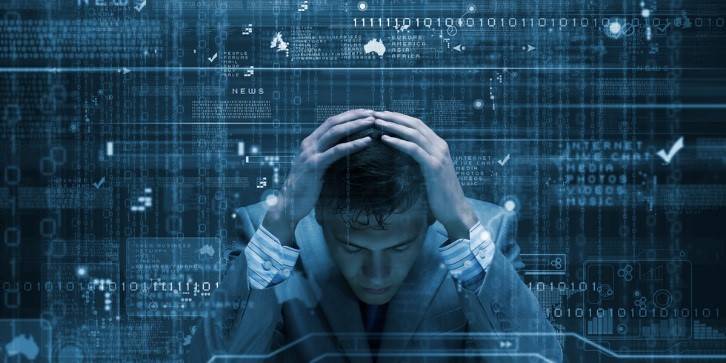
Bring in Effective Data Norms
Add Your Heading Text Here
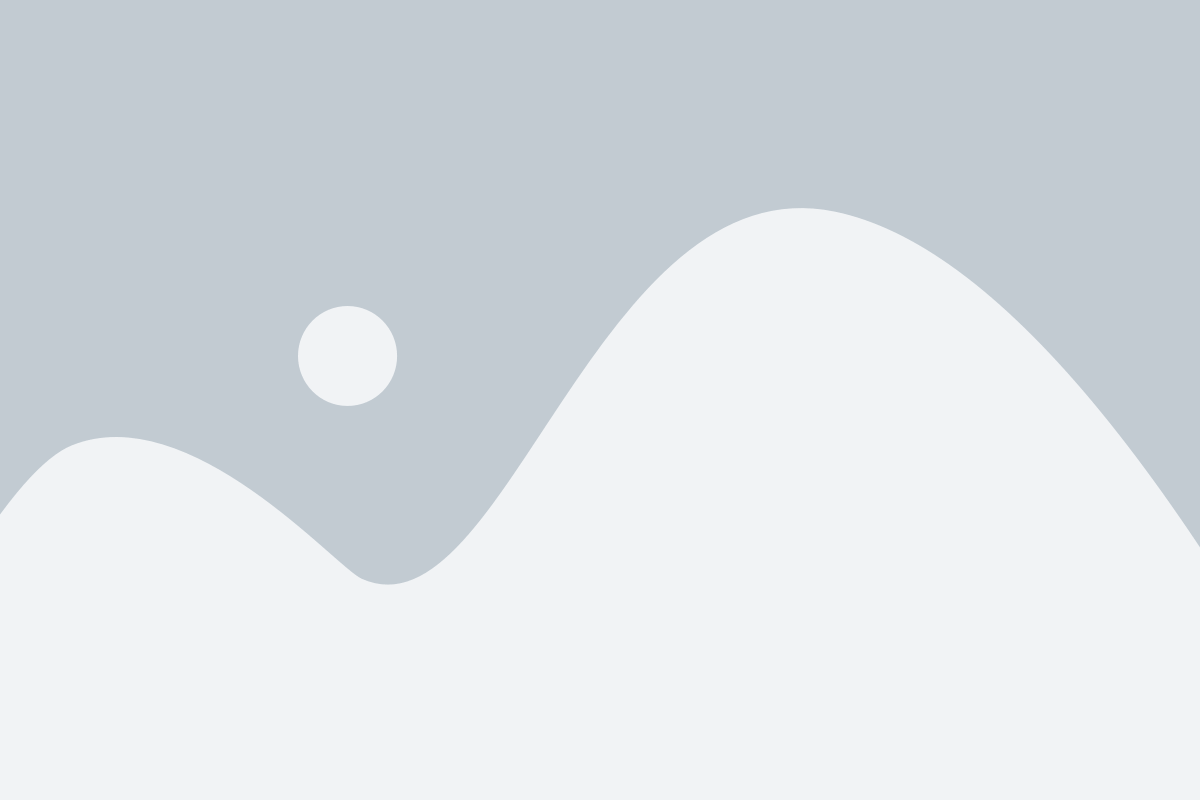
What constitutes ‘fair use’ of data is increasingly coming under scrutiny by regulators across the world. With the digital detonation that has been unleashed in the past few years, leading to a deluge of data – organisations globally have jumped at the prospect of achieving competitive advantage through more refined data mining methods. In the race for mining every bit of data possible and using it to inform and improve algorithmic models, we have lost sight of what data we should be collecting and processing. There also seems to be a deficit of attention to what constitutes a breach and how offending parties should be identified and prosecuted for unfair use.
There’s growing rhetoric that all these questions be astutely addressed through a regulation of some form. With examples of detrimental use of data surfacing regularly, businesses, individuals and society at large are demanding an answer for exactly what data can be collected – and how it should be aggregated, stored, managed and processed.
If data is indeed the new oil, we need to have a strong understanding of what constitutes the fair use of this invaluable resource. This article attempts to highlight India’s stance on triggering regulatory measures to govern the use of data.Importance of Data Governance
Importance of Data Governance
Before we try to get into what data governance should mean in the Indian context, let us first look at the definition of data governance and why it is an important field of study to wrap our head around.
In simple terms, data governance is the framework that lays down the strategy of how data is used and managed within an organisation. Data governance leaders must stay abreast of the legal and regulatory frameworks specific to the geographies that they operate in and ensure that their organisations are compliant with the rules and regulations. A lot of their effort at present is aimed at maintaining the sanctity of organisational data and ensuring that it does not fall in the wrong hands. As such, the amount of time and effort expended on ensuring that these norms are adequately adhered to is contingent upon the risk associated with a potential breach or loss of data.
In effect, a framework of data governance is intended to ensure that a certain set of rules is applied and enforced to ensure that data is used in the right perspective within an organisation.
Data Governance in Indian Context
India is rapidly moving towards digitisation. Internet connectivity has exploded in the last few years, leading to rapid adoption of internet-enabled applications — social media, online shopping, digital wallets etc. The result of this increasing connectivity and adoption is a fast-growing digital footprint of Indian citizens. Add to this the Aadhaar programme proliferation and adoption – and we have almost every citizen that has personal digital footprint somewhere – codified in the form of data.
