
Lock in winning AI deals : Strategic recommendations for enterprises & GCCs
Add Your Heading Text Here
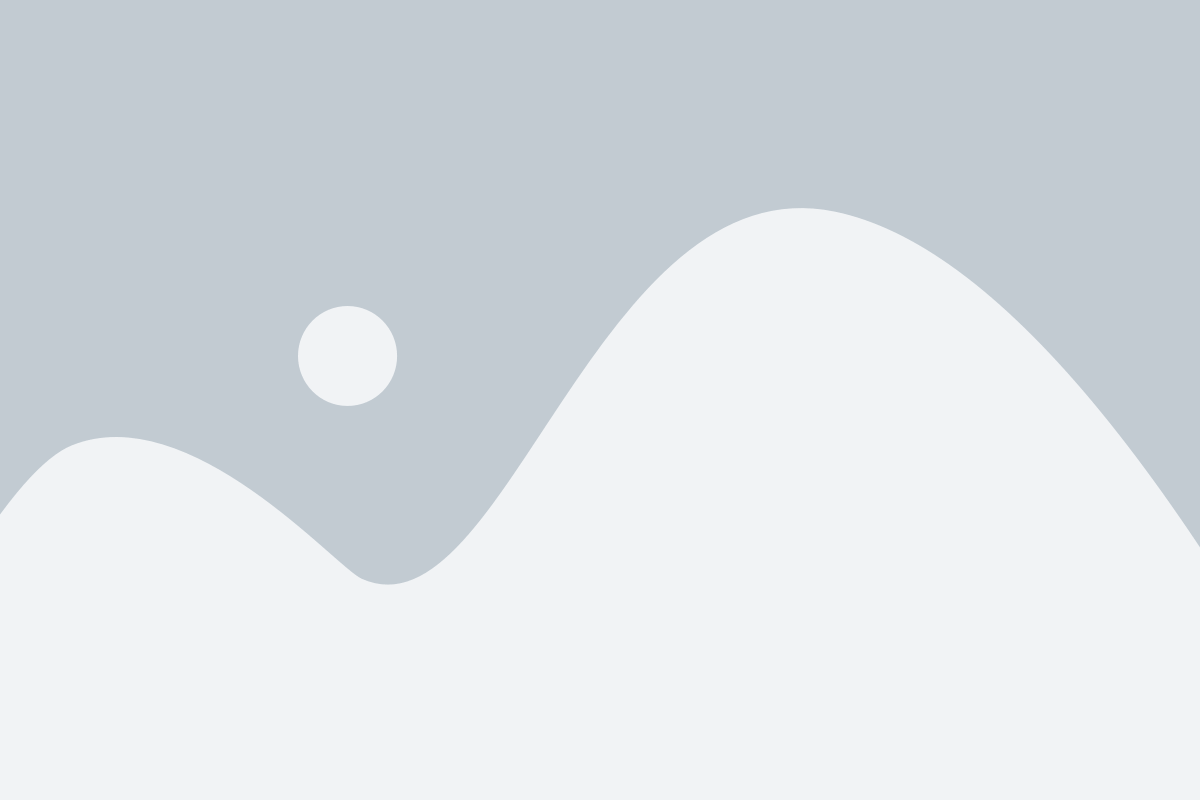
Artificial Intelligence is unleashing exciting growth opportunities for the enterprises & GCCs , at the same time , they also present challenges and complexities when sourcing, negotiating and enabling the AI deals . The hype surrounding this rapidly evolving space can make it seem as if AI providers hold the most power at the negotiation table. After all, the market is ripe with narratives from analysts stating that enterprises and GCCs failing to embrace and implement AI swiftly run the risk of losing their competitiveness. With pragmatic approach and acknowledgement of concerns and potential risks, it is possible to negotiate mutually beneficial contracts that are flexible, agile and most importantly, scalable. The following strategic choices will help you lock in winning AI deals :
Understand AI readiness & roadmap and use cases
It can be difficult to predict exactly where and how AI can be used in the future as it is constantly being developed, but creating a readiness roadmap and identifying your reckoner of potential use cases is a must. Enterprise and GCC readiness and roadmap will help guide your sourcing efforts for enterprises and GCCs , so you can find the provider best suited to your needs and able to scale with your business use cases. You must also clearly frame your targeted objectives both in your discussions with vendors as well as in the contract. This includes not only a stated performance objective for the AI , but also a definition of what would constitute failure and the legal consequences thereof.
Understand your service provider’s roadmap and ability to provide AI evolution to steady state
Once you begin discussions with AI vendors & providers, be sure to ask questions about how evolved their capabilities and offerings are and the complexity of data sets that were used to train their system along with the implementation use cases . These discussions can uncover potential business and security risks and help shape the questions the procurement and legal teams should address in the sourcing process. Understanding the service provider’s roadmap will also help you decide whether they will be able to grow and scale with you. Gaining insight into the service provider’s growth plans can uncover how they will benefit from your business and where they stand against their competitors. The cutthroat competition among AI rivals means that early adopter enterprises and GCCs that want to pilot or deploy AI@scale will see more capabilities available at ever-lower prices over time. Always mote that the AI service providers are benefiting significantly from the use cases you bring forward for trial as well as the vast amounts of data being processed in their platforms. These points should be leveraged to negotiate a better deal.
Identify business risk cycles & inherent bias
As with any implementation, it is important to assess the various risks involved. As technologies become increasingly interconnected, entry points for potential data breaches and risk of potential compliance claims from indirect use also increase. What security measures are in place to protect your data and prevent breaches? How will indirect use be measured and enforced from a compliance standpoint? Another risk AI is subject to is unintentional bias from developers and the data being used to train the technology. Unlike traditional systems built on specific logic rules, AI systems deal with statistical truths rather than literal truths. This can make it extremely difficult to prove with complete certainty that the system will work in all cases as expected.
Develop a sourcing and negotiation plan
Using what you gained in the first three steps, develop a sourcing and negotiation plan that focuses on transparency and clearly defined accountability. You should seek to build an agreement that aligns both your enterprise’s and service provider’s roadmaps and addresses data ownership and overall business and security related risks. For the development of AI , the transparency of the algorithm used for AI purposes is essential so that unintended bias can be addressed. Moreover, it is appropriate that these systems are subjected to extensive testing based on appropriate data sets as such systems need to be “trained” to gain equivalence to human decision making. Gaining upfront and ongoing visibility into how the systems will be trained and tested will help you hold the AI provider accountable for potential mishaps resulting from their own erroneous data and help ensure the technology is working as planned.
Develop a deep understanding of your data, IP, commercial aspects
Another major issue with AI is the intellectual property of the data integrated and generated by an AI product. For an artificial intelligence system to become effective, enterprises would likely have to supply an enormous quantity of data and invest considerable human and financial resources to guide its learning. Does the service provider of the artificial intelligence system acquire any rights to such data? Can it use what its artificial intelligence system learned in one company’s use case to benefit its other customers? In extreme cases, this could mean that the experience acquired by a system in one company could benefit its competitors. If AI is powering your business and product, or if you start to sell a product using AI insights, what commercial protections should you have in place?
In the end , do realize the enormous value of your data, participate in AI readiness, maturity workshops and immersion sessions and identification of new and practical AI use cases. All of this is hugely beneficial to the service provider’s success as well and will enable you to strategically source and win the right AI deal.
(AIQRATE advisory & consulting is a bespoke global AI advisory & consulting firm and provides strategic advisory services to boards, CXOs, senior leaders to curate , design building blocks of AI strategy , embed AI@scale interventions & create AI powered enterprises . Visit www.aiqrate.ai , reach out to us at consult@aiqrate.ai )
Related Posts
AIQRATIONS
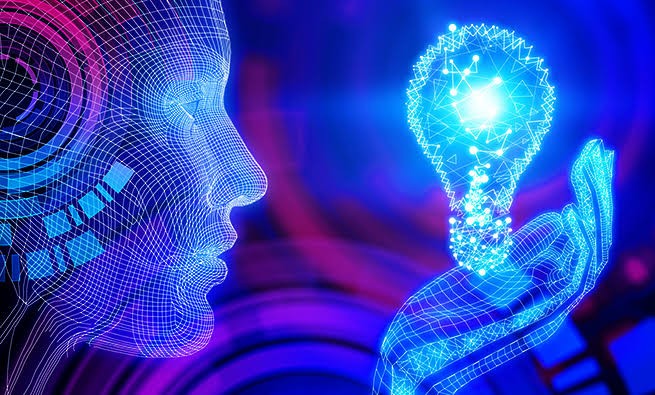
AI for Strategic Innovation
Add Your Heading Text Here
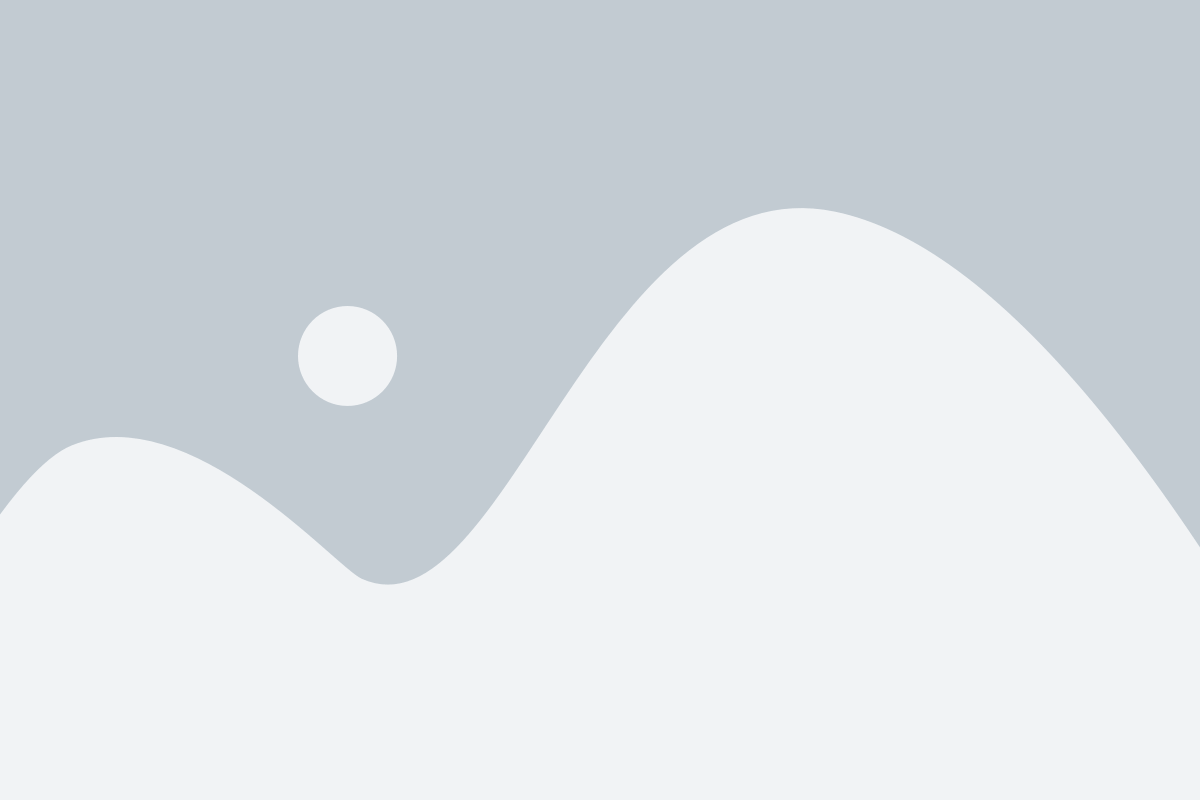
The extra ordinary promise of AI : Global & Indian enterprises have a lot to gain from unleashing innovation with AI —but harnessing their potential demands focused investment and a new way of working with external partners.
