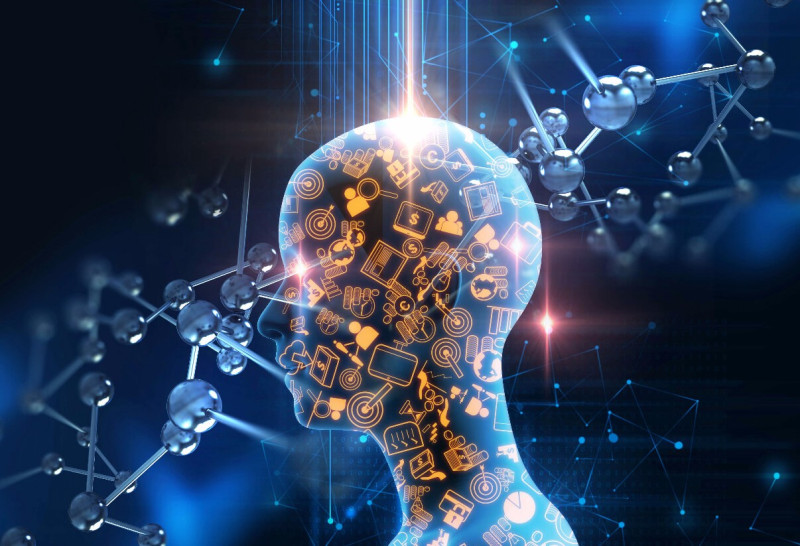
Understanding Burgeoning AI Landscape; A Perspective for PE/VC & Investment Funds
Add Your Heading Text Here
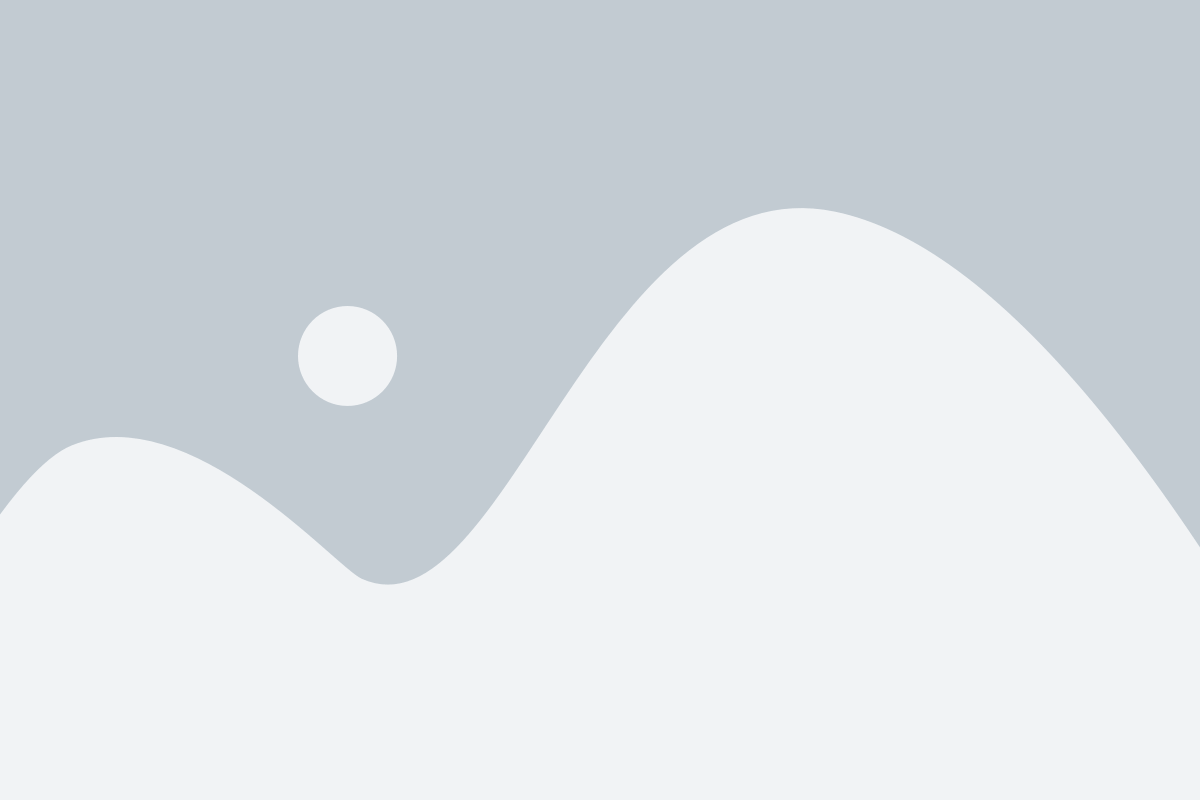
There seems to be a glaring ambiguity as to exactly what artificial intelligence (AI) is, and how the discipline of AI should be categorized. Is AI a form of analytics or is it a totally new discipline that is distinct from analytics? I firmly believe that AI is more closely related to predictive analytics and data sciences than to any other discipline. One might even argue that AI is the next generation of predictive analytics and is born out of sophistication of analytics . Additionally, AI is often utilized in situations where it is necessary to operationalize the analytics process. So, in that sense, AI is also often pushing the envelope of prescriptive, operationalized analytics. It would be a mistake to say that AI is not a form of analytics.