With a footprint of this magnitude, there is an element of risk attached. What if this data falls in the wrong hands? What if personal data is used to manipulate citizens? What are the protection mechanisms citizens have against potential overreach by stewards of the data themselves? It is time we found answers to these very pertinent questions – and data governance regulation is the way we will find comprehensive answers to these impending conversations
Perspectives for India
The pertinent departments are mulling over on a collective stand that should be taken while formulating data governance norms. For one, Indian citizens are protected by a recent Supreme Court ruling that privacy is a fundamental right. This has led to a heightened sense of urgency around arriving at a legislative framework for addressing genuine concerns around data protection and privacy, as well as cybersecurity.
As a result of these concerns, the Central government recently set up a committee of experts, led by Justice BN Srikrishna, tasked with formulating data governance norms. This committee is expected to maintain the delicate balance between protecting the privacy of citizens and fostering the growth of the digital economy simultaneously. Their initial work – legal deliberations and benchmarking activity against similar legal frameworks such as GDPR (General Data Protection Regulation) – has resulted in the identification of seven key principles around which any data protection framework needs to be built. Three of the most crucial pointers include:
1. Informed Consent: Consent is deemed to be an expression of human autonomy. While collecting personal data, it is critical that the users be informed adequately about the implications around how this data is intended to be used before capturing their express consent to provide this data
2. Data Minimisation: Data should not be collected indiscriminately. Data collected should be minimal and necessary for purposes for which the data is sought and other compatible purposes beneficial for the data subject.
3. Structured Enforcement: Enforcement of the data protection framework must be by a high-powered statutory authority with sufficient capacity. Without statutory authority, any remedial measures sought by citizens over data privacy infringement will be meaningless.
Striking the right balance between fostering an environment in which the digital economy can grow to its full potential, whilst protecting the rights of citizens is extremely difficult.
With a multitude of malafide parties today seeking to leverage personal data of citizens for malicious purposes, it is crucial that the government and the legal system set out a framework that protects the sovereignty and interests of the people. By allaying fears of misuse of data, the digital economy will grow as people become less fearful and more enthusiastically contribute information where a meaningful end outcome can be achieved.
Related Posts
AIQRATIONS
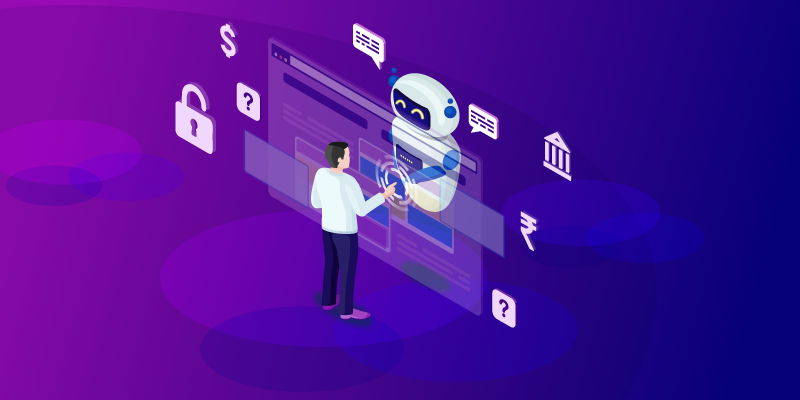
How artificial intelligence is changing the face of banking in India
Add Your Heading Text Here
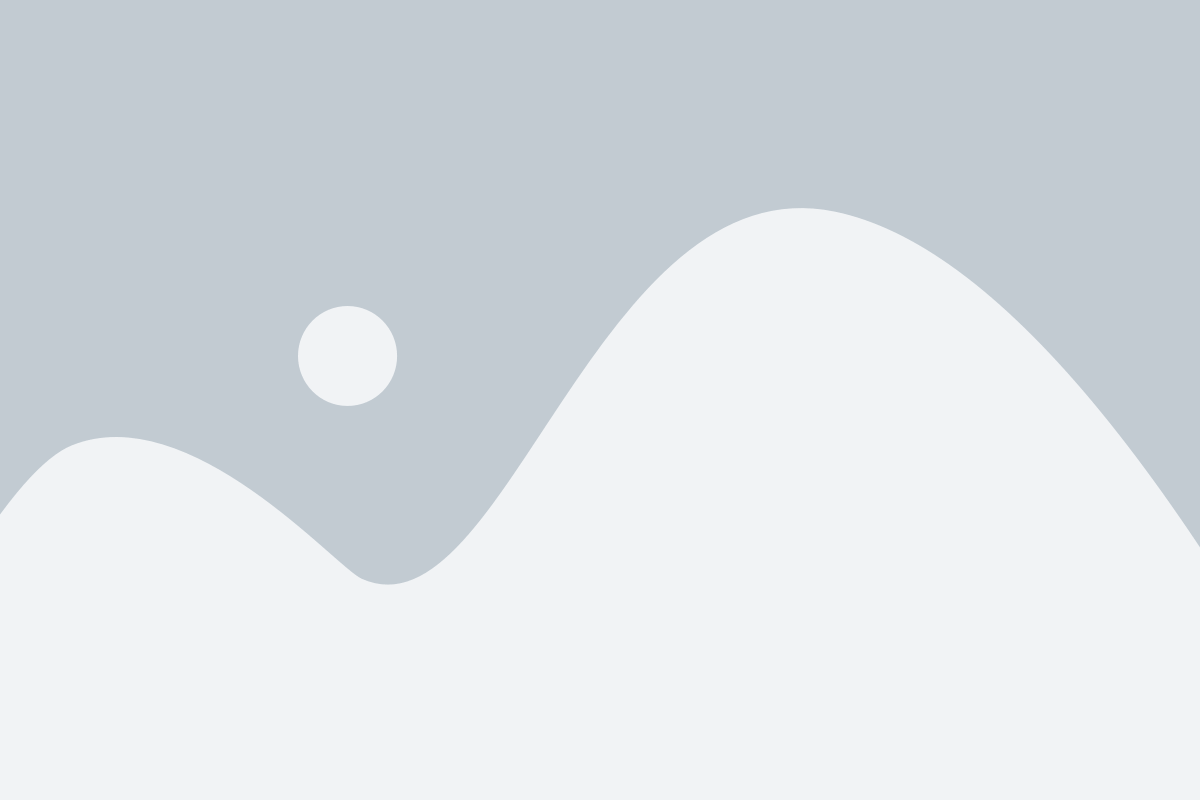
Artificial intelligence (AI) will empower banking organisations to completely redefine how they operate, establish innovative products and services, and most importantly impact customer experience interventions. In this second machine age, banks will find themselves competing with upstart fintech firms leveraging advanced technologies that augment or even replace human workers with sophisticated algorithms. To maintain a sharp competitive edge, banking corporations will need to embrace AI and weave it into their business strategy.
In this post, I will examine the dynamics of AI ecosystems in the banking industry and how it is fast becoming a major disrupter by looking at some of the critical unsolved problems in this area of business. AI’s potential can be looked at through multiple lenses in this sector, particularly its implications and applications across the operating landscape of banking. Let us focus on some of the key artifiicial intelligence technology systems: robotics, computer vision, language, virtual agents, and machine learning (including deep learning) that underlines many recent advances made in this sector.
Industry Changes
Banks entering the intelligence age are under intense pressure on multiple fronts. Rapid advances in AI are coming at a time of widespread technological and digital disruption. To manage this impact, many changes are being triggered.
- Leading banks are aggressively hiring Chief AI Officers while investing in AI labs and incubators
- AI-powered banking bots are being used on the customer experience front.
- Intelligent personal investment products are available at scale
- Multiple banks are moving towards custom in-house solutions that leverage sophisticated ontologies, natural language processing, machine learning, pattern recognition, and probabilistic reasoning algorithms to aid skilled employees and robots with complex decisions
Some of the key characteristics shaping this industry include:
- Decision support and advanced algorithms allow the automation of processes that are more cognitive in nature
- Solutions incorporate advanced self-learning capabilities
- Sophisticated cognitive hypothesis generation/advanced predictive analytics
Surge of AI in Banking
Banks today are struggling to reduce costs, meet margins, and exceed customer expectations through personal experience. To enable this, implementing AI is particularly important. And banks have started embracing AI and related technologies worldwide. According to a survey by the National Business Research Institute, over 32 percent of financial institutions use AI through voice recognition and predictive analysis. The dawn of mobile technology, data availability and the explosion of open-source software provides artificial intelligence huge playing field in the banking sector. The changing dynamics of an app-driven world is enabling the banking sector to leverage AI and integrate it tightly with the business imperatives.
AI in Banking Customer Services
Automated AI-powered customer service is gaining strong traction. Using data gathered from users’ devices, AI-based relay information using machine learning by redirecting users to the source. AI-related features also enable services, offers, and insights in line with the user’s behaviour and requirements. The cognitive machine is trained to advise and communicate by analysing users’ data. Online wealth management services and other services are powered by integrating AI advancements to the app by capturing relevant data.
The tested example of answering simple questions that the users have and redirecting them to the relevant resource has proven successful. Routine and basic operations i.e. opening or closing the account, transfer of funds, can be enabled with the help of chatbots.
Fraud and risk management
Online fraud is an area of massive concern for businesses as they digitise at scale. Risk management at internet scale cannot be managed manually or by using legacy information systems. Most banks are looking to deploy machine or deep learning and predictive analytics to examine all transactions in real-time. Machine learning can play an extremely critical role in the bank’s middle office.
The primary uses include mitigating fraud by scanning transactions for suspicious patterns in real-time, measuring clients for creditworthiness, and enabling risk analysts with right recommendations for curbing risk.
Trading and Securities
Robotic Process Automation (RPA) plays a key role in security settlement through reconciliation and validation of information in the back office with trades enabled in the front office. Artificial intelligence facilitates the overall process of trade enrichment, confirmation and settlement.
Credit Assessment
Lending is a critical business for banks, which directly and indirectly touches almost all parts of the economy. At its core, lending can be seen as a big data problem. This makes it an effective case for machine learning. One of the critical aspects is the validation of creditworthiness of individuals or businesses seeking such loans. The more data available about the borrower, the better you can assess their creditworthiness.
Usually, the amount of a loan is tied to assessments based on the value of the collateral and taking future inflation into consideration. The potential of AI is that it can analyse all of these data sources together to generate a coherent decision. In fact, banks today look at creditworthiness as one of their everyday applications of AI.
Portfolio Management
Banks are increasingly relying on machine learning to make smarter, real-time investment decisions on behalf of their investors and clients.
These algorithms can progress across distinct ways. Data becomes an integral part of their decision-making tree, this enables them to experiment with different strategies on the fly to broaden their focus to consider a more diverse range of assets.
Banks are focussed to leverage an AI and machine learning-based technology platforms that make customised portfolio profiles of customers based on their investment limits, patterns and preferences.
Banking and artificial intelligence are at a vantage position to unleash the next wave of digital disruption. A user-friendly AI ecosystem has the potential for creating value for the banking industry, but the desire to adopt such solutions across all spectrums can become roadblocks. Some of the issues can be long implementation timelines, limitations in the budgeting process, reliance on legacy platforms, and the overall complexity of a bank’s technology environment.
To overcome the above challenges of introducing and building an AI-enabled environment. Banks need to enable incremental adoption methods and technologies. The critical part is ensuring that the transition allows them to overcome the change management/behavioural issues. The secret sauce of successful deployment is to ensure a seamless fit into the existing technology architecture landscape, making an effective AI enterprise environment.
