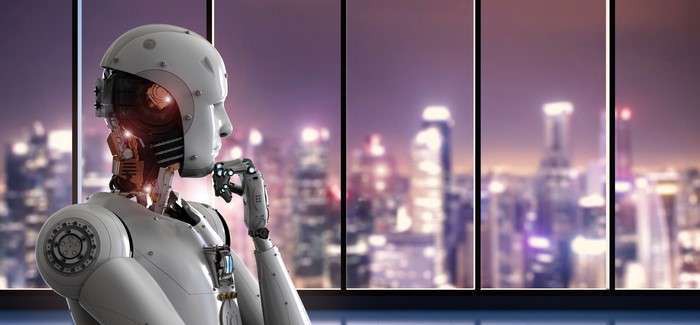
Better business with AI
Add Your Heading Text Here
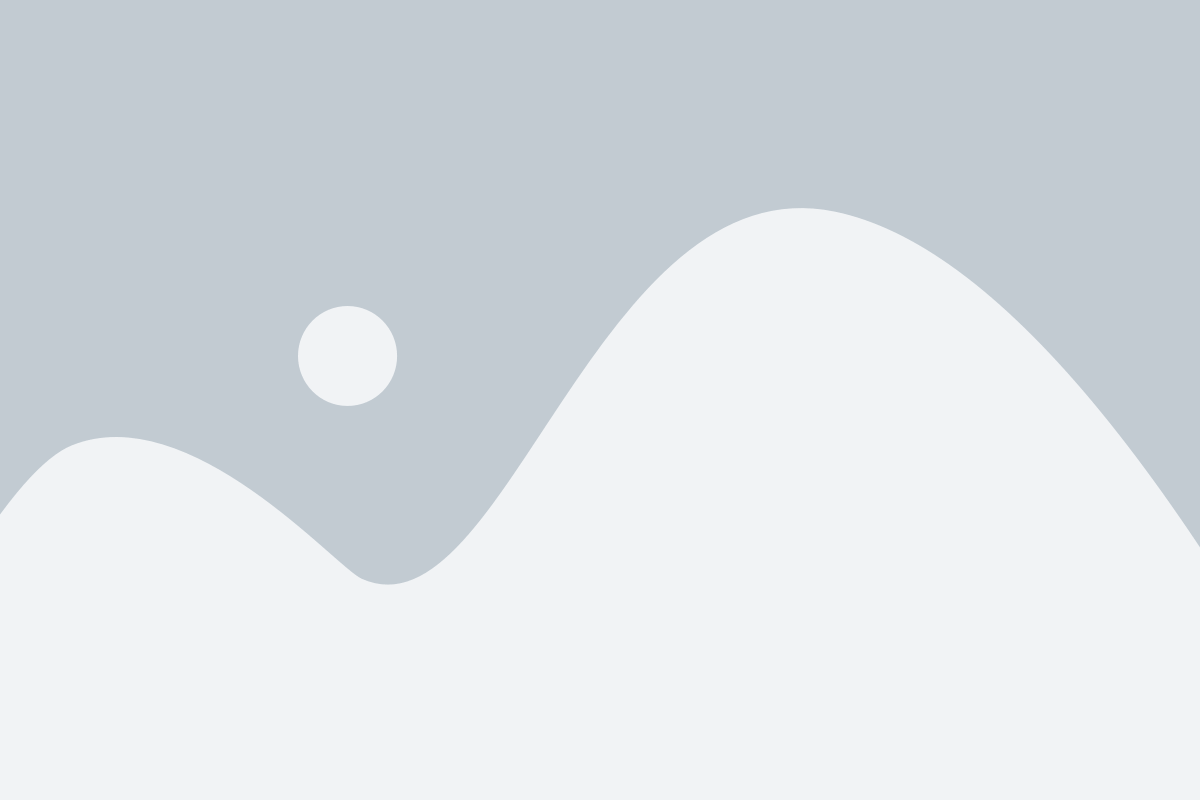
The Artificial Intelligence revolution in the enterprise is well under way. According to Gartner’s 2018 CEO and Senior Business Executive Survey, 65% of respondents think that AI will have a ‘material impact on an area of their business’. Due to the combination of three critical factors – improved data availability and machine learning techniques, increased computing power and storage, and a strong enterprise thrust on data-driven decision-making – AI has taken a strong foothold in some of the largest corporations in the world today, commanding executive-level interest, attention and urgency.
Beyond simple automation, AI is powering complex, critical decisions in several areas from Renaissance Technologies’ Medallion Fund, which uses statistical probabilities and quantitative models and has become one of the startling successes in the hedge fund industry, to complex image annotation and deep learning that helps radiologists detect cancer in MRI scans. Here is a look at some of the critical areas where AI is augmenting human decision-making:
Healthy Healthcare
As multiple countries grapple problems from an ageing population, rising healthcare costs and low doctor-to-patient ratios, AI can help improve healthcare outcomes in a variety of ways. For instance, AI is being leveraged for public health studies – from detection of potential physical or psychological pandemics to epidemiology – by mining social media and other data sources.
Further, startups and conglomerates are working on AI for diagnostics – from detection of early warning signals to identifying and quantifying abnormalities/tumours. In the pharma industry, AI is helping improve site studies, drug development and clinical trials through analysis of meaningful data.
Financial Services
A common AI use case for financial services is in the domain of fraud detection and anti-money laundering. AI can help surface bad actors by quickly scanning data for anomalous behaviour. Similarly, AI is also powering customer interaction decisions through intelligent chatbots that can address common concerns, thus reducing the need for human intervention in repetitive, menial tasks. We’re also seeing increased proliferation of robo-advisers – which are advanced AI tools that help make investment decisions by matching investible capital and returns expected.
Managing Media
The media and entertainment industry is going through an AI and digital disruption due to the combination of huge datasets and success of torchbearers like Netflix and Spotify. Content recommendation and personalisation are decisions that are autonomously delivered by AI, which can quickly scan a user’s history and match it with the preferences of similar users.
The industry is also relying on AI to make decisions around content creation, again taking a leaf out of Netflix to make content more engaging and sticky. There is also a strong use case of AI helping identify and attract customers by surfacing tailored content and promotions to increase subscriptions, loyalty and share-of-wallet.
Retail Rejig
Retail was one of the first industries to witness the rise of a data-powered competitor that eventually decimated incumbents. The brick-and-mortar retail industry is now incorporating AI in its decision-making process to replicate the customer experience expectations set by Amazon and the like.
Retailers leverage user purchase to identify next-best product and create tailored loyalty programmes. It is also being increasingly used for rapid experimentation to define store location, layouts and product-shelf decisions. Retailers can better anticipate demand, leading to leaner supply chains and warehouses, optimised inventory and fewer stockouts.
Efficient Manufacturing
Manufacturing companies are bringing in AI interventions to run leaner supply chains to cut the cost of transportation and wastage. AI also enables them to better anticipate demand by looking at historical sales, current uptake and other business environment factors to run on-demand production.
Some AI-led decisions are pervasive across multiple industries. For instance, digital personalisation, ie, serving targeted promotions to customers based on their key purchase drivers, is a multi-industry example of AI in action.
The other is for detecting security threats through anomaly detection and video analytics to identify unauthorised entry. Human Resources is another function that is rapidly changing, with companies using AI to speed up talent acquisition by scanning resumes for relevancy and reducing attrition by identifying key drivers that lead to employees leaving.
Successful AI-led Decisions
The business value of AI is significantly lowered when performed ad hoc, without a strong foundational strategy. It is important that the organisation clearly defines the decisions that should be powered by AI to maintain a high standard of outcomes. The responses will differ from company to company and from industry to industry, but it is important that corporations establish transparent standards for fair use.
We see enough examples of hastily implemented AI, leading to calamitous consequences and companies can no longer hide by saying, ‘The AI made me do it’. To demarcate the clear go and no-go zones for AI, here’s a handy questionnaire to ask yourself:
– Do we have enough superior quality data now and in the future for AI to make the best decision?
– Do we need to bring in insights from multiple sources to contribute to the decision-making process at a speed and scale, which cannot be efficiently handled by human cognition?
– Is human decision-fatigue or bias currently creating a sub-optimal outcome in this area?
– Could there be ethical or moral implications to an AI-led decision that might lead to disastrous consequences?
We also need to address the confidence issues. For instance, a lot of executives look down upon some of the black-box processes performed by AI algorithms. We need to find a way to address these issues by creating a transparent trail of AI decisions and the reasons why AI took a decision. Even in unsupervised learning scenarios, a trail of decisions will not only boost confidence but will also help build better AI and better businesses.
Re-imagined AI-powered decisions will become de rigueur only by the quality of the outcomes they deliver. According to Dr John Kelly, SVP — IBM Research and Solutions portfolio, “The success of cognitive computing will not be measured by Turing tests or a computer’s ability to mimic humans. It will be measured in more practical ways, like return on investment, new market opportunities, diseases cured and lives saved.” This is a crucial way to look at and measure the impact of AI on our businesses, society and lives.
Related Posts
AIQRATIONS

The New Age Enterprise – Enabled by AI
Add Your Heading Text Here
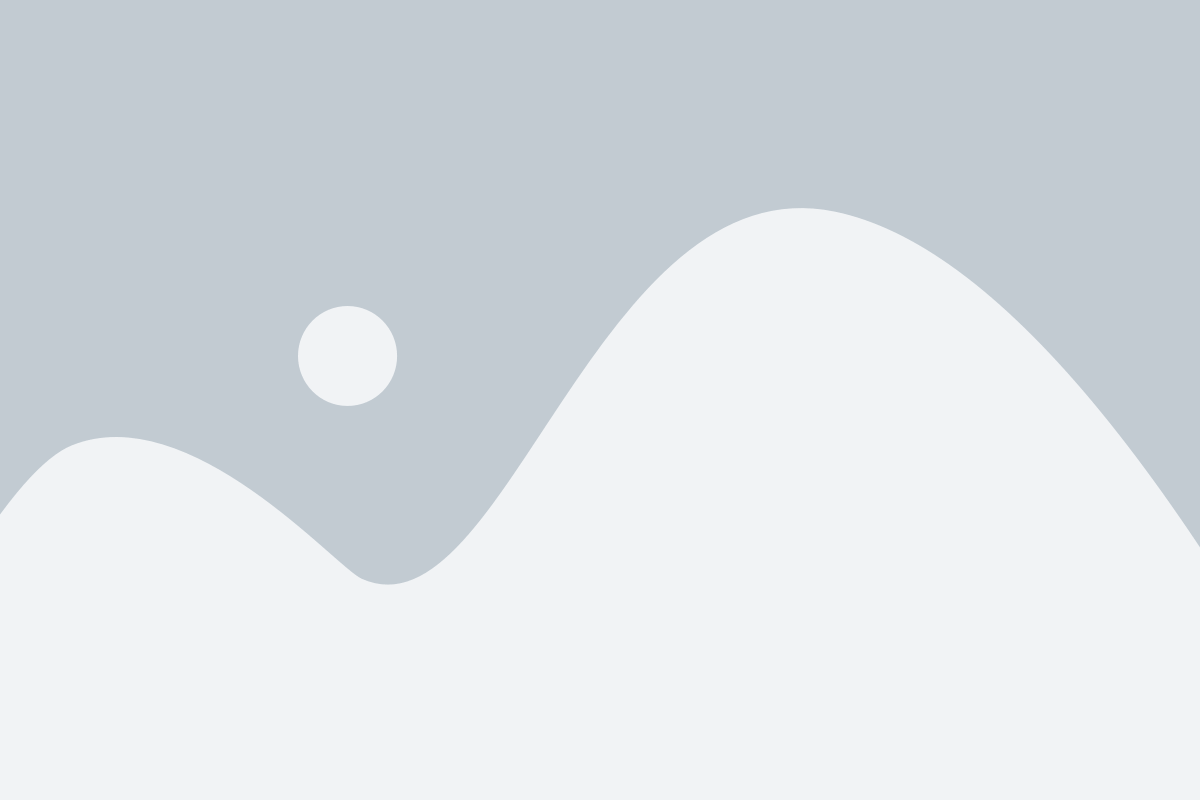
The excitement around artificial intelligence is palpable. It seems that not a day goes by without one of the giants in the industry coming out with a breakthrough application of this technology, or a new nuance is added to the overall body of knowledge. Horizontal and industry-specific use cases of AI abound and there is always something exciting around the corner every single day.
However, with the keen interest from global leaders of multinational corporations, the conversation is shifting towards having a strategic agenda for AI in the enterprise. Business heads are less interested in topical experiments and minuscule productivity gains made in the short term. They are more keen to understand the impact of AI in their areas of work from a long-term standpoint. Perhaps the most important question that they want to see answered is – what will my new AI-enabled enterprise look like?
The question is as strategic as it is pertinent. For business leaders, the most important issues are – improving shareholder returns and ensuring a productive workforce – as part of running a sustainable, future-ready business. Artificial intelligence may be the breakout technology of our time, but business leaders are more occupied with trying to understand just how this technology can usher in a new era of their business, how it is expected to upend existing business value chains, unlock new revenue streams, and deliver improved efficiencies in cost outlays. In this article, let us try to answer these questions.
AI is Disrupting Existing Value Chains
Ever since Michael Porter first expounded on the concept in his best-selling book, Competitive Advantage: Creating and Sustaining Superior Performance, the concept of the value chain has gained great currency in the minds of business leaders globally. The idea behind the value chain was to map out the interlinkages between the primary activities that work together to conceptualize and bring a product / service to market (R&D, manufacturing, supply chain, marketing, etc.), as well as the role played by support activities performed by other internal functions (finance, HR, IT etc.). Strategy leaders globally leverage the concept of value chains to improve business planning, identify new possibilities for improving business efficiency and exploit potential areas for new growth.
Now with AI entering the fray, we might see new vistas in the existing value chains of multinational corporations. For instance:
- Manufacturing is becoming heavily augmented by artificial intelligence and robotics. We are seeing these technologies getting a stronger foothold across processes requiring increasing sophistication. Business leaders need to now seriously consider workforce planning for a labor force that consists both human and artificial workers at their manufacturing units. Due attention should also be paid in ensuring that both coexist in a symbiotic and complementary manner.
- Logistics and Delivery are two other areas where we are seeing a steady growth in the use of artificial intelligence. Demand planning and fulfilment through AI has already reached a high level of sophistication at most retailers. Now Amazon – which handles some of the largest and most complex logistics networks in the world – is in advanced stages of bringing in unmanned aerial vehicles (drones) for deliveries through their Amazon Prime Air program. Business leaders expect outcomes to range from increased customer satisfaction (through faster deliveries) and reduction in costs for the delivery process.
- Marketing and Sales are constantly on the forefront for some of the most exciting inventions in AI. One of the most recent and evolved applications of AI is Reactful. A tool developed for eCommerce properties, Reactful helps drive better customer conversions by analyzing the clickstream and digital footprints of people who are on web properties and persuades them into making a purchase. Business leaders need to explore new ideas such as this that can help drive meaningful engagement and top line growth through these new AI-powered tools.
AI is Enabling New Revenue Streams
The second way business leaders are thinking strategically around AI is for its potential to unlock new sources of revenue. Earlier, functions such as internal IT were seen as a cost center. In today’s world, due to the cost and competitive pressure, areas of the business which were traditionally considered to be cost centers are require to reinvent themselves into revenue and profit centers. The expectation from AI is no different. There is a need to justify the investments made in this technology – and find a way for it to unlock new streams of revenue in traditional organizations. Here are two key ways in which business leaders can monetize AI:
- Indirect Monetization is one of the forms of leveraging AI to unlock new revenue streams. It involves embedding AI into traditional business processes with a focus on driving increased revenue. We hear of multiple companies from Amazon to Google that use AI-powered recommendation engines to drive incremental revenue through intelligent recommendations and smarter bundling. The action item for business leaders is to engage stakeholders across the enterprise to identify areas where AI can be deeply ingrained within tech properties to drive incremental revenue.
- Direct Monetization involves directly adding AI as a feature to existing offerings. Examples abound in this area – from Salesforce bringing in Einstein into their platform as an AI-centric service to cloud infrastructure providers such as Amazon and Microsoft adding AI capabilities into their cloud offerings. Business leaders should brainstorm about how AI augments their core value proposition and how it can be added into their existing product stack.
AI is Bringing Improved Efficiencies
The third critical intervention for a new AI-enabled enterprise is bringing to the fore a more cost-effective business. Numerous topical and early-stage experiments with AI have brought interesting success for reducing the total cost of doing business. Now is the time to create a strategic roadmap for these efficiency-led interventions and quantitatively measure their impact to business. Some food for thought for business leaders include:
- Supply Chain Optimization is an area that is ripe for AI-led disruption. With increasing varieties of products and categories and new virtual retailers arriving on the scene, there is a need for companies to reduce their outlay on the network that procures and delivers goods to consumers. One example of AI augmenting the supply chain function comes from Evertracker – a Hamburg-based startup. By leveraging IOT sensors and AI, they help their customers identify weaknesses such as delays and possible shortages early, basing their analysis on internal and external data. Business leaders should scout for solutions such as these that rely on data to identify possible tweaks in the supply chain network that can unlock savings for their enterprises.
- Human Resources is another area where AI-centric solutions can be extremely valuable to drive down the turnaround time for talent acquisition. One such solution is developed by Recualizer – which reduces the need for HR staff to scan through each job application individually. With this tool, talent acquisition teams need to first determine the framework conditions for a job on offer, while leaving the creation of assessment tasks to the artificial intelligence system. The system then communicates the evaluation results and recommends the most suitable candidates for further interview rounds. Business leaders should identify such game-changing solutions that can make their recruitment much more streamlined – especially if they receive a high number of applications.
- The Customer Experience arena also throws up very exciting AI use cases. We have now gone well beyond just bots answering frequently asked questions. Today, AI-enabled systems can also provide personalized guidance to customers that can help organizations level-up on their customer experience, while maintaining a lower cost of delivering that experience. Booking.com is a case in point. Their chatbot helps customers identify interesting activities and events that they can avail of at their travel destinations. Business leaders should explore such applications that provide the double advantage of improving customer experience, while maintaining strong bottom-line performance.
The possibilities for the new AI-enabled enterprises are as exciting as they are varied. The ideas shared in this article are by no means exhaustive, but hopefully seed in interesting ideas for powering improved business performance. Strategy leaders and business heads need to consider how their AI-led businesses can help disrupt their existing value chains for the better, and unlock new ideas for improving bottom-line and top-line performance. This will usher in a new era of the enterprise, enabled by AI.
Related Posts
AIQRATIONS
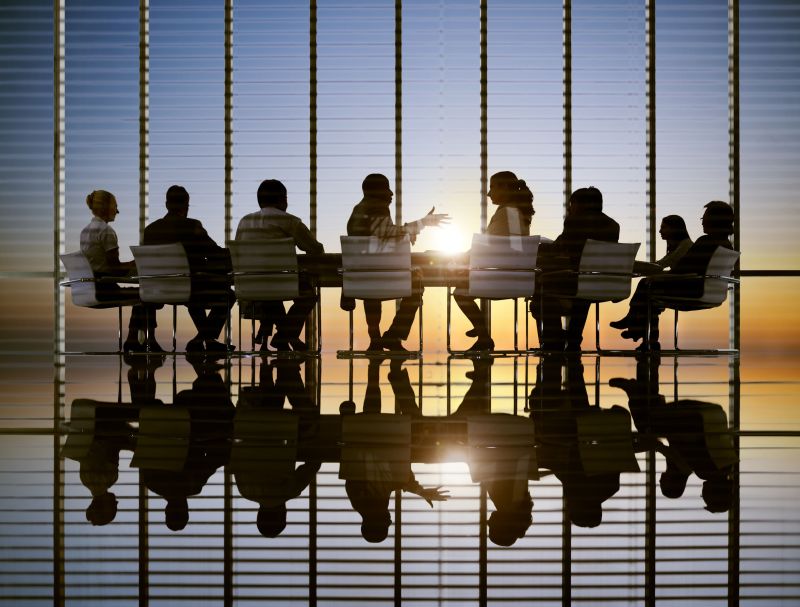
How CXOs are Leveraging AI to Pivot Business Strategy and Operational Models
Add Your Heading Text Here
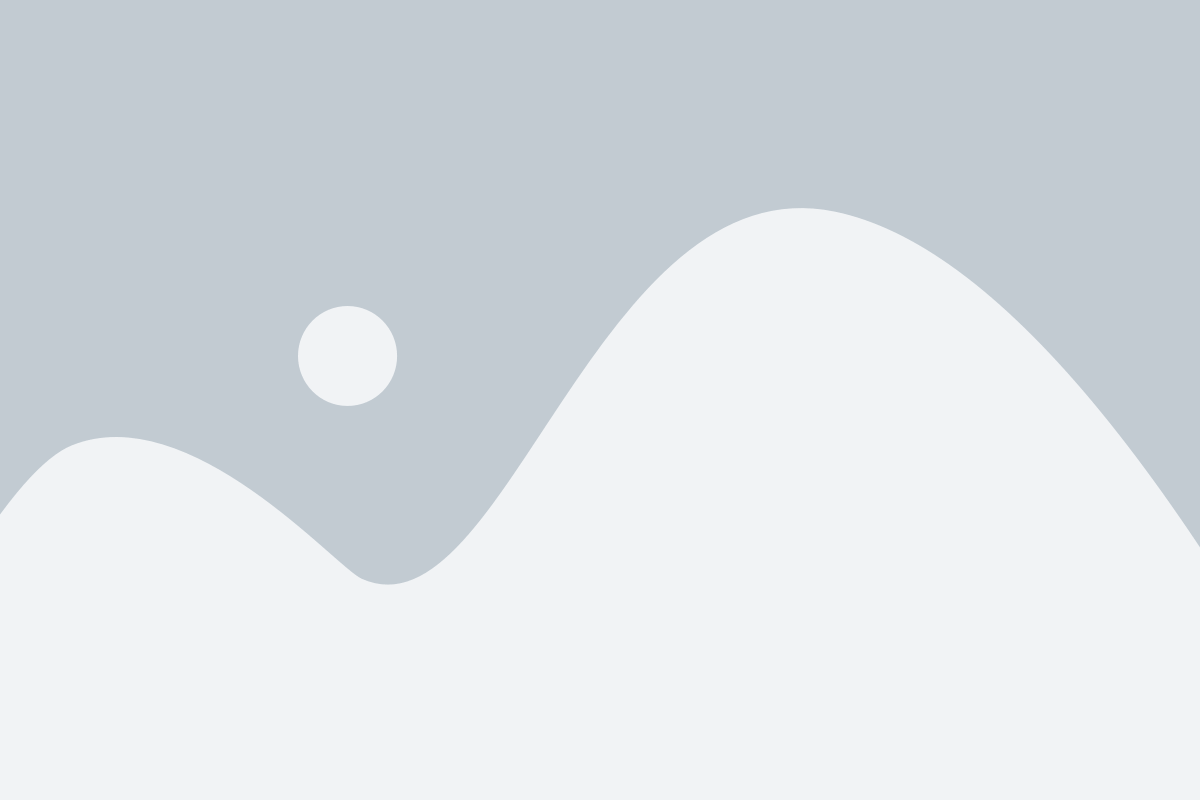
AlphaGo caused a stir by defeating 18-time world champion Lee Sedol in Go, a game thought to be impenetrable by AI for another 10 years. AlphaGo’s success is emblematic of a broader trend: An explosion of data and advances in algorithms have made technology smarter than ever before. Machines can now carry out tasks ranging from recommending movies to diagnosing cancer — independently of, and in many cases better than, humans. In addition to executing well-defined tasks, technology is starting to address broader, more ambiguous problems. It’s not implausible to imagine that one day a “strategist in a box” could autonomously develop and execute a business strategy. We’ve spoken to CXOs and leaders who express such a vision — and companies such as Amazon and Alibaba are already beginning to make it a reality.
Business Processes – Increasing productivity by reducing disruptions
AI algorithms are not natively “intelligent.” They learn inductively by analyzing data. While most leaders are investing in AI talent and have built robust information infrastructures,
As Airbus started to ramp up production of its new A350 aircraft, the company faced a multibillion-euro challenge. The plan was to increase the production rate of that aircraft faster than ever before. To do that, they needed to address issues like responding quickly to disruptions in the factory. Because they will happen. Airbus turned to artificial intelligence. It combined data from past production programs, continuing input from the A350 program, fuzzy matching, and a self-learning algorithm to identify patterns in production problems.
AI led to rectification of about 70% of the production disruptions for Airbus, by matching to solutions used previously — in near real time.
Just as it is enabling speed and efficiency at Airbus, AI capabilities are leading directly to new, better processes and results at other pioneering organizations. Other large companies, such as BP, Infosys, Wells Fargo, and Ping An Insurance, are already solving important business problems with AI. Many others, however, have yet to get started.
Integrated Strategy Machine – The Implementation Scope Augmented AI
The integrated strategy machine is the AI analog of what new factory designs were for electricity. In other words, the increasing intelligence of machines could be wasted unless businesses reshape the way they develop and execute their strategies. No matter how advanced technology is, it needs human partners to enhance competitive advantage. It must be embedded in what we call the integrated strategy machine. An integrated strategy machine is the collection of resources, both technological and human, that act in concert to develop and execute business strategies. It comprises a range of conceptual and analytical operations, including problem definition, signal processing, pattern recognition, abstraction and conceptualization, analysis, and prediction. One of its critical functions is reframing, which is repeatedly redefining the problem to enable deeper insights.
Amazon represents the state-of-the-art in deploying an integrated strategy machine. It has at least 21 data science systems, which include several supply chain optimization systems, an inventory forecasting system, a sales forecasting system, a profit optimization system, a recommendation engine, and many others. These systems are closely intertwined with each other and with human strategists to create an integrated, well-oiled machine. If the sales forecasting system detects that the popularity of an item is increasing, it starts a cascade of changes throughout the system: The inventory forecast is updated, causing the supply chain system to optimize inventory across its warehouses; the recommendation engine pushes the item more, causing sales forecasts to increase; the profit optimization system adjusts pricing, again updating the sales forecast.
Manufacturing Operations – An AI assistant on the floor
CXOs at industrial companies expect the largest effect in operations and manufacturing. BP plc, for example, augments human skills with AI in order to improve operations in the field. They have something called the BP well advisor that takes all of the data that’s coming off of the drilling systems and creates advice for the engineers to adjust their drilling parameters to remain in the optimum zone and alerts them to potential operational upsets and risks down the road. They are also trying to automate root-cause failure analysis to where the system trains itself over time and it has the intelligence to rapidly assess and move from description to prediction to prescription.
Customer-facing activities – near real time scoring
Ping An Insurance Co. of China Ltd., the second-largest insurer in China, with a market capitalization of $120 billion, is improving customer service across its insurance and financial services portfolio with AI. For example, it now offers an online loan in three minutes, thanks in part to a customer scoring tool that uses an internally developed AI-based face-recognition capability that is more accurate than humans. The tool has verified more than 300 million faces in various uses and now complements Ping An’s cognitive AI capabilities including voice and imaging recognition.
AI Strategy for Different Operational Models
To make the most of this technology implementation in various business operations in your enterprise, consider the three main ways that businesses can or will use AI:
Assisted intelligence
Now widely available, improves what people and organizations are already doing. For example, Google’s Gmail sorts incoming email into “Primary,” “Social,” and “Promotion” default tabs. The algorithm, trained with data from millions of other users’ emails, makes people more efficient without changing the way they use email or altering the value it provides. Assisted intelligence tends to involve clearly defined, rules-based, repeatable tasks.
Assisted intelligence apps often involve computer models of complex realities that allow businesses to test decisions with less risk. For example, one auto manufacturer has developed a simulation of consumer behavior, incorporating data about the types of trips people make, the ways those affect supply and demand for motor vehicles, and the variations in those patterns for different city topologies, marketing approaches, and vehicle price ranges. The model spells out more than 200,000 variations for the automaker to consider and simulates the potential success of any tested variation, thus assisting in the design of car launches. As the automaker introduces new cars and the simulator incorporates the data on outcomes from each launch, the model’s predictions will become ever more accurate.
Augmented intelligence
Augmented Intelligence, emerging today, enables organizations and people to do things they couldn’t otherwise do. Unlike assisted intelligence, it fundamentally alters the nature of the task, and business models change accordingly.
For example, Netflix uses machine learning algorithms to do something media has never done before: suggest choices customers would probably not have found themselves, based not just on the customer’s patterns of behavior, but on those of the audience at large. A Netflix user, unlike a cable TV pay-per-view customer, can easily switch from one premium video to another without penalty, after just a few minutes. This gives consumers more control over their time. They use it to choose videos more tailored to the way they feel at any given moment. Every time that happens, the system records that observation and adjusts its recommendation list — and it enables Netflix to tailor its next round of videos to user preferences more accurately. This leads to reduced costs and higher profits per movie, and a more enthusiastic audience, which then enables more investments in personalization (and AI).
Autonomous intelligence
Being developed for the future, Autonomous Intelligence creates and deploys machines that act on their own. Very few autonomous intelligence systems — systems that make decisions without direct human involvement or oversight — are in widespread use today. Early examples include automated trading in the stock market (about 75 percent of Nasdaq trading is conducted autonomously) and facial recognition. In some circumstances, algorithms are better than people at identifying other people. Other early examples include robots that dispose of bombs, gather deep-sea data, maintain space stations, and perform other tasks inherently unsafe for people.
As you contemplate the introduction of artificial intelligence, articulate what mix of the three approaches works best for you.
- Are you primarily interested in upgrading your existing processes, reducing costs, and improving productivity? If so, then start with assisted intelligence, probably with a small group of services from a cloud-based provider.
- Do you seek to build your business around something new — responsive and self-driven products, or services and experiences that incorporate AI? Then pursue an augmented intelligence approach, probably with more complex AI applications resident on the cloud.
- Are you developing a genuinely new technology? Most companies will be better off primarily using someone else’s AI platforms, but if you can justify building your own, you may become one of the leaders in your market.
The transition among these forms of AI is not clean-cut; they sit on a continuum. In developing their own AI strategy, many companies begin somewhere between assisted and augmented, while expecting to move toward autonomous eventually.