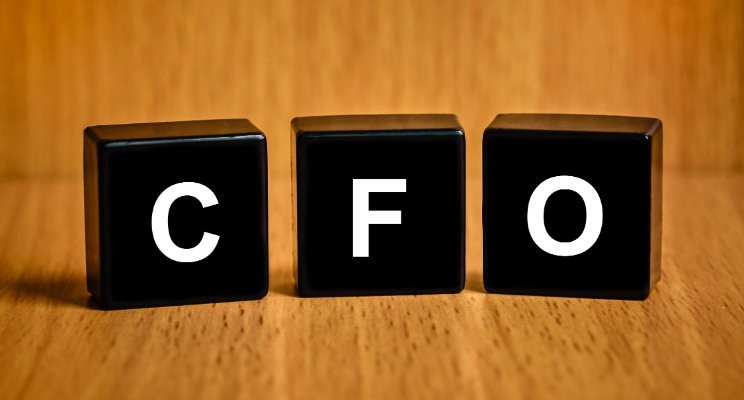
Financial transformation accomplished by AI – a perspective for Chief Financial Officer (CFO)
Add Your Heading Text Here
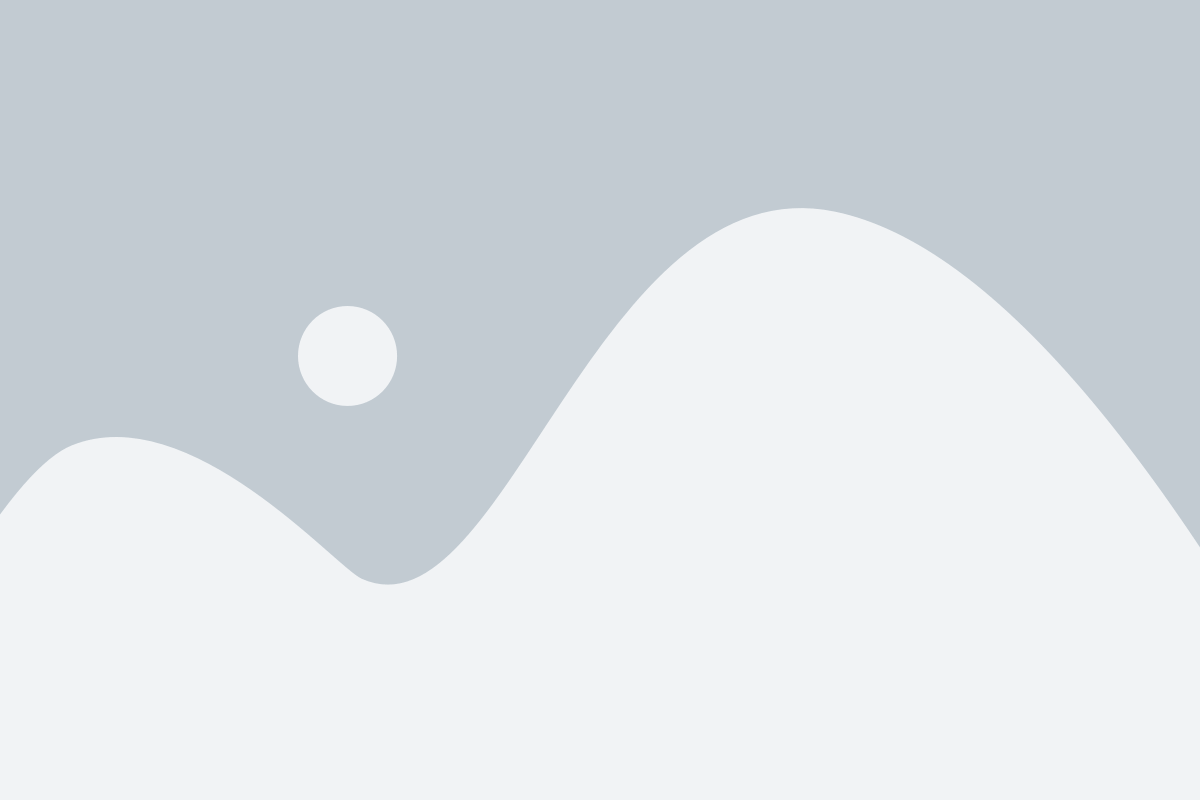
For organizations, transforming finance and accounting function via adoption of topical technology means improving how they pre-empt red flags around the financial transactions within the organizations.
In fact, prudent finance and accounting operability represents the single biggest challenge firms have to deliver on their priorities, according to a survey by Econsultancy.
What’s more, 45% of respondents indicated that embedding analytics &AI relevant as possible in the finance and accounting function is their key focus.
CFOs around the world are not asking if digital disruption will occur, but instead, what it means for their function. So the question asked in this article, is how can CFO’s leverage digital transformation wave using AI to advance their organization’s competitive position and improve performance of their function ?
CFO of Tomorrow
With business around the world undergoing digital transformation, the roles of the c-suite is also changing. Perhaps most significantly, the role of the chief financial officer is moving from simply counting pennies to being a major driver of change within companies.
In the past, the role of the CFO was all about getting the numbers. That was 90 per cent of their time, but that is changing and now it’s about understanding the source of the number, understanding what created the number, and understanding the business drivers behind the number. A CFO then needs to try to make sense of the business drivers, and be able to present to the board what the outlook for the organization is, what the costs are, and what actions need to be taken.
This means that the CFO has to have a solid handle on data and analytics, and once they have that in their arsenal, they can become a strategic adviser to the business and they are able to tell the CEO things like what impact customer satisfaction has on the business.
I want to further highlight a few use cases showing how disruptive technologies such as artificial intelligence (AI) and machine learning will be used in the office of the CFO to increase productivity, simplify processes, and support decision-making, and aid in digital finance evolution:
Digital chatbots : Digital assistants for CFOs could impact analytics and the way they handle them. Today, almost everybody in Financial Planning & Analysis (FP&A) receives countless calls asking for information like, “What was our revenue in Q3 last year for this product? What has our growth been over the last three years for this line of business?”