Here are few salient features of how AI has become game changing trend in spurring innovation; existing challenges and few strategic approaches of unlocking innovation with AI :
- 22% growth : From 2015 through 2019, disclosed private investment in seven deep tech sectors grew an average of 22% per year, equaling nearly $60 billion in total investment. Corporate venture capital is also playing an increasingly active role.
- Total investment : Nearly $60 Billion Invested in Deep Tech’s Fastest-Growing Sectors in 2019; Artificial intelligence corners close to $25 Bn
- About 1800 AI led startups in the US accounted for roughly half of this total investment, but other countries are catching up fast.
Existing Challenges
- Complex ecosystems : Multiple types of players including startups, venture capital firms, governments, universities and research centers, and early-adopter user groups
- Dynamic Interactions : Few central orchestrators; business relationships based on informal networks rather than formal contracts
Strategic approaches of unlocking innovation with AI :
- Cooperate in order to compete : Think beyond the enterprise’s immediate goals; commit to a long-term vision for the development of the ecosystem as whole
- Identify capabilities that add value : Define what the enterprise can offer to nurture the ecosystem and bring AI to market—not only money but also access to customers, data, networks, mentors, and technical experts
- Don’t pick winners in advance : AI startups are evolving rapidly. Continuously monitor the ecosystem to identify successful startups, applications, and business models as they emerge
- Blur the boundaries with partners : Make it easy for AI partners to navigate your corporate system. Define a clear role for them in your innovation strategy, ensure senior-executive sponsorship, and engage the core businesses
- Streamline decision making and governance : Success requires partnering more nimbly with fast-moving AI startups. Embrace agile ways of working.
- Develop breakthrough solutions by combining expertise from previously unconnected fields or industries. Be alert for game hanging opportunities that deliver both economic and social value.
AI will transform business and society in the future. The time to craft a AI strategy for unleashing innovation is now.
AIQRATE works closely with global & Indian enterprises , GCCs , VC/PE firms and has an extensive yet curated database of 1000 + global AI startups , boutique and niche firms benchmarked on our “Glow Curve” assessment.
(AIQRATE advisory & consulting is a bespoke AI advisory and consulting firm and provide strategic advisory services to boards , CXOs, senior leaders to curate , design building blocks of AI strategy , embed AI@scale interventions and create AI powered enterprises . visit : www.aiqrate.ai ; reach out to us at consult@aiqrate.ai )
Related Posts
AIQRATIONS
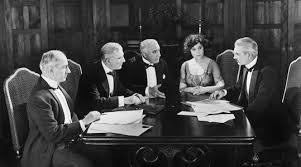
AI led strategy for business transformation : A guided approach for CXOs
Add Your Heading Text Here
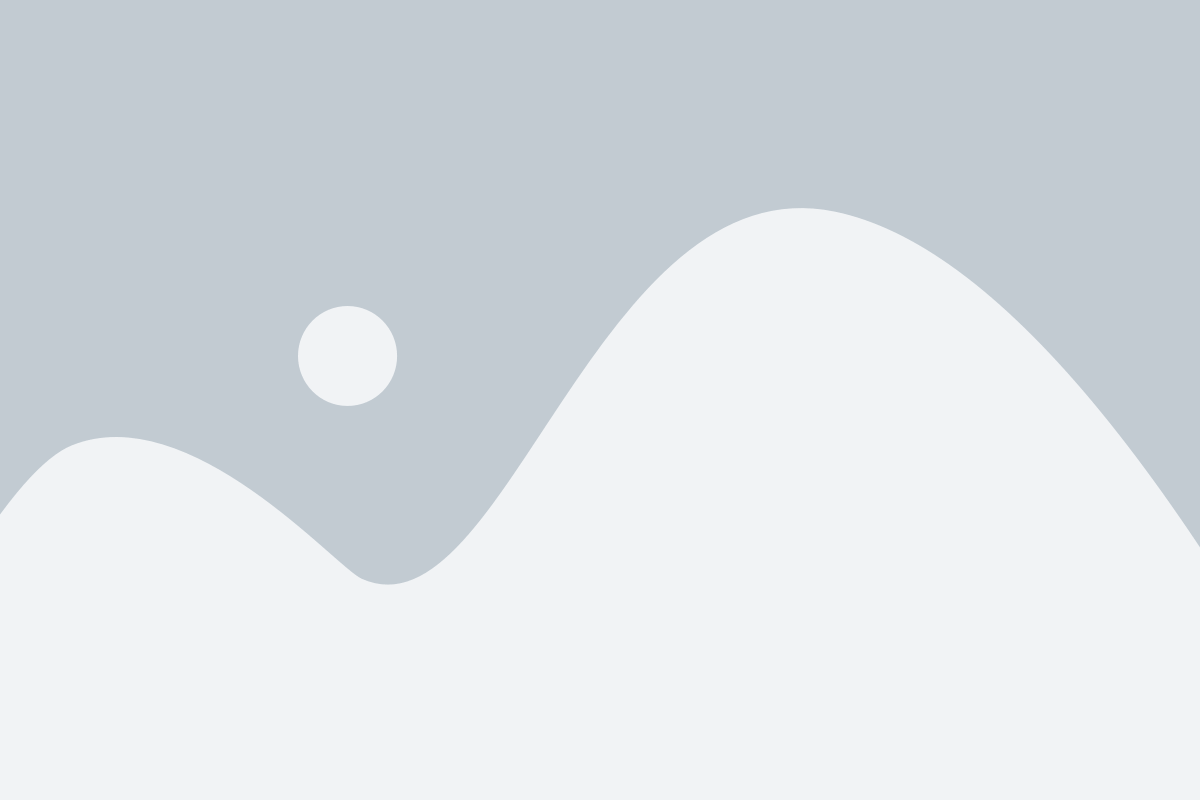
Business transformation programs have long focused on productivity enhancements —taking a “better, faster, cheaper” approach to how the enterprise works. And for good reason: disciplined efforts can boost productivity as well as accountability, transparency, execution, and the pace of decision making. When it comes to delivering fast results to the bottom line, it’s a proven recipe that works.
The problem is, it’s no longer enough. Artificial Intelligence enabled disruption are upending industry after industry, pressuring incumbent companies not only to scratch out stronger financial returns but also to remake who and what they are as enterprises.
Doing the first is hard enough. Tackling the second—changing what your company is and does—requires understanding where the value is shifting in your industry (and in others), spotting opportunities in the inflection points, and taking purposeful actions to seize them. The prospect of doing both jobs at once is sobering.
How realistic is it to think your company can pull it off? The good news is that AIQRATE can demonstrate that it’s entirely possible for organizations to ramp up their bottom-line performance even as they secure game-changing portfolio wins that redefine what a company is and does. What’s more, AL led transformations that focus on the organization’s performance and portfolio appear to load the dice in favor of transformation results. By developing these two complementary sets of muscles, companies can aspire to flex them in a coordinated way, using performance improvements to carry them to the next set of portfolio moves, which in turn creates momentum propelling the company to the next level.
Strategic Steps towards AI led Transformation:
This aspect covers AI led “portfolio-related” moves. The first is active resource reallocation towards building AI led transformation units, which I define as the company shifting more than 20 percent of its capital spending across its businesses or markets over ten years. Such firms create 50 percent more value than counterparts that shift resources at a slower clip.
Meanwhile, a big move in programmatic M&A driven by AI led spot trending—the type of deal making that produces more reliable performance boosts than any other—requires the company to execute at least one deal per year, cumulatively amounting to more than 30 percent of a company’s market capitalization over ten years, and with no single deal being more than 30 percent of its market capitalization.
Making big moves tends to reduce the risk profile and adds more upside than downside. The way I explain this to senior executives is that when you’re parked on the side of a volcano, staying put is your riskiest move.
AI led Transformations that go ‘all in’ by addressing both a company’s performance and its portfolio yield the highest odds.
The implication of these transformation stories is clear: approaches that go all in by addressing both a company’s performance and its portfolio yield the highest odds of lasting improvement. Over the course of a decade, companies that followed this path nearly tripled their likelihood of reaching the top quin tile of the AI transformation power curve relative to the average company in the middle.
Play to win with AI
Life would be simpler if story ended here. However, you’re not operating in a competitive vacuum. As I described earlier, other forces influence your odds of success in significant ways—in particular, how your industry is performing. Research studies have indicated that companies facing competitive headwinds would face longer odds of success than those with tailwinds.
Companies that combined big performance moves with big portfolio moves (including capital expenditures, when not the only portfolio move employed) saw a big lift in their odds. Life is still challenging for these companies—their net odds are dead even—yet this is superior to the negative odds of the other situations.
Winning thru competitive advantage with AI
In an improving industry, the returns to performance improvement are amplified massively. This runs contrary to the very human tendency of equating performance transformations with turnaround cases
The takeaway from all this is that two big rules stand out as commonly and powerfully true whatever your context: first, get moving with AI , don’t be static; second, go all in if you can with AI led transformation programs —it’s always the best outcome (and also the rarest).
Running the AI led transformation program
In my experience, the companies that are most successful at transforming themselves with AI ,sequence their moves so that the rapid lift of performance improvement provides oxygen and confidence for big moves in M&A, capital investment, and resource reallocation. And when the right portfolio moves aren’t immediately available or aren’t clear, the improved performance helps buy a company time until the strategy can catch up.
To illustrate this point, consider the anecdote about Apple that Professor Richard Rumelt describes in his book, Good Strategy/Bad Strategy. It was the late 1990s; Steve Jobs had returned to Apple and cleaned house through productivity-improving cutbacks and a radically simplified product line. Apple was much stronger, yet it remained a niche player in its industry. When Rumelt asked Jobs how he planned to address this fact, Jobs just smiled and said, ‘I am going to wait for the next big thing.’
While no one can guarantee that your “next big thing” will be an iPod-size breakthrough, there’s nothing stopping you from laying the groundwork for a successful AI led transformation. To see how prepared, you are for such an undertaking, ask yourself—and your team—the following five questions. I sincerely hope they provoke productive and transformative discussion among your team.
1.Where is the new business value chain that’s driven by AI
Achieving success with big, portfolio-related moves requires understanding where the business value flows in your business and why. The structural attractiveness of markets, and your position in them, can and does change over time. Ignore this and you might be shifting deck chairs on the Titanic. Meanwhile, to put this thinking into action, you must also view the company as an ever-changing portfolio. This represents a sea change for managers who are used to plodding, once-a-year strategy sessions that are more focused on “getting to yes” and on protecting turf than on debating real alternatives. Get high-powered decision-making algorithms to navigate you thru this transformation.
2. Put your money in building an AI led strategy
Only 10% of the US fortune 200 companies have AI led strategy; this is an impending strategic aspect that cannot be ignored. The dimensions of reimagining customer experience, building innovative products and services and transforming the businesses need to have an AI led strategy move by the CXOs
3.Are you ready for disruption?
Increasingly, incumbent organizations are getting to the pointy end of disruption, where they must accelerate the transition from legacy business models to new ones and even allow potentially cannibalizing businesses to flourish. Sometimes this requires a very deliberate two-speed approach where legacy assets are managed for cash while new businesses are nurtured for growth.
4.Will our company take this seriously?
Embracing AI led transformative change requires commitment, and gaining commitment requires a compelling change story that everyone in the company can embrace. Philips recognized this in 2011 when it launched its “Accelerate” program. Along with productivity improvements and portfolio changes (including a big pivot from electronics to health tech), the company shaped its change story around improving three billion lives annually by 2030, as part of a broader goal of making the world healthier and more sustainable through innovation. Massive thrust and investment was laid by Phillips leadership team on AI led transformation programs.
5.Is the leadership ready for the transformation?
Leading a successful AI led transformation requires a lot more than just picking the right moves and seeing them through. Among your other priorities: build momentum, engage your workforce, and make the change personal for yourself and your company. All of this means developing new leadership skills and ways of working, while embracing a level of commitment as a leader that may be unprecedented for you.
In the end, AI led strategy for transformation is a process and start of a journey …. embrace it or feel the heat of leaving behind. The new age competition is agile and nimble and AI led transformation strategy is a right move to thwart the competition.
Related Posts
AIQRATIONS
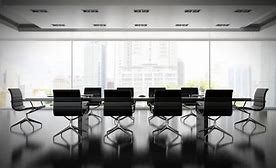
AI led Strategy for Boards : The “new” strategy counselor
Add Your Heading Text Here
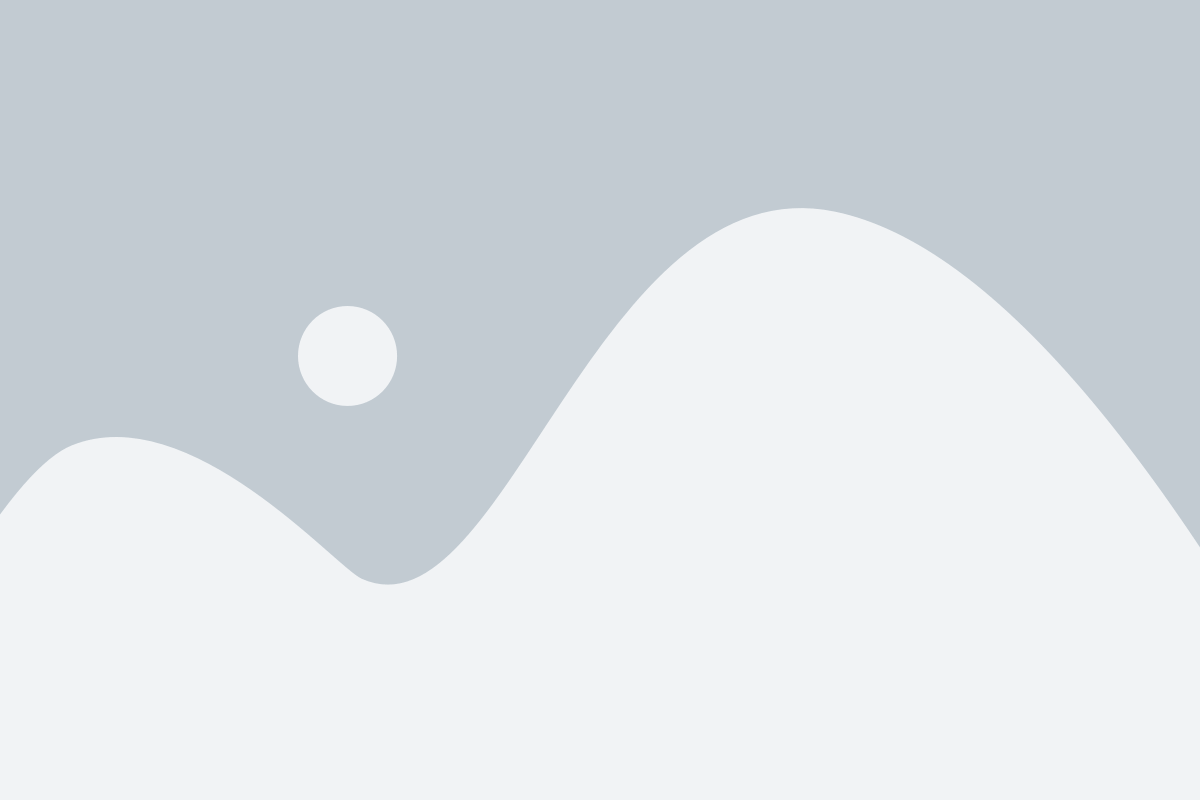
It’s time for boards to craft an AI led strategy . Three strategic aspects can help them and senior leaders to augment decision making process in the board meetings
In the boardroom, and the head of a major global conglomerate is in the hot seat. A director with a background in the manufacturing industry is questioning the economics, an assumption underlying the executive’s industry forecast: that the industry’s ratio of forecast will remain relatively constant. The business leader appears confident about the assumption of stability, which has implications for both the competitive environment and for financial results. But the director isn’t convinced: “In my experience, the forecast changes continuously with the economic cycle and needs to bake in assumptions,” he says, “and I’d feel a whole lot better about these estimates if you had some facts to prove that this has changed.” and the rest of the board doesn’t have it. Finally, the chairman intervenes: “The question being raised is critical and not just for our manufacturing business but for our entire strategy. We’re not going to resolve this today, but let’s make sure it’s covered thoroughly during our strategy off-site and he added , “let’s have some good staff work in place to inform the discussion.”
If the preceding exchange sounds familiar, it should: in the wake of the financial crisis, we find that uncomfortable conversations such as this one are increasingly common in boardrooms around the world as corporate directors and executives come to grips with a changed environment. Ensuring that a company has a great strategy is among a board’s most important functions and the ultimate measure of its stewardship. Yet even as new governance responsibilities and faster competitive shifts require much more—and much better—board engagement on strategy, a great number of boards remain hamstrung by familiar challenges.
Enter AI led strategy for boards
For starters, there’s the problem of time: most boards have about six to eight meetings a year and are often hard pressed to get beyond compliance-related topics to secure the breathing space needed for developing strategy. A recent survey of board members to learn where they’d most like to spend additional time, two out of three picked strategy. A related finding was that 44 percent of directors said their boards simply reviewed and approved management’s proposed strategies. Why such limited engagement? One likely reason is an expertise gap: only 10 percent of the directors felt that they fully understood the industry dynamics in which their companies operated. As a result, only 21 percent of them claimed to have a complete understanding of the current strategy .
What’s more, there’s often a mismatch between the time horizons of board members and of top executives , and that lack of alignment can diminish a board’s ability to engage in well-informed give-and-take about strategic trade-offs. “The chairman of my company has effectively been given a decade,” says the CEO of a company “and I have three years—tops—to make my mark. If I come up with a strategy that looks beyond the current cycle, I can never deliver the results expected from me. Yet I am supposed to work with him to create long-term shareholder value. How am I supposed to make this work?” It’s a fair question, particularly since recent shows that major strategic moves involving active capital reallocation deliver higher shareholder returns than more passive approaches over the long haul, but lower returns over time frames of less than three years.
Compounding these challenges is the increased economic volatility prompting many companies to rethink their strategic rhythm, so that it becomes less calendar driven and formulaic and more a journey involving frequent and regular dialogue among a broader group of executives. To remain relevant, boards must join management on this journey, and management in turn must bring the board along—all while ensuring that strategic co-creation doesn’t become confusion or, worse, shadow management. This is where curating AI strategy for competitive advantage and informed decision making comes to the picture.
Three strategic aspects to ponder on AI led strategy for Boards :
While no one-size-fits-all solution can guide companies as they set out, board members and senior managers ask themselves three simple questions as they approach the development of AI strategy. Using it should raise the quality of decision making , overall engagement and help determine the practical steps each group must take to get there. The usual annual strategic refresh is unlikely to provide the board with an appreciation of the context it would need to address the questions fully, let alone to generate fresh insights in response.
1.Can AI make the boards understand the industry dynamics
Most boards spend most of their strategic time reviewing plans, yet relatively few directors feel they have a complete understanding of the dynamics of the industries their companies operate in or even of how those companies create value. To remedy this problem and to avoid the superficiality it can engender, boards need time—some without management present—so they can more fully understand the structure and economics of the business, as well as how it creates value. They should use this time to get ahead of issues rather than always feeling a step behind during conversations on strategy or accepting management biases or ingrained habits of thought.AI can lay out comprehensive picture of industry and competitive industry dynamics with historical and future forward looking scenarios to make the job of the boards simpler.
2. Can AI trigger enough board–management debate before a specific strategy is discussed?
Aided thru AI and armed with a foundational view based on a clearer understanding of industry and company economics, boards are in a better position to have the kinds of informed dialogue with senior managers that ultimately help them prepare smarter and more refined strategic options for consideration. Board members should approach these discussions with data driven mind-set and with the goal of helping management to broaden its thinking by considering new, even unexpected, perspectives.
During such debates, management’s role is to introduce key pieces of content: a detailed review of competitors, key external trends likely to affect the business, and a view of the specific capabilities the company can use to differentiate itself. The goal of the dialogue is to develop a stronger, shared understanding of the skills and resources the company can use to produce strong returns, as opposed to merely moving with the tide. This is where boards can evangelize and seep in AI in the senior executives group for broader knowledge augmentation .
3.Can AI bring in all strategic options and approaches to the table for board and management ?
Very often, the energizing discussions between the board and management about the business, its economics, and the competition represent the end of the debate. Afterward, the CEO and top team go off to develop a plan that is then presented to the board for approval. Instead, what’s needed at this point is for management to take some time—go thru the self-learning enabled algorithm —to formulate a robust set of strategic options, each followed through to its logical end state, including the implications for the allocation of people, capital, and other resources. These strategic options through the revised algorithmic exercise can then be brought back to the board for discussion and decision making.
Developing AI led strategy is a new phenomenon and will take time to mature —yet will become more powerful algorithmic based decision making process and with board’s increased involvement, which introduces new voices and expertise to the debate and puts pressure on management teams and board members alike to find the best answers. Yet this form of AI led strategy development, when done well, is invaluable. It not only leads to clearer strategies but also creates the alignment necessary to make bolder moves with more confidence and to follow through by committing resources to key decisions. AI led decision making for the boards is here….
(AIQRATE advisory & consulting is a bespoke AI advisory and consulting firm and provide strategic advisory services to boards , CXOs, senior leaders to curate , design building blocks of AI strategy , embed AI@scale interventions and create AI powered enterprises . visit : www.aiqrate.ai )
Related Posts
AIQRATIONS
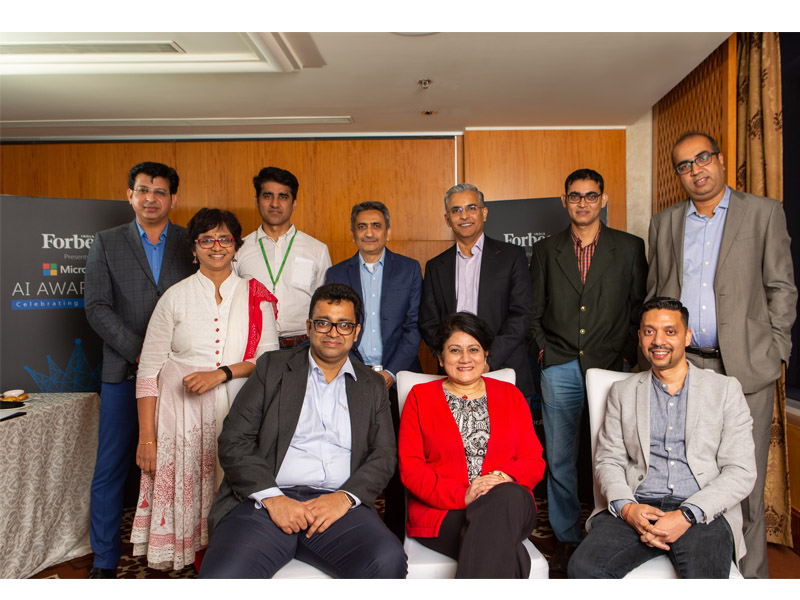
AIQRATE at AI Roundtable organized by Forbes & Microsoft
Add Your Heading Text Here
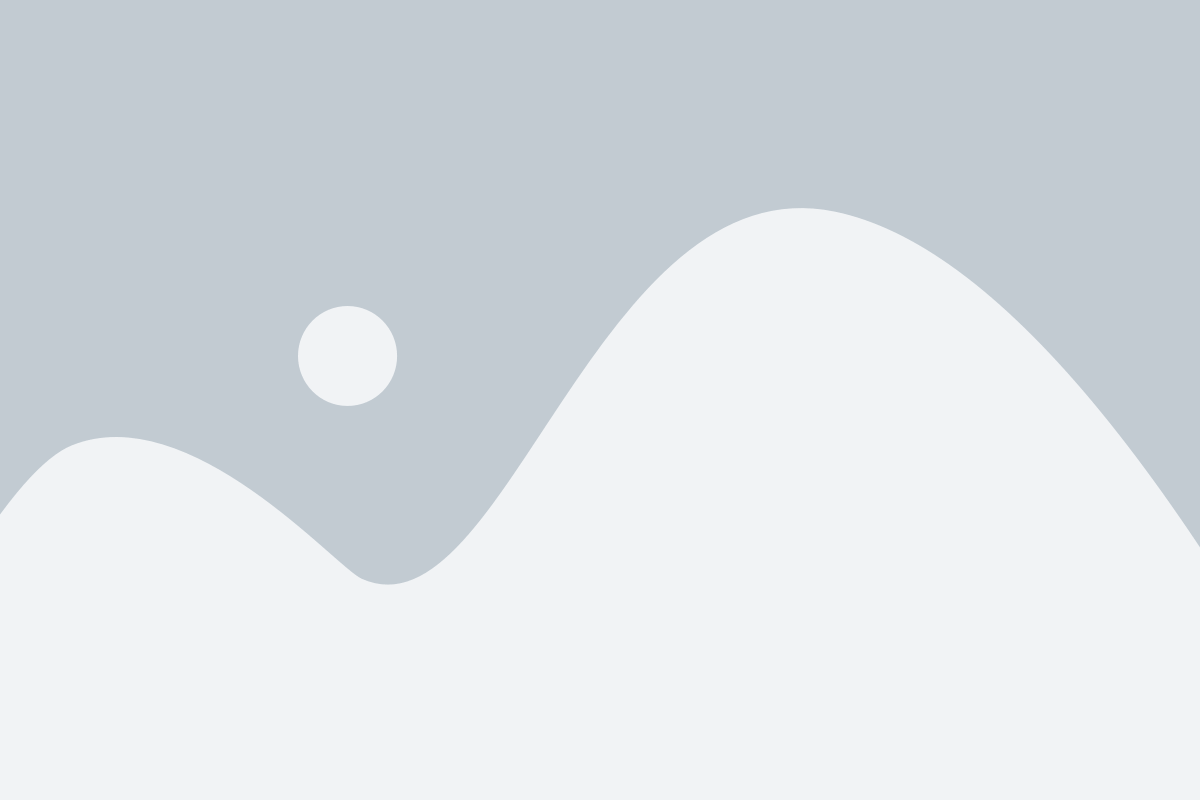
A group of CXOs from several enterprises in Bengaluru came together recently at a round table organised by Forbes India in partnership with Microsoft. They discussed the state of AI and its impact on innovation in their businesses. Below are the edited excerpts:
“Whether we like it or not, AI is absolutely all-pervasive. It’s been there for about 50 years as a technology, but the adoption rate has gone up significantly in just the last four to five years due to multiple reasons – capability, visioning algorithms, better underlying infrastructure to make it run and just the sheer drive of the industry to drive more and more ROI.” said Rohit Adlakha, chief digital and information officer and global head, Wipro HOLMES at Wipro. “Human capability is getting pushed to the limits. How do we augment that with a certain technology that can work hand in hand, while not truly replacing them, but more in terms of enhancement? Looking at the size and scale of a seven billion population across the globe, it is clear that mere technology adoption will not get us there. Something as drastic as an Uber to the power of Uber is what we need actually to make this happen.”
“In terms of AI, adoption is really happening now — it’s no longer just theoretical. Some factors that are helping this are first, the ecosystem. Second, the computing power, the actual physical infrastructure for all this that we have today.” said Satyakam Mohanty, founder of Lymbyc, an AI startup, which has been acquired by Larsen & Toubro Infotech. “One of the challenges is that people are looking at technologies instead of problems. This is creating a lot of silos. If instead, one looked at the problem that needs to be solved and then asked how AI can be applied, then you have a better way to solve this. To get to the world hunger solving stage, you have to industrialise AI, which doesn’t exist today.
Right now, all of the conversation we’re having is the mechanics of how do you make these things operational, but for adoption beyond or even within organizations for larger issues, we have to look at the risk factor.” Satyakam explains further. “Because, as with any technology or business process, there is always a risk factor, right? And the larger the organization, the greater the risk, therefore, slower the adoption that’s the standard paradigm, so how do you de-risk it?”
“At Tech Mahindra we always ask – How can I bring AI into it? The use cases that we did initially were more towards leading process automation or IT operations automation and so on. Now, we have also invested in an open-source AI project called Acumos with AT&T, one of our largest customers,” said George Mundassery, senior VP and global head, Automation and AI at Tech Mahindra. Acumos AI is a platform and open source framework that makes it easy to build, share, and deploy AI apps. Acumos standardises the infrastructure stack and components required to run an out-of-the-box general AI environment. This frees data scientists and model trainers to focus on their core competencies and accelerates innovation. “When it comes to applying the AI and making it more and more vibrant and applying it on the ground, I’m sure that that’s when the real benefits will start to be felt,” said George.
“Companies today have no option but to adopt digital. So at every point, they have to redesign their operations, be it their supply chain or the way they connect with various networks or with customer support. So that’s where those possibilities are enormous with AI,” said Prithvijit Roy, CEO and co-founder of BRIDGEi2i, a data analytics startup. “How can we embed AI in terms of creating our customer support without talking to customers, or how do you give the client or the customer what they need without having them articulate it? So there it’s not necessarily just customer experience. How do you train machines to learn on their own and create an application?” Roy said.
“A customer of mine made this statement: ‘Can we go AI with AI?’ What he meant was can we go ‘All In with AI’!” said Sayandeb Banerjee, CEO, and co-founder of a startup The Math Company. “And then we started talking about what is really stopping us from going AI with AI. The point that came out is the democratisation of the thinking is not happening as fast or the democratisation of the ideas is not happening as fast, which in my mind is what is creating a roadblock. Most of the time, my experience is that the roadblock is really the imagination and the visions of what can be done,” Banerjee said. “If you invariably have a good vision, good leader, things are moving, when you don’t have, everybody has access to the same technology, as we have access to the same platform. Why does it finally boils down to vision from a few?”
“When AI becomes more industrial and gets embedded in many places, the question of our biases becomes more important because you’re no longer thinking about them,” said Rohini Srivathsa, National Technology Officer at Microsoft India. “Take the case of an AI driven translator that interprets a doctor as he, and a nurse as she. We are coming to assume that that’s okay. We are not questioning it because it is so much a part of our thinking that the previous data has brought the pronoun to be changed to.”
Rohini continues, “So I think it creates a bigger question as AI becomes pervasive, industrialised and democratised. Are we putting in the right checks and balances? And when I talk to organisations about checks and balances, I think in some ways it is making us think about our own values first.”
“Eventually, what we are saying is that an algorithm is more about reimagining the decision making in your enterprise,” said Sameer Dhanrajani, CEO and co-founder of AIQRATE, an AI consultancy. “Now, if historically, all the decisions in the boards by the CXOs have been taken in the usual manner, in the conventional organisational structure, that may not be relevant anymore. But when you have an algorithm that works for you – embedded let’s say in the value chain of your business and doing a trade for you – it is, therefore, top of the mindshare for boardrooms, senior leaders, CXOs. Eventually, everyone is saying – look we want AI to revolutionise or reimagine our decision making.”
Sameer clarifies further, “if AI is about mimicking the human brain, organisations must have strategies, which are not defined piecemeal, isolated ad-hoc projects, or the Geek Squad. That’s a fundamental challenge.”
“Where AI is going, I think the new systems will be objective basically because you say I just want to increase my visitors to my store by 5 percent and that’s what it should do — help you make the right changes,” said Atul Batra, CTO at Manthan Software Service. “That’s the sophistication one is looking for. So basically, the systems are getting much more contextual for a specific business role like a merchandiser and store operation and so on. And one is seeing a lot of those systems deployed globally by a lot of vendors. I think that’s where it’s going – where there’s continuous feedback because you’re talking to the system and you’re getting feedback, and you’re helping evolve it.”
“We work to impact livelihoods across 14 disabilities. Purpose driven approach will make people do the right AI,” said Shanti Raghavan, founder of Enable India. “With AI, I’m expecting to be able to nudge people in their journey. Can I make them better at crowdsourcing solutions? We’ve done a lot of product management on this, like, how do you get more people to be like your TripAdvisor contributors, right? So we started introducing star users. The next time somebody comes on the program and says, you know what, I’m a star user, you can see that it’s making a difference. So, we have tons of data on how people are behaving on it, how often they log in, what do they actually listen to? We have all of that. We need AI to make sense of it. Now imagine all this data for the entire country; I cannot do this without having AI.”
“The human brain is not tuned towards trust very easily. So when you look at something physical, it’s very easy to understand. But now you come back and say that beyond the computer’s physical screen there is something, which sits on the cloud, which is a bot, which runs intelligence. I’m telling you, close to 100% will disagree.” Says Rohit Adlakha, chief digital and information officer and global head, Wipro HOLMES at Wipro. “The good part is we feel that AI is going to push the limits of the human brain to do much more than what you were able to do.”
“The challenge is that if a human is ultimately going to train a data set, which is going to train data set, you will always have your biases. So given the practical situation in mind, how do you make sure that you have a larger set of people, which will nullify each other’s biases?” says Rohit. “How do you balance it? How do you augment? How do you know humans and bots coexist? How do you make sure that both coexist and build the cast factor? I think we as an industry should push to move it from an enterprise scale to a global scale.”
“There is one more point out there, which is very, very topical today – that AI itself is not enough,” said Ritwik Batabyal, chief of technology and engineering head, Next-gen Business Products at Wipro. “Now we’re talking about these large, complex unresolved documents in office use cases. I mean somewhere algorithms the best of let’s say these kinds of models cannot solve. And in some way, I think there is a facet that is being understood by enterprises, which is can you bring in behavioral science? How do you design for a subconscious mind? You can have an algorithm, but if it’s not adopted, if it’s not implemented? What’s the use there?”
“I was certainly saying when you look at the most applied systems today, it’s getting better and better in terms of cognitive, but all said and done it is coming from some pattern,” said George Mundassery, senior VP and global head, Automation and AI at Tech Mahindra. “Today, I don’t think, it’s able to tell you that decision you made is the right one,” he said.
“There is no going back in this game; once the genie is out of the bottle we can’t put it back. So it’s like any other revolution, I think it’s going to happen,” says Prithvijit Roy. “The truth is that AI is going to take care of certain kinds of jobs that are repetitive. And that’s going to have an impact because mundane work is people’s livelihood in many parts.”
“So it’s not about replacing the human with other parts; it is making humans do certain things, which they were not able to do, which is the bigger part of the story, but this is going to be an impact in the short-term, which we all know how we will face it. So, it will work with enough time to balance out and will hopefully have better augmentation with it.”