Related Posts
AIQRATIONS
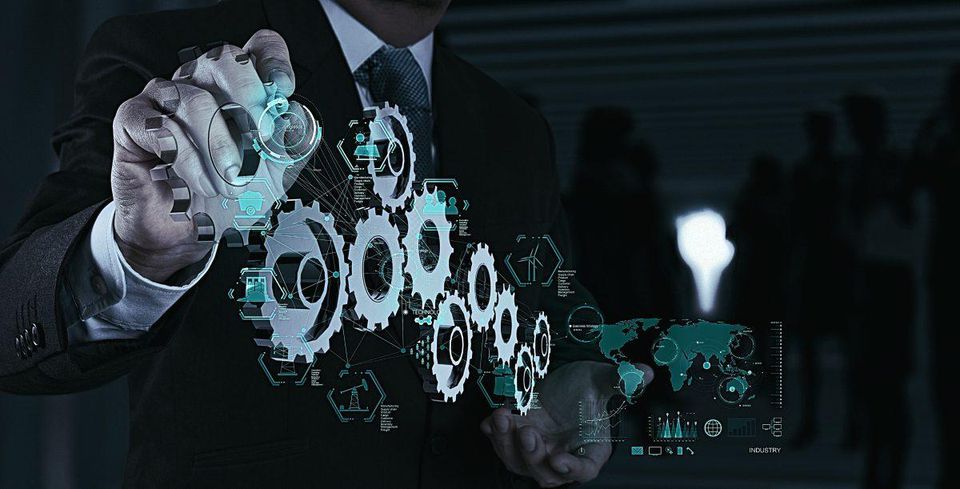
Building AI-enabled organisations
Add Your Heading Text Here
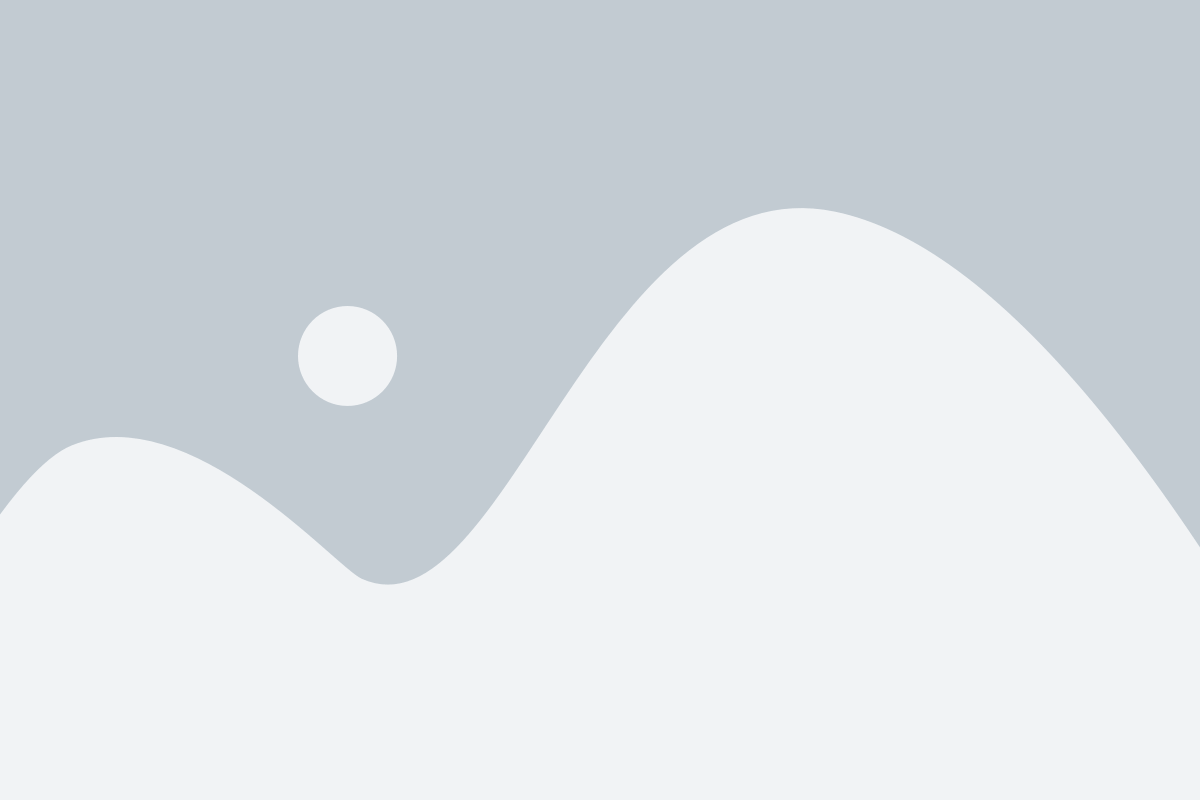
The adoption and benefit realisation from cognitive technologies is gaining increasing momentum. According to a PwC report, 72% of business executives surveyed believe that artificial intelligence (AI) will be a strong business advantage and 67% believe that a combination of human and machine intelligence is a more powerful entity than each one on its own.
Another survey conducted by Deloitte reports that on an average, 83% of respondents who have actively deployed AI in the enterprise see moderate to substantial benefits through AI – a number that goes further up with the number of AI deployments.
These studies make it abundantly clear that AI is occupying a high and increasing mindshare among business executives – who have a strong appreciation of the bottom line impact delivered by cognitive systems, through improved efficiencies.
AI-first Mindset
Having said that, with AI becoming more and more mainstream in an organisational setup, piecemeal implementations will deliver a lower marginal impact to organisations’ competitive advantage. While once early adopters were able to realise transformational benefits through siloed AI deployments, now that it is fast maturing as a must-have in the enterprise and we will need a different approach.
To realise true competitive advantage, organisations need to have an AI-first mindset. It is the new normal in accelerating business decisions. It was once said that every company is a technology company – meaning that all companies were expected to have mature technology backbones to deliver business impact and customer satisfaction. That dictum is now being amended to say – every company is a cognitive company.
To deliver on this promise, companies need to weave AI into the very fabric of their strategy. To realise competitive advantage tomorrow, we need to embed AI across the organisation today, with a strong, stable and scalable foundation. Here are three building blocks that are needed to create that robust foundation.
1. Enrich Data & Algorithm Repositories
If data is indeed the new oil (which it is), organisations that hold the deepest reserves and the most advanced refinery will be the ones that win in this new landscape. Companies having the most meaningful repository of data, along with fit-for-purpose proprietary algorithms will most likely enjoy a sizeable competitive advantage.
So, companies need to improve and re-invent their data generation and collection mechanisms. Data generation will help reduce their reliance on external data providers and help them own the data for conducting meaningful, real-time analysis by continuously enriching the data set.