Smart assistants like Amazon’s Alexa and Apple’s Siri can already answer questions on weather forecasts, stock quotes, and so forth, but what if they could provide the latest financial results and give decision makers instant access to information? A CFO could have a conversation with his or her ERP system using a digital assistant to get an immediate response or a clarifying question, without having to open a dashboard or dig into a database.
Risk assessments: When we assess commercial proposals for our services projects, we evaluate each project individually based on the customer characteristics – maturity, industry, size, current system landscape, and so on – as well as the complexity of the products to be implemented. To qualify this assessment, we depend on managers who have previously worked on similar projects. That can limit us to the individual perspective of those managers.
Machine learning could give finance teams and executives the power to access decades’ worth of projects, around the world, at the touch of a button. In levering these insights, teams could then develop a better-informed risk assessment, mapping the project against a much larger database of historical projects.
Invoice clearing: In finance departments today, accounts receivable or treasury clerks can often be challenged in clearing invoice payments, as customers often combine invoices in one payment, pay incorrect amounts, or forget to include invoice numbers with their payments. To clear the invoice, the employee then has two options: manually add up various invoices that could possibly match the payment amount, or reach out to the customer to clarify. In the case of short payment, the employee either has to ask for approvals to accept the short payment or request the remaining amount from the customer.
What if an intelligent system could help streamline this process by suggesting invoices in real time that might match the paid amount and, based on established thresholds, automatically clear the short payments or automatically generate a delta invoice?
Expense-claim auditing: Expense-claim auditing is another routine, transactional finance task. Finance teams are tasked with ensuring that receipts are genuine, match claimed amounts, and are in line with company policy. While state-of-the-art travel-and-expense solutions can simplify the process, a manual audit still needs to be performed.
Machine learning and AI technologies could improve this process, auditing 100% of all claims, and sending only questionable claims to a manager for approval. The machine could read receipts – regardless of language – to ensure that they are genuine, and match them against the policy.
Accruals: Artificial intelligence and machine learning also offer promise when it comes to determining bonus accruals. Today, teams have a myriad of factors to consider when determining bonus accruals. CFO teams look at current headcount salaries and bonus plans, and try to forecast all KPIs in compensation plans. From there, teams try to calculate the most accurate accrual (likely adding a buffer, to be safe). However, oftentimes, accuracy ends of being a matter of luck more than anything else.
By applying machine learning to these calculations, predictive analytics could serve as a valuable tool to generate unbiased accrual figures, leaving finance teams more time during closing periods for other activities that require human review and judgment.
Customer Journey: This is an area where the CFO is ideally placed to play a greater role in contributing to company growth and profits. His perspective on new customer acquisition, retention activities, customer development and predictive customer behavior models is crucial.
AI is what’s making all of this possible. With his new 360° vision and customer knowledge, the CFO can become a strategic business leader. Via AI and the breaking down of old company silos, the customer journey becomes everyone’s concern. And customer engagement wins its rightful place at the heart of business strategy.
The overall impact on jobs in finance: As these advanced technologies continue to penetrate the finance function, a new crop of skills are rising to the forefront when it comes to hiring finance talent. Routine, transactional roles will become less prevalent, while the need for strategic thinkers with cross-functional knowledge and technology prowess will be critical. Additionally, while transactional tasks will be fewer, digital transformation will require additional finance resources to be developed and supported, creating an opportunity to redefine processes and roles.
CFO’s, like everyone else, will have to adopt AI
In the future, it will be the companies that can harness AI that will set themselves apart. They will become fully digital businesses. Forward-thinking CFOs will help this happen. Because, by making AI accessible company-wide, they now have the power to unleash infinite company value.
Related Posts
AIQRATIONS
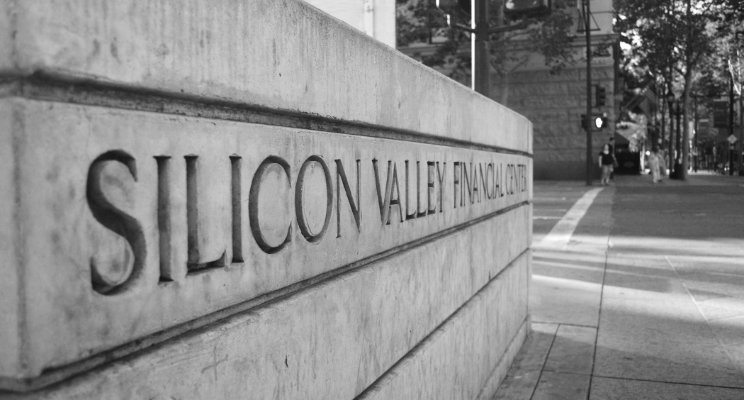
The gold rush for AI – silicon valley vs. China – a perspective hard to ignore
Add Your Heading Text Here
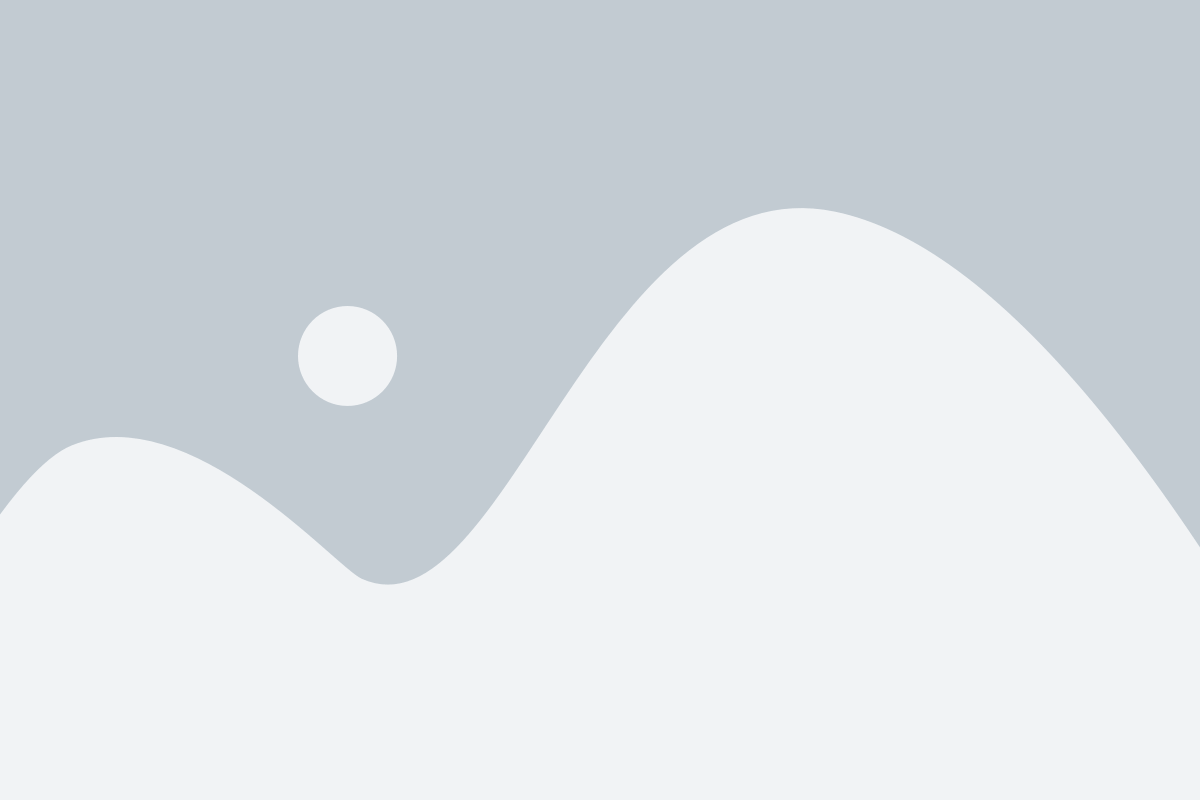
The buzzword among the business and tech communities in China for the past year has been ‘AI’, or artificial intelligence. Artificial intelligence, which allows software to “learn” human ways of thinking, is being incorporated into the largest e-commerce platforms, including Baidu, Alibaba, and Tencent, as well as into data-intensive traditional sectors. With strong government backing and concentrated research in this area, AI is poised to drive China’s economy forward toward higher levels of growth.
China is developing artificial intelligence in improving the capabilities of robotics, developing driverless cars, divining consumer preferences, inventory forecasting, selling enhanced products, and marketing goods and services. According to Liu Lihua, Vice Minister of Industry and Information Technology, China has thus far applied for 15,745 AI patents.
China plans to launch a national AI plan, which will strengthen AI development and application, introduce policies to contain risks associated with AI, and work toward international cooperation. The plan will also provide funds to back these endeavors. Some municipalities also support AI research programs. Beijing, for example, is home to the CAS Institute of Automation, a consortium of universities and firms that provides venture capital funding of 1 billion RMB ($150 million) to AI development. Zhejiang province has also embraced AI programs. Already, Geely Automobile in Zhejiang is using intelligent manufacturing and internet marketing services based on AI to boost sales.
BAT – Chinese AI Frontier Giants
China’s BAT, or Baidu, Alibaba and Tencent, is leading the way for AI in China. Baidu was the first Chinese company to embark upon research in AI, using a system known as Duer to be used in home devices and driverless cars. Driverless auto software provided by Baidu will be made available to car manufacturers under the Apollo Project. Alibaba is using AI to forecast regional order quantities and to improve logistics efficiency, while Tencent has released a platform for deep learning using social data.
Baidu, Alibaba and Tencent have been vying for top talent in AI in order to become leaders in this area. Making headlines several days ago, Alibaba lured Ren Xiaofeng from Amazon.com to lead its own technology lab, which aims to make headway in artificial intelligence. Tencent brought Baidu’s AI expert Zhang Tong on board in March. In 2014, Baidu poached Andrew Ng from the Google Brain project to lead the Baidu Research Institute (though he recently stepped down).
Bay Area dominates this year’s AI funding
Venture investment in startups that are applying artificial intelligence or machine learning has more than tripled in the U.S. since 2013, according to PitchBook Data, with about 60 percent of that coming to founders in the Silicon Valley Bay Area.
The Seattle investment research firm put together a ranking of the top 20 AI deals done around the world this year for me while I was researching this week’s Silicon Valley Business Journal cover story. Almost half of the startups that were funded and nearly three-quarters of the investors involved were from San Francisco and the Silicon Valley region.
The new era in Silicon Valley centers on artificial intelligence and robots, a transformation that many believe will have a payoff on the scale of the personal computing industry or the commercial internet, two previous generations that spread computing globally. Computers have begun to speak, listen and see, as well as sprout legs, wings and wheels to move unfettered in the world.
Silicon Valley’s financiers and entrepreneurs are digging into artificial intelligence with remarkable exuberance. The region now has at least 19 companies designing self-driving cars and trucks, up from a handful five years ago. There are also more than a half-dozen types of mobile robots, including robotic bellhops and aerial drones, being commercialized.
Funding in A.I. start-ups has increased more than fourfold to $681 million in 2015, from $145 million in 2011, according to the market research firm CB Insights. The firm estimates that new investments will reach $1.2 billion this year, up 76 percent from last year.
Even Silicon Valley’s biggest social media companies are now getting into artificial intelligence, as are other tech behemoths. Facebook is using A.I. to improve its products. Google will soon compete with Amazon’s Echo and Apple’s Siri, which are based on A.I., with a device that listens in the home, answers questions and places e-commerce orders. Satya Nadella, Microsoft’s chief executive, recently appeared at the Aspen Ideas Conference and called for a partnership between humans and artificial intelligence systems in which machines are designed to augment humans.
The auto industry has also set up camp in the valley to learn how to make cars that can do the driving for you. Both technology and car companies are making claims that increasingly powerful sensors and A.I. software will enable cars to drive themselves with the push of a button as soon as the end of this decade — despite recent Tesla crashes that have raised the question of how quickly human drivers will be completely replaced by the technology.
AI is in it for the long-haul
Whenever there is a new idea, the valley swarms it. But you have to wait for a good idea, and good ideas don’t happen every day. Silicon Valley’s new A.I. era underscores the region’s ability to opportunistically reinvent itself and quickly follow the latest tech trend. This is at the heart of the region’s culture that goes all the way back to the Gold Rush. The valley is built on the idea that there is always a way to start over and find a new beginning.
Related Posts
AIQRATIONS
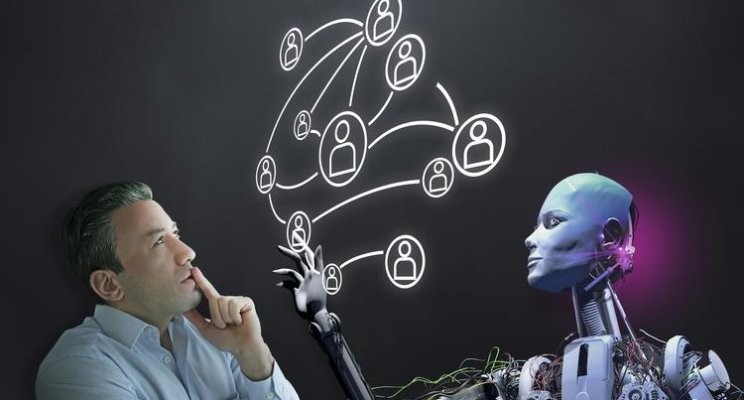
Future of HR :Redefined by AI – perspectives for chief people officer
Add Your Heading Text Here
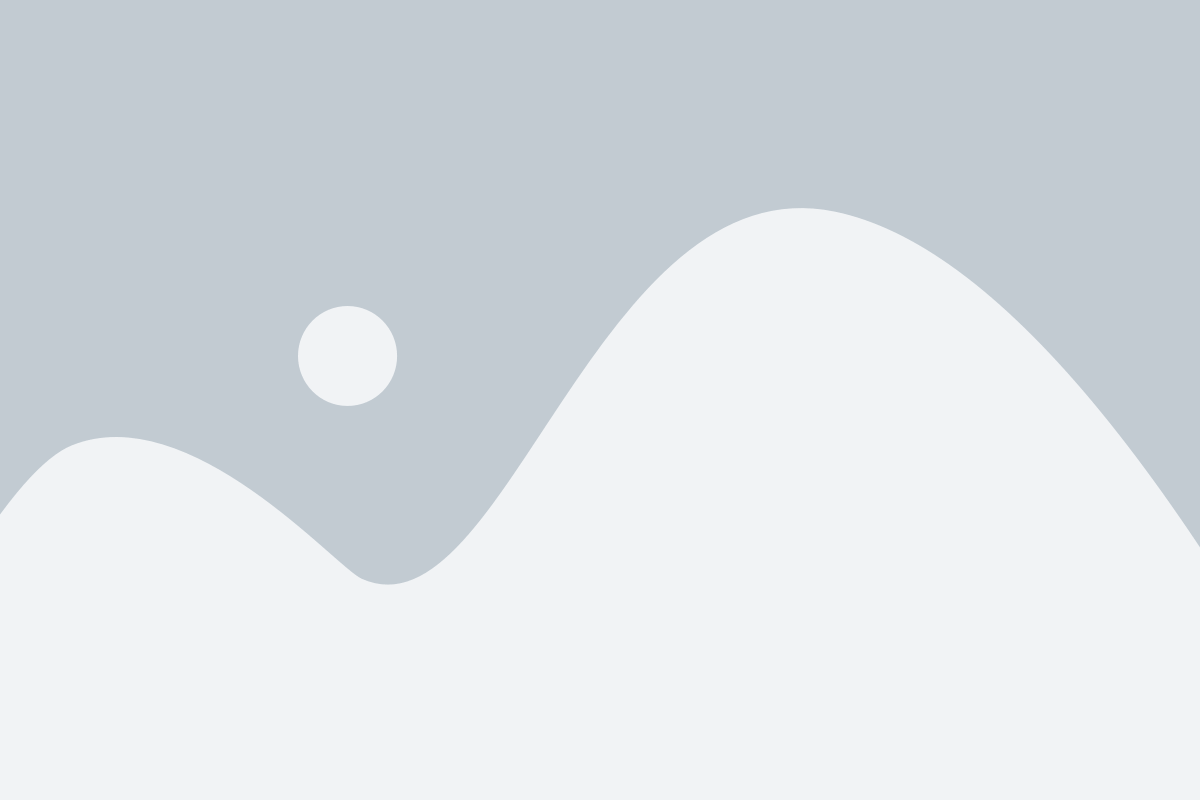
Artificial intelligence is transforming our lives at home and at work. At home, you may be one of the 1.8 million people who use Amazon’s Alexa to control the lights, unlock your car, and receive the latest stock quotes for the companies in your portfolio. In total, Alexa is touted as having more than 3,000 skills and growing daily. In the workplace, artificial intelligence is evolving into an intelligent assistant to help us work smarter. Artificial intelligence is not the future of the workplace, it is the present and happening today.
Investment in AI has accelerated from $282 million in 2011 to $2.4 billion in 2015, a 746% increase in five years. In 2016, this continued to increase with roughly another $1.5 billion being invested in more than 200 AI-focused companies in 2016. AI is becoming indispensable in the healthcare industry. Will we be saying the same thing about CHROs using artificial intelligence in the workplace? Will we consider it unthinkable not to use intelligent assistants to transform recruiting, HR service centers, and learning and development? I believe the answer is yes. HR leaders will need to begin experimenting with all facets of AI to deliver value to their organizations. As intelligent assistants become more widely used in our personal lives, we will expect to see similar usage in the workplace.
For employees, chatbots deliver an unmatched level of employee experience, from real time answers for HR questions to personalized learning and development. In addition, they are critically important to the 3.7 million workers, or 2.8% of the workforce, who work remotely at least half time and do not have easy access to an HR department.
For HR leaders, chatbots are well suited to improving talent acquisition and on-boarding processes by increasing speed and providing greater consistency in answering frequently asked HR questions, improving the talent acquisition process, and enhancing the online learning experience.
AI Chatbots To Answer Frequently Asked Employee Questions
Let’s consider Jane, a chatbot created by Loka, in 2014. Jane provides real time answers to a range of HR questions, including, “Are we off on President’s Day?” or “What are my dental benefits?” Jane is capable of answering any question and answer set that can be stored in a database. In addition to answering frequently asked questions, CEO Bobby Mukherjee says Jane is designed to proactively promote benefits to employees they may not yet know about. Says Mukherjee, “Companies are coming up with lots of new benefits, but they do not have an effective way to promote usage.” Imagine Jane can reach out to employees with, “Hey John, have you tried our Yoga class that we are offering in your building today at 3:00 pm? Click here to automatically book yourself. You’ve been working hard and you deserve it!”
Another value of Jane is the opportunity to track employee issues using real time analytics and then apply sentiment analysis to address these issues. Let’s say that a majority of employees are asking questions about late payments for travel reimbursements. This data can indicate something in the system isn’t working correctly. Before things become a full blown issue, HR leaders can uncover the issue and communicate a solution.
Granted there will be questions Jane cannot answer yet, but the opportunity is here to provide AI for all types of HR related questions that might be coming into your HR Service Center.
AI To Improve Talent Acquisition
Talent acquisition and new hire on-boarding are ripe areas where intelligent assistants can tap multiple data sources to develop candidate profiles, schedule interviews, and make decisions about prospective job candidates.
Talla is a chatbot designed to augment the HR processes that source job candidates. Talla can provide a set of interview questions based upon the role, and can even conduct a Net Promoter Score survey following the recruiting process. Rob May, CEO of Talla, sees, “an intelligent assistant as being able to augment a mid-level HR professionals’ job so she can focus on more strategic HR issues.” The vision behind launching Talla is to ultimately become a real time advisor to HR professionals in how they source and on-board new hires.
May estimates that Talla will save many hours in recruiting and on-boarding new hires and will greatly enhance the employee experience. Improving talent acquisition and new hire on-boarding is a priority for CHROs. According to Eric Lesser, Research Director of IBM Institute for Business Value, “More than half of the CHROs surveyed believe cognitive computing will affect a wide range of roles in the HR organization, ranging from senior executives to individuals working in service centers.”
AI for Talent Management Inside Workplace
AI can also be used for intricate data collection of employees in their workplace in an automated fashion. One option would be to track the movements of the employees inside office space to assess their activities on ground. Companies can track employees’ whereabouts in the office. Bluvision makes radio badges that track movement of people or objects in a building, and display it in an app and send an alert if a badge wearer violates a policy set by the customer—say, when a person without proper credentials enters a sensitive area. The system can also be used to track time employees spend, say, at their desks, in the cafeteria or in a restroom.
Bluvision’s AI compensates for the margin of error in determining location of radio transmitters, allowing the system to locate badges with one-meter accuracy, according to COO John Sailer. Without it, people near one another would be indistinguishable, and the positions of doors, desks, walls and the like—useful information for security and optimizing use of space—would be blurred.
The system is also useful in situations where contractors are paid hourly or piecemeal, such as on a construction site, where subcontractors must complete work in order and on schedule to avoid cost overruns. Although Bluvision tracks individuals, it can also be set to present only aggregate trends. That allows customers to take advantage of location tracking without breaking privacy laws or agreements protecting personally identifying information about employees.
The limits of AI Currently
For all their promise, these systems raise a number of issues. Some are evident today, in the early stages of adoption, while others may take time to become clear.
Privacy is an obvious concern when tracking employees, particularly personal behavior. Systems that sort job candidates also raise questions. Entelo’s may emphasize people with a large online footprint; SAP’s might prefer those who best match characteristics of people who were hired in the past.
Also, the limitations of current approaches boil down to the difficulty of drawing valid conclusions from incomplete data. For instance, measurements of employee performance at any given company are based on the set of people hired and lack information about candidates who were passed over—or weren’t even interviewed—who may have, say, produced more in less time. Aggregating data from many customers, as some larger vendors including SAP and Workday do, can reduce bias, but the problem remains that different companies may not track the same variables in the same way, and subtle but important ones are likely to be missing.