– www.forbesindia.com
Related Posts
AIQRATIONS
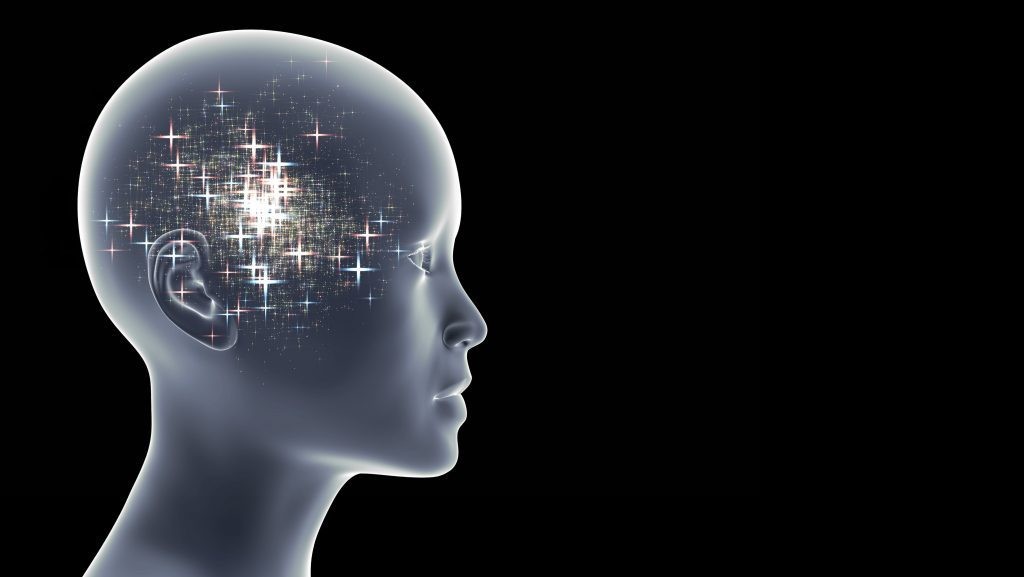
The ‘Dark’ side of AI: Algorithm Bias, influenced decision making.. Defining AI Ethics Strategy
Add Your Heading Text Here
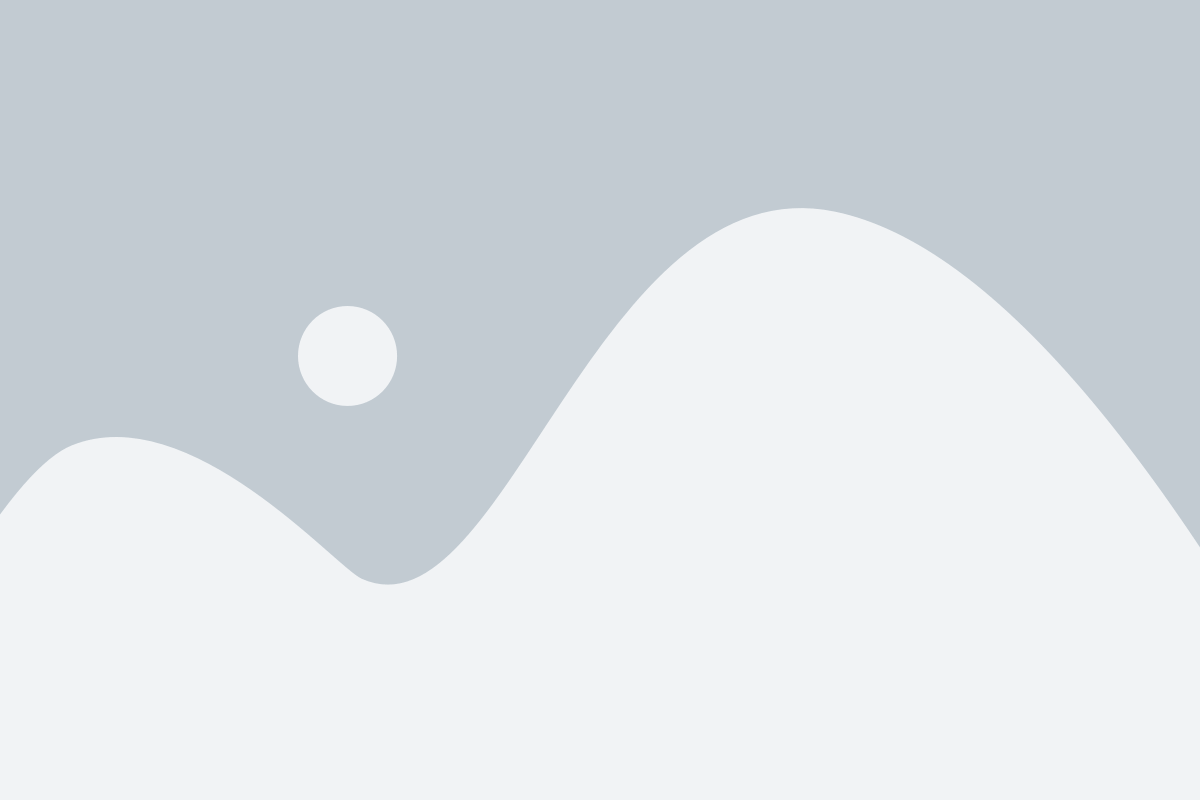
According to a 2019 report from the Centre for the Governance of AI at the University of Oxford, 82% of Americans believe that robots and AI should be carefully managed. Concerns cited ranged from how AI is used in surveillance and in spreading fake content online (known as deep fakes when they include doctored video images and audio generated with help from AI) to cyber attacks, infringements on data privacy, hiring bias, autonomous vehicles, and drones that don’t require a human controller.
What happens when injustices are propagated not by individuals or organizations but by a collection of machines? Lately, there’s been increased attention on the downsides of artificial intelligence and the harms it may produce in our society, from unequitable access to opportunities to the escalation of polarization in our communities. Not surprisingly, there’s been a corresponding rise in discussion around how to regulate AI.
AI has already shown itself very publicly to be capable of bad biases — which can lead to unfair decisions based on attributes that are protected by law. There can be bias in the data inputs, which can be poorly selected, outdated, or skewed in ways that embody our own historical societal prejudices. Most deployed AI systems do not yet embed methods to put data sets to a fairness test or otherwise compensate for problems in the raw material.
There also can be bias in the algorithms themselves and in what features they deem important (or not). For example, companies may vary their product prices based on information about shopping behaviors. If this information ends up being directly correlated to gender or race, then AI is making decisions that could result in a PR nightmare, not to mention legal trouble. As these AI systems scale in use, they amplify any unfairness in them. The decisions these systems output, and which people then comply with, can eventually propagate to the point that biases become global truth.
The unrest on bringing AI Ethics
Of course, individual companies are also weighing in on what kinds of ethical frameworks they will operate under. Microsoft president Brad Smith has written about the need for public regulation and corporate responsibility around facial recognition technology. Google established an AI ethics advisory council board. Earlier this year, Amazon started a collaboration with the National Science While we have yet to reach certain conclusions around tech regulations, the last three years have seen a sharp increase in forums and channels to discuss governance. In the U.S., the Obama administration issued a report in 2016 on preparing for the future of artificial intelligence after holding public workshops examining AI, law, and governance; AI technology, safety, and control; and even the social and economic impacts of AI. The Institute of Electrical and Electronics Engineers (IEEE), an engineering, computing, and technology professional organization that establishes standards for maximizing the reliability of products, put together a crowdsourced global treatise on ethics of autonomous and intelligent systems. In the academic world, the MIT Media Lab and Harvard University established a $27 million initiative on ethics and governance of AI, Stanford is amid a 100-year study of AI, and Carnegie Mellon University established a centre to explore AI ethics.
Corporations are forming their own consortiums to join the conversation. The Partnership on AI to Benefit People and Society was founded by a group of AI researchers representing six of the world’s largest technology companies: Apple, Amazon, DeepMind/Google, Facebook, IBM, and Microsoft. It was established to frame best practices for AI, including constructs for fair, transparent, and accountable AI. It now has more than 80 partner companies. Foundation to fund research to accelerate fairness in AI — although some immediately questioned the potential conflict of interest of having research funded by such a giant player in the field.
Are data regulations around the corner?
There is a need to develop a global perspective on AI ethics, Different societies around the world have very different perspectives on privacy and ethics. Within Europe, for example, U.K. citizens are willing to tolerate video camera monitoring on London’s central High Street, perhaps because of IRA bombings of the past, while Germans are much more privacy oriented, influenced by the former intrusions of East German Stasi spies , in China, the public is tolerant of AI-driven applications like facial recognition and social credit scores at least in part because social order is a key tenet of Confucian moral philosophy. Microsoft’s AI ethics research project involves ethnographic analysis of different cultures, gathered through close observation of behaviours, and advice from external academics such as Erin Meyer of INSEAD. Eventually, we could foresee that there will be a collection of policies about how to use AI and related technologies. Some have already emerged, from avoiding algorithmic bias to model transparency to specific applications like predictive policing.
The longer take is that although AI standards are not top of the line sought after work, they are critical for making AI not only more useful but also safe for consumer use. Given that AI is still young but quickly being embedded into every application that impacts our lives, we could envisage an array of AI ethics guidelines by several countries for AI that are expected to lead to mid- and long-term policy recommendations on AI-related challenges and opportunities.
Chief AI ethical officer on the cards?
As businesses pour resources into designing the next generation of tools and products powered by AI, people are not inclined to assume that these companies will automatically step up to the ethical and legal responsibilities if these systems go awry.
The time when enterprises could simply ask the world to trust artificial intelligence and AI-powered products is long gone. Trust around AI requires fairness, transparency, and accountability. But even AI researchers can’t agree on a single definition of fairness: There’s always a question of who is in the affected groups and what metrics should be used to evaluate, for instance, the impact of bias within the algorithms.
Since organizations have not figured out how to stem the tide of “bad” AI, their next best step is to be a contributor to the conversation. Denying that bad AI exists or fleeing from the discussion isn’t going to make the problem go away. Identifying CXOs who are willing to join in on the dialogue and finding individuals willing to help establish standards are the actions that organizations should be thinking about today. There comes the aspect of Chief AI ethical officer to evangelize, educate, ensure that enterprises are made aware of AI ethics and are bought into it.
When done correctly, AI can offer immeasurable good. It can provide educational interventions to maximize learning in underserved communities, improve health care based on its access to our personal data, and help people do their jobs better and more efficiently. Now is not the time to hinder progress. Instead, it’s the time for enterprises to make a concerted effort to ensure that the design and deployment of AI are fair, transparent, and accountable for all stakeholders — and to be a part of shaping the coming standards and regulations that will make AI work for all
Related Posts
AIQRATIONS

AI For CXOs — Redefining The Future Of Leadership In The AI Era
Add Your Heading Text Here
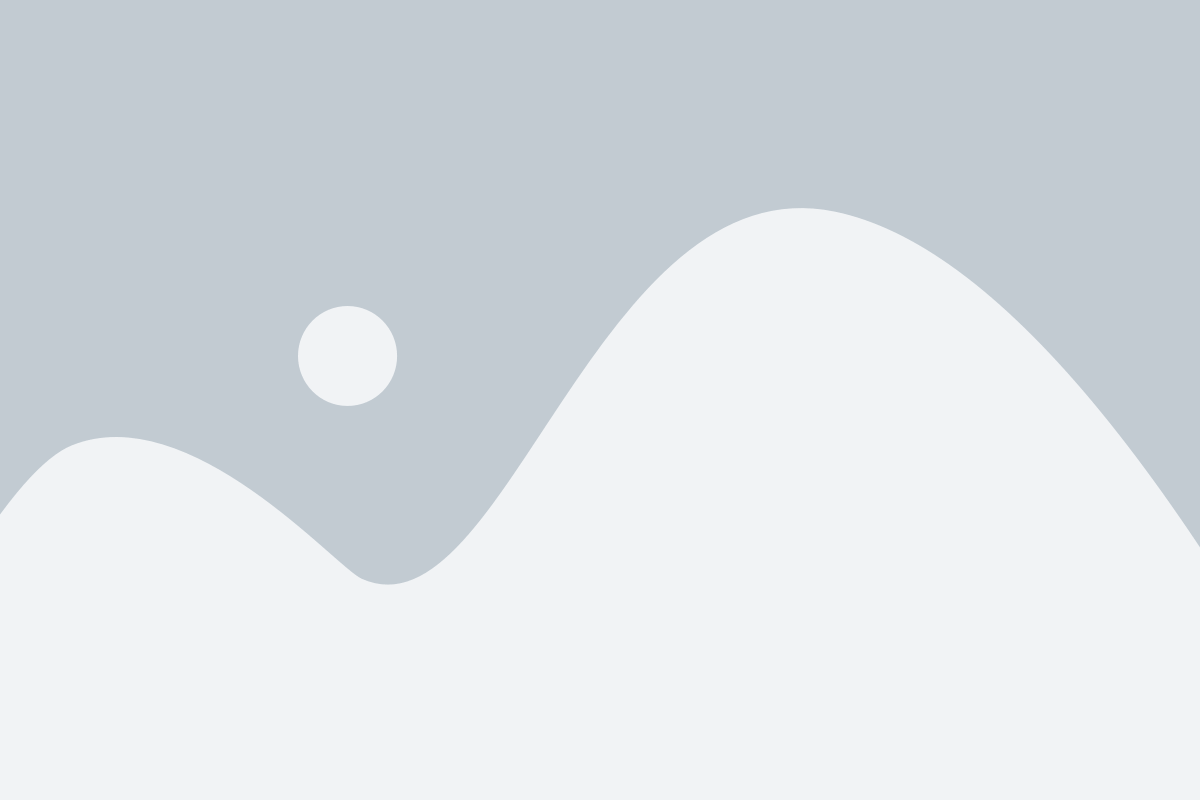
Artificial intelligence is getting ubiquitous and is transforming organizations globally. AI is no longer just a technology. It is now one of the most important lenses that business leaders need to look through to identify new business models, new sources of revenue and bring in critical efficiencies in how they do businesses.
Artificial intelligence has quickly moved beyond bits and pieces of topical experiments in the innovation lab. AI needs to be weaved into the fabric of business. Indeed, if you see the companies leading with AI today, one of the common denominators is that there is a strong executive focus around artificial intelligence. AI transformation can be successful when there is a strong mandate coming from the top and leaders make it a strategic priority for their enterprise.
Given AI’s importance to the enterprise, it is fair to say that AI will not only shape the future of the enterprise, but also the future for those that lead the enterprise mandate on artificial intelligence.
Curiosity and Adaptability
To lead with AI in the enterprise, top executives will need to demonstrate high levels of adaptability and agility. Leaders need to develop a mindset to harness the strategic shifts that AI will bring in an increasingly dynamic landscape of business – which will require extreme agility. Leaders that succeed in this AI era will need to be able to build capable, agile teams that can rapidly take cognizance of how AI can be a game changer in their area of business and react accordingly. Agile teams across the enterprise will be a cornerstone of better leadership in this age of AI.
Leading with AI will also require leaders to be increasingly curious. The paradigm of conducting business in this new world is evolving faster than ever. Leaders will need to ensure that they are on top of the recent developments in the dual realms of business and technology. This requires CXOs to be positively curious and constantly on the lookout for game changing solutions that can have a discernible impact on their topline and bottom-line.
Clarity of Vision
Leadership in the AI era will be strongly characterized by the strength and clarity with which leaders communicate their vision. Leaders with an inherently strong sense of purpose and an eye for details will be forged as organizations globally witness AI transformation.
It is not only important for those that lead with AI to have a clear vision. It is equally important to maintain a razor sharp focus on the execution aspect. When it comes to scaling artificial intelligence in the organization, the devil is very often in the details – the data and algorithms that disrupt existing business processes. For leaders to be successful, they must remain attentive to the trifecta of factors – completeness of their vision for AI transformation, communication of said vision to relevant stakeholders and monitoring the entire execution process. While doing so, it is important to remain agile and flexible as mentioned in my earlier section – in order to be aware of possible business landscape shifts on the horizon.
Engage with High EQ
AI transformation can often seem to be all about hard numbers and complex algorithms. However, leaders need to also infuse the human element to succeed in their efforts to deliver AI @ Scale. The third key for top executives to lead in the age of AI is to ensure that they marry high IQs with equally or perhaps higher levels of EQ.
Why is this so very important? Given the state of this technology today, it is important that we build systems that are completely free of bias and are fair in how they arrive at strategic and tactical decisions. AI learns from the data that it is provided and hence it is important to ensure that the data it is fed is free from bias – which requires a human aspect. Secondly, AI causes severe consternation among the working population – with fears of job loss abounding. It is important to ensure that these irrational fears of an ‘AI Takeover’ are effectively abated. For AI to be successful, it is important that both types of intelligence – artificial and human – symbiotically coexist to deliver transformational results.
AI is undoubtedly going to become one of the sources of lasting competitive advantage for enterprises. According to research, 4 out of 5 C-level executives believe that their future business strategy will be informed through opportunities made available by AI technology. This requires a leadership mindset that is AI-first and can spot opportunities for artificial intelligence solutions to exploit. By democratizing AI solutions across the organization, enterprises can ensure that their future leadership continues to prioritize the deployment of this technology in use cases where they can deliver maximum impact.
Related Posts
AIQRATIONS
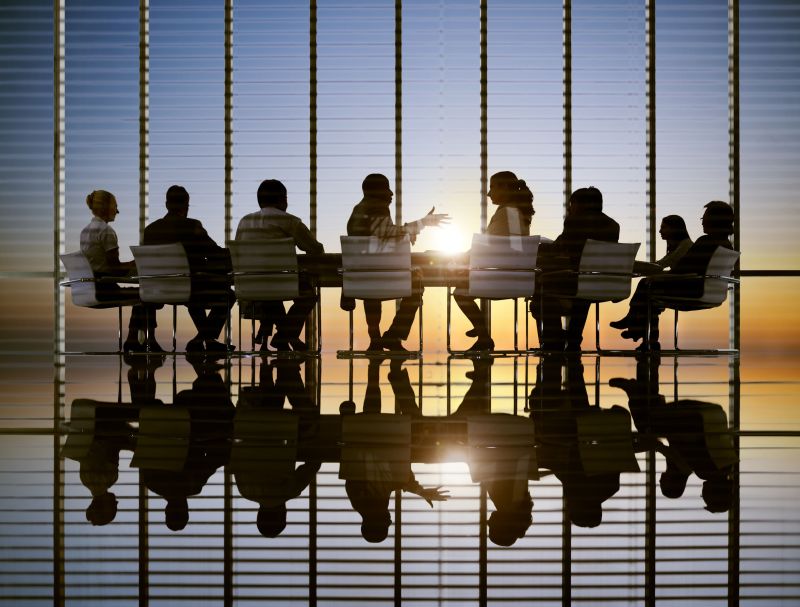
How CXOs are Leveraging AI to Pivot Business Strategy and Operational Models
Add Your Heading Text Here
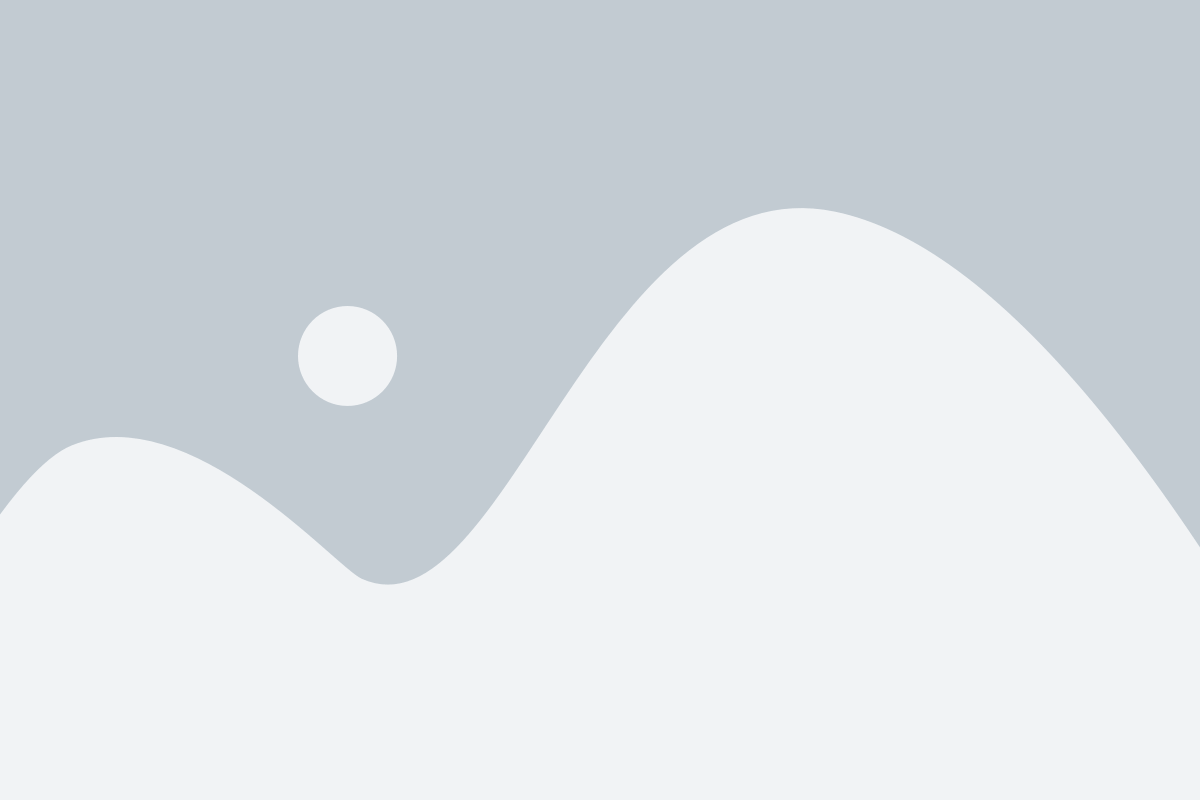
AlphaGo caused a stir by defeating 18-time world champion Lee Sedol in Go, a game thought to be impenetrable by AI for another 10 years. AlphaGo’s success is emblematic of a broader trend: An explosion of data and advances in algorithms have made technology smarter than ever before. Machines can now carry out tasks ranging from recommending movies to diagnosing cancer — independently of, and in many cases better than, humans. In addition to executing well-defined tasks, technology is starting to address broader, more ambiguous problems. It’s not implausible to imagine that one day a “strategist in a box” could autonomously develop and execute a business strategy. We’ve spoken to CXOs and leaders who express such a vision — and companies such as Amazon and Alibaba are already beginning to make it a reality.
Business Processes – Increasing productivity by reducing disruptions
AI algorithms are not natively “intelligent.” They learn inductively by analyzing data. While most leaders are investing in AI talent and have built robust information infrastructures,
As Airbus started to ramp up production of its new A350 aircraft, the company faced a multibillion-euro challenge. The plan was to increase the production rate of that aircraft faster than ever before. To do that, they needed to address issues like responding quickly to disruptions in the factory. Because they will happen. Airbus turned to artificial intelligence. It combined data from past production programs, continuing input from the A350 program, fuzzy matching, and a self-learning algorithm to identify patterns in production problems.
AI led to rectification of about 70% of the production disruptions for Airbus, by matching to solutions used previously — in near real time.
Just as it is enabling speed and efficiency at Airbus, AI capabilities are leading directly to new, better processes and results at other pioneering organizations. Other large companies, such as BP, Infosys, Wells Fargo, and Ping An Insurance, are already solving important business problems with AI. Many others, however, have yet to get started.
Integrated Strategy Machine – The Implementation Scope Augmented AI
The integrated strategy machine is the AI analog of what new factory designs were for electricity. In other words, the increasing intelligence of machines could be wasted unless businesses reshape the way they develop and execute their strategies. No matter how advanced technology is, it needs human partners to enhance competitive advantage. It must be embedded in what we call the integrated strategy machine. An integrated strategy machine is the collection of resources, both technological and human, that act in concert to develop and execute business strategies. It comprises a range of conceptual and analytical operations, including problem definition, signal processing, pattern recognition, abstraction and conceptualization, analysis, and prediction. One of its critical functions is reframing, which is repeatedly redefining the problem to enable deeper insights.
Amazon represents the state-of-the-art in deploying an integrated strategy machine. It has at least 21 data science systems, which include several supply chain optimization systems, an inventory forecasting system, a sales forecasting system, a profit optimization system, a recommendation engine, and many others. These systems are closely intertwined with each other and with human strategists to create an integrated, well-oiled machine. If the sales forecasting system detects that the popularity of an item is increasing, it starts a cascade of changes throughout the system: The inventory forecast is updated, causing the supply chain system to optimize inventory across its warehouses; the recommendation engine pushes the item more, causing sales forecasts to increase; the profit optimization system adjusts pricing, again updating the sales forecast.
