licensed seven AAs in 2018 to address emerging questions around how data can be most effectively leveraged to benefit individuals while ensuring appropriate data protection and privacy, with consent being a key element in this. RBI created AAs to address the challenges posed by the proliferation of data by enabling data-sharing among financial institutions with customer consent. The intent is to provide a method through which customers can consent (or not) to a financial services provider accessing their personal data held by other entities. Providers are interested in these data, in part, because information shared by customers, such as bank statements, will allow providers to better understand customer risk profiles. The hypothesis is that consent-based data-sharing will help poorer customers qualify for a wider range of financial products—and receive financial products better tailored to their needs.
Data Sharing Model : The new perspective:
Paper based data collection is inconvenient , time consuming and costly for customers and providers. Where models for digital-sharing exist, they typically involve transferring data through intermediaries that are not always secure or through specialized agencies that offer little protection for customers. India’s consent-based data-sharing model provides a digital framework that enables individuals to give and withdraw consent on how and how much of their personal data are shared via secure and standardized channels. India’s guiding principles for sharing data with user consent—not only in the financial sector— are outlined in the National Data Sharing and Accessibility Policy (2012) and the Policy for Open Application Programming Interfaces for the Government of India. The Information Technology Act (2000) requires any entity that shares sensitive personal data to obtain consent from the user before the information is shared. The forthcoming Personal Data Protection Bill makes it illegal for institutions to share personal data without consent.
India’s Ministry of Electronics and Information Technology (MeitY) has issued an Electronic Consent Framework to define a comprehensive mechanism to implement policies for consensual data-sharing. It provides a set of guiding design principles, outlines the technical format of the data request, and specifies the parameters governing the terms of use of the data requested. It also specifies how to log both consent and data flows. This “consent artifact” was adopted by RBI, SEBI, IRDAI, and PFRDA. Components of the consent artifact structure include :
- Identifier : Specifies entities involved in the transaction: who is requesting the data, who is granting permission, who is providing the data, and who is recording consent.
- Data : Describes the type of data being accessed and the permissions for use of the data. Three types of permissions are available: view (read only), store, and query (request for specific data). The artifact structure also specifies the data that are being shared, date range for which they are being requested, duration of storage by the consumer, and frequency of access.
- Purpose : Describes end use, for example, to compute a loan offer.
- Log : Contains logs of who asked for consent, whether it was granted or not, and data flows.
- Digital signature : Identifies the digital signature and digital ID user certificate used by the provider to verify the digital signature. This allows providers to share information in encrypted form
The Approach :
THE AA consent based data sharing model mediates the flow of data between producers and users of data, ensuring that sharing data is subject to granular customer consent. AAs manage only the consent and data flow for the benefit of the consumer, mitigating the risk of an FIU pressuring consumers to consent to access to their data in exchange for a product or service. However, AAs, as entities that sit in the middle of this ecosystem, come with additional costs that will affect the viability of the business model and the cost of servicing consumers. FIUs most likely will urge consumers to go directly to an AA to receive fast, efficient, and low-cost services. However, AAs ultimately must market their services directly to the consumer. While AA services are not an easy sell, the rising levels of awareness among Indian consumers that their data are being sold without their consent or knowledge may give rise to the initial wave of adopters. While the AA model is promising, it remains to be seen how and when it will have a direct impact on the financial lives of consumers.
Differences between Personal Data Protection & GDPR ?
There are some major differences between the two.
First, the bill gives India’s central government the power to exempt any government agency from the bill’s requirements. This exemption can be given on grounds related to national security, national sovereignty, and public order.
While the GDPR offers EU member states similar escape clauses, they are tightly regulated by other EU directives. Without these safeguards, India’s bill potentially gives India’s central government the power to access individual data over and above existing Indian laws such as the Information Technology Act of 2000, which dealt with cyber crime and e-commerce.
Second, unlike the GDPR, India’s bill allows the government to order firms to share any of the non personal data they collect with the government. The bill says this is to improve the delivery of government services. But it does not explain how this data will be used, whether it will be shared with other private businesses, or whether any compensation will be paid for the use of this data.
Third, the GDPR does not require businesses to keep EU data within the EU. They can transfer it overseas, so long as they meet conditions such as standard contractual clauses on data protection, codes of conduct, or certification systems that are approved before the transfer.
The Indian bill allows the transfer of some personal data, but sensitive personal data can only be transferred outside India if it meets requirements that are similar to those of the GDPR. What’s more, this data can only be sent outside India to be processed; it cannot be stored outside India. This will create technical issues in delineating between categories of data that have to meet this requirement, and add to businesses’ compliance costs.
Related Posts
AIQRATIONS
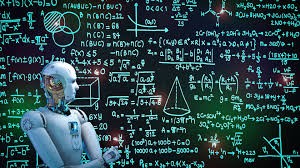
AI Strategy: The Epiphany of Digital Transformation
Add Your Heading Text Here
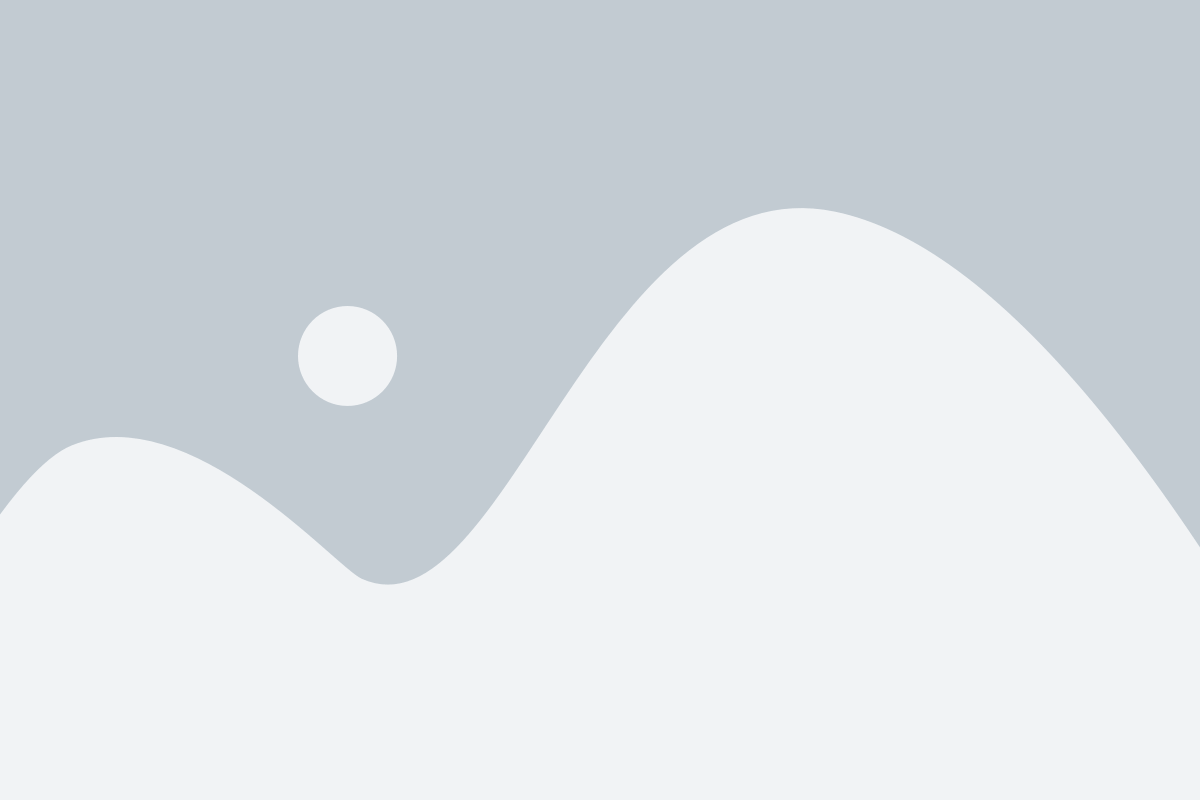
In the past months due to lockdowns and WFH, enterprises have got an epiphany of massive shifts of business and strategic models for staying relevant and solvent. Digital transformation touted as the biggest strategic differentiation and competitive advantages for enterprises faced a quintessential inertia of mass adoption in the legacy based enterprises and remained more on business planning slides than in full implementation. However, Digital Transformation is not about aggregation of exponential technologies and adhoc use cases or stitching alliances with deep tech startups. The underpinning of Digital transformation is AI and how AI strategy has become the foundational aspect of accomplishing digital transformation for enterprises and generating tangible business metrics. But before we get to the significance of AI strategy in digital transformation, we need to understand the core of digital transformation itself. Because digital transformation will look different for every enterprise, it can be hard to pinpoint a definition that applies to all. However, in general terms: we define digital transformation as the integration of core areas of business resulting in fundamental changes to how businesses operate and how they deliver value to customers.
Though, in specific terms digital transformation can take a very interesting shape according to the business moment in question. From a customer’s point of view, “Digital transformation closes the gap between what digital customers already expect and what analog businesses actually deliver.”
Does Digital Transformation really mean bunching exponential technologies? I believe that digital transformation is first and foremost a business transformation. Digital mindset is not only about new age technology, but about curiosity, creativity, problem-solving, empathy, flexibility, decision-making and judgment, among others. Enterprises needs to foster this digital mindset, both within its own boundaries and across the company units. The World Economic Forum lists the top 10 skills needed for the fourth industrial revolution. None of them is totally technical. They are, rather, a combination of important soft skills relevant for the digital revolution. You don’t need to be a technical expert to understand how technology will impact your work. You need to know the foundational aspects, remain open-minded and work with technology mavens. Digital Transformation is more about cultural change that requires enterprises to continually challenge the status quo, experiment often, and get comfortable with failure. The most likely reason for business to undergo digital transformation is the survival & relevance issue. Businesses mostly don’t transform by choice because it is expensive and risky. Businesses go through transformation when they have failed to evolve. Hence its implementation calls for tough decisions like walking away from long-standing business processes that companies were built upon in favor of relatively new practices that are still being defined.
Business Implementation aspects of Digital Transformation
Disruption in digital business implies a more positive and evolving atmosphere, instead of the usual negative undertones that are attached to the word. According to the MIT Center for Digital Business, “Companies that have embraced digital transformation are 26 percent more profitable than their average industry competitors and enjoy a 12 percent higher market valuation.” A lot of startups and enterprises are adopting an evolutionary approach in transforming their business models itself, as part of the digital transformation. According to Mckinsey, One-third of the top 20 firms in industry segments will be disrupted by new competitors within five years.
The various Business Models being adopted in Digital Transformation era are:
- The Subscription Model (Netflix, Dollar Shave Club, Apple Music) Disrupts through “lock-in” by taking a product or service that is traditionally purchased on an ad hoc basis, and locking-in repeat custom by charging a subscription fee for continued access to the product/service
- The Freemium Model (Spotify, LinkedIn, Dropbox) Disrupts through digital sampling, where users pay for a basic service or product with their data or ‘eyeballs’, rather than money, and then charging to upgrade to the full offer. Works where marginal cost for extra units and distribution are lower than advertising revenue or the sale of personal data
- The Free Model (Google, Facebook) Disrupts with an ‘if-you’re-not-paying-for-the-product-you-are-the-product’ model that involves selling personal data or ‘advertising eyeballs’ harvested by offering consumers a ‘free’ product or service that captures their data/attention
- The Marketplace Model (eBay, iTunes, App Store, Uber, Airbnb) Disrupts with the provision of a digital marketplace that brings together buyers and sellers directly, in return for a transaction or placement fee or commission
- The Access-over-Ownership Model (Zipcar, Peer buy) Disrupts by providing temporary access to goods and services traditionally only available through purchase. Includes ‘Sharing Economy’ disruptors, which takes a commission from people monetizing their assets (home, car, capital) by lending them to ‘borrowers’
- The Hypermarket Model (Amazon, Apple) Disrupts by ‘brand bombing’ using sheer market power and scale to crush competition, often by selling below cost price
- The Experience Model (Tesla, Apple) Disrupts by providing a superior experience, for which people are prepared to pay
- The Pyramid Model (Amazon, Microsoft, Dropbox) Disrupts by recruiting an army of resellers and affiliates who are often paid on a commission-only mode
- The On-Demand Model (Uber, Operator, TaskRabbit) Disrupts by monetizing time and selling instant-access at a premium. Includes taking a commission from people with money but no time who pay for goods and services delivered or fulfilled by people with time but no money
- The Ecosystem Model (Apple, Google) Disrupts by selling an interlocking and interdependent suite of products and services that increase in value as more are purchased. Creates consumer dependency
Since Digital Transformation and its manifestation into various business models are being fast adopted by startups, there are providing tough competition to incumbent corporate houses and large enterprises. Though enterprises are also looking forward to digitally transform their enterprise business, the scale and complexity makes it difficult and resource consuming activity. It has imperatively invoked the enterprises to bring certain strategy to counter the cannibalizing effect in the following ways:
- The Block Strategy. Using all means available to inhibit the disruptor. These means can include claiming patent or copyright infringement, erecting regulatory hurdles, and using other legal barriers.
- The Milk Strategy. Extracting the most value possible from vulnerable businesses while preparing for the inevitable disruption
- The Invest in Disruption Model. Actively investing in the disruptive threat, including disruptive technologies, human capabilities, digitized processes, or perhaps acquiring companies with these attributes
- The Disrupt the Current Business Strategy. Launching a new product or service that competes directly with the disruptor, and leveraging inherent strengths such as size, market knowledge, brand, access to capital, and relationships to build the new business
- The Retreat into a Strategic Niche Strategy. Focusing on a profitable niche segment of the core market where disruption is less likely to occur (e.g. travel agents focusing on corporate travel, and complex itineraries, book sellers and publishers focusing on academia niche)
- The Redefine the Core Strategy. Building an entirely new business model, often in an adjacent industry where it is possible to leverage existing knowledge and capabilities (e.g. IBM to consulting, Fujifilm to cosmetics)
- The Exit Strategy. Exiting the business entirely and returning capital to investors, ideally through a sale of the business while value still exists (e.g. MySpace selling itself to Newscorp)
The curious evolution of AI and its relevance in digital transformation
So here’s an interesting question, AI has been around for more than 60 years, then why is it that it is only gaining traction with the advent of digital? The first practical application of such “machine intelligence” was introduced by Alan Turing, British mathematician and WWII code-breaker, in 1950. He even created the Turing test, which is still used today, as a benchmark to determine a machine’s ability to “think” like a human.The biggest differences between AI then and now are Hardware limitations, access to data, and rise of machine learning.
Hardware limitations led to the non-sustenance of AI adoption till late 1990s. There were many instances where the scope and opportunity of AI led transformation was identified and appreciated by implementation saw more difficult circumstances. The field of AI research was founded at a workshop held on the campus of Dartmouth College during the summer of 1956. But Eventually it became obvious that they had grossly underestimated the difficulty of the project due to computer hardware limitations. The U.S. and British Governments stopped funding undirected research into artificial intelligence, leading to years known as an “AI winter”.
In another example, again in 1980, a visionary initiative by the Japanese Government inspired governments and industry to provide AI with billions of dollars, but by the late 80s the investors became disillusioned by the absence of the needed computer power (hardware) and withdrew funding again. Investment and interest in AI boomed in the first decades of the 21st century, when machine learning was successfully applied to many problems in academia and industry due to the presence of powerful computer hardware. Teaming this with the rise in digital, leading to an explosion of data and adoption of data generation in every aspect of business, made it highly convenient for AI to not only be adopted but to evolve to more accurate execution.
The Core of Digital Transformation: AI Strategy
According to McKinsey, by 2023, 85 percent of all digital transformation initiatives will be embedded with AI strategy at its core. Due to radical computational power, near-endless amounts of data, and unprecedented advances in ML algorithms, AI strategy will emerge as the most disruptive business scenario, and its manifestation into various trends that we see and will continue to see, shall drive the digital transformation as we understand it. The following will the future forward scenarios of AI strategy becoming core to digital transformation:
AI’s growing entrenchment: This time, the scale and scope of the surge in attention to AI is much larger than before. For starters, the infrastructure speed, availability, and sheer scale has enabled bolder algorithms to tackle more ambitious problems. Not only is the hardware faster, sometimes augmented by specialized arrays of processors (e.g., GPUs), it is also available in the shape of cloud services , data farms and centers
Geography, societal Impact: AI adoption is reaching institutions outside of the industry. Lawyers will start to grapple with how laws should deal with autonomous vehicles; economists will study AI-driven technological unemployment; sociologists will study the impact of AI-human relationships. This is the world of the future and the new next.
Artificial intelligence will be democratized: As per the results of a recent Forrester study , it was revealed that 58 percent of professionals researching artificial intelligence ,only 12 percent are actually using an AI system. Since AI requires specialized skills or infrastructure to implement, Companies like Facebook have realized this and are already doing all they can to simplify the implementation of AI and make it more accessible. Cloud platforms like Google APIs, Microsoft Azure, AWS are allowing developers to create intelligent apps without having to set up or maintain any other infrastructure.
Niche AI will Grow: By all accounts, 2020 & beyond won’t be for large, general-purpose AI systems. Instead, there will be an explosion of specific, highly niche artificial intelligence adoption cases. These include autonomous vehicles (cars and drones), robotics, bots (consumer-orientated such as Amazon Echo , and industry specific AI (think finance, health, security etc.).
Continued Discourse on AI ethics, security & privacy: Most AI systems are immensely complex sponges that absorb data and process it at tremendous rates. The risks related to AI ethics, security and privacy are real and need to be addressed through consideration and consensus. Sure, it’s unlikely that these problems will be solved in 2020, but as long as the conversation around these topics continues, we can expect at least some headway.
Algorithm Economy: With massive data generation using flywheels, there will be an economy created for algorithms, like a marketplace for algorithms. The engineers, data scientists, organizations, etc. will be sharing algorithms for using the data to extract required information set.
Where is AI Heading in the Digital Road?While much of this is still rudimentary at the moment, we can expect sophisticated AI to significantly impact our everyday lives. Here are four ways AI might affect us in the future:
Humanizing AI: AI will grow beyond a “tool” to fill the role of “co-worker.” Most AI software is too hidden technologically to significantly change the daily experience for the average worker. They exist only in a back end with little interface with humans. But several AI companies combine advanced AI with automation and intelligent interfaces that drastically alter the day to day workflow for workers
Design Thinking & behavioral science in AI: We will witness Divergence from More Powerful Intelligence To More Creative Intelligence. There have already been attempts to make AI engage in creative efforts, such as artwork and music composition. we’ll see more and more artificial intelligence designing artificial intelligence, resulting in many mistakes, plenty of dead ends, and some astonishing successes.
Rise of Cyborgs: As augmented AI is already the mainstream thinking; the future might hold witness to perfect culmination of man-machine augmentation. AI augmented to humans, intelligently handling operations which human cannot do, using neural commands.
AI Oracle : AI might become so connected with every aspect of our lives, processing though every quanta of data from every perspective that it would perfectly know how to raise the overall standard of living for the human race. People would religiously follow its instructions (like we already follow GPS navigations) leading to leading to an equation of dependence closer to devotion.
The Final Word
Digital business transformation is the ultimate challenge in change management. It impacts not only industry structures and strategic positioning, but it affects all levels of an organization (every task, activity, process) and even its extended supply chain. Hence to brace Digital led disruption, one has to embrace AI-led strategy. Organizations that deploy AI strategically will ultimately enjoy advantages ranging from cost reductions and higher productivity to top-line benefits such as increasing revenue and profits, richer customer experiences, and working-capital optimization.
( AIQRATE, A bespoke global AI advisory and consulting firm. A first in its genre, AIQRATE provides strategic AI advisory services and consulting offerings across multiple business segments to enable clients navigate their AI powered transformation, innovation & revival journey and accentuate their decision making and business performance.
AIQRATE works closely with Boards, CXOs and Senior leaders advising them on their Analytics to AI journey construct with the art of possible AI roadmap blended with a jump start approach to AI driven transformation with AI@scale centric strategy; AIQRATE also consults on embedding AI as core to business strategy within business processes & functions and augmenting the overall decision-making capabilities. Our bespoke AI advisory services focus on curating & designing building blocks of AI strategy, embed AI@scale interventions and create AI powered organizations.
AIQRATE’s path breaking 50+ AI consulting frameworks, methodologies, primers, toolkits and playbooks crafted by seasoned and proven AI strategy advisors enable Indian & global enterprises, GCCs, Startups, SMBs, VC/PE firms, and Academic Institutions enhance business performance & ROI and accelerate decision making capability. AIQRATE also provide advisory support to Technology companies, business consulting firms, GCCs, AI pure play outfits on curating discerning AI capabilities, solutions along with differentiated GTM and market development strategies.
Visit www.aiqrate.ai to experience our AI advisory services & consulting offerings. Follow us on Linkedin | Facebook | YouTube | Twitter | Instagram )
Related Posts
AIQRATIONS
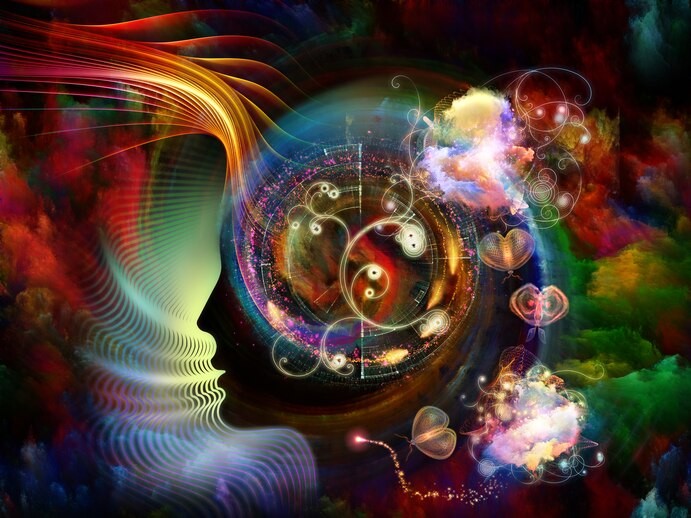
Business Decisions Transformation : AI meets Behavioral Sciences : New Strategic Approach
Add Your Heading Text Here
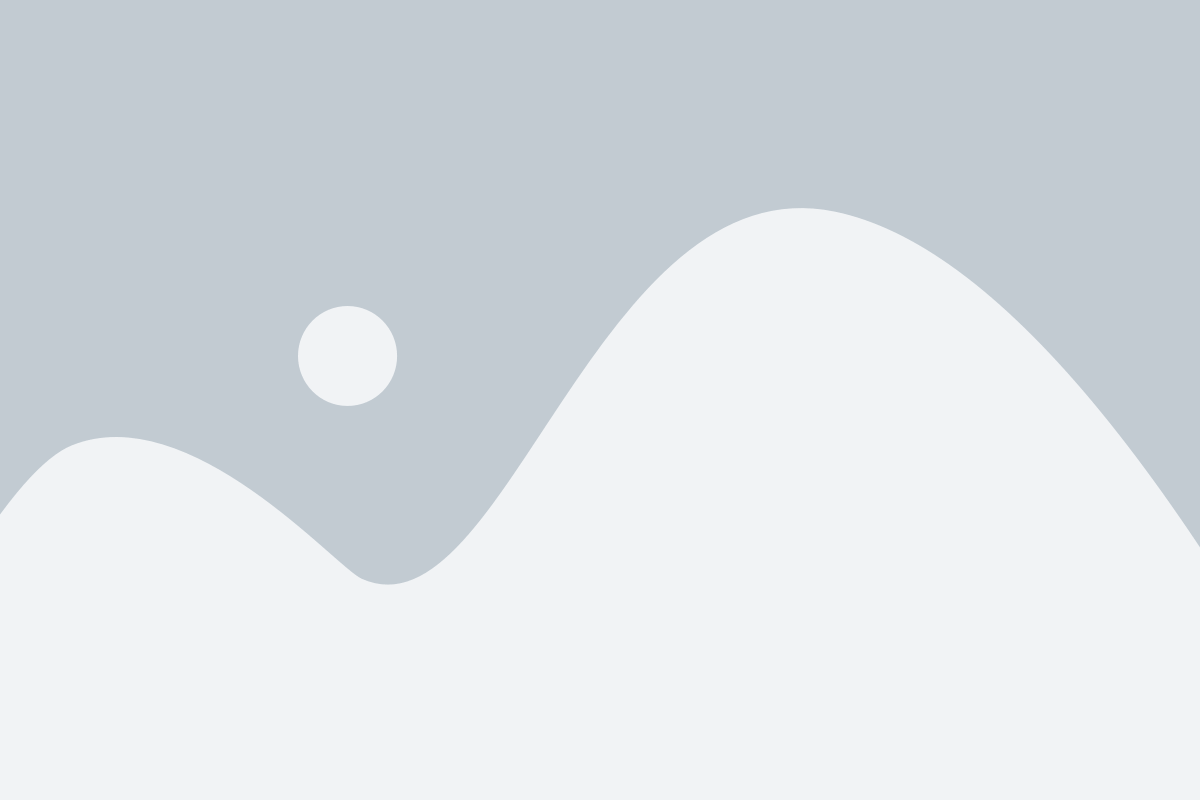
Ascendancy of artificial intelligence (AI) revolution has been made possible by the machine enabled , ensemble configured algorithm revolution. The machine learning algorithms researchers have been developing for decades, when cleverly applied to today’s web-scale data sets, can yield surprisingly good forms of intelligence. For instance, the United States Postal Service has long used neural network models to automatically read handwritten zip code digits. Today’s deep learning neural networks can be trained on millions of electronic photographs to identify faces, and similar algorithms may increasingly be used to navigate automobiles and identify tumors in X-rays.