Alongside, corporations also need to build an ‘algorithm factory’ – to speed up the development of accurate, fit-for-purpose and meaningful algorithms. The algorithm factory would need to push out data models in an iterative process in a way that improves the speed and accuracy.
This would enable the data and analysis capabilities of companies to grow in a scalable manner. While this task would largely fall under the aegis of data science teams, business teams would be required to provide timely interventions and feedback – to validate impact delivered by these models, and suggest course-corrections where necessary.
Another key aspect of this process is to enable a transparent cross-organisation view into these repositories. This will allow employees to collaborate and innovate rapidly by learning what is already been done and will reduce needless time and effort spent in developing something that’s already there.
2. AI Education for Workforce
Operationalising AI requires a convergence of different skill sets. According to the above-cited Deloitte survey, 37% of respondents felt that their managers didn’t understand cognitive technology – which was a hindrance to their AI deployments.
We need to mix different streams of people to build a scalable AI-centric organisation. For instance, business teams need to be continuously trained on the operational aspects of AI, its various types, use cases and benefits – to appreciate how AI can impact their area of business.
Technology teams need to be re-skilled around the development and deployment of AI applications. Data processing and analyst teams need to better understand how to build scalable computational models, which can run more autonomously and improve fast.
Unlike a typical technology transformation, AI transformation is a business reengineering exercise and requires cross-functional teams to collaborate and enrich their understanding of AI and how it impacts their functions, while building a scalable AI programme.
The implicit advantage of developing topical training programmes and involving a larger set of the workforce is to mitigate the FUD that is typically associated with automation initiatives. By giving employees the opportunity to learn and contribute in a meaningful way, we can eliminate bottlenecks, change-aversion and enable a successful AI transformation.
3. Ethical and Security Measures
The 4th Industrial Revolution will require a re-assessment of ethical and security practices around data, algorithms and applications that use the former two.
By introducing renewed standards and ethical codes, enterprises can address two important concerns people typically raise – how much power can/should AI exercise and how can we stay protected in cases of overreach.
We are already witnessing teething trouble – with accidents involving self-driving cars resulting in pedestrian deaths, and the continuing Facebook-Cambridge Analytica saga.
Building a strong grounding for AI systems will go a long way in improving customer and social confidence – that personal data is in safe hands and is protected from abuse – enabling them to provide an informed consent to their data. To that end, we need to continue refining our understanding around the ethical standards of AI implementations
AI and other cyber-physical systems are key components of the next generation of business. According to a report by semiconductor manufacturer, ARM, 61% of respondents believe that AI can make the world a better place. To increase that sentiment even further, and to make AI business-as-usual, and power the cognitive enterprise, it is critical that we subject machine intelligence to the same level of governance, scrutiny and ethical standards that we would apply to any core business process.
Related Posts
AIQRATIONS
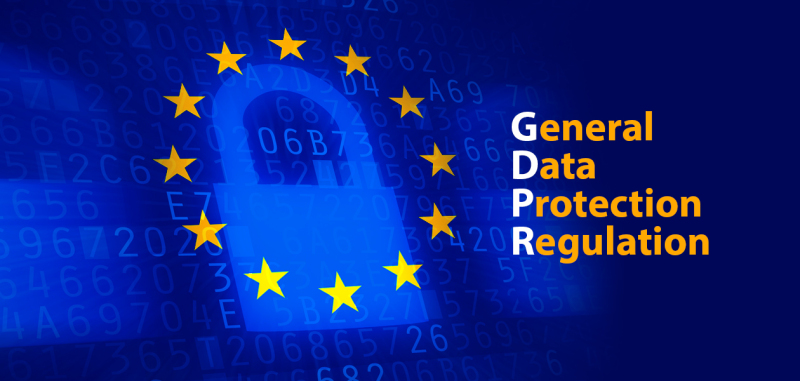
HOW AI CAN ENABLE ENTERPRISES TO IMPLEMENT GENERAL DATA PROTECTION REGULATION (GDPR) POLICIES
Add Your Heading Text Here
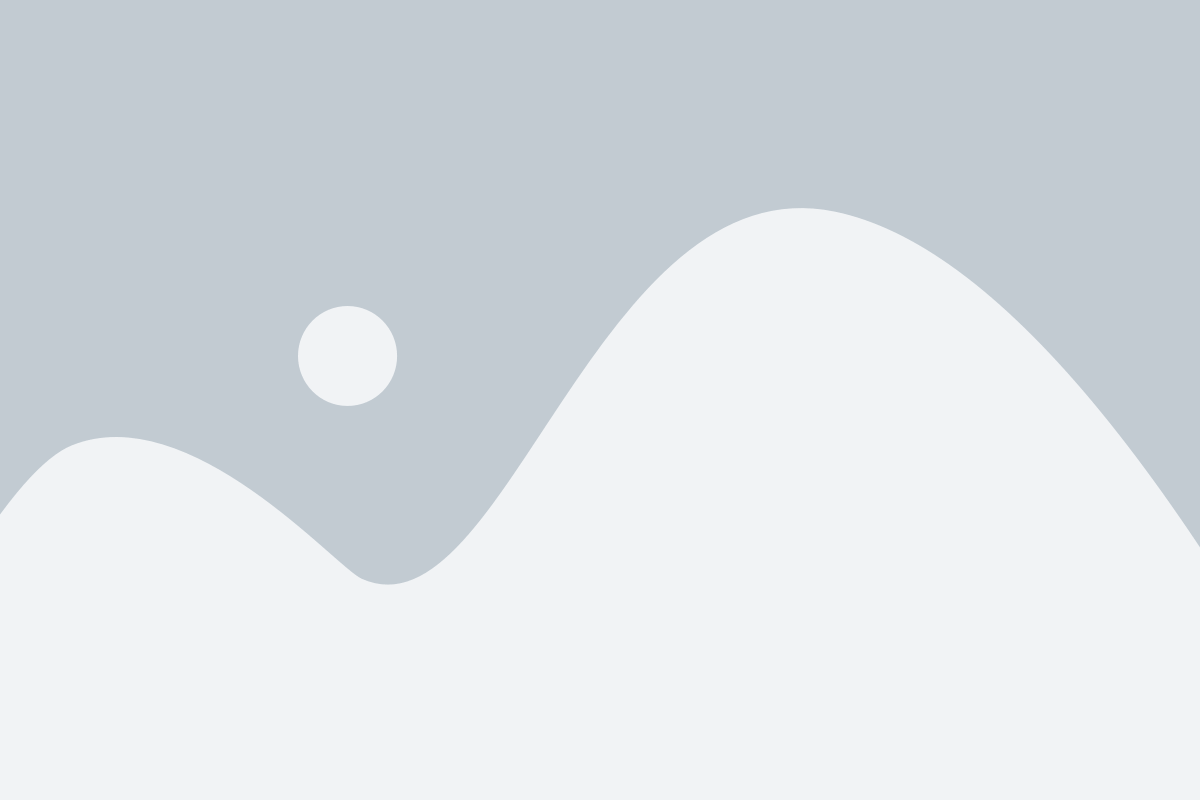
The GDPR General Data Protection Regulation (GDPR), which goes into effect May 25, 2018, requires all companies that collect data on citizens in EU countries to provide a “reasonable” level of protection for personal data. The ramifications for non-compliance are significant, with fines of up to 4% of a firm’s global revenues.