I’ve seen AI applied to some of the most obscure topics you can imagine, ranging from industrial energy usage all the way to finding the right GIFs. Using Artificial Intelligence to improve and create solutions to today’s pressing business and social problems is one of the defining trends of the tech world for me.
So, if you are a PE / VC entity and are looking for investment opportunity in AI space, you will have to understand what kinds of AI companies exist and how this AI practice has evolved from Analytics practice.
There are three types of AI companies — core, applied, and industry
1. Core AI Companies
Core AI companies develop technology that improves parts of the AI creation or deployment process itself. Here are a few selected parts of that process and a few companies that are innovating in each:
Data scrubbing and cleaning: Trifacta, Paxata, Wealthport, Datalogue
Modeling: Sentient, Petuum, MLJar
Deployment: Yhat, Seldon
These companies all innovate in some specific, industry-agnostic part of the AI pipeline. Some of them are specific tools, while others purport to have an entirely new approach to AI that will revolutionize how it’s done (see Geometric Intelligence circa 2015).
If you’re investing in Core AI companies, you should probably have a good understanding of how this pipeline works. If you’re founding one of these companies, you should probably have experience deploying Machine Learning and AI at scale.
2. Applied AI Companies
A bit on the more specific side, Applied AI start-ups develop technology that helps companies across different industries perform a specific task using AI. As with the above, here are some examples of those applications and a few interesting companies in each:
Analysing and understanding text: Indico, Synapsify, Lexalytics
Analysing and understanding images and videos: Clarifai, Kairos, Imagry, Affectiva, Deepomatic
Bots / Voice: Init.ai, MindMeld
While investors can get away with not having experience in one of these specific applications, founders will likely have done projects involving this stuff in the past.
The implementation of AI in this scenario corresponds to the implementation of predictive analytics. At its core, predictive analytics is, naturally, about predicting something. Who will buy? Will certain equipment break? Which price will maximize profits? Each of these questions can be addressed by following a familiar workflow – First, we identify a metric or state that we want to predict and gather historical information on that metric or state. Next, we gather additional data that we believe could be relevant to predicting our target. Then, we pass the data through one or more algorithms that attempt to find a relationship between the target and the additional data. Through this process, a model is created that produces a prediction if new data is fed to it. If a customer had this profile, how likely would she be to respond? If we priced at this point, how much profit might we expect?
The goals and steps followed within an AI process are the same. Let’s look at two examples:
Take image recognition. First, we identify a bunch of cat pictures. Then, we grab a bunch of non-cat pictures. We pass a deep learning algorithm over the images to learn to accurately predict whether an image is a cat. When provided with a new image, the model will answer with the probability that the image is a cat. Sounds a lot like predictive analytics, doesn’t it?
Let’s now consider natural language processing (NLP). We gather a wide range of statements that have specific meanings we care about. We also gather a wide range of other statements. We run NLP procedures against the data to try to tease out how to tell what is important and how to tell what is being asked. As we feed a new line of text to the process, it will identify what the point of the statement is in probabilistic terms. The NLP process will assign probabilities to various possible interpretations and send those back (think Watson playing jeopardy). This also sounds a lot like predictive analytics.