But current AI technologies are a collection of big data-driven point solutions, and algorithms are reliable only to the extent that the data used to train them is complete and appropriate. One-off or unforeseen events that humans can navigate using common sense can lead algorithms to yield nonsensical outputs.
Design thinking is defined as human-centric design that builds upon the deep understanding of our users (e.g., their tendencies, propensities, inclinations, behaviors) to generate ideas, build prototypes, share what you’ve made, embrace the art of failure (i.e., fail fast but learn faster) and eventually put your innovative solution out into the world. And fortunately for us humans (who really excel at human-centric things), there is a tight correlation between the design thinking and artificial intelligence.
Artificial intelligence technologies could reshape economies and societies, but more powerful algorithms do not automatically yield improved business or societal outcomes. Human-centered design thinking can help organizations get the most out of cognitive technologies.
Divergence from More Powerful Intelligence To More Creative Intelligence
Whilst algorithms can automate many routine tasks, the narrow nature of data-driven AI implies that many other tasks will require human involvement. In such cases, algorithms should be viewed as cognitive tools capable of augmenting human capabilities and integrated into systems designed to go with the grain of human—and organizational—psychology. We don’t want to ascribe to AI algorithms more intelligence than is really there. They may be smarter than humans at certain tasks, but more generally we need to make sure algorithms are designed to help us, not do an end run around our common sense.
Design Thinking at Enterprise Premise
Although cognitive design thinking is in its early stages in many enterprises, the implications are evident. Eschewing versus embracing design thinking can mean the difference between failure and success. For example, a legacy company that believes photography hinges on printing photographs could falter compared to an internet startup that realizes many customers would prefer to share images online without making prints, and embraces technology that learns faces and automatically generates albums to enhance their experience.
To make design thinking meaningful for consumers, companies can benefit from carefully selecting use cases and the information they feed into AI technologies. In determining which available data is likely to generate desired results, enterprises can start by focusing on their individual problems and business cases, create cognitive centers of excellence, adopt common platforms to digest and analyze data, enforce strong data governance practices, and crowd source ideas from employees and customers alike.
In assessing what constitutes proper algorithmic design, organizations may confront ethical quandaries that expose them to potential risk. Unintended algorithmic bias can lead to exclusionary and even discriminatory practices. For example, facial recognition software trained on insufficiently diverse data sets may be largely incapable of recognizing individuals with different skin tones. This could cause problems in predictive policing, and even lead to misidentification of crime suspects. If the training data sets aren’t really that diverse, any face that deviates too much from the established norm will be harder to detect. Accordingly, across many fields, we can start thinking about how we create more inclusive code and employ inclusive coding practices.
CXO Strategy for Cognitive Design Thinking & Behavioral Science
CIOs can introduce cognitive design thinking to their organizations by first determining how it can address problems that conventional technologies alone cannot solve. The technology works with the right use cases, data, and people, but demonstrating value is not always simple. However, once CIOs have proof points that show the value of cognitive design thinking, they can scale them up over time.
CIOs benefit from working with business stakeholders to identify sources of value. It is also important to involve end users in the design and conception of algorithms used to automate or augment cognitive tasks. Make sure people understand the premise of the model so they can pragmatically balance algorithm results with other information.
Enterprise Behavioral Science – From Insights to Influencing Business Decisions
Every January, how many people do you know say that they want to resolve to save more, spend less, eat better, or exercise more? These admirable goals are often proclaimed with the best of intentions, but are rarely achieved. If people were purely logical, we would all be the healthiest versions of ourselves.
However, the truth is that humans are not 100% rational; we are emotional creatures that are not always predictable. Behavioral economics evolved from this recognition of human irrationality. Behavioral economics is a method of economic analysis that applies psychological insights into human behavior to explain economic decision-making.
Decision making is one of the central activities of business – hundreds of billions of decisions are made every day. Decision making sits at the heart of innovation, growth, and profitability, and is foundational to competitiveness. Despite this degree of importance, decision making is poorly understood, and badly supported by tools. A study by Bain & Company found that decision effectiveness is 95% correlated with companies’ financial performance.
Enterprise Behavioral Science is not only about understanding potential outcomes, but to completely change outcomes, and more specifically, change the way in which people behave. Behavioral Science tells us that to make a fundamental change in behavior that will affect the long-term outcome of a process, we must insert an inflection point.
As an example, you are a sales rep and two years ago your revenue was $1 million. Last year it was $1.1 million, and this year you expect $1.2 million in sales. The trend is clear, and your growth has been linear and predictable. However, there is a change in company leadership and your management has increased your quota to $2 million for next year. What is going to motivate you to almost double your revenues? The difference between expectations ($2 million) and reality ($1.2 million) is often referred to as the “behavioral gap” . When the behavioral gap is significant, an inflection point is needed to close that gap. The right incentive can initiate an inflection point and influence a change in behavior. Perhaps that incentive is an added bonus, President’s Club eligibility, a promotion, etc.
Cognitive Design Thinking – The New Indispensable Reskilling Avenue
Artificial intelligence, machine learning, analytics and mobile and cloud engineering will be the top technology areas where the need for re-skilling will be the highest.whilst there is a high probability that machine learning and artificial intelligence will play an important role in whatever job you hold in the future, there is one way to “future-proof” your career…embrace the power of design thinking & behavioral science.
In fact, integrating design thinking , behavioral science and artificial intelligence can give you “immense synergies ” that future-proof whatever career you decide to pursue. To meld these three disciplines together, one must:
Understand where and how artificial intelligence and behavioral science can impact your business initiatives. While you won’t need to write machine learning algorithms, business leaders do need to learn how to “Think like a data scientist” in order understand how AI can optimize key operational processes, reduce security and regulatory risks, uncover new monetization opportunities.
Understand how design thinking techniques, concepts and tools can create a more compelling and emphatic user experience with a “delightful” user engagement through superior insights into your customers’ usage objectives, operating environment and impediments to success.
Design thinking & Behavioral Science is a mindset. IT firms are trying to move up the curve. Higher-end services that companies can charge more is to provide value and for that you need to know that end-customers’ needs. For example, to provide value services to banking customers is to find out what the bank’s customer needs are in that country the banking client is based. Latent needs come from a design thinking philosophy where you observe customer data, patterns and provide a solution that the customer does not know. Therefore, Companies will hire design thinkers as they can predict what the consumer does not know and hence charge for the product/service from their clients. Idea in design thinking is to provide agile product creation or solutions.
Without Design Thinking & Behavioral Science, AI Will be Only an Incremental Value
Though organizations understand the opportunity that big data presents, many struggles to find a way to unlock its value and use it in tandem with design thinking – making “AI an colossal waste of time & money.” Only by combining quantitative insights gathered using AI, machine/deep learning, and qualitative research through behavioral science, and finally design thinking to uncover hidden patterns and leveraging it to understand what the customer would want, will we be able to paint a complete picture of the problem at hand, and help drive towards a solution that would create value for all stakeholders.
(AIQRATE, A bespoke global AI advisory and consulting firm. A first in its genre, AIQRATE provides strategic AI advisory services and consulting offerings across multiple business segments to enable clients navigate their AI powered transformation, innovation & revival journey and accentuate their decision making and business performance.
AIQRATE works closely with Boards, CXOs and Senior leaders advising them on their Analytics to AI journey construct with the art of possible roadmap blended with a jump start to AI driven transformation with AI@scale centric strategy followed by consulting them on embedding AI as core to business strategy within business functions and augmenting the decision-making capabilities with AI. Our bespoke AI advisory services focuses on curating & designing building blocks of AI strategy, embed AI@scale interventions and create AI powered organizations.
AIQRATE’s path breaking 50+ AI consulting frameworks, methodologies, primers, toolkits and playbooks crafted by seasoned and proven AI strategy advisors enable Indian & global enterprises, GCCs, Startups, SMBs, VC/PE firms, and Academic Institutions enhance business performance & ROI and accelerate decision making capability.
Visit www.aiqrate.ai to experience our AI advisory services & consulting offerings. Write to us :consult@aiqrate.ai . Follow us on Linkedin | Facebook | Twitter | Instagram )
Related Posts
AIQRATIONS
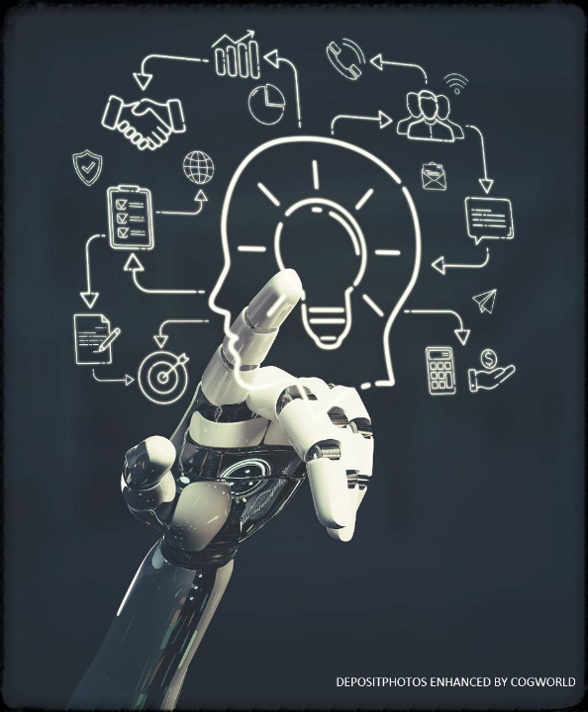
AI-Driven Disruption And Transformation: New Business Segments To Novel Market Opportunities
Add Your Heading Text Here
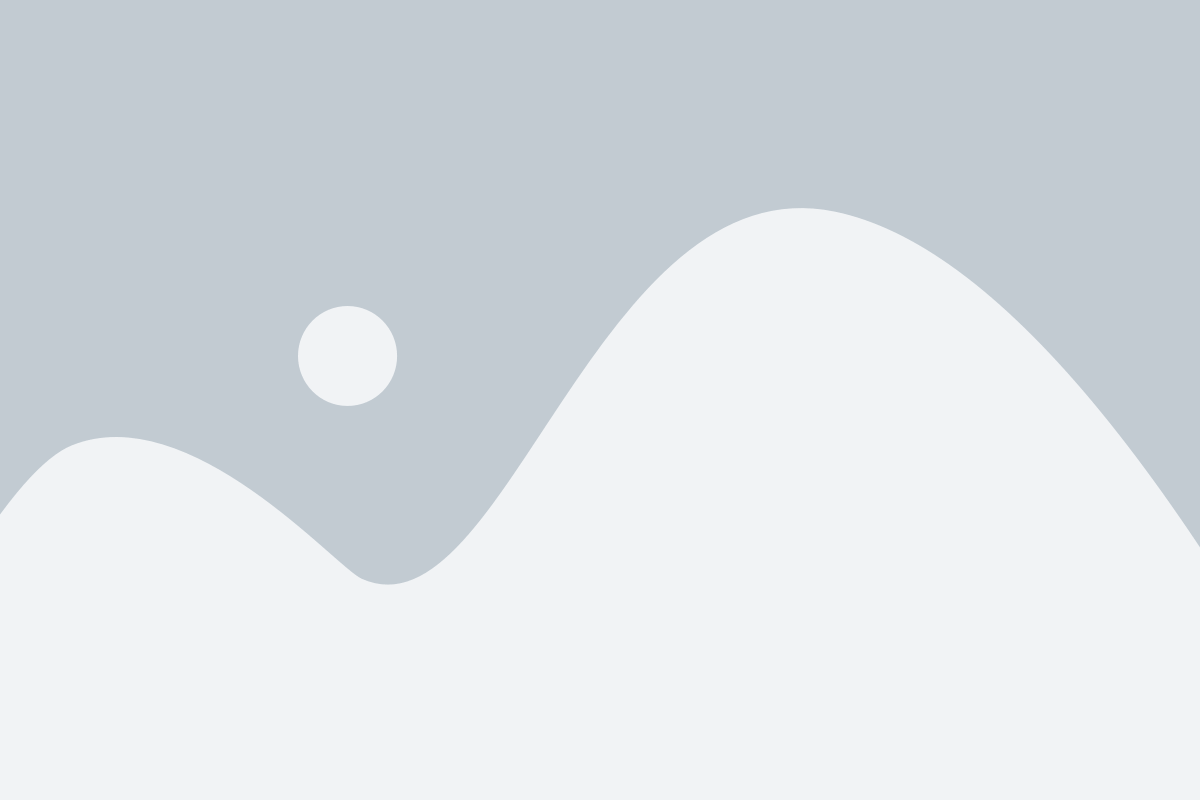
There’s little doubt that Artificial Intelligence (AI) is driving the decisive strategic elements in multiple industries, and algorithms are sitting at the core of every business model and in the enterprise DNA. Conventional wisdom, based on no small amount of research, holds that the rise of AI will usher radical, disruptive changes in the incumbent industries and sectors in the next five to 10 years.
Additionally, it’s never been a better time to launch an AI venture. Investments in AI-focused ventures have grown 1800% in just six years. The rationale behind these numbers comes, in part, from the fact that enterprises expect AI to enable them to move into new business segments, or to maintain a competitive edge in their industry.