This European Union’s sweeping new data privacy law, is triggering a lot of sleepless nights for CIOs grappling with how to effectively comply with the new regulations and help their organizations avoid potentially hefty penalties.
Will AI be the only answer to the highly regulated GDPR to come?
The bar for GDPR compliance is set high. The regulation broadly interprets what constitutes personal data, covering everything from basic identity information to web data such as IP addresses and cookies, along with more personal artifacts including biometric data, sexual orientation, and even political opinions. The new regulation mandates, among other things, that personal data be erased if deemed unnecessary. Maintaining compliance over such a broad data set is all the more challenging when it is distributed among on-premises data centers, cloud offerings, and business partner systems.
The complexity of the problem has made GDPR a top data protection priority. A PwC survey found that 77% of U.S. organizations plan to spend $1 million or more to meet GDPR requirements. An Ovum report found that two-thirds of U.S. companies believe they will have to modify their global business strategies to accommodate new data privacy laws, and over half are expecting to face fines for non-compliance with the pending GDPR legislation.
This begs the question: Can AI help organizations meet the GDPR’s compliance deadline and avoid penalties? After all, AI is all about handling and deriving insights from vast amounts of data, and GDPR demands that organizations comb through their databases for rafts of personal information that falls under GDPR’s purview. The answer is not only in the affirmative, but there are several significant instances where AI solutions to regulation compliance and governance are already on the high.
For example, Informatica is utilizing advances in artificial intelligence (AI) to help their organizations improve visibility and control over geographically dispersed data. It will provide companies with a holistic, intelligent, and automated approach to governance, for the challenges posed by GDPR.
AI interventions in Data Regulation Compliance and Governance
Data location Discovery and PII Management
It’s essential to learn the location of all customer data in all systems. The first action a company need to do is creating a risk assessment with a guess about what kind of data is likely to be requested how many requests might be expected. Locating all customer data and ensuring GDPR compliant management can be a daunting task, but there are options for automating those processes.
With AI, one can quite easily recognize concepts like ‘person names,’ which is important in this context. To find out how many documents you have that refer to persons (as opposed to companies), or to find out how many documents, social security numbers, phone numbers you have in any one repository, one can combine those analytics, and then begin to understand that the odds are that they have a lot of personal data in this repository, which provides a way to prioritize in the context of GDPR.
For example, M-Files uses Artificial Intelligence to streamline the process of locating and managing PII (personally identifiable information), which often resides in a host of different systems, network folders and other information silos, making it even more challenging for companies to control and protect it.
AI based data cataloguing
A solution that utilizes AI-based machine learning techniques to improve tracking and cataloging data across hybrid deployments can help companies do more accurate reporting while boosting overall efforts to achieve GDPR compliance. By automating the process of discovering and properly recording all types of data and data relationships, organizations can develop a comprehensive view of compliance-related data tucked away in non-traditional sources such as email, social media, and financial transactions – a near-impossible task using traditional solutions and manual processes.
Contextual Engines for Diversely Changing Data Environments
The GDPR changes how companies should look at storage of data. The risk of data getting compromised is increased based on how is stored, in how many different systems it’s stored, how many people are involved in that process, and how long it’s kept. Now that PII on job applications is regulated under GDPR, a company may want to routinely get rid of that data fairly quickly to avoid risk of data breach or audit. There are those kinds of procedural things that organizations will have to really think about.
There are instances where completely removing all data is impossible. You have to retain some data like billing records and there might be conflicting regulations, such as records retention laws. Now, if the citizen asks you to remove that, it’s going to add a lot of complexity to the process, in terms of understanding what data can be removed from the system and what cannot be removed. There will be conflicting situations where this regulation says something, and then you might have an Accounting Act or something in a local or state regulation that says something else.
This requires contextual engines built using AI that can be highly context aware based on the changing circumstances around the data and create a plan of how each data should be stored, managed and purged. This can also provide accurate insights on the levels of encryption and complex data storage techniques that need to be implemented for different data, thereby conserving hardware resources and increasing protection against malignant attacks and data breaches while minimizing risk of GDPR violations.
Working out the Kinks in AI led GDPR
GDPR aims to give EU citizens greater control over their personal data and to hold companies accountable on matters such as data use consent, data anonymization, breach notification, cross-border data transfer, and appointment of data protection officers. For example, organizations will have to honor individuals’ “right to be forgotten,” where applicable — fulfilling requests to delete information and providing proof that it was done. They must also obtain explicit, rather than implied, permission to gather data. And they are required to allow people to see their own data in a commonly readable format.
The system will undoubtedly work those issues out, but, in the meantime, companies should roll up their sleeves and take a thorough, systematic multi-step approach. The multi-step strategy should include:
Data. A comprehensive plan to document and categorize the personal data an organization has, where it came from, and who it is shared with.
Privacy notices. A review of privacy notices to align with new GDPR requirements.
Individuals’ rights. People have enhanced rights, such as the right to be forgotten, and new rights, such as data portability. This demands a check of procedures, processes, and data formats to ensure the new terms can be met.
Legal basis for processing personal data. Companies will need to document the legal basis for processing personal data, in privacy notices and other places.
Consent. Companies should review how they obtain and record consent, as they will be required to document it. Consent must be a positive indication; it cannot be inferred. An audit trail is necessary.
Children. There will be new safeguards for children’s data. Companies will need to establish systems to verify individuals’ ages and gather parental or guardian consent for data-processing activity.
Data breaches. New breach notification rules and new fines will affect many organizations, making it essential to understand how to detect, report, and investigate personal data breaches.
Privacy by design. A privacy by design and data minimization approach will become an express legal requirement. It’s important for organizations to plan how to meet the new terms.
Data protection officers. Organizations may need to designate a data protection officer and figure out who will take responsibility for compliance and how they will position the role.
Will GDPR Aligning Measures Be Necessarily Disruptive?
Many companies are going through significant changes as a result of the new regulations, and the efficiency and speed the AI-powered regulation compliance platform offer can significantly help streamline the entire process if companies want to ensure compliance.
Hence, there are plenty of challenges keeping CIOs up at night. By taking a more intelligence-driven approach to data discovery, preparation, management, and governance, the impending GDPR mandate doesn’t have to be one of them.
Related Posts
AIQRATIONS
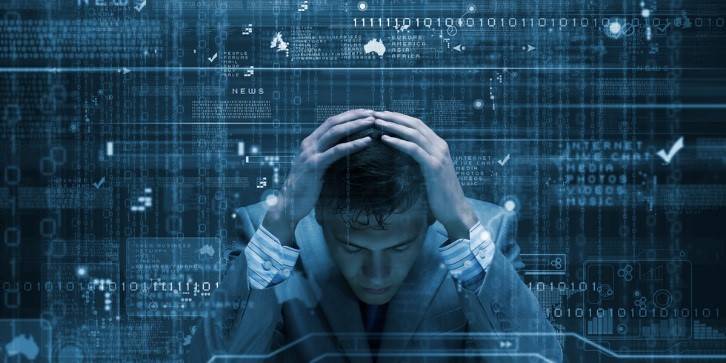
Data Glut to Data Abundance; The Fight for Data Supremacy – Enter the Age of Algorithm Ascendancy
Add Your Heading Text Here
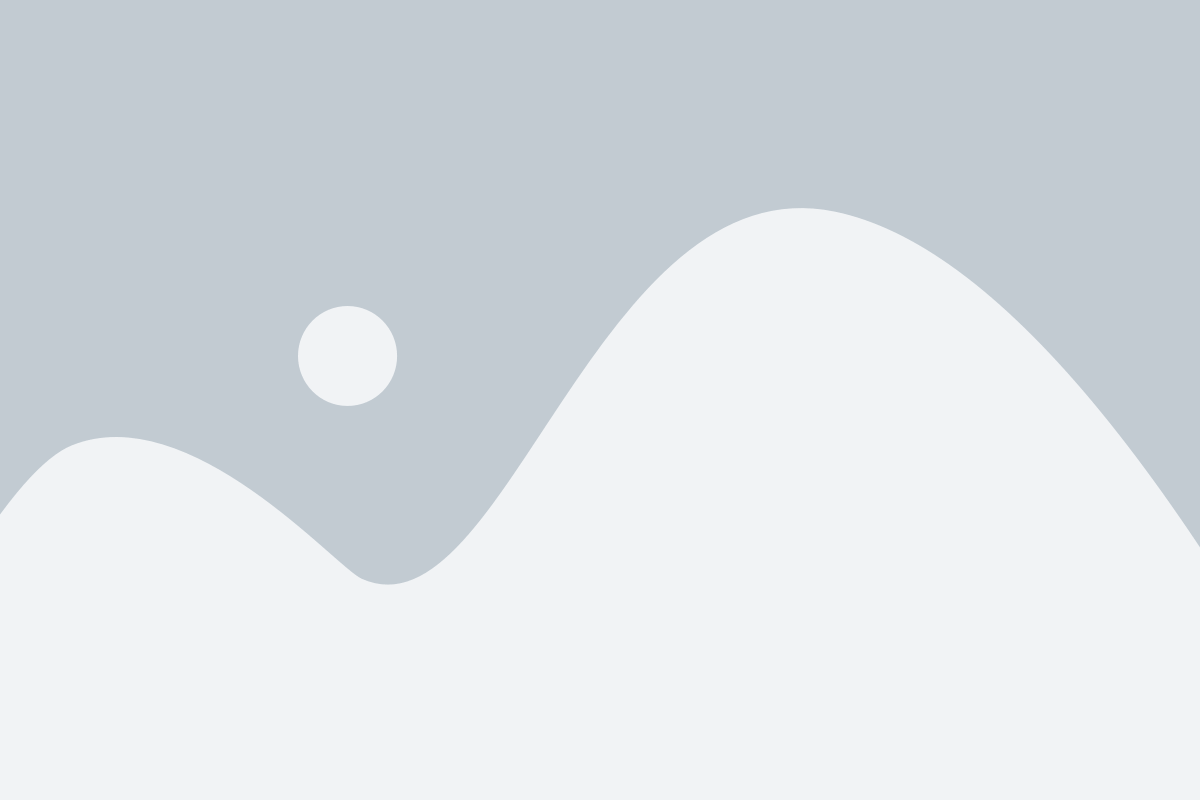
The definition of Data Breaches in current times have evolved from, happening under ‘malicious intent’, to also cover those which have been occurring as a consequences of bad data policies and regulation oversight. This means even policies that have been deemed legally screened might end up, in certain circumstances, in opening doors to some significant breach of data, user privacy and ultimately user trust.
For example, recently, Facebook banned data analytics company Cambridge Analytica from buying ads from its platform. The voter profiling firm allegedly procured 50 million physiological profiles of people through a research application developer Aleksandr Kogan, who broke Facebook’s data policies by sharing data from his personality-prediction app, that mined information from the social network’s users.