3. Industry AI Companies
The final category of ML/AI companies apply these techniques to specific business problems in specific verticals. This is undoubtedly the lion’s share of the actual number of companies being founded, and in many ways, represents the true promise of AI — solving actual and immediate problems with new techniques. Here, it’s easier to give companies as examples. The format is always “AI for ________”:
DigitalGenius: AI for customer support
Cylance: AI for cyber threat prevention
X.ai: AI for scheduling meetings
Drive.ai: AI for autonomous vehicles
The implementation of AI in this scenario corresponds to industrialized embedded analytics. A major trend today is to embed analytics into business applications so that the models are utilized in an automated, embedded, prescriptive fashion at the point of a business decision. For example, as a person navigates a web page, models are utilized to predict what offers should appear on the next page. There is no human intervention once the process is in place. The process makes offers until told to stop.
Many applications of AI today also require industrialization. For example, as an image is posted on social media, it is immediately analysed to identify who is present in the image. As I make a statement to Siri or Alexa, it attempts to determine what I said and what the best answer is. While this qualifies as a more advanced application of predictive analytics that moves into embedded, prescriptive, automated processes, it is still very much in line with how industrialized embedded analytics are being used today.
The common theme among these companies is that they take Machine Learning / AI and use it on a specific problem or space. When researching investments like this, investors should look at both the AI itself (if it works well) and the business case (whether it’s compelling). In x.ai’s case, investors need to know if the AI works, but they should also consider whether AI is the best way to solve the scheduling problem, and whether scheduling is a problem worth solving at all. With the other two types of companies, this is rarely a consideration. Founders of these types of companies can often not have AI experience and can even be non-technical (with the right supporting team and CTO, of course).
Final Word
Your journey to a fruitful AI investment will be far easier if you recognize and embrace AI as sophistication of analytics and understand the true categorization, and then task your analysts with leading the charge. Don’t cause confusion and redundancy by considering AI to be something completely different.
Related Posts
AIQRATIONS
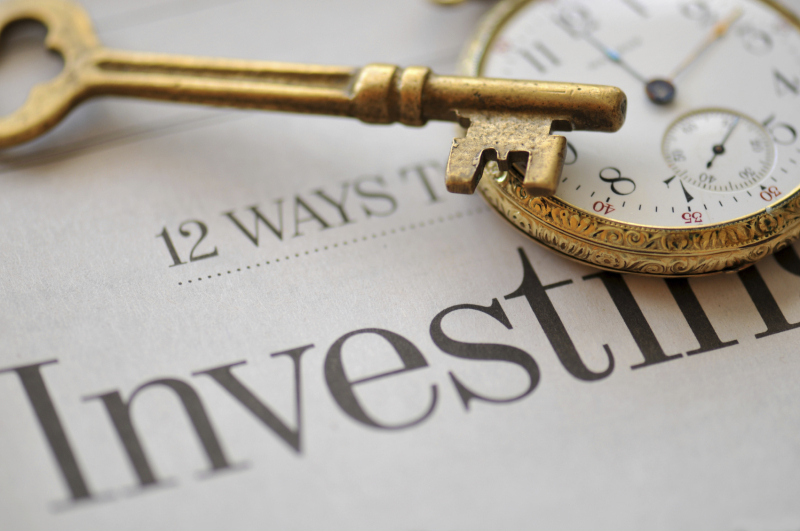
Investment Analytics for VC & PE Firms
Add Your Heading Text Here
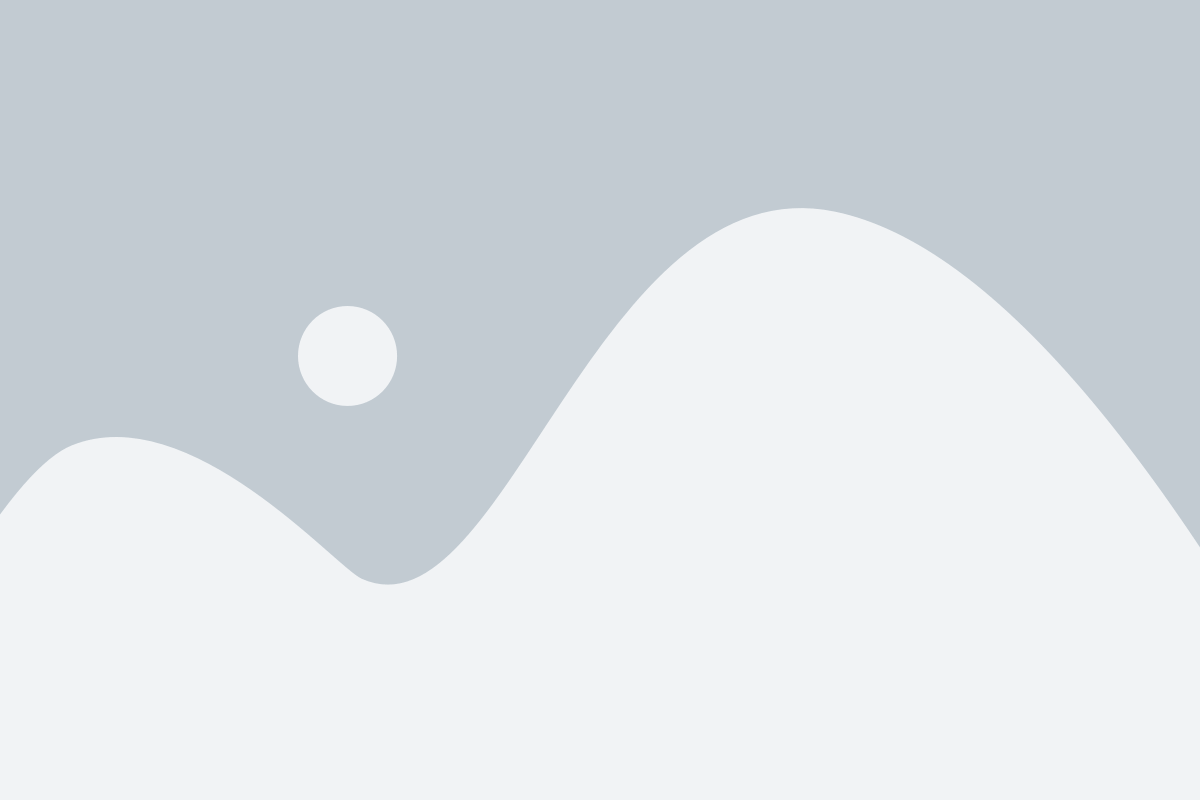
In the venture capital world, it’s all about the “hits.” A hit is a startup that makes it big, returning many multiples of a venture fund’s initial investment. Hits are great for everyone—investors, entrepreneurs, job seekers—but the problem is they don’t happen very often. The odds of a big hit are about one in 10.
Boosting the odds for VCs