Strategists believe this won’t come as a surprise to CXOs and decision-makers as acceleration of AI adoption and proliferation of smart, intuitive and ML algorithms spawn the creation of new industries and business segments and overall, trigger new opportunities for business monetization. However, a few questions loom large for CXOs: How will these new industries and business segments be created with AI? And, what strategic shifts can leadership make to monetize these new business opportunities?
The creation of new industries and business segments depends on dramatic advances in AI that can take a swift adoption journey to move from discovery to commercial application to a new industry. New industry segments around AI are in the making and are far from tapped. A cursory look at new age businesses: Micro-segmented, hyper-personalized online shopping platforms, GPS driven ride-sharing companies, recommendation-driven streaming channels, adaptive learning based EdTech companies, conversational AI-driven new and work scheduling are just a few of the imminent and visible examples. Yet a lot more can be done in this space.
AI adoption brings intentional efforts to adapt to this onslaught of algorithms and how it’s affecting customer and employee behavior. As algorithms become a permanent fixture in everyday life, organizations are forced to update legacy technology strategies and supporting methodologies to better reflect how the real world is evolving. And the need to do so is becoming increasingly obligatory.
On the other side, traditional and incumbent enterprises are reverse engineering investments, processes, and systems to better align with how markets are changing. Because it’s focusing on customer behavior, AI is actually in its own way, making businesses more human. As such, Artificial Intelligence is not specifically about technology, it’s empowered by it. Without an end in mind, self-learning algorithms continually seek out how to use technology in ways that improve customer experiences and relationships. It also represents an effort that introduces new models for business and, equally, creates a way of staying in business as customers become increasingly aware and selective.
Today, AI expertise is focused more on developing commercial applications that optimize efficiencies in existing industries and is focused less on developing patented algorithms that could lead to new industries. These efficiencies are accelerating the sectoral consolidation and convergence, and are less about new industry creation.
However, AI’s most potent, long-term economic use may just be to augment the discovery and pursuit of solving large, complex and unresolved problems that could be the foundations of new industry segments. Enterprises have started realizing the significance of having a long-term strategic interest and investments in using AI in this way. Yet few of the above mentioned examples are testimony to AI triggering new industry segments and business opportunities. The real winners in the algorithm-driven economy will be business leaders that align their strategies to augment AI expertise from ground zero, keep a continuous tab on blockbuster algorithms, and redefine new business segments that enable monetization of new opportunities.
AI has immense potential to jumpstart the creation of new industries and the disruption of existing ones. The curation of this as a strategic roadmap for business leaders is far from easy, but it carries great rewards for businesses. It takes a village to bring about change, and it also takes the spark and perseverance of an AI strategist to spot important trends and create a sense of urgency around new possibilities.
Related Posts
AIQRATIONS
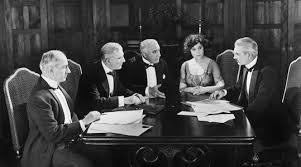
AI led strategy for business transformation : A guided approach for CXOs
Add Your Heading Text Here
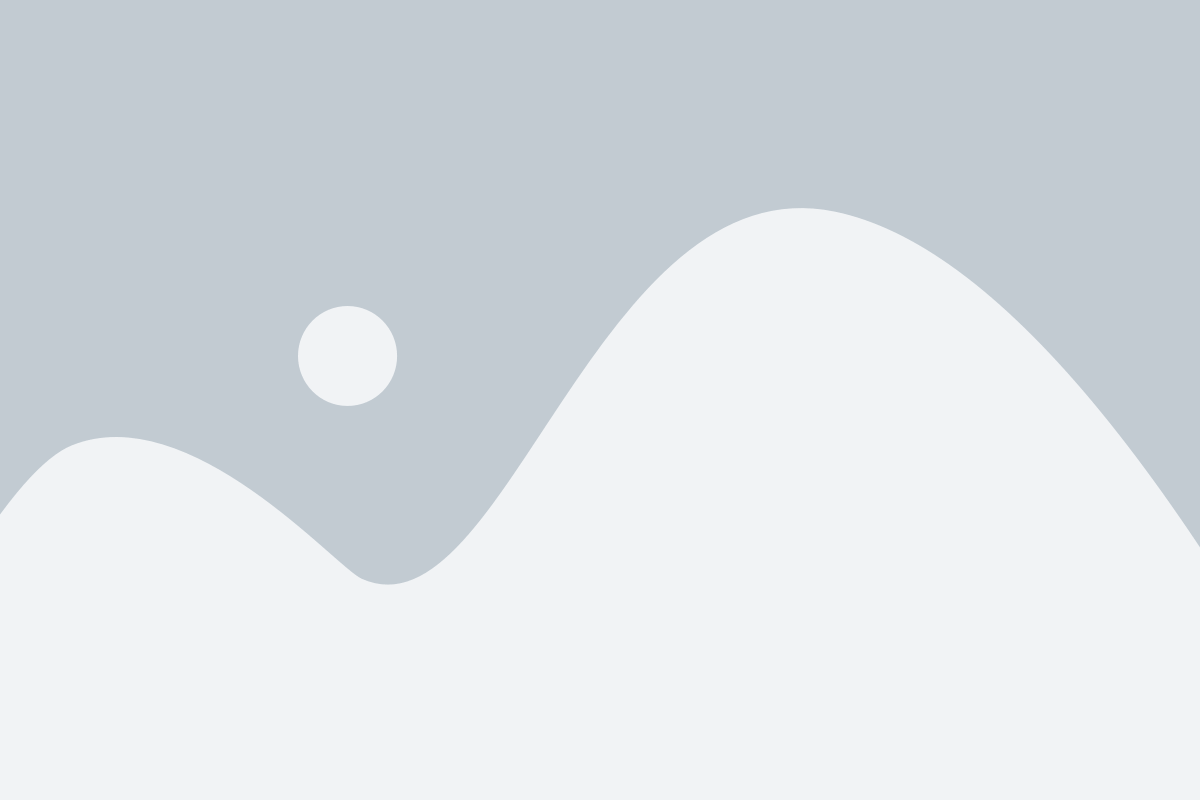
Business transformation programs have long focused on productivity enhancements —taking a “better, faster, cheaper” approach to how the enterprise works. And for good reason: disciplined efforts can boost productivity as well as accountability, transparency, execution, and the pace of decision making. When it comes to delivering fast results to the bottom line, it’s a proven recipe that works.
The problem is, it’s no longer enough. Artificial Intelligence enabled disruption are upending industry after industry, pressuring incumbent companies not only to scratch out stronger financial returns but also to remake who and what they are as enterprises.
Doing the first is hard enough. Tackling the second—changing what your company is and does—requires understanding where the value is shifting in your industry (and in others), spotting opportunities in the inflection points, and taking purposeful actions to seize them. The prospect of doing both jobs at once is sobering.
How realistic is it to think your company can pull it off? The good news is that AIQRATE can demonstrate that it’s entirely possible for organizations to ramp up their bottom-line performance even as they secure game-changing portfolio wins that redefine what a company is and does. What’s more, AL led transformations that focus on the organization’s performance and portfolio appear to load the dice in favor of transformation results. By developing these two complementary sets of muscles, companies can aspire to flex them in a coordinated way, using performance improvements to carry them to the next set of portfolio moves, which in turn creates momentum propelling the company to the next level.
Strategic Steps towards AI led Transformation:
This aspect covers AI led “portfolio-related” moves. The first is active resource reallocation towards building AI led transformation units, which I define as the company shifting more than 20 percent of its capital spending across its businesses or markets over ten years. Such firms create 50 percent more value than counterparts that shift resources at a slower clip.
Meanwhile, a big move in programmatic M&A driven by AI led spot trending—the type of deal making that produces more reliable performance boosts than any other—requires the company to execute at least one deal per year, cumulatively amounting to more than 30 percent of a company’s market capitalization over ten years, and with no single deal being more than 30 percent of its market capitalization.
Making big moves tends to reduce the risk profile and adds more upside than downside. The way I explain this to senior executives is that when you’re parked on the side of a volcano, staying put is your riskiest move.
AI led Transformations that go ‘all in’ by addressing both a company’s performance and its portfolio yield the highest odds.
The implication of these transformation stories is clear: approaches that go all in by addressing both a company’s performance and its portfolio yield the highest odds of lasting improvement. Over the course of a decade, companies that followed this path nearly tripled their likelihood of reaching the top quin tile of the AI transformation power curve relative to the average company in the middle.
Play to win with AI
Life would be simpler if story ended here. However, you’re not operating in a competitive vacuum. As I described earlier, other forces influence your odds of success in significant ways—in particular, how your industry is performing. Research studies have indicated that companies facing competitive headwinds would face longer odds of success than those with tailwinds.
Companies that combined big performance moves with big portfolio moves (including capital expenditures, when not the only portfolio move employed) saw a big lift in their odds. Life is still challenging for these companies—their net odds are dead even—yet this is superior to the negative odds of the other situations.
Winning thru competitive advantage with AI
In an improving industry, the returns to performance improvement are amplified massively. This runs contrary to the very human tendency of equating performance transformations with turnaround cases
The takeaway from all this is that two big rules stand out as commonly and powerfully true whatever your context: first, get moving with AI , don’t be static; second, go all in if you can with AI led transformation programs —it’s always the best outcome (and also the rarest).
Running the AI led transformation program
In my experience, the companies that are most successful at transforming themselves with AI ,sequence their moves so that the rapid lift of performance improvement provides oxygen and confidence for big moves in M&A, capital investment, and resource reallocation. And when the right portfolio moves aren’t immediately available or aren’t clear, the improved performance helps buy a company time until the strategy can catch up.
To illustrate this point, consider the anecdote about Apple that Professor Richard Rumelt describes in his book, Good Strategy/Bad Strategy. It was the late 1990s; Steve Jobs had returned to Apple and cleaned house through productivity-improving cutbacks and a radically simplified product line. Apple was much stronger, yet it remained a niche player in its industry. When Rumelt asked Jobs how he planned to address this fact, Jobs just smiled and said, ‘I am going to wait for the next big thing.’
While no one can guarantee that your “next big thing” will be an iPod-size breakthrough, there’s nothing stopping you from laying the groundwork for a successful AI led transformation. To see how prepared, you are for such an undertaking, ask yourself—and your team—the following five questions. I sincerely hope they provoke productive and transformative discussion among your team.
1.Where is the new business value chain that’s driven by AI
Achieving success with big, portfolio-related moves requires understanding where the business value flows in your business and why. The structural attractiveness of markets, and your position in them, can and does change over time. Ignore this and you might be shifting deck chairs on the Titanic. Meanwhile, to put this thinking into action, you must also view the company as an ever-changing portfolio. This represents a sea change for managers who are used to plodding, once-a-year strategy sessions that are more focused on “getting to yes” and on protecting turf than on debating real alternatives. Get high-powered decision-making algorithms to navigate you thru this transformation.
2. Put your money in building an AI led strategy
Only 10% of the US fortune 200 companies have AI led strategy; this is an impending strategic aspect that cannot be ignored. The dimensions of reimagining customer experience, building innovative products and services and transforming the businesses need to have an AI led strategy move by the CXOs
3.Are you ready for disruption?
Increasingly, incumbent organizations are getting to the pointy end of disruption, where they must accelerate the transition from legacy business models to new ones and even allow potentially cannibalizing businesses to flourish. Sometimes this requires a very deliberate two-speed approach where legacy assets are managed for cash while new businesses are nurtured for growth.
4.Will our company take this seriously?
Embracing AI led transformative change requires commitment, and gaining commitment requires a compelling change story that everyone in the company can embrace. Philips recognized this in 2011 when it launched its “Accelerate” program. Along with productivity improvements and portfolio changes (including a big pivot from electronics to health tech), the company shaped its change story around improving three billion lives annually by 2030, as part of a broader goal of making the world healthier and more sustainable through innovation. Massive thrust and investment was laid by Phillips leadership team on AI led transformation programs.
5.Is the leadership ready for the transformation?
Leading a successful AI led transformation requires a lot more than just picking the right moves and seeing them through. Among your other priorities: build momentum, engage your workforce, and make the change personal for yourself and your company. All of this means developing new leadership skills and ways of working, while embracing a level of commitment as a leader that may be unprecedented for you.
In the end, AI led strategy for transformation is a process and start of a journey …. embrace it or feel the heat of leaving behind. The new age competition is agile and nimble and AI led transformation strategy is a right move to thwart the competition.
Related Posts
AIQRATIONS

AI For CXOs — Redefining The Future Of Leadership In The AI Era
Add Your Heading Text Here
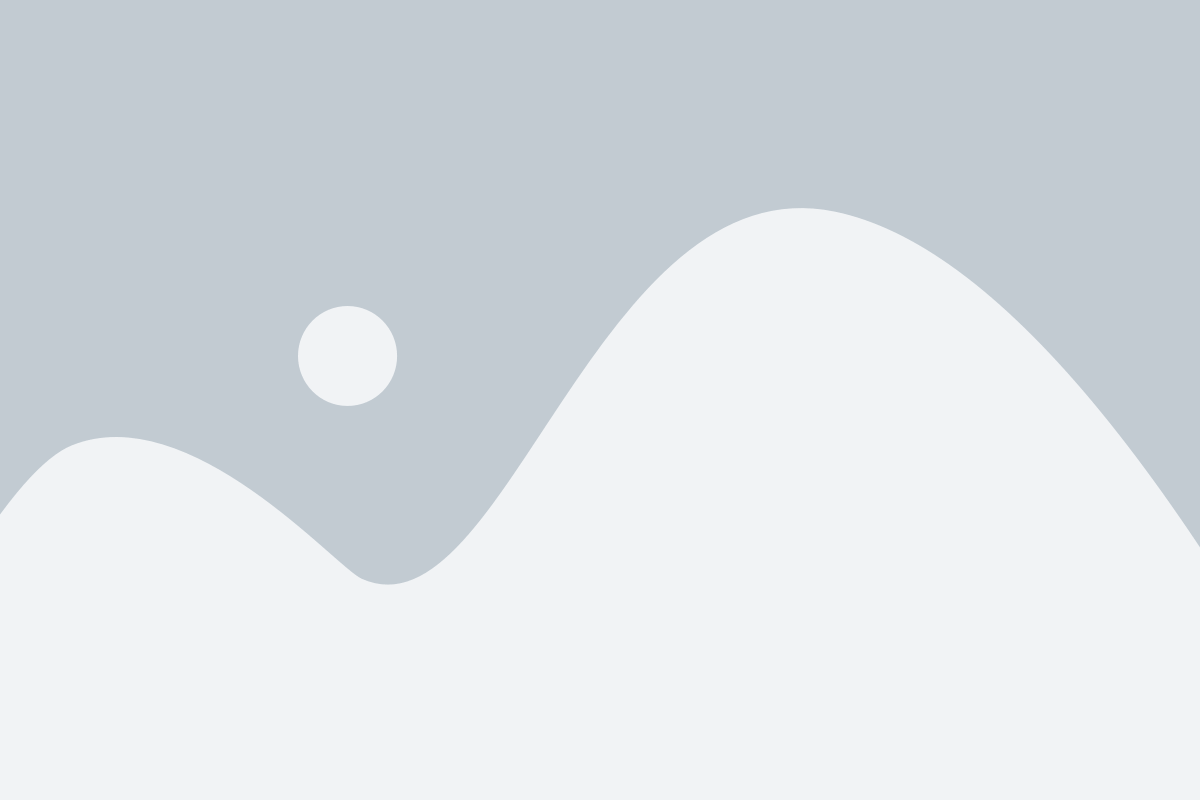
Artificial intelligence is getting ubiquitous and is transforming organizations globally. AI is no longer just a technology. It is now one of the most important lenses that business leaders need to look through to identify new business models, new sources of revenue and bring in critical efficiencies in how they do businesses.
Artificial intelligence has quickly moved beyond bits and pieces of topical experiments in the innovation lab. AI needs to be weaved into the fabric of business. Indeed, if you see the companies leading with AI today, one of the common denominators is that there is a strong executive focus around artificial intelligence. AI transformation can be successful when there is a strong mandate coming from the top and leaders make it a strategic priority for their enterprise.
Given AI’s importance to the enterprise, it is fair to say that AI will not only shape the future of the enterprise, but also the future for those that lead the enterprise mandate on artificial intelligence.
Curiosity and Adaptability
To lead with AI in the enterprise, top executives will need to demonstrate high levels of adaptability and agility. Leaders need to develop a mindset to harness the strategic shifts that AI will bring in an increasingly dynamic landscape of business – which will require extreme agility. Leaders that succeed in this AI era will need to be able to build capable, agile teams that can rapidly take cognizance of how AI can be a game changer in their area of business and react accordingly. Agile teams across the enterprise will be a cornerstone of better leadership in this age of AI.
Leading with AI will also require leaders to be increasingly curious. The paradigm of conducting business in this new world is evolving faster than ever. Leaders will need to ensure that they are on top of the recent developments in the dual realms of business and technology. This requires CXOs to be positively curious and constantly on the lookout for game changing solutions that can have a discernible impact on their topline and bottom-line.
Clarity of Vision
Leadership in the AI era will be strongly characterized by the strength and clarity with which leaders communicate their vision. Leaders with an inherently strong sense of purpose and an eye for details will be forged as organizations globally witness AI transformation.
It is not only important for those that lead with AI to have a clear vision. It is equally important to maintain a razor sharp focus on the execution aspect. When it comes to scaling artificial intelligence in the organization, the devil is very often in the details – the data and algorithms that disrupt existing business processes. For leaders to be successful, they must remain attentive to the trifecta of factors – completeness of their vision for AI transformation, communication of said vision to relevant stakeholders and monitoring the entire execution process. While doing so, it is important to remain agile and flexible as mentioned in my earlier section – in order to be aware of possible business landscape shifts on the horizon.
Engage with High EQ
AI transformation can often seem to be all about hard numbers and complex algorithms. However, leaders need to also infuse the human element to succeed in their efforts to deliver AI @ Scale. The third key for top executives to lead in the age of AI is to ensure that they marry high IQs with equally or perhaps higher levels of EQ.
Why is this so very important? Given the state of this technology today, it is important that we build systems that are completely free of bias and are fair in how they arrive at strategic and tactical decisions. AI learns from the data that it is provided and hence it is important to ensure that the data it is fed is free from bias – which requires a human aspect. Secondly, AI causes severe consternation among the working population – with fears of job loss abounding. It is important to ensure that these irrational fears of an ‘AI Takeover’ are effectively abated. For AI to be successful, it is important that both types of intelligence – artificial and human – symbiotically coexist to deliver transformational results.
AI is undoubtedly going to become one of the sources of lasting competitive advantage for enterprises. According to research, 4 out of 5 C-level executives believe that their future business strategy will be informed through opportunities made available by AI technology. This requires a leadership mindset that is AI-first and can spot opportunities for artificial intelligence solutions to exploit. By democratizing AI solutions across the organization, enterprises can ensure that their future leadership continues to prioritize the deployment of this technology in use cases where they can deliver maximum impact.
Related Posts
AIQRATIONS
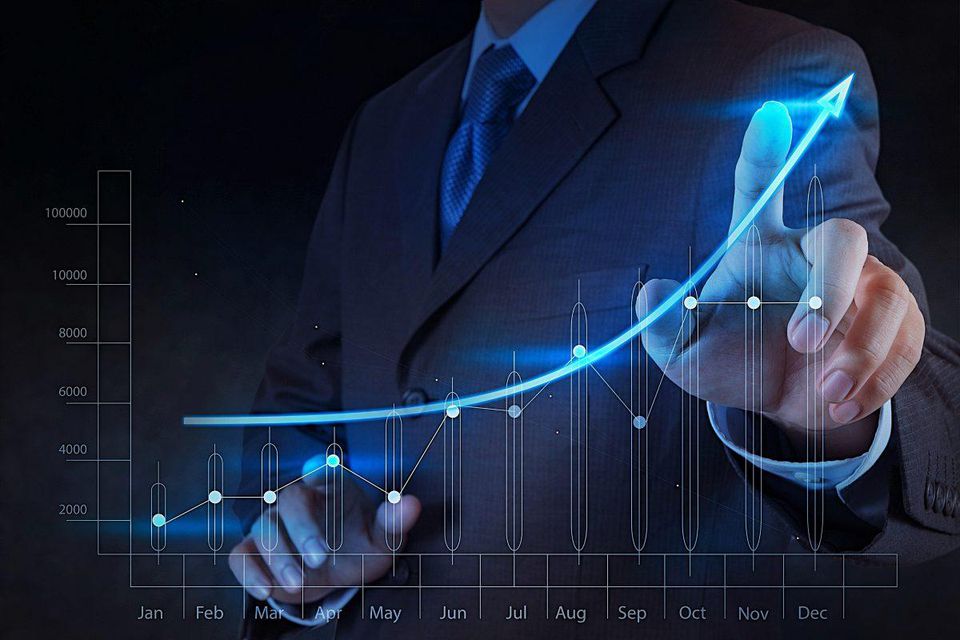
Delivering Business Value Through AI To Impact Top Line, Bottom Line And Unlock ROI
Add Your Heading Text Here
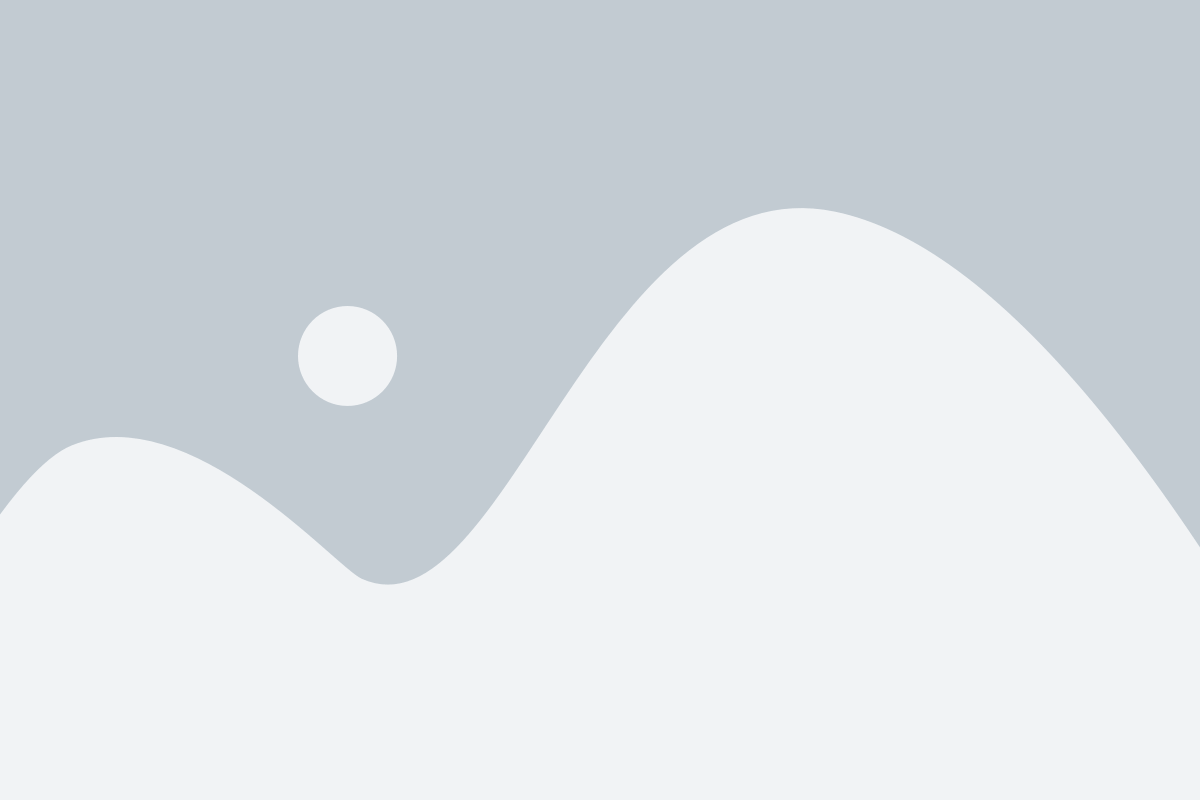
As is the case with investments in any other area of technology, AI needs to deliver demonstrable impact to business top line and bottom line. In today’s competitive landscape of business, enterprises are expected to measure the incremental ROI for every expense and every investment made – technology or otherwise. The case of Artificial Intelligence is no different. It is critical that technology and business leaders demand ROI impact for this technology in order to foster its growth and justify its proliferation in business.
To be sure, there are two key areas where Artificial Intelligence can contribute immense value; Increasing top line figures by unlocking new revenue streams and improving the bottom line through efficiencies in operations. Needless to say, top line gains eventually percolate their way into showcasing bottom line improvement – but for the purpose of this post, we’ll refer to bottom line impact as areas where AI brings in cost efficiencies by helping organizations reduce their overall cost of operations.
Artificial Intelligence driven applications can have a discernible impact on business top lines and bottom lines and help organizations unlock ROI from their implementation.
AI-Powered Topline Growth
Artificial Intelligence-led applications have huge potential to add to top line revenue growth for any organization. Typical AI interventions for this purpose range from improving the effectiveness of marketing and sales functions, improving customer loyalty through laser-guided customer experience initiatives and direct and indirect data monetization.