Kogan’s app, ‘thisisyourdigitallife’ harvested data not only from the individuals participating in the game, but also from everyone on their friend list. Since Facebook’s terms of services weren’t so clear back in 2014 the app allowed Kogan to share the data with third parties like Cambridge Analytica. This means policy wise it is a grey area whether the breach could be considered ‘unauthorized’, but it is clear that it happened without any express authorization from Facebook. This personal information was subsequently used to target voters and sway public opinion
This is different than the site hackings where credit card information was actually stolen at major retailers, the company in question, Cambridge Analytica, actually had the right to use this data. The problem is they used this information without permission in a way that was overtly deceptive to both Facebook users and Facebook itself.
Fallouts of Data Breaches: Developers left to deal with Tighter Controls
Facebook will become less attractive to app developers if it tightens norms for data usage as a fallout of the prevailing controversy over alleged misuse of personal information mined from its platform, say industry members.
India has the second largest developer base for Facebook, a community that builds apps and games on the platform and engage its users. With 241 million users, the country last July over took the US as the largest userbase for the social network platform.
There will be more scrutiny now. When you do, say, a sign on. The basic data (you can get) is the user’s name and email address, even which will undergo tremendous scrutiny before they approve it. That will have an impact on the timeline. The viral effect) could decrease. Now, without explicit rights from users, you cannot reach out to his/her contacts. Thus, the overhead goes on to the developers because of such data breaches, which shouldn’t have occurred in the first place had the policies surrounding user data were more distinct and clear.
Renewed Focus to Conflicting Data Policies and Human Factors
These kinds of passive breaches that happen because of unclear and conflicting policies instituted by Facebook provides us a very clear example of how active breaches (involving malicious attacks) and passive breaches (involving technically authorized but legally unsavoury data sharing) need to be given equal priority and should both be considered pertinent focus of data protection.
While Facebook CEO Mark Zuckerberg has vowed to make changes to prevent these types of information grabs from happening in the future, many of those tweaks will be presumably made internally. Individuals and companies still need to take their own action to ensure their information remains as protected and secure as possible.
Humans are the weakest link in data protection, and potentially even the leading cause for the majority of incidents in recent years. This debacle demonstrates that cliché to its full extent. Experts believe that any privacy policy needs to take into account all third parties who get access to the data too. While designing a privacy policy one needs to keep the entire ecosystem in mind. For instance, a telecom player or a bank while designing their privacy policy will have to take into account third parties like courier agencies, teleworking agencies, and call centers who have access to all their data and what kind of access they have.
Dealing with Privacy in Analytics: Privacy-Preserving Data Mining Algorithms
The problem of privacy-preserving data mining has become more important in recent years because of the increasing ability to store personal data about users, and the increasing sophistication of data mining algorithms to leverage this information. A number of algorithmic techniques such as randomization and k-anonymity, have been suggested in recent years in order to perform privacy-preserving data mining. Different communities have explored parallel lines of work in regards to privacy preserving data mining:
Privacy-Preserving Data Publishing: These techniques tend to study different transformation methods associated with privacy. These techniques include methods such as randomization, k-anonymity, and l-diversity. Another related issue is how the perturbed data can be used in conjunction with classical data mining methods such as association rule mining.
Changing the results of Data Mining Applications to preserve privacy: In many cases, the results of data mining applications such as association rule or classification rule mining can compromise the privacy of the data. This has spawned a field of privacy in which the results of data mining algorithms such as association rule mining are modified in order to preserve the privacy of the data.
Query Auditing: Such methods are akin to the previous case of modifying the results of data mining algorithms. Here, we are either modifying or restricting the results of queries.
Cryptographic Methods for Distributed Privacy: In many cases, the data may be distributed across multiple sites, and the owners of the data across these different sites may wish to compute a common function. In such cases, a variety of cryptographic protocols may be used in order to communicate among the different sites, so that secure function computation is possible without revealing sensitive information.
Privacy Engineering with AI
Privacy by Design is a policy concept was introduced the Data Commissioner’s Conference in Jerusalem, and over 120 different countries agreed they should contemplate privacy in the build, in the design. That means not just the technical tools you buy and consume, [but] how you operationalize, how you run your business; how you organize around your business and data.
Privacy engineering is using the techniques of the technical, the social, the procedural, the training tools that we have available, and in the most basic sense of engineering to say, “What are the routinized systems? What are the frameworks? What are the techniques that we use to mobilize privacy-enhancing technologies that exist today, and look across the processing lifecycle to build in and solve for privacy challenges?”
It’s not just about individual machines making correlations; it’s about different data feeds streaming in from different networks where you might make a correlation that the individual has not given consent to with personally identifiable information. For AI, it is just sort of the next layer of that. We’ve gone from individual machines, networks, to now we have something that is looking for patterns at an unprecedented capability, that at the end of the day, it still goes back to what is coming from what the individual has given consent to? What is being handed off by those machines? What are those data streams?
Also, there is the question of ‘context’. The simplistic policy of asking users if an application can access different venues of their data is very reductive. This does not, in an measure give an understanding of how that data is going to be leveraged and what other information about the users would the application be able to deduce and mine from the said data? The concept of privacy is extremely sensitive and not only depends on what data but also for what purpose. Have you given consent to having it used for a particular purpose? So, I think AI could play a role in making sense of whether data is processed securely.
The Final Word: Breach of Privacy as Crucial as Breach of Data
It is undeniably so that we are slowly giving equal, if not more importance to breach of privacy as compared to breach of data, which will eventually target even the policies which though legally acceptable or passively mandated but resulted in compromise of privacy and loss of trust. Because there is no point claiming one is legally safe in their policy perusal if the end result leads to the users being at the receiving end.
This would require a comprehensive analysis of data streams, not only internal to an application ecosystem, like Facebook, but also the extended ecosystem involving all the players it is channeling the data sharing to, albeit in a policy-protected manner. This will require AI enabled contextual decision making to come to terms as what policies could be considered as eventually breaching the privacy in certain circumstances.
Longer-term, though, you’ve got to write that ombudsman. We need to be able to engineer an AI to serve as an ombudsman for the AI itself.