Manufacturing Operations – An AI assistant on the floor
CXOs at industrial companies expect the largest effect in operations and manufacturing. BP plc, for example, augments human skills with AI in order to improve operations in the field. They have something called the BP well advisor that takes all of the data that’s coming off of the drilling systems and creates advice for the engineers to adjust their drilling parameters to remain in the optimum zone and alerts them to potential operational upsets and risks down the road. They are also trying to automate root-cause failure analysis to where the system trains itself over time and it has the intelligence to rapidly assess and move from description to prediction to prescription.
Customer-facing activities – near real time scoring
Ping An Insurance Co. of China Ltd., the second-largest insurer in China, with a market capitalization of $120 billion, is improving customer service across its insurance and financial services portfolio with AI. For example, it now offers an online loan in three minutes, thanks in part to a customer scoring tool that uses an internally developed AI-based face-recognition capability that is more accurate than humans. The tool has verified more than 300 million faces in various uses and now complements Ping An’s cognitive AI capabilities including voice and imaging recognition.
AI Strategy for Different Operational Models
To make the most of this technology implementation in various business operations in your enterprise, consider the three main ways that businesses can or will use AI:
Assisted intelligence
Now widely available, improves what people and organizations are already doing. For example, Google’s Gmail sorts incoming email into “Primary,” “Social,” and “Promotion” default tabs. The algorithm, trained with data from millions of other users’ emails, makes people more efficient without changing the way they use email or altering the value it provides. Assisted intelligence tends to involve clearly defined, rules-based, repeatable tasks.
Assisted intelligence apps often involve computer models of complex realities that allow businesses to test decisions with less risk. For example, one auto manufacturer has developed a simulation of consumer behavior, incorporating data about the types of trips people make, the ways those affect supply and demand for motor vehicles, and the variations in those patterns for different city topologies, marketing approaches, and vehicle price ranges. The model spells out more than 200,000 variations for the automaker to consider and simulates the potential success of any tested variation, thus assisting in the design of car launches. As the automaker introduces new cars and the simulator incorporates the data on outcomes from each launch, the model’s predictions will become ever more accurate.
Augmented intelligence
Augmented Intelligence, emerging today, enables organizations and people to do things they couldn’t otherwise do. Unlike assisted intelligence, it fundamentally alters the nature of the task, and business models change accordingly.
For example, Netflix uses machine learning algorithms to do something media has never done before: suggest choices customers would probably not have found themselves, based not just on the customer’s patterns of behavior, but on those of the audience at large. A Netflix user, unlike a cable TV pay-per-view customer, can easily switch from one premium video to another without penalty, after just a few minutes. This gives consumers more control over their time. They use it to choose videos more tailored to the way they feel at any given moment. Every time that happens, the system records that observation and adjusts its recommendation list — and it enables Netflix to tailor its next round of videos to user preferences more accurately. This leads to reduced costs and higher profits per movie, and a more enthusiastic audience, which then enables more investments in personalization (and AI).
Autonomous intelligence
Being developed for the future, Autonomous Intelligence creates and deploys machines that act on their own. Very few autonomous intelligence systems — systems that make decisions without direct human involvement or oversight — are in widespread use today. Early examples include automated trading in the stock market (about 75 percent of Nasdaq trading is conducted autonomously) and facial recognition. In some circumstances, algorithms are better than people at identifying other people. Other early examples include robots that dispose of bombs, gather deep-sea data, maintain space stations, and perform other tasks inherently unsafe for people.
As you contemplate the introduction of artificial intelligence, articulate what mix of the three approaches works best for you.
- Are you primarily interested in upgrading your existing processes, reducing costs, and improving productivity? If so, then start with assisted intelligence, probably with a small group of services from a cloud-based provider.
- Do you seek to build your business around something new — responsive and self-driven products, or services and experiences that incorporate AI? Then pursue an augmented intelligence approach, probably with more complex AI applications resident on the cloud.
- Are you developing a genuinely new technology? Most companies will be better off primarily using someone else’s AI platforms, but if you can justify building your own, you may become one of the leaders in your market.
The transition among these forms of AI is not clean-cut; they sit on a continuum. In developing their own AI strategy, many companies begin somewhere between assisted and augmented, while expecting to move toward autonomous eventually.
Related Posts
AIQRATIONS
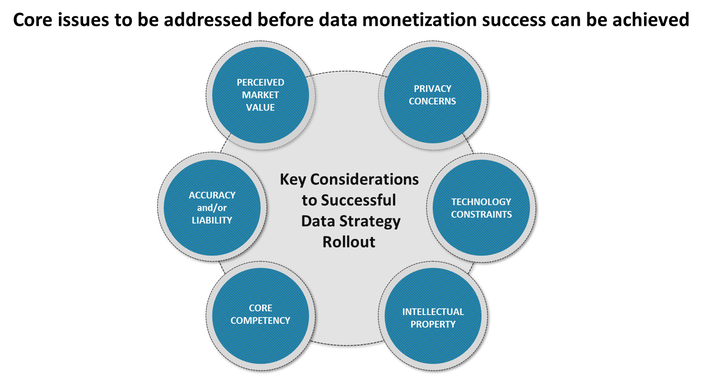
Building a Robust Data Strategy Roadmap – Part III
Add Your Heading Text Here
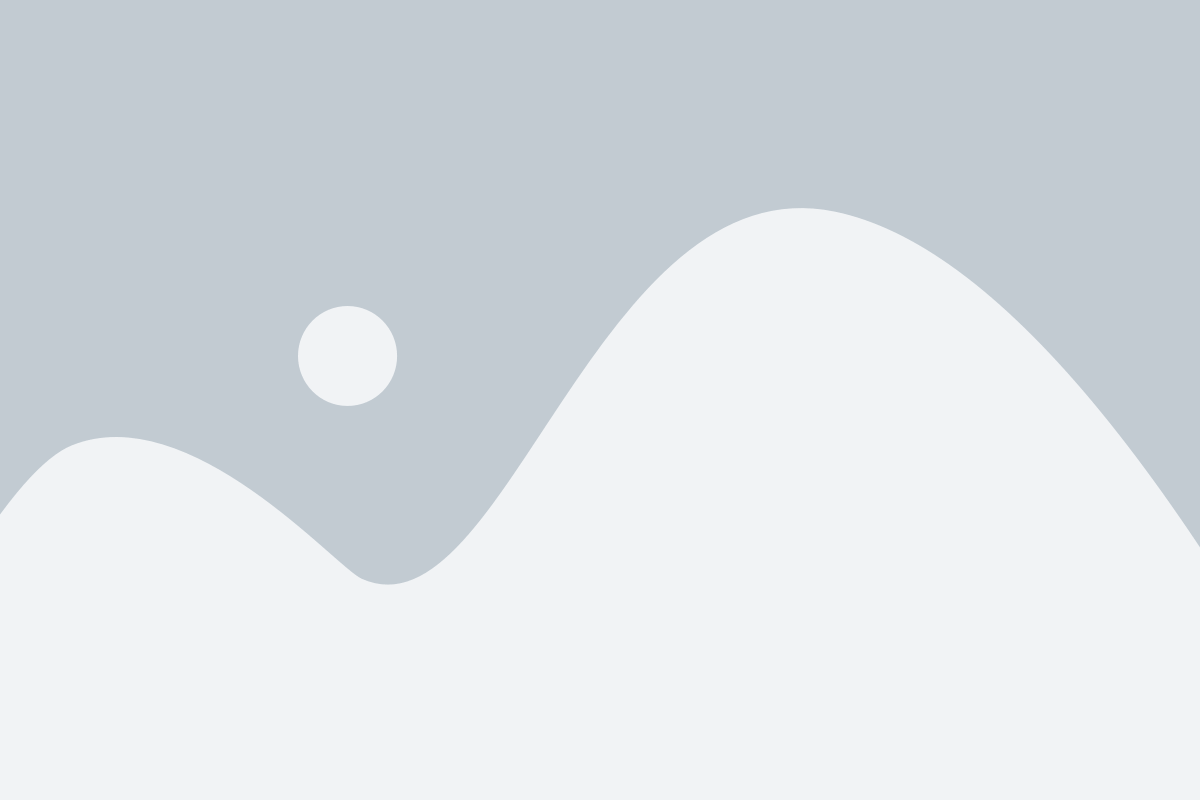
In continuation to my last article on Building a Robust Data Strategy, let me meaningfully conclude it by highlighting some of the core issues which need to be addressed before data monetization could really be called our as a success and ROI is achieved.
Privacy Concerns
Company needs to have the implicit and/or explicit statutory or legal right, or the ethical right, to divulge private consumer data – either personalized or depersonalized, individualized or at an aggregated level. Especially in industries where regulatory bodies have a heavy clout over what data is being used to cull out actionable insights or even the data flow within or beyond the walls of the organizations. Numerous articles, reports & surveys have highlighted how crucial is for businesses to operate within the ethical boundaries of data gathering or dissemination. Leave no stone unturned to see what policies/restrictions/guidelines are in place for the industry you operate in, how easy/difficult is to access data, and what are customer or end user reactions. You definitely do not intend to burn bridges with your existing customer base or repel away new prospects. Legal actions can be fatal to business at times. Be doubly sure what you are up for!
Technology Constraints
Do have a thorough understanding of the technological or hardware-related considerations to implement the strategy chosen to monetize the data. At times, organizations don’t have the requisite resources to execute on their strategy, may be because that’s not their core area of operation or it’s happening in silo’es across the organization which the business unit in question is not privy to. A complete landscaping exercise to understand the current state of business, what’s new in the market & what the competition is up to, what’s the future state & a step-by-step roadmap to mature technological prowess. In many cases, businesses hire external consultants or seek handholding by analytics service providers who have the requisite experience in recommending about the gaps & even executing on filling those. A thorough detailed analysis (but not analysis-paralysis) is crucial to the overall success.
Intellectual Property
At times, organizations sitting on huge pile of valuable data choose to make it available in the market (as another viable revenue model to monetize data). How much data to sell and how to determine costs vs. benefits in putting valuable data on the open market should be thought through. Be privy to the pros & cons of each approach & choose your business model accordingly.
Core Competency
Depending on its core competency, organization needs to identify at which level it wants to monetize the data in the data value chain. Data at each & every touchpoint in the value chain may have its own peculiar problems (missing data, incorrect data etc) and not all of it may be relevant. If your differentiator is “speedy delivery” of goods to your customers, focus on picking the right data sets across the value chain which helps streamlining operations, optimize inventory & transit time. Know what you are best at or what you are known for in the market and harness data capabilities to strengthen your business on that front.
Data Accuracy and/or Liability
Potential problems with inaccurate or directly or indirectly regulated data insights or products hitting the market place. Make sure that data assimilation, aggregation & cleansing exercise is robust enough to ensure the analysis/insights being generated out of it have a high probability of giving the right sense of direction to the business. At times, over-ambitious expectations or poor data quality can directly impact the quality of the outcomes. Garbage-in Garbage-out is the mantra & business managers should perfectly understand the gaps in the data & be cautious before making any solid commitments.
Perceived Market Value
For larger market opportunities, it is likely that an organization would want to play at the higher level in the data value chain. Umpteen times that completely derails the whole Analytics ROI & data monetization exercise. Focus should be specifically on business model(s) used to monetize the data than otherwise.
All the aforementioned considerations should set a good pretext to the data monetization exercise and may be the key to unlocking true value from data strategy initiative. In my subsequent edition, I shall bring to light the “Analytics Centre of Excellence” concept & how can organizations setup a full-fledged Analytics unit to deliver insights to departmants/LOB’s/functions across the business and also serve as a backbone to building a data-driven organization of the future. Stay tuned !
Related Posts
AIQRATIONS
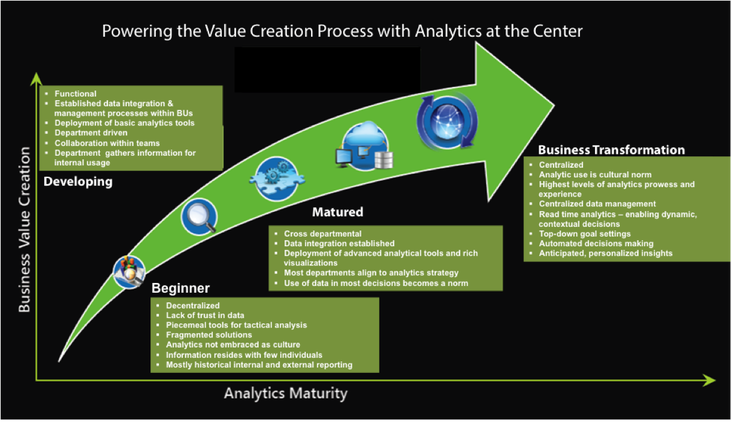
Building a Robust Data Strategy Roadmap – Part II
Add Your Heading Text Here
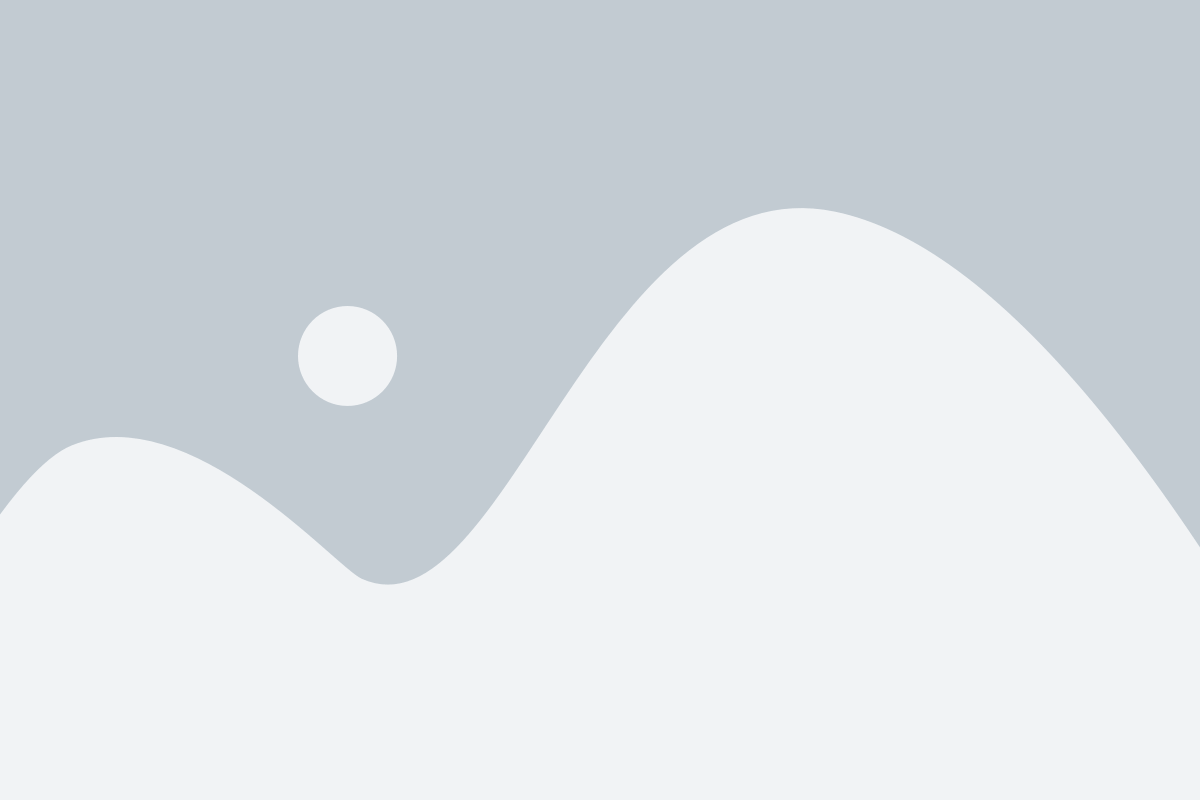
Unarguably, data and technology is truly redefining & rehashing the way companies do business. Organizations have always had data, which they have utilized to run their businesses more efficiently but recent developments have transformed the way data is utilized by such organizations.
In today’s disruptive economic environment, all leaders are vying for identifying new revenue streams and identifying existing value streams inside the organization especially data. This is where the concept of crafting a Robust Data Strategy comes in, how do we make most of the Dark Data ? Data is now being looked as an asset and business models are now being build around this vast value pool which is hidden inside the data being stored. Enterprises are now anticipating future needs based on preference insights culled out from past & present data. They are creating new products and services in tune with what their customers exactly seek. They are lending an ear to all suggestions/recommendations/feedback shared and also responding to queries/concerns in real time. They are doing it all with data and analytics.
While many companies are becoming aware of the opportunities embedded in their enterprise data, only a few have developed active strategies to monetize it successfully. Data Strategy requires companies to not only understand their data, but also to uncover gaps and evaluate suitable business model(s) for appropriately monetizing the enterprise data. To evaluate their respective monetization opportunities in a more informed and results-driven manner, companies need to assess the value of enterprise data, determine how best to maximize its potential and figure out how to get the data to the market efficiently.
Four Stages to Analytics Sophistication
Based on the current state of data affairs, any organization can be categorized as a beginner, developing, matured or leader. In the initial stages of transformation, organization typically
lacks synergies due to silo’ed efforts, is less agile and more prone to errors, with perennial data quality concerns. As they mature to be leaders in the Analytics space, data sits at the heart of business, with increasingly automated, instant, accurate and seamless data driven decision-making.
- Beginner: Basic infrastructure and tools, proliferation of dashboards and reports
- Developing: Building tools and processes for historical as well as deep diving analysis to gain some insights for future actions
- Matured: Organization adoption of advanced analytical capabilities to predict future outcomes
- Business Transformation or Leader: Centralized analytics focus with capabilities to anticipate future and act in a data driven manner
Time’s ripe to ride on the Data & Analytics wave
Enterprises capture a lot of data, most of which is often overlooked. With reducing costs of capturing and storing data, increasing data analysis capabilities and superior analytical technologies available, enterprises have started to recognize data as one of their most valuable assets. In the few years, enterprises who lead the way in reorienting their approach, initiating enterprise wide data-led transformations and effectively monetizing their data are expected to be in the forefront. Typical market forces driving widespread adoption of Analytics are:
Technological Advancement
- Technology advancement has facilitated real time data analysis and personalized communication
- Big data technologies, cloud computing, machine intelligence and other advancements etc. have made analysis simpler & efficient
Rise of Consumerism
- Influx of more demanding consumers will force a wave of change
- Consumer engagement and experience management are key levers to success
Data Explosion
- Daily volume of data being captured increasing rapidly
- Cost of storing data decreasing massively
- Recognition of amount of under-utilized data that can be used to derive additional value
Increasing importance of Analytics & BI
- Business Intelligence and Analytics becoming an integral part of organization’s decision making
Economic Pressures
- Pressure on profit margins are forcing increased focus on efficiency and cost reduction
- Increasing competitive pressure
Is your Data truly worth it?
How much business value can be created via data on which organizations are sitting on depends primarily on the following factors & to an extent determines the success of any Analytics initiative.
Predict Behaviour (Patterns)
Enterprise data should be detailed enough to build a successful data monetization strategy. E.g. Customer data should be detailed enough to be able to predict customer behavior, patterns etc.
Size of the Ecosystem
Businesses with high volume, large breadth of data have the ability to generate highest value from the data. Companies with national or global scale can easily establish market view, which makes it more meaningful and valuable
Accessibility and Actionable
Data becomes valuable only if its rich, actionable and accessible. Structured, & readily scalable data makes the process of monetization simpler and efficient, providing higher potential for data monetization
Customer Identification (Granularity)
Data becomes valuable only if it is granular enough to be able to identify the end user/ customer. Ability to identify/ profile customers helps in expanding the range of products and servives that can be offered
Uniqueness
Uniqueness of the enterprise data is extremely valuable. It makes the products/services offered by the enterprise exclusive to the enterprise, sustainable differentiation which most organization yearn for
Stages to Data Maturity
Based on maturity of organization’s data, it can take a call what kind of a player it wants to be in the market – a “data seller” or a “full services provider”.
Raw Data
- Selling raw unprocessed data to outside stakeholders
- Companies with rich pool of high quality raw data can onsell such data with little investment required
E.g. – Pharma related data or even NASDAQ’s “Data on Demand” service to its ecosystem of partners in the capital markets
Processed Data
- Companies collect and integrate data from multiple sources
- Data is processed, stored and leveraged in summary form
- Secure capture and transport of data
- Proper storage and management of data using a data platform
E.g. Card Advisory companies provide processed data to merchants and/ or use it for improving its operational efficiency
Business Intelligence/ Predictive Insights
- Tools and technologies such as data mining, predictive modeling and analytics convert data into insights
- Insights are made available to the stakeholders (both internal and external) to drive business decisions
E.g. Wal-Mart segments its customers into three primary groups based on purchasing patterns to spur growth
Products & Solutions Implementation
- Data-driven interactions with end users
- APIs and ability for companies to access platform and data to build comprehensive products and solutions
- Companies use the intelligence to improve product and solutions offering portfolio
E.g. Tesco bank uses Clubcard customer data to identify customer needs and creates new personalized offers
Key Elements to Designing a Robust Data Strategy
Unravel Customer Needs
- Continually understand the customer needs to unearth customer requirements and preferences
- Understanding the delivery and integration models that clients require in order to benefit from enhancements
- Create a business model which fits into the core competency and create offerings which fir into client platforms and applications
- Invest in continuous learning and management of customers’ unmet needs ranging from enhancements to new products/ solutions
Decrypting the Enterprise Data
- Understand the enterprise data captured across all business lines and develop an enterprise wide nomenclature for the same
- Identify and map data and analytics services across business units to understand what types of capabilities can be leveraged to build new products and services using the appropriate business model
Gauging the Market Potential
- Calculate the market potential for the various opportunities identified
- Estimate the revenue potential, internal rate of return, investment required, cost reduction, efficiency etc. for the process
- Understand the key competition, factor in macro and micro factor which can affect the marketplace demand
- Seek out opportunities to enhance the core business or develop new products and services.
Deciphering the Value Chain
- Develop insights into partners and competitors across the value chain including upstream suppliers, data partners etc.
- Identify the new opportunities that can be available across the value chain
- Create a comprehensive view of the data ecosystem
Enhance the existing infrastructure
- Develop a sophisticated yet flexible architecture, suitable technology and applications which can help unlock the value that the opportunities might presents
- Put in place a data infrastructure that can provide the necessary foundation to enable the organization to unlock the value of data assets
The crux of the matter is that with the huge amount of data available with the enterprise’s in today’s competitive and converging business environment, they should start looking for market opportunities leveraging the data available with them. Most of the enterprises still do not consider data as an asset which they can monetize if they choose the correct business strategy and build the required capabilities. Enterprises can not only make better use of their internal data to enhance the current product and services portfolio, it can also provide new insights into the value chain and could transform the enterprise, unleashing a whole new set of products and services for the customers.
By utilizing internal data with external data, powerful generation of high margin solutions can be developed which can transform an entire organization which possesses enormous revenue potential. Done properly, data ecosystems can fund the transformation, create value for customers, and build long lasting relationships with other partners firms, 3rd party vendors & suppliers. But to ensure the true value of data is being monetized by the enterprise, it is essential that it follows a streamlined process to identify the most suitable business model(s) taking into account all constraints which the process might need addressing.