New Revenue Streams Enabled by Data Monetization:
Business leaders need to realize AI’s potential to unlock new sources of revenue in addition to improving customer targeting and loyalty. One of these ways is data monetization. What is data monetization? Simply put, data monetization refers to the act of generating measurable economic benefits from available data resources. According to Gartner, there are two distinct ways in which business leaders can monetize data. The most commonly seen method from the two is Direct Monetization. The way to realize value from this avenue involves directly adding AI as a feature to existing offerings. Companies like Nielsen, D&B, TransUnion, Equifax, Acxiom, Bloomberg and IMS run their business on licensing their data in a raw format or as part of their application infrastructure. With emerging Data-as-a-Service models and the application for direct insight delivery through intelligent application of AI, direct data monetization is simpler than ever. By wrapping insights alongside the data source, vendors can create a symbiotically powerful exchange of information for both the buyers and sellers of data. On the other hand, Indirect Monetization involves embedding AI into traditional business processes with a focus on driving increased revenue. A popular example of this is corporations who come out with branded, paid-for reports based on the data they own. For instance, professional services companies such as Aon, Deloitte, McKinsey, etc., regularly bring forward insightful industry and function-specific reports based on the data they collect as part of their consulting assignments.
Enabling Intelligent Marketing and Sales
Many of the most prominently cited successes of AI-enabled business transformation comes from the marketing and sales arena. Sales and marketing are constantly on the forefront for exciting inventions in AI since they contribute directly to top line growth. Use cases discovered in this arena span social media sentiment mining, programmatic selection of advertising properties, measuring effectiveness of marketing programs, ensuring customer loyalty and intelligent sales recommendations. AI also has huge potential to drive businesses to explore and exploit eCommerce platforms as a credible channel for sales and to help drive the digital agenda forward. Available tools are helping drive better customer conversions on eCommerce properties – by analysing the digital footprints (clickstream, etc.) of prospective customers, persuading them into making a purchase. In such use cases, AI helps improve personalization at the point-of-purchase, improve conversions and reduce cart abandonment. Marketing and sales use cases today are pretty much at the epicentre of an AI disruption and business leaders need to uncover more use cases that can help drive effective top line growth.
AI Redefining Customer Experience
Customers are the epicentre of every successful organization. Today, we live in times where customers have numerous competitor options to choose from while the switching costs for customers are increasingly lower. Given this scenario, for businesses to win with their customers they need to have a smarter approach to customer experience management.
We have progressed well beyond pre-programmed bots addressing frequently asked questions. AI-enabled systems today go further and provide customers with personalized guidance. The travel and hospitality industries, for instance, are ripe for such disruptive innovations. In many cases, we see chatbots that help customers identify and recommend interesting activities and events that tourists can avail. When applied with human creativity, AI can ensure this redefined understanding of customer experience, while maintaining a lower cost of delivering that experience.
AI for Improving Bottom Line Performance
At an operational level as well, AI can help organizations run a more efficient business. For instance, corporations across industries need to find innovative and fail-safe ways to reduce the cost of manufacturing as well as capping their outlay on the supply chain network. AI-centric solutions can drive down the turnaround time for talent acquisition and transform other facets of the Human Capital function too.
AI Driving Operational Efficiencies
Traditional manufacturing processes are now increasingly augmented by robotics and AI. These technologies are bringing increasing sophistication to the manufacturing process. The successes combine human and machine intelligence making AI-augmented manufacturing a pervasive phenomenon. Today, business leaders in the Industry 4.0 generation need to seriously consider planning a hybrid labour force powered by human and artificial intelligence – and ensure that the two coexist by implementing the right policies and plans in place.
Smarter Supply Chains Powered by AI
Orchestrating a leaner, more predictable supply chain is ripe for an AI-led disruption. We are witnessing not just new products and categories but also new formats of retailers proliferating the industry. This varied portfolio of offerings and channels requires corporations to manage their outlay efficiently on the overall network responsible for the network that manages the entire process from procurement and assembly to stocking and last mile delivery. Multiple use cases exist that leverage multi-source data from internal and external repositories, combining them with information from IOT sensors. AI algorithms are then applied over this combined data infrastructure with the objective of helping business users quickly identify possible weaknesses/flaws in the process such as delays and possible shortages. Business leaders are constantly on the lookout for solutions that can directly lift their bottom line by bringing in more intelligence and automation to their supply chain networks – thus unlocking savings for their businesses.
An Artificial Facelift for the Human Resources Function
The human resources function has historically been considered a cost-center in organizations. In addition to bringing down the costs associated with talent acquisition and management – AI would also help HR teams become leaner, more organized and reduce the turnaround time for talent acquisition. AI interventions are being seen in the areas of employee engagement and attrition management, but some of the most exciting use cases come from the talent acquisition area within the HR function. Multiple organizations are already working on solutions that can eliminate the need for HR staff to scan through each job application individually. By using AI intelligently, talent acquisition teams can determine the framework conditions for a job on offer and leave the creation of assessment tasks to Artificial Intelligence-powered systems. The AI-empowered system can then communicate the evaluation results and recommend the most suitable candidates for further interview rounds.
One of the key reasons why AI is in vogue today is the demonstrable ROI impact that it promises to bring to business processes. With greater computational power and more data, AI has become more practicable than before, but what will sustain its growth is how much incremental value it can eventually unlock for businesses across the globe and power new revenue models for businesses to tap into. It is critical that business and technology leaders earnestly kick off discussions around how to justify the impact of AI and mark down the key metrics that will be used to measure it. Partners and service providers too need to stay on top of finding ways to showcase measurable improvements that their software or services can bring to technology buyers. This will enable the entire AI ecosystem to flourish.
Related Posts
AIQRATIONS
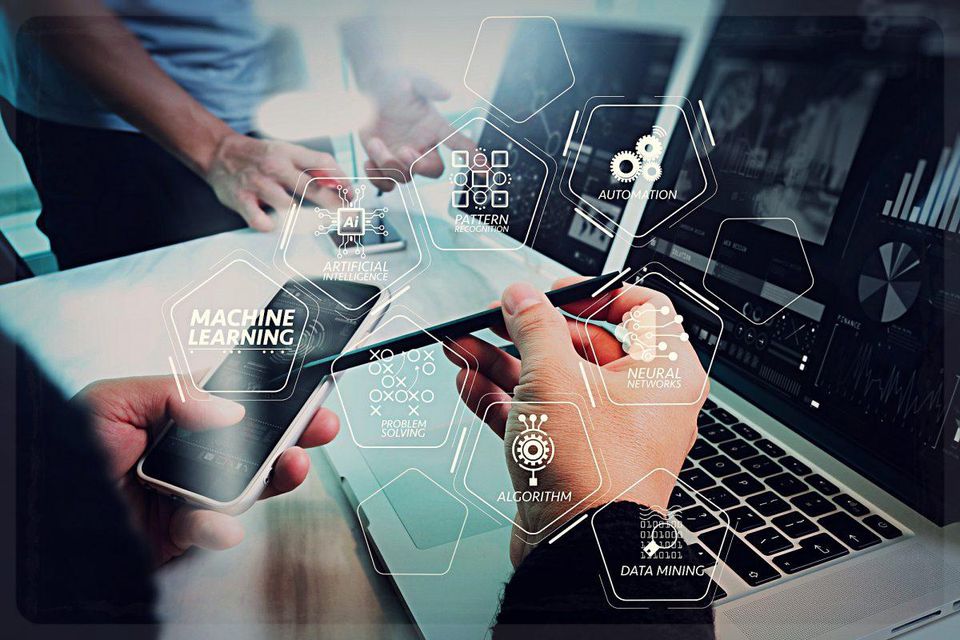
Reimagining Enterprise Decision-Making With Artificial Intelligence
Add Your Heading Text Here
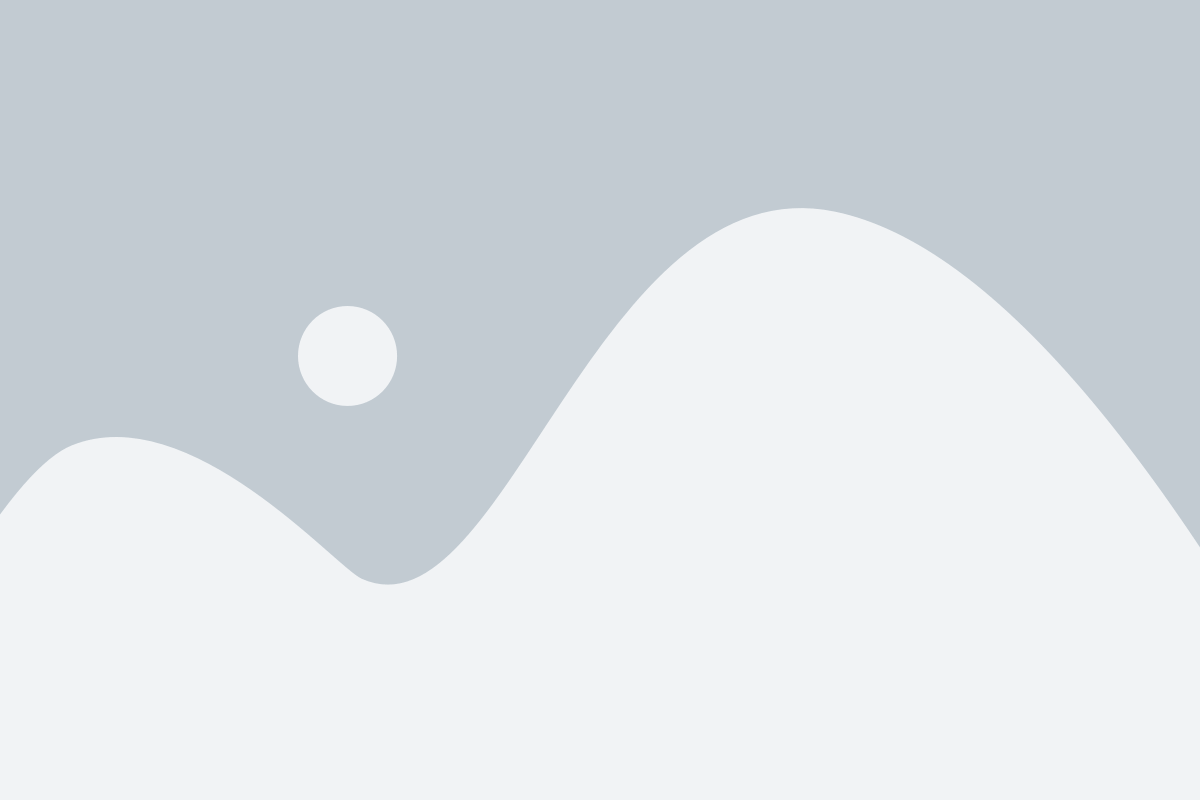
Artificial Intelligence will deliver revolutionary impact on how enterprises make decisions today. In the last few years alone, we have rapidly moved beyond heuristics-based decision-making to analytics-driven decision-support. In the VUCA phase, businesses globally are now pivoting to an AI-led, algorithm-augmented style of decision-making. With huge computing power and ever-increasing data storage and analytics prowess, we are entering a new paradigm, a probable and interesting scenario wherein, Artificial Intelligence will play a huge role in augmenting human intelligence and enabling decision-making with complete autonomy. The big hope is that this new paradigm will not only reduce human biases and errors that are common with heuristic decisions, but also reduce the time involved in making these critical decisions.
Here, I’ll attempt to focus on how we moved from simpler data driven decision-support to AI-powered decisions. The evolution of this technology has been breathtaking to behold and just might provide clues as to what we can expect in the future. Further, I’ll cover a few critical aspects that need to be inculcated by organizations on the AI transformation journey, and provide a few insightful cues that will make this journey exciting and fruitful.
Transformation of Decision-Making: From Analytics to AI
First, let us look at how we got here. Some truly pathbreaking events happened along the way while we were trying to make more accurate business decisions, leading us to reimagine how decisions will be made in the enterprise.
Organizations are Becoming Math Houses
With data deluge and digital detonation, combined with the appreciation of the fact that robust analytical capabilities lead to more informed decisions, we are witnessing AI savvy organizations rapidly maturing into ‘math houses.’ Data science – the ability to extract meaningful insights out of data has become de rigueur. Why? Because we now know that data, when seen in isolation, is inherently dumb. It is the ability to process this data and identify patterns and anomalies – using sophisticated algorithms and ensemble techniques – that makes all the difference. These self-intuitive algorithms are where real value resides – as they define the intelligence required to uncover insights and make smart recommendations. Organizations today are evolving into algorithm factories. There is a real understanding today that by enabling continuous advancement in mathematical algorithms, we can deliver consistent decisions based on prescribed as well as evolving business rules.
It is now an established reality that companies with robust mathematical capabilities possess a huge advantage over those that don’t. Indeed, it’s this math-house orientation that separates companies like Amazon and Google from the ones they leave in their wake, with their ability to understand their customers better, identify anomalies and recognize key patterns.
AI: From Predictive to Prescriptive
We saw a similar evolution in the age of analytics – wherein the science and value veered from descriptive analytics, providing diagnostics of past events to prescriptive analytics, helping see and shape the future. We are seeing a similar evolution in how AI gets leveraged in the enterprise and where its maximum value lies.