But what if venture capital could boost its odds to 50-50, or even two out of three? With $48 billion in VC investment in 2014, such an improvement would prevent huge amounts of money from being lost on startups that never had much of a chance of surviving the harsh competitive environment. The challenge is to identify those likely laggards well before the market rejects their idea and, perhaps more importantly, to see the big hits before anyone else. Venture capital has long relied on subjective, intuitive methods of assessing startups, but that’s changing as more firms are bringing data science and consistency into their decision-making.
The next is to try to do the science, build the tools, and do the research all around this one question: How can we better predict when innovations will survive or fail, both for startups and when corporations launch new products or do acquisitions? There’s no human subjectivity involved anywhere along the line. All the algorithms converge on a discrete yes or no. That yes or no depends on majorly two areas – those inside the startup, and those external to the startup. Only around 20% of the predictive value to come from details specific to the startup itself whereas 80% comes from things outside of the startup, such as the market, customers, competitors, technology trends, and timing.
In this scenario, managers are trying to predict the future before investing money in it. According to analysis of data from the Small Business Administration, and data on startups, between 20% and 30% of new businesses survive to their 10th birthday. Startups with VC-backing, aren’t doing much better than spin-offs from large corporations. Has the entrepreneurship economy gotten any better at predicting any business? Data suggest no, not in any statistically significant amount. But Investment Analytics is turning that around. When it comes to predicting survivorship of companies after a 10-year period, implementing investment analytics at present setup can point you to the right answer 67% of the time, and totally wrong on the remaining third. So, If investment analytics has vastly improved the odds of investing in new technologies and businesses, why isn’t the entire VC world knocking on its door? Because A lot of venture capitalists, like the scouts in Moneyball reacting to sabermetrics, are skeptical. Most of the people don’t see quants taking over VC, even in the distant future, but they do see the potential of using data to help venture capitalists make decisions.
Data analytics are undoubtedly creeping into venture capital—Google Ventures uses an algorithm to help with investment decisions, and a Silicon Valley firm called Correlation Ventures is built upon an algorithmic investing strategy. But the old-fashioned process of detailed research and human judgment still has a lot going for it. Just ask the people at Lux Research, an emerging-technology consulting firm in Boston. For the past 10 years, Lux’s science-trained analysts have been scouring the business landscape for new technology firms, interviewing employees of those firms, and slowly compiling their own database of companies that succeeded or failed. Lux rates each company it profiles according to nine key factors, which are available to the public on its website in a report called “Measuring and Quantifying Success in Innovation. The result of that rating is a company profile with a “Lux Take,” which ranges from “strong positive” to “strong caution.”
The company recently looked back at five years’ worth of profiles and found that 50% of the companies that earned a “positive” rating went on to be successful, an outcome which Lux defines as an IPO, acquisition, or transition to standalone profitability. Given the usual odds of new business survival, the Lux system seems to inject a significant amount certainty into the process of evaluating startups. The company’s high rate of accuracy is attributable to two things: capability and methodology.
People talk a lot about the importance of innovation to economic growth. In a recent survey of voters in swing states by the Economic Innovation Group, 75% of those surveyed agreed that America needs more entrepreneurs and investors in order to improve long-standing economic problems. The innovation economy has an information problem. The information that drives it isn’t good. How can countries become innovation economies in a more efficient way? Let’s get better at funding the startup companies that will grow and drive employment. For every dollar that goes in the wrong place, that’s a bad dollar.
Better Investment Strategy for PEs
Data, and specifically the analysis of data is becoming a critical component in enabling private equity firms to create and maintain value for their investors. I know some of you are thinking, “What does a data management geek know about private equity?” One thing to note about private equity firms is that there has been a greater focus on IT and a growing need for data analytics services recently, especially for deals that involve middle-market companies and/or are add-ons to existing platforms. Increasing acquisition valuations and the dearth of available debt financing are making it increasingly difficult for private equity firms to generate outsized returns for their investors. This is causing private equity firms to focus more on operational improvements within their portfolio companies as a means of driving growth and value creation. Given the strong correlation between operational improvement and higher returns for limited partners, private equity managers’ hands-on involvement is, and will continue to be, critical to the future success of the fund. For private equity managers, this means identifying key drivers of EBITDA, understanding customer behavior, developing competitor analyses, determining the best place to invest capital, managing budgets, and controlling costs—for each of their portfolio companies. And to do it effectively and efficiently, managers must leverage data.
Nobody said it was going to be easy and the fact is: it’s not. Unfortunately, most of the private equity managers are not in a position to dig deep into the recesses of their portfolio company systems and extract the data tables necessary to produce key operational metrics. Therefore, managers will have to rely on the current workforce at each of their portfolio companies or hire an outside party to do the ‘dive into the data’ for them. Many of them struggle with capturing, extracting, and ultimately analyzing operational data within their businesses. This is largely due to the fact that, historically, middle-market companies have rarely made technology a priority, leaving them with an unsophisticated IT platform. As a result, when dealing with legacy and perhaps “home-grown” solutions, there are a variety of challenges that arise when attempting to harmonize, integrate, and perform data analytics. Additionally, many middle-market companies are experiencing data volumes that are growing exponentially and are held in disparate systems throughout the organization. In short, private equity managers are faced with massive amounts of “dirty” data that must be converted into meaningful metrics. This leaves private equity firm managers asking themselves, “How much time and money do I need to commit to information technology and data analytics?” Answer: more than you have in the past. The key is to invest wisely and in a way that will get you the greatest returns in the shortest amount of time. There are an infinite number of technology solutions out there. From off-the-shelf, in-the-box solutions to massive enterprise resource planning (ERP) solutions, software companies have done their best to provide an answer to your “big data” problems. Although there are many great tools out there, there is no cookie-cutter solution when trying to capitalize on the presumed synergies of integration. Private equity managers must understand the specific business needs and work to develop strategic analytical solutions driven from the data that already exists in their organization. It is critical, especially in today’s competitive market, to “leverage your data” and apply these targeting solutions in a way that will provide the greatest returns for your investors.
Big data may indeed be able to help, but it’s more likely to be a piece of the puzzle, not the solution. For instance, academic studies have shown that serial entrepreneurs successful in the past are more likely to do well in new ventures. That implies there is some explanatory power in looking backwards for guidance on what’s ahead. “But the nature of entrepreneurship is always changing,” says Josh Lerner, the Jacob H. Schiff Professor of Investment Banking at Harvard Business School. Most regressions predicting entrepreneurial success in the literature have very low goodness of fit, which suggests the limits of a ‘Moneyball’ approach here. Predicting which startup is going to be successful is much harder than [predicting] which baseball player is. It is as if the baseball rules are being changed every year in unpredictable ways.”