Related Posts
AIQRATIONS
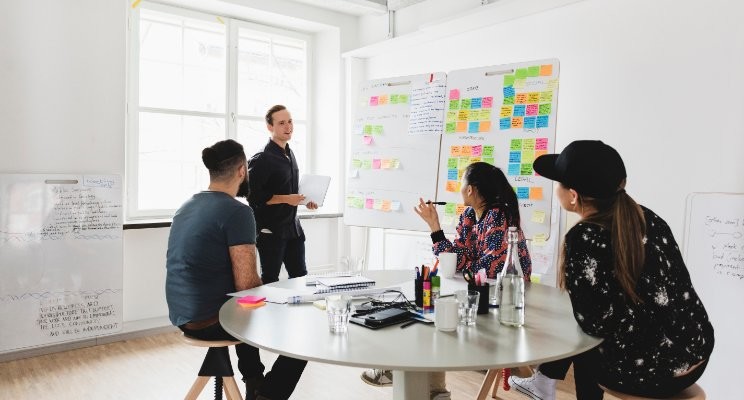
How Startups can leverage AI to gain competitive advantage
Add Your Heading Text Here
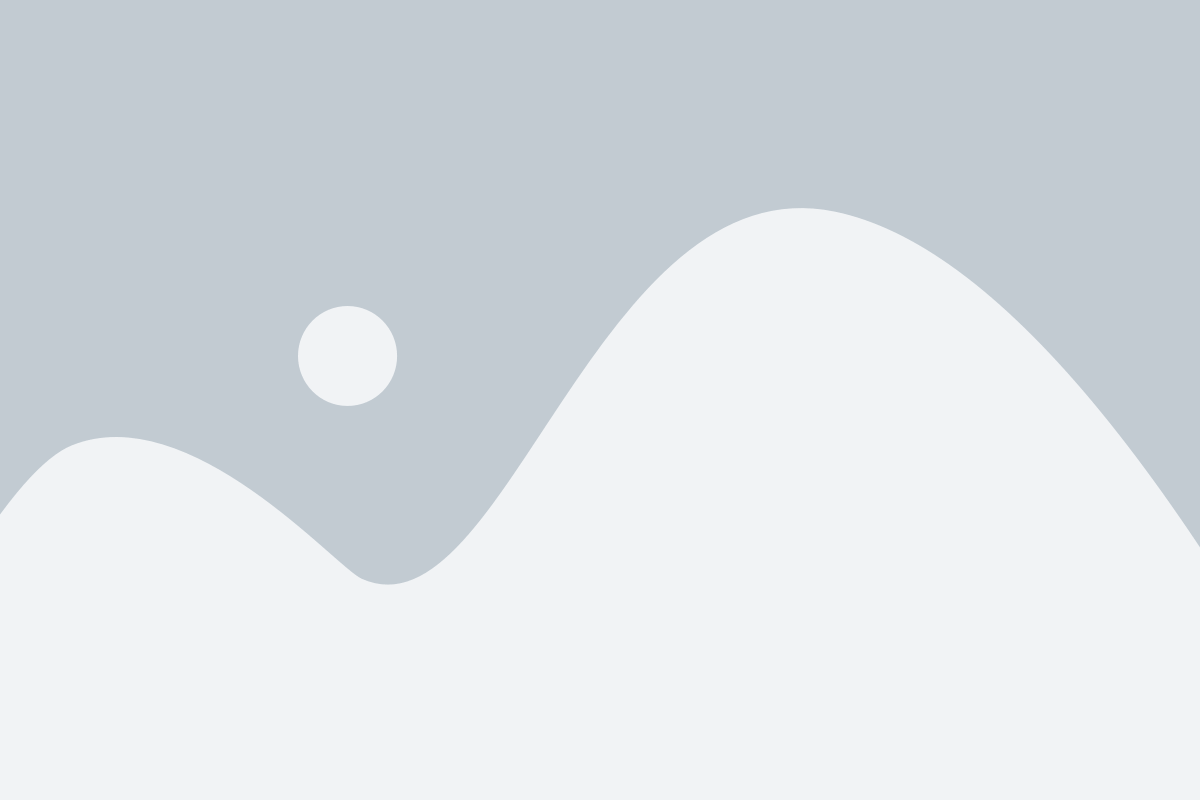
Despite nationwide venture funding hitting a multiyear low, venture capital deployed to artificial intelligence (AI) startups has reached a record high.
Last year, VCs struck 658 deals with AI companies, nearly five times the number that signed on the dotted line four years before. To date, the market contains 2,045 AI startups and more than 17,000 market followers, with more joining by the day.
AI’s rapid rise has swept up startups and enterprises alike, including U.S. automaker Ford, which recently bought AI startup Argo for $1 billion. The acquisition cements experts’ suspicions of Ford’s coming foray into self-driving technology. Other startups — so many, in fact, that entrepreneurs need a “best of” guide — are betting heavily on bot platforms.
So while we’ve just glimpsed the tip of this innovation iceberg, it’s clear AI is no longer some nebulous technology of the future. Sixty-eight percent of marketing executives, report using AI in their operations. For a technology that only went mainstream in 2016 and barely existed four years ago, that’s a remarkable adoption rate. How, regardless of the platform you choose, can you join forward-thinking entrepreneurs and build your business with AI? Over the last few years , I have worked closely with multiple start ups across genres and ,So far, four ways stand out:
1. Get to know your next customer.
A politician wouldn’t dream of delivering a small-town stump speech to her urban constituents. Why? Because you’ve got to know your audience. The same is true for entrepreneurs. Before you broadcast your message, you need to know who you’re trying to reach.
Node, an account-based intelligence startup, uses natural language processing — a fancy term for teaching a computer to understand how we humans speak and write — to develop customer profiles. Node is crunching vast swaths of data to connect the dots between marketers and the companies they’re trying to reach.
Once you have ample customer data — Node uses data crawlers to scrape information from social media, news sites and more — pair machine learning and natural language processing models to extract sentiments from unstructured data. Then, just as senators segment constituents into demographic groups, Node uses cluster analysis to sort clients’ customers into like cohorts.
2. See how people truly use your product.
If, heaven forbid, you forgot to tag your neighbor at last week’s house party, Facebook was no doubt there to remind you of your error. How does Facebook know which of your friends you left untagged? It has gone all-in on an AI technique called convolutional neural networks.
Convolutional neural networks, which loosely model how the brain’s visual cortex interacts with the eyes, work by separating an image into tiny portions before running each of those specks through a multilayered filter. It then “sees” where each speck overlaps with other parts of the image, and through automated iterations, it puts together a full image.
Many different ways exist to apply this technology, but retail businesses can start with image classification. Try using a convolutional neural network to break down photos of your products posted online. The model can identify customer segments that frequently use your product, where they’re using it and whether they commonly pair other products with yours. Essentially, this automated image analysis can show you how your products fit into customers’ lives, allowing you to tailor your marketing materials to fit.
3. Get inside the user’s head.
Success on social media requires careful listening and quick action. When a social campaign isn’t working, it’s best to put it out of its misery quickly. On the other hand, when one strikes a chord with customers, doubling down pays dividends.
But to do so, you need real-time insights about customers’ reactions to your content. Fortunately, AI can take the emotional temperature of thousands of customers at once. Dumbstruck, a video-testing and analytics startup that I advise, has added natural language processing to its emotional analytics stack. This allows it to provide moment-by-moment insights into viewers’ reactions to media. Dumbstruck’s model grows stronger with each reaction analyzed, producing a program that perceives human emotions even better than some people can.
4. Provide affordable, always-on support.
Customer service is — or should be, according to consumers — the department that never sleeps. More than half of people, 50.6 percent to be precise, believe a business should be available 24/7 to answer their every question and concern. When asked whether businesses should be available via a messaging app, the “yes” votes jump to nearly two in three.
Fortunately, bots don’t sleep, eat or go off-script. A well-built bot can offer cost-effective, constant customer service. Of course, grooming your bot to serve customers requires front-end data — ideally hundreds of thousands of example conversations — but you can get started with a human-chatbot hybrid. With this approach, the bot answers run-of-the-mill questions, while a human takes over for the more complex ones. Then, as the data builds and the model matures, you can phase in full automation.
AI’s Impact on small businesses and startups
Small enterprises will begin to use the tried and tested platforms in innovative ways. While startups will gain a competitive edge in capturing the AI market, the larger enterprises will provide the infrastructure to startups for building innovative services. It is somewhat similar to the business model followed when the cable technology was introduced.
Startups leveraging AI technology for industry verticals, like agriculture, manufacturing or insurance are bound to be successful.
Startups can empower established insurance companies like State Farm, Allstate and Farmers with technology enabling them to become more proactive in policy planning. For instance, a new AI insurance underwriter will help to forecast natural disasters and accidents, and adjust premiums.
The predictive decision-making capabilities are more than just a novel technology. You can manage food supply chains with the help of AI. Startups could develop end-to-end farming solutions with AI analytics for reducing food waste. It will have a huge impact in tackling global issues of hunger and famine.
Whether serving as a research assistant in a large corporation, acting as a voice-activated resource in difficult medical procedures, AI is fast becoming a reality. The AI revolution will benefit new players who learn quickly to use it to their advantage. AI will be a fundamental predictive enabler helping us solve large-scale problems, and startups are poised to gain a competitive edge.
So what’s the ground level AI sentiment of Startups? – Mix of Hope & Fear
Regardless of which industry you operate, be careful that AI will affect your world in some way. Look into what is present now and how you can utilize it to gain a competitive edge.
The possibilities with AI are endless; enterprises will become efficient, intelligent and cost-effective.
Undoubtedly, the digital revolution and AI will advance to a point where it will offer real-world benefits to every business- large and small.
Mark Zuckerberg says, “We’re working on AI because we think more intelligent services will be much more useful for you to use.”
AI is relevant because of its immense power to deliver useful solutions; its other building blocks including cloud computing and superfast connectivity. But, if you want to take advantage of this novel technology you will need a reliable, secure, and continuously evolving infrastructure.
Related Posts
AIQRATIONS
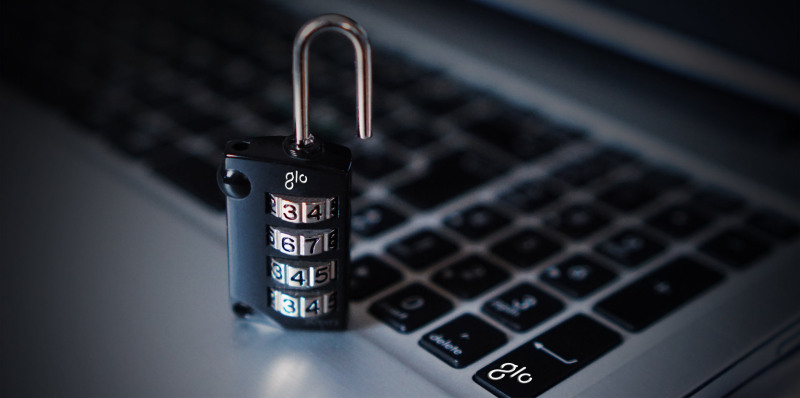
Beating Back Cyber Attacks with Analytics – A Topical Perspective
Add Your Heading Text Here
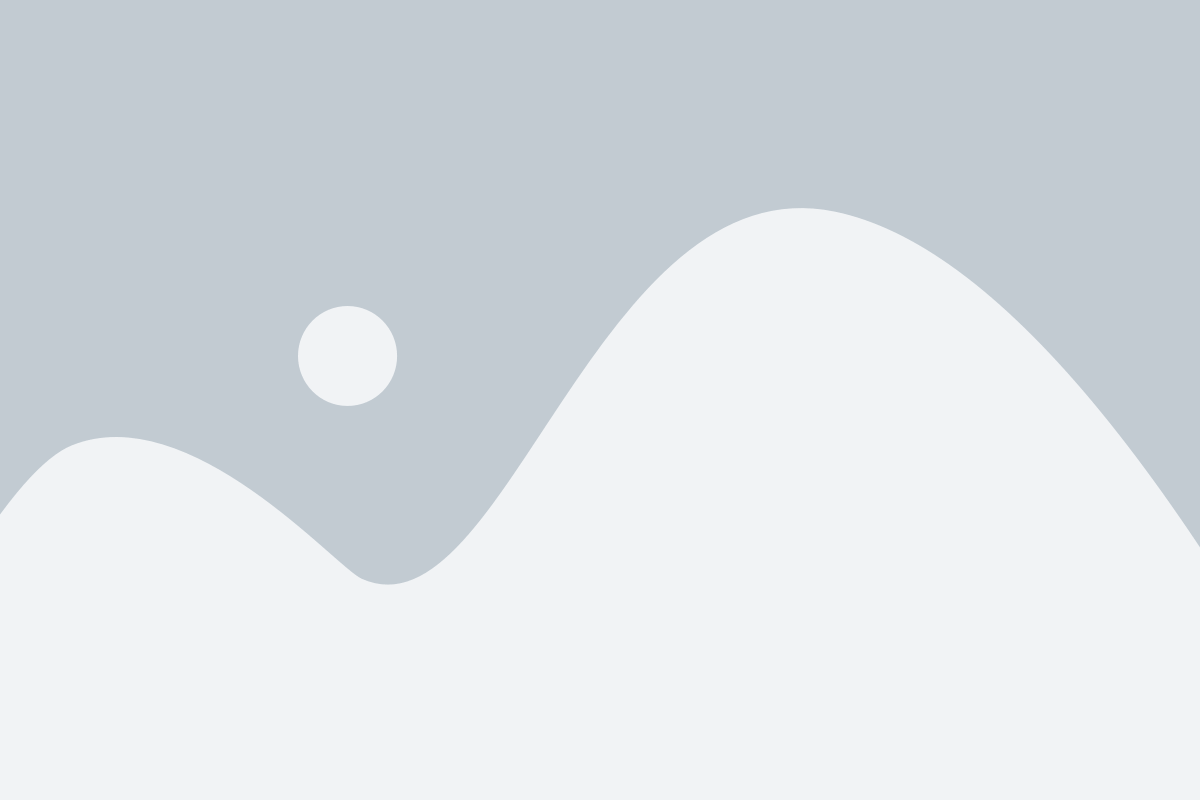
The worldwide cyber attack that began last Friday and goes by the name of “WannaCry” has highlighted the need for governments and businesses to strengthen their security infrastructure, in addition to calling attention to the need to mandate security updates and educate lawmakers about the intricacies of cyber security.
During the WannaCry attacks, hospitals had to turn away patients, and their ability to provide care was altered significantly. Even though the threat is widely acknowledged to be real by the information security community and anyone not living under a rock, and the stakes are higher than ever, most organizations and almost all healthcare providers are still using old-school cybersecurity technologies and retain their reactive security postures.
The WannaCry ransomware attack moved too quickly for security teams to respond, but a few organizations were able to spot the early indicators of the ransomware and contain it before the infection spread across their networks. While it wreaked havoc across the globe, there was nothing subtle about it. All of the signs of highly abnormal behavior on the networks were there, but the pace of the attack was far beyond the capacity of human teams contain it. The latest generation of AI technology enabled those few organizations to defend their networks at the first sign of threat.
Meanwhile, threats of similar – or perhaps worse – attacks have continued to surface. This was not the big one. This was a precursor of a far worse attack that will inevitably strike — and it is likely, unfortunately, that [the next] attack will not have a kill switch. This is an urgent call for action for all of us to get the fundamentals finally in place to enable us to withstand robustly this type of a crisis situation when the next one hits.
Modern malware is now almost exclusively polymorphic and designed in such a way as to spread immediately upon intrusion into a network, infecting every sub-net and system it encounters in near real-time speed. Effective defense systems have to be able to respond to these threats in real time and take on an active reconnaissance posture to seek out these attacks during the infiltration phase. We now have defense systems that have applied artificial intelligence and advanced machine learning techniques and are able to detect and eradicate these new forms of malware before they become fully capable of executing a breach, but their adoption has not matched the early expectations.
As of today, the vast majority of businesses and institutions have not adopted nor installed these systems and they remain at high risk. The risk is exacerbated further by targets that are increasingly involved with life or death outcomes like hospitals and medical centers. All of the new forms of ransomware and extortionware will increasingly be aimed at high-leverage opportunities like insulin pumps, defibrillators, drug delivery systems and operating room robotics.
Network behavioral analytics that leverage artificial intelligence can stop malware like WannaCry and all of its strains before it can form into a breach. And new strains are coming. In fact, by the time this is published, it would not surprise me to see a similar attack in the headlines.
Aanlytics is Turning the Table on Security Threats
The more comprehensive, sensitive and greater volume of end user and customer data you store, the more tempting you are to someone wanting to do harm. That said, the same data attracting the threat can be used to thwart an attack. Analytics includes all events, activities, actions, and occurrences associated with a threat or attack:
- User: authentication and access location, access date and time, user profiles, privileges, roles, travel and business itineraries, activity behaviors, normal working hours, typical data accessed, application usage
- Device: type, software revision, security certificates, protocols
- Network: locations, destinations, date and time, new and non-standard ports, code installation, log data, activity and bandwidth
- Customer: customer database, credit/debit card numbers, purchase histories, authentication, addresses, personal data
- Content: documents, files, email, application availability, intellectual property
The more log data you amass, the greater the opportunity to detect, diagnose and protect an organization from cyber-attacks by identifying anomalies within the data and correlating them to other events falling outside of expected behaviors, indicating a potential security breach. The challenge lies in analyzing large amounts of data to uncover unexpected patterns in a timely manner. That’s where analytics comes into play.
Leveraging Data Science & Analytics to Catch a Thief
Using data science, organizations can exercise real-time monitoring of network and user behaviors, identifying suspicious activity as it occurs. Organizations can model various network, user, application and service profiles to create intelligence-driven security measures capable of quickly identifying anomalies and correlating events indicating a threat or attack:
- Traffic anomalies to, from or between data warehouses
- Suspicious activity in high value or sensitive resources of your data network
- Suspicious user behaviors such as varied access times, levels, location, information queries and destinations
- Newly installed software or different protocols used to access sensitive information
- Identify ports used to aggregate traffic for external offload of data
- Unauthorized or dated devices accessing a network
- Suspicious customer transactions
Analytics can be highly effective in identifying an attack not quite underway or recommending an action to counter an attack, thus minimizing or eliminating losses. Analytics makes use of large sets of data with timely analysis of disparate events to thwart both the smallest and largest scale attacks.
The Analytics Solution to Security Monitoring
If security monitoring is a data storage problem, then it requires a analytics solution capable of analyzing large amounts of data in real time. The natural place to look for that solution is within Apache Hadoop, and the ecosystem of dependent technologies. But although Hadoop does a good job performing analytics on large amounts of data, it was developed to provide batch analysis, not real-time streaming analytics required to detect security threats.
In contrast, the solution for real-time streaming analytics is Apache Storm, a free and open source real-time computation system. Storm functions similar to Hadoop, but was developed for real-time analytics. Storm is fast and scalable, supporting not only real-time analytics but machine learning as well, necessary to reduce the number of false positives found in security monitoring. Storm is commonly found in cloud solutions supporting antivirus programs, where large amounts of data is analyzed to identify threats, supporting quick data processing and anomaly detection.
The key is real-time analysis. Big data contains the activities and events signaling a potential threat, but it takes real-time analytics to make it an effective security tool, and the statistical analysis of data science tools to prevent security breaches.
When do you need to start? – Yesterday
Yesterday would have been a good time for companies and institutions to arm themselves against this pandemic. Tomorrow will be too late.