In early implementations, it was common to see AI as just a tool to predict and forecast future conditions, while accounting for the dynamism seen in the external environment. Today, AI-enabled decision-making is more prescriptive, with AI providing enterprises not just a look into the future, but also key diagnostics and suggestions on potential decision options and their payoffs. Such evolved applications of AI can help businesses make decisions that can potentially exploit more business opportunities, while averting potential threats much earlier.
Mr. Algorithm to Drive Decision Making
The culmination of this AI-era advancement would be the introduction of smart algorithms in every walk of life and business. Algorithms will become further mainstream leading to what will be the most sweeping business change since the industrial revolution. Organizations – those that already aren’t – will start developing a suite of algorithmic IP’s that will de-bias most enterprise decisions.
If Mr. Algorithm is going to drive most enterprise decisions of tomorrow, we need to create some checks and balances to ensure that it does not go awry. It is more critical today than ever before that the algorithmic suite developed by enterprises has a strong grounding in ethics and can handle situations appropriately for which explicit training may not have been provided.
How to Enable this AI Era of Change
Ushering into an AI-centric era of decision-making will require organizational transformation from business, cultural and technical standpoints. The following facets will be the enablers of this change:
Developing an Engineering Mindset
Instrumenting AI in the enterprise requires a combination of data scientists and computer scientists. As AI matures in the enterprise, the users, use cases and data will increase exponentially. To deliver impactful AI applications, scale and extensibility is critically important. This is where having an engineering mindset comes in. Imbibing an engineering mindset will help standardize the use of these applications while ensuring that they are scalable and extensible.
Learning, Unlearning, Relearning
The other critical aspect to a culture where AI can thrive is creating an environment supporting continuous unlearning and relearning. AI can succeed if the people developing and operating it are rewarded for continuous experimentation and exploration. And just like AI, people should be encouraged to incorporate feedback loops and learn continuously. As technology matures it’s important that the existing workforce keeps up. For one, it’s critical that the knowledge of algorithm theory, applied math alongside training on AI library and developer tools, is imparted into the workforce – and is continuously updated to reflect new breakthroughs in this space.
Embedding Design-Thinking and Behavioral Science at the Center of this Transformation
Finally, given the nature of AI applications, it’s critical that they are consumed voraciously. User input very often activates the learning cycles of artificial intelligence applications. To ensure high usage of these applications, it’s very important that we put the user at the center while designing these applications. This is where the application of behavioral sciences and human-centered design will deliver impact. By imparting empathy in these applications for the user, we will be able to design better and more useful AI applications.
As we augment decision-making with algorithmic, AI-centered systems and platforms – the big expectation is that they will bring untold efficiencies in terms of cost, alongside improvement in the speed and quality with which decisions get made. It’s time to reimagine and deliver on enterprise decision-making that is increasingly shaped through artificial intelligence. These aspects – how the AI is progressing and how to exploit its potential are of paramount importance to keep in mind for an AI transformation.
Related Posts
AIQRATIONS
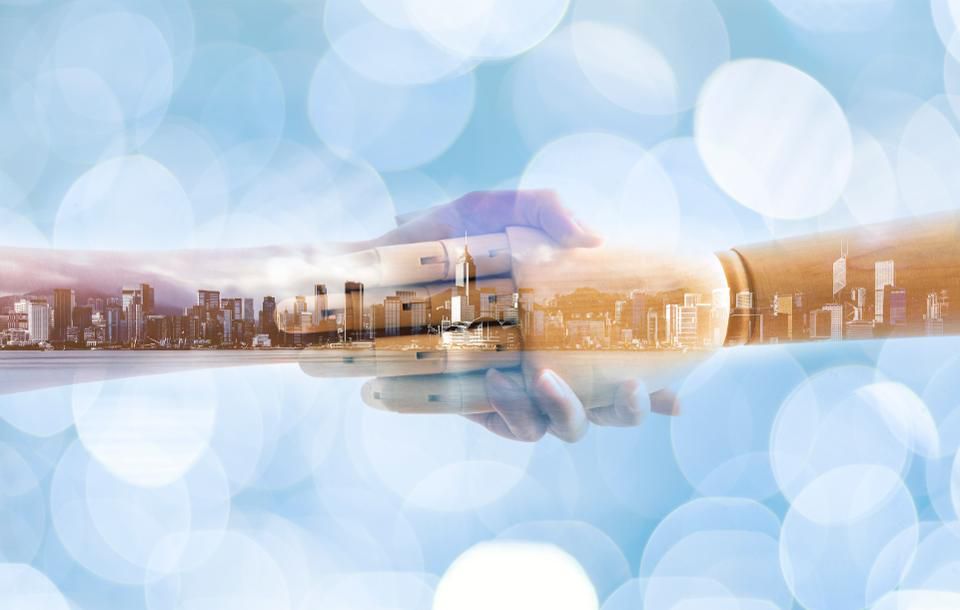
Mapping the AI Transformation Journey In Your Organization
Add Your Heading Text Here
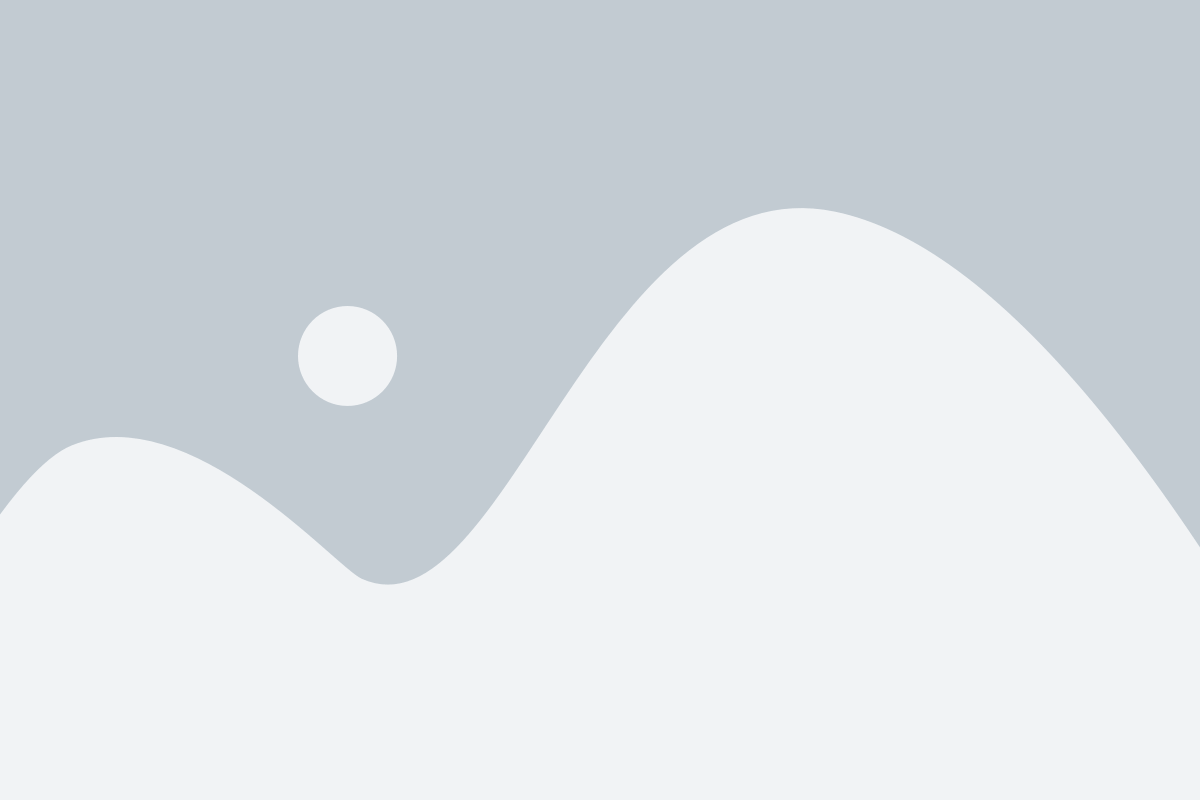
We are well and truly in the midst of the AI revolution. Research houses, academicians, think-tanks, business and technology leaders all agree upon the significant value waiting to be unlocked through the positive and progressive use of Artificial Intelligence – by re-engineering the old and envisioning the new. According to a research by Gartner, organizations using cognitive ergonomics and system design in new artificial intelligence projects will achieve long term success four times more often than others. Citing research by the MIT Center for Digital Business, from a competitive standpoint, companies that embrace digital transformation are 26 percent more profitable than their average industry competitors and enjoy a 12 percent higher market valuation.
The writing is on the wall. Intelligent business interventions made through AI will, to a large extent, define if your business will be an industry leader or a laggard tomorrow. And with that end in mind, businesses are rapidly changing their mindset and approach to AI – from topical experiments performed by forward-thinking business units, to more of a strategic mandate for enabling competitive differentiation. Businesses realize that for truly unlocking business value, they need to not only weave AI into the fabric of their enterprise, but also operationalize it – with the right personnel and change management initiatives. Given that AI can bring both cost efficiencies to business as well as potentially new revenue streams, businesses today are exploring an ‘AI Transformation’ – moving the dial on what is truly possible through a business model, engineered around AI. To enable your organization to do so, here are three powerful ideas to map the AI Transformation journey of your business.
Ensure Enterprise Readiness to Build and Adopt AI
The first step in the journey to AI Transformation for your enterprise is to understand and address if there are any disparities between your vision for AI and the ability of your organization to follow through with it. To that end, it is important to assess just how ready your enterprise is, in its current state, to build, deploy, adopt and benefit from AI-centric solutions. Ideas for AI Transformation need to be communicated clearly and grounded in the realities of organizational capabilities. When they are not, even the best intentions can go awry.
To do so, it is critical that business leaders measure their current AI maturity and assess the availability of internal skills. This will enable you to baseline just how empowered your current workforce is to develop industry-leading AI solutions. Once such a baseline is established on workforce readiness for building and adopting AI-led solutions, organizations need to start improving on these metrics – through internal trainings and external capability augmentation.
By developing this baseline score for AI readiness – organizations can have an objective view of where they are, how far they need to go and what the potential milestones to be achieved are in the journey to AI Transformation. This sort of pre-survey, combined with relevant training and assessment can help organizations craft a relevant roadmap with realistic timelines, as well as concrete actionables.
Build an AI ‘Win Team’
An AI Transformation is not unlike an extremely complex business re-engineering exercise. It entails massive changes – from the way you do business to how you run internal processes and staff multiple business units. Not only is it important to reskill a huge section of the workforce, there is also an important aspect of enabling change management to reinforce the importance of an AI-centric mindset.
To overcome this challenge, enterprises need to foster the consensus and engagement of a ‘win-team.’ This win-team would typically comprise functional and technical leaders who would be responsible for enabling the AI Transformation within their business units – from orienting the employees to the new mindset and ensuring capability readiness for the tasks at hand. On one hand, functional leaders can help their teams identify the processes that can be re-imagined using AI and manage resistance to change. On the other hand, technical leaders would lead the solutioning of technical components, while setting the training priorities and calendars for the workforce.
On change management, enterprises need employees to clearly appreciate the topline and bottomline benefits of an AI Transformation and focus towards enabling it. Employees stand to benefit themselves – as the professional benefits of making this transformation will accrue for their future. To further explore how companies can reduce the defensiveness in implementing AI-led processes further, they could also set innovation objectives for stakeholders as part of their performance metrics. Doing this will help create a strong alignment between individual, team and organizational objectives. A key aspect of AI transformation is ensuring large-scale adoption and usage of AI-powered solutions. AI applications typically fare better with every incremental user feedback and enriched data sources. Adoption and continuous use is a key parameter for the success of this transformation.
Integrated Business Processes over Siloed Business Functions
For years, the view of technology transformation and procurement has been of one that happens at a department / functional level – HR teams buy talent management software, finance teams sanction the purchase of accounting software, and CRMs get implemented to aid the efforts of sales teams. While this serves small technology initiatives, a sea-change is required for progressing an AI Transformation. To foster this, enterprises need to make a shift from a siloed, function-centric mindset to an integrated, process-centric mindset.
This is because AI use cases can often span multiple business units and functions, while tapping into multiple data sources for providing cross-team value, seamlessly. The very nature of AI deployments thus requires a process-centric view, with a strong consensus and buy-in from multiple stakeholders. Furthermore, the budget for purchasing AI services / applications is likely to come from the allocations of multiple beneficiaries across functions. This makes it all the more imperative that enterprises deprioritize functions in favor of processes.
An AI Transformation is doubtless the most strategic subject to be tackled by organizations today. Successful transformations will ensure enterprises go beyond mere automation and cost-cutting strategies and unveil previously unseen business and revenue opportunities. It is also extremely important to consider the role of digitization in building a new technology infrastructure that is AI-ready – possibly decentralized, cloud-based and highly available. There is now an urgent need for business leaders to have more than just a superficial understanding of AI and its successes. They will now be tasked with building and delivering a concrete, value-oriented roadmap for enabling a key transformation in the history of their organizations.
Related Posts
AIQRATIONS
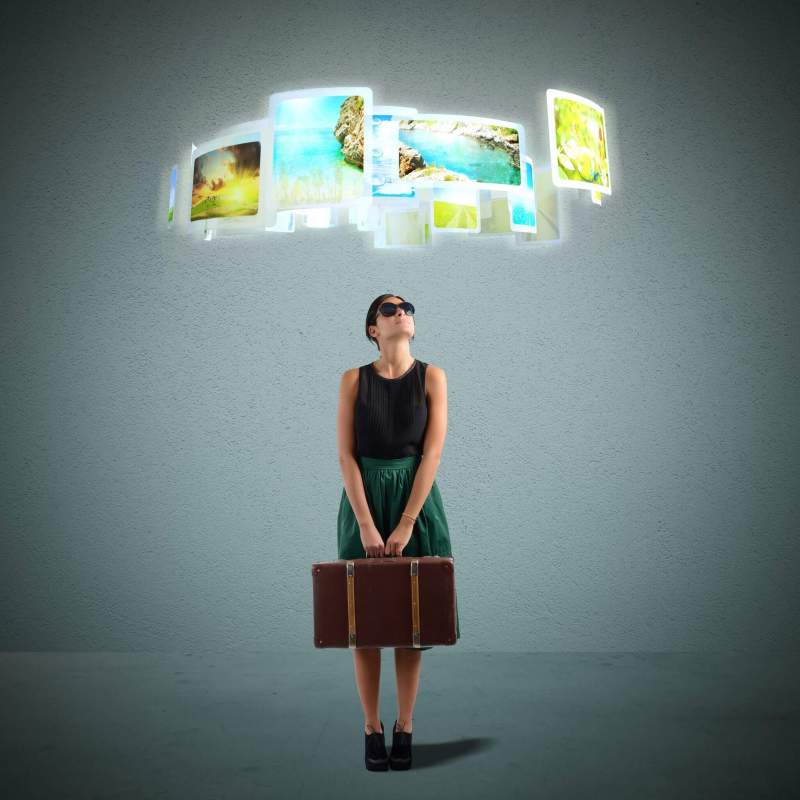
Travel & Hospitality Industry Transformation: Served Fast with AI
Add Your Heading Text Here
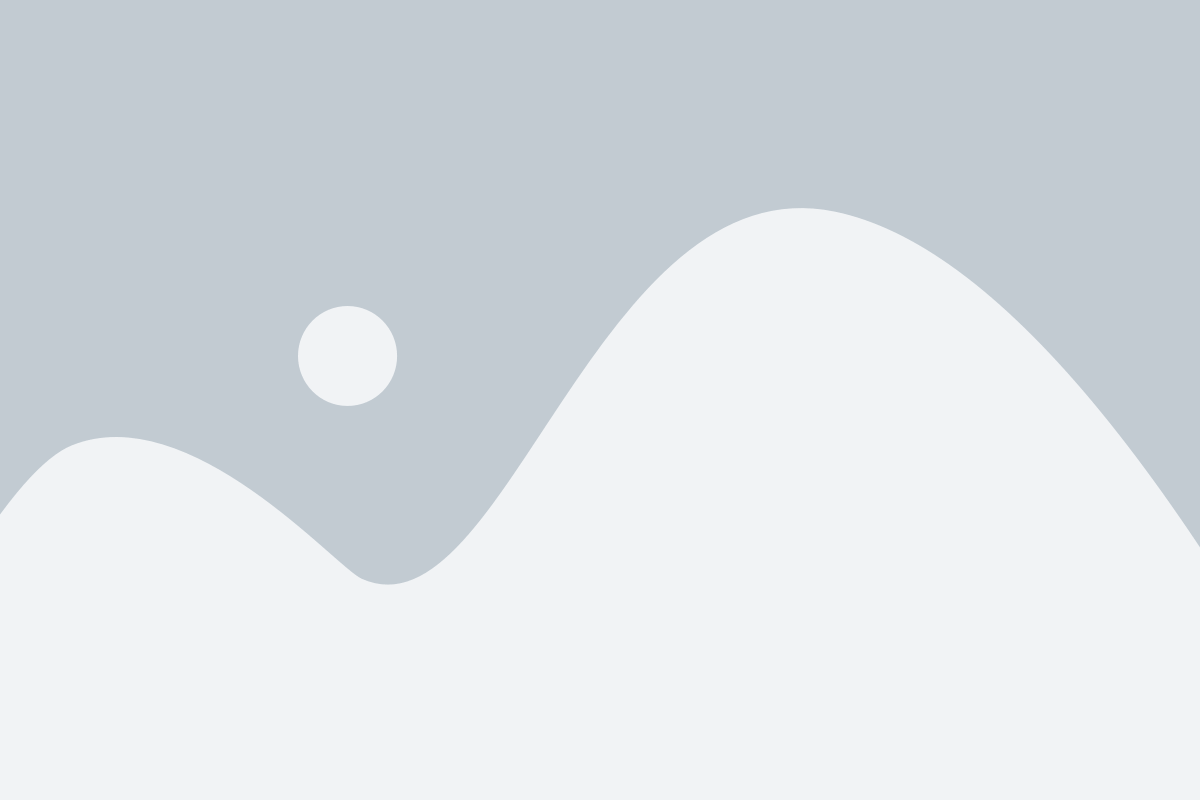
Over the years, the influence of AI has spread to almost every aspect of the travel and the hospitality industry. 30% of hospitality businesses use artificial intelligence to augment at least one of their primary sales processes and most customer personalization is done using AI.
30% of hospitality businesses use artificial intelligence to augment at least one of their primary sales processes.
The sudden popularity of Artificial Intelligence in the Travel industry can be credited to the humongous amount of data being generated today. Artificial Intelligence helps analyse unstructured data, brings value in partnership with Big Data and turns it into meaningful and actionable insights. Trends, outliers and patterns are figured out using this smart data which helps in guiding a Travel company to make informed decisions. The discounts, schemes, tour packages, seasons to target and people to target are formulated using this data. Usually, surveys and social media sensing are done to know customer’s insights and behaviour.
Let’s look at how AI has influenced each aspect of the business
Bleisure – Personalized Experience
There are always a few who are up for a new challenge and adopt to new technology. Many hotels have started using an AI concierge. One great example of an AI concierge is Hilton World wide’s Connie, who is the first true AI-powered concierge bot.
Connie stands at 2 feet high and guests can interact with it during their check-in. Connie is powered by IBM’s Watson AI and uses WayBlazer travel database. It can provide information to guests on local attractions, places to visit, etc. Being an AI, it can learn and adapt and respond to each guest.
In the Travel business, Mezi, using Artificial Intelligence and Natural Language Processing, provides a personalized experience to Business travellers who usually are strapped for time. It talks about bringing on a concept of bleisure (business+leisure) to address the needs of the workforce. A research done by them states that 84% of business travellers return feeling frustrated, burnt out and unmotivated. The kind of tedious and monotonous planning that goes into the travel booking could be the reason for it. With AI and NLP, Mezi collects preferences and generates suggestions so that a customized and streamlined experience is given and the issues faced by them are addressed properly.
Increased Productivity – Instant Connectivity
Increased productivity now begins with the search for the hotel, and technology has paved its way for the customer to access more data than ever before. Booking sites like Lola (www.lola.com) who provide on-demand travel services have developed technologies that can not only instantly connects people to their team of travel agents who find and book flights, hotels, and cars but have been able to empower their agents with tremendous technology to make research and decisions an easy process.
Intelligent Travel Assistants – Chatbots
Chatbot technology is another big strand of AI, and unsurprisingly, many travel brands have already launched their own versions in the past year or so. Skyscanner is just one example, creating a bot to help consumers find flights in Facebook Messenger. Users can also use it to request travel recommendations and random suggestions. Unlike ecommerce or retail brands using chatbots, which can appear gimmicky, there is an argument that examples like Skyscanner are much more relevant and useful for everyday consumers.
After all, with the arrival of many more travel search websites, consumers are being overwhelmed by choice – not necessarily helped by it. Consequently, a bot like Skyscanner is able to cut through the noise, connecting with consumers in their own time and in the social media spaces they most frequently visit.
Recently, Aeromexico started using Facebook Messenger chatbot to answer the very generic questions by the customers. The main idea was to cater to 80% of questions which are usually the repeated ones and about common topics. Thus, to avoid a repetitive process, artificial intelligence is of great application. Airlines hugely benefit from this. KLM Royal Dutch Airlines uses artificial intelligence to respond to the queries of customers on twitter and facebook. It uses an algorithm from a company called Digital Genius which is trained on 60,000 questions and answers. Not only this, Deutsche Lufthansa’s bot Mildred can help in searching the cheapest fares.
Discovery & Data Analysis – Intelligent Recommendations
International hotel search engine Trivago acquired Hamburg, Germany machine learning startup, Tripl, as it ramps up its product with recommendation and personalization technology, giving them a customer-centric approach.
The AI algorithm gives tailored travel recommendations by identifying trends in users’ social media activities and comparing it with in-app data of like-minded users. With its launch in July 2015, users could sign up only through Facebook, potentially sharing oodles of profile information such as friends, relationship status, hometown, and birthday.
Persona based travel recommendations, use of customised pictures and text are now gaining ground to entice travellers to book your hotels. KePSLA’s travel recommendation platform is one of the first in the world to do this by using deep learning and NLP solutions.
With 81% of people believing that robots would be better at handling data than humans, there is also a certain level of confidence in this area from consumers.
Knowing your Travellers – Deep Customer Behaviour
Dorchester Collection is another hotel chain to make use of AI. However, instead of using it to provide a front-of-house service, it has adopted it to interpret and analyse customer behaviour in the form of raw data.
Partnering with technology company, RicheyTX, Dorchester Collection has helped to develop an AI platform called Metis.
Delving into swathes of customer feedback such as surveys and reviews (which would take an inordinate amount of time to manually find and analyse) it is able to measure performance and instantly discover what really matters to guests.
For example, Metis helped Dorchester to discover that breakfast it not merely an expectation – but something guests place huge importance on. As a result, the hotels began to think about how they could enhance and personalise the breakfast experience.
Flight Fare and Hotel Price Forecasting
Flight fares and hotel prices are ever-changing and vary greatly depending on the provider. No one has time to track all those changes manually. Thus, smart tools which monitor and send out timely alerts with hot deals are currently in high demand in the travel industry.
The AltexSoft data science team has built such an innovative fare predictor tool for one of their clients, a global online travel agency, Fareboom.com. Working on its core product, a digital travel booking website, they could access and collect historical data about millions of fare searches going back several years. Armed with such information, they created a self-learning algorithm, capable of predicting the future price movements based on a number of factors, such as seasonal trends, demand growth, airlines special offers, and deals.
With the average confidence rate at 75 percent, the tool can make short-term (several days) as well as long-term (a couple of months) forecasts.
Optimized Disruption Management
While the previous case is focused mostly on planning trips and helping users navigate most common issues while traveling, automated disruption management is somewhat different. It aims at resolving actual problems a traveler might face on his/her way to a destination point.
Mostly applied to business and corporate travel, disruption management is always a time-sensitive task, requiring instant response. While the chances to get impacted by a storm or a volcano eruption are very small, the risk of a travel disruption is still quite high: there are thousands of delays and several hundreds of canceled flights every day.
With the recent advances in technology, it became possible to predict such disruptions and efficiently mitigate the loss for both the traveler and the carrier. The 4site tool, built by Cornerstone Information Systems, aims at enhancing the efficiency of enterprise travel. The product caters to travelers, travel management companies, and enterprise clients, providing a unique set of features for real-time travel disruption management.
For example, if there is a heavy snowfall at your destination point and all flights are redirected to another airport, a smart assistant can check for available hotels there or book a transfer from your actual place of arrival to your initial destination.
Not only passengers are affected by travel disruptions; airlines bear significant losses every time a flight is canceled or delayed. Thus, Amadeus, one of the leading global distribution systems (GDS), has introduced Schedule Recovery system, aiming to help airlines mitigate the risks of travel disruption. The tool helps airlines instantly address and efficiently handle any threats and disruptions in their operations.
Future potential
So, we’ve already seen the travel industry capitalise on AI to a certain extent. But how will it evolve in the coming year?
Business travel
Undoubtedly, we’ll see many more brands using AI for data analysis as well as launching their own chatbots. There’s already been a suggestion that Expedia is next in line, but it is reportedly set to focus on business travel rather than holidaymakers. Due to the greater need for structure and less of a desire for discovery, it certainly makes sense that artificial intelligence would be more suited to business travellers.
Specifically, it could help to simplify the booking process for companies, as well as help eliminate discrepancies around employee expenses. With reducing costs and improving efficiency two of the biggest benefits, AI could start to infiltrate business travel even more so than leisure in the next 12 months.
Voice technology
Lastly, we can expect to see greater development in voice-activated technology.
With voice-activated search, the experience of researching and booking travel has the potential to become quicker and easier than ever before. Similarly, as Amazon Echo and Google Home start to become commonplace, more hotels could start to experiment with speech recognition to ramp up customer service.
This means devices and bots could become the norm for brands in the travel industry.