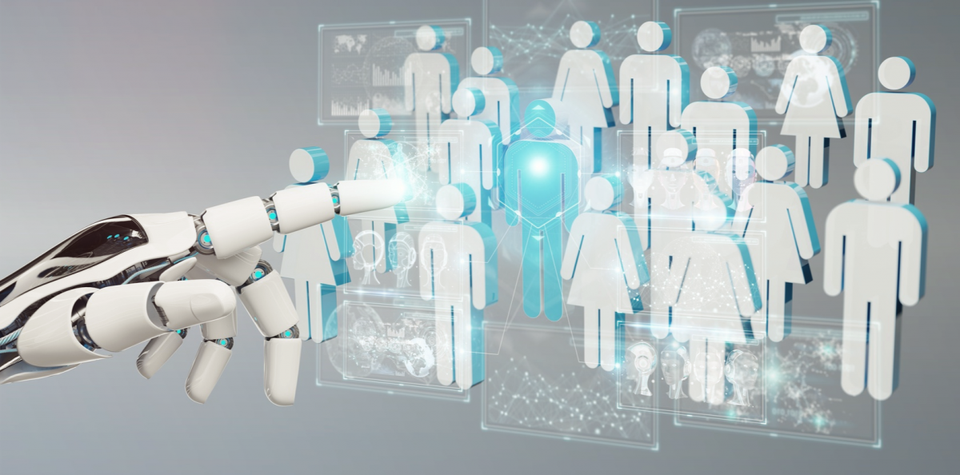
AI And Societal Impact – Addressing Large, Complex Unresolved Problems With AI
Add Your Heading Text Here
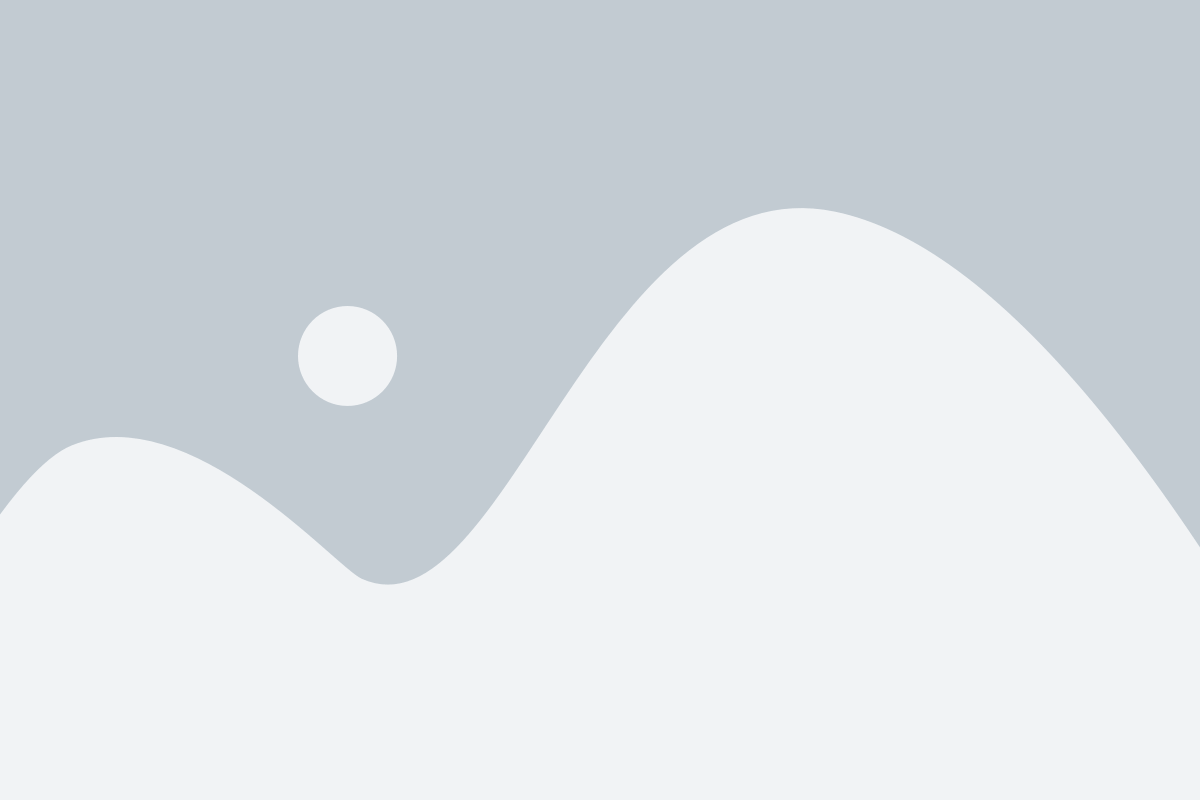
The idea that AI will conjure up an apocalyptic, robot-ruled future, where mechanical overlords govern humans is an extremely low probability event, even in the very distant future. In fact, not only are AI-driven interventions accelerating business outcomes – AI is also helping nations around the world find new avenues for enabling positive social outcomes for their people.
For all the evolution and development of humanity and technology over the years, our world still faces pressing systemic challenges that affect humanity at a large scale. From our complex and labyrinthine legal systems to the inefficiencies in our healthcare sector, large-scale problems still abound. The need of the hour is to better connect the people with the basic facilities they require. AI may not be a panacea in and of itself, but it offers a huge potential to improve the quality of life of people across the globe. Thankfully, today multiple nations have the intellectual capital – our peers in the software engineering and AI domains – that can bring substantial dividends for the population at large.
In this article, I will attempt to touch upon how AI can be used to address large, complex and unsolved problems and contribute to improving the quality of life for humanity. In keeping with WTISD’s topic for this year – Enabling the Positive Use of AI for All – I’ll share a social perspective on how AI-powered innovations can be hugely transformational to the world:
Improve Access to Healthcare Facilities
Available statistics show that over 45% of WHO Member States report to have less than 1 physician per 1000 people. (World Health Organization recommends a ratio of 1:1000). When you add to that the inequitable spread of doctors across certain countries, we have a poorly served population. While the life expectancy at a global level is 72 years (average across both males and females), the disparity between regions can be startling. For instance, the average in the WHO’s Africa region is a low 61.2 years. By imbibing AI, we can deliver an exponential improvement in health outcomes by improving medical adherence to reduce readmissions, tracking patient medical histories, improving access to physicians, reducing the time spent in clinics and prescribing personalized treatment pathways. Using AI, we can:
- Identify high population density areas that are currently underserved by hospitals. This can provide policymakers with inputs on how they can improve the deployment and availability of doctors, medical equipment and medication
- Leverage early warning signals through alternative mediums such as social media tracking for public health studies to provide guided diagnosis and interventions
- Create a digital record of patients’ medical histories and their clinical notes and use that as a reference for prescribing evidence-based treatment options and developing tailored treatment pathways
- Improve patient medical adherence by identifying individuals without health insurance, providing coverage and incentivizing the use of appropriate medication and treatment
- Speed up routine clinical processes such as scanning and annotating X-Rays and CT-Scans using computer vision and prescribing actions to physicians.
Revamp the Education System
The education system is undeniably critical for shaping future generations. However, both quality of and access to education is incredibly disparate across the developed and developing worlds. Curricula can often be outdated, thus not providing students with the skills they need for their careers. Problems abound in the education sector – from a high level of student dropouts, quality and methodology of teaching and lack of workforce readiness among students. While policymakers mull over how education can be made more contemporary and effective, AI can help provide guided interventions in the field of education by:
- Mapping the aptitude and interest of students in schools and universities with skills that are demanded by the market. This will help provide prescriptive career guidance that will be beneficial to both the employers and the future workforce
- Tracking the demand for skills in the market and the educational infrastructure available to supply those skills, through a Skills Repository. This will help keep education concurrent with current market demands and ensure much better alignment between academia and corporates
- Automate routine, time-consuming tasks – from creating and grading test papers, developing personalized benchmarks for each student, identifying gaps in student development, tracking aptitude and attentiveness within each subject, and enabling teachers to focus on curriculum development, coaching and mentoring, and improving behavioral and personality aspects of students
- Identify potential school and university-level dropouts and their root-causes so educational institutions can take proactive steps to ensure student retention and course completion.
Address Legal and Law Enforcement Challenges
Globally, we face structural issues in areas of law enforcement and jurisprudence. Globally the average police-to-people ratio is 1 police personnel per 604 people, which is lower than the UN recommended standard of 1 per 454. Poor law enforcement eventually lends itself to a high crime rate and an overburdened legal system. AI can be a hugely pertinent gamechanger for global governance systems and help law enforcement officers improve surveillance by augmenting police efforts, automate a variety of routinized legal tasks and improve transparency in governance. By bringing the potential of AI in law enforcement, we can offer:
- Surveillance and identification of wrongdoers; areas recognized for high criminal activity can be done through computer vision
- Review and summary-creation of long drawn cases and their history can be done through natural language processing and voice recognition
- Routing Right-to-Information and governance-related citizen requests through intelligent bots, thus making it more efficient to get critical information
- Employ Anomaly Detection frameworks to surface fraudulent transactions – especially among land deals.
A global population of over 7.7 billion people, distributed across a huge landmass throws up a sizeable challenge when it comes to scalability. With many individual nations crippled by the inability to serve their populations, their public services need technology-centric solutions that are scalable and intelligent at the same time. Artificial intelligence will effectively address a number of these problems which are of socio-economic importance, and will go a long way in improving the quality of life of humanity at large. To enable this, public services need to act sooner rather than later and ramp up their data sets, identify and onboard technology, innovation and research partners for ideating and applying AI techniques that can power humanity’s next big leap.
Related Posts
AIQRATIONS
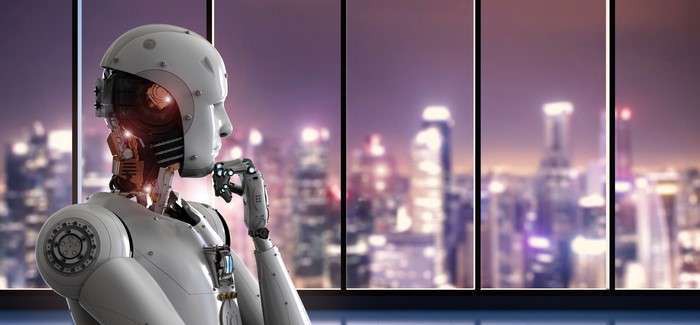
Better business with AI
Add Your Heading Text Here
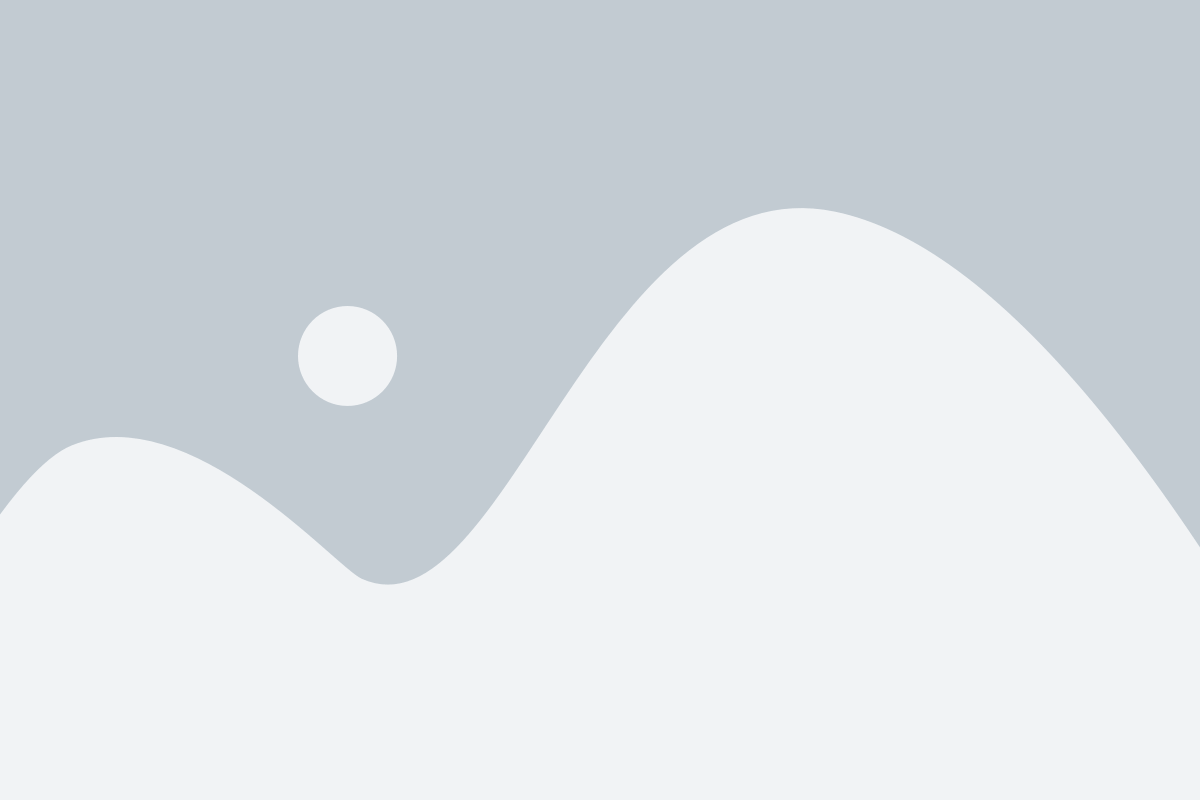
The Artificial Intelligence revolution in the enterprise is well under way. According to Gartner’s 2018 CEO and Senior Business Executive Survey, 65% of respondents think that AI will have a ‘material impact on an area of their business’. Due to the combination of three critical factors – improved data availability and machine learning techniques, increased computing power and storage, and a strong enterprise thrust on data-driven decision-making – AI has taken a strong foothold in some of the largest corporations in the world today, commanding executive-level interest, attention and urgency.
Beyond simple automation, AI is powering complex, critical decisions in several areas from Renaissance Technologies’ Medallion Fund, which uses statistical probabilities and quantitative models and has become one of the startling successes in the hedge fund industry, to complex image annotation and deep learning that helps radiologists detect cancer in MRI scans. Here is a look at some of the critical areas where AI is augmenting human decision-making:
Healthy Healthcare
As multiple countries grapple problems from an ageing population, rising healthcare costs and low doctor-to-patient ratios, AI can help improve healthcare outcomes in a variety of ways. For instance, AI is being leveraged for public health studies – from detection of potential physical or psychological pandemics to epidemiology – by mining social media and other data sources.
Further, startups and conglomerates are working on AI for diagnostics – from detection of early warning signals to identifying and quantifying abnormalities/tumours. In the pharma industry, AI is helping improve site studies, drug development and clinical trials through analysis of meaningful data.
Financial Services
A common AI use case for financial services is in the domain of fraud detection and anti-money laundering. AI can help surface bad actors by quickly scanning data for anomalous behaviour. Similarly, AI is also powering customer interaction decisions through intelligent chatbots that can address common concerns, thus reducing the need for human intervention in repetitive, menial tasks. We’re also seeing increased proliferation of robo-advisers – which are advanced AI tools that help make investment decisions by matching investible capital and returns expected.
Managing Media
The media and entertainment industry is going through an AI and digital disruption due to the combination of huge datasets and success of torchbearers like Netflix and Spotify. Content recommendation and personalisation are decisions that are autonomously delivered by AI, which can quickly scan a user’s history and match it with the preferences of similar users.
The industry is also relying on AI to make decisions around content creation, again taking a leaf out of Netflix to make content more engaging and sticky. There is also a strong use case of AI helping identify and attract customers by surfacing tailored content and promotions to increase subscriptions, loyalty and share-of-wallet.
Retail Rejig
Retail was one of the first industries to witness the rise of a data-powered competitor that eventually decimated incumbents. The brick-and-mortar retail industry is now incorporating AI in its decision-making process to replicate the customer experience expectations set by Amazon and the like.
Retailers leverage user purchase to identify next-best product and create tailored loyalty programmes. It is also being increasingly used for rapid experimentation to define store location, layouts and product-shelf decisions. Retailers can better anticipate demand, leading to leaner supply chains and warehouses, optimised inventory and fewer stockouts.
Efficient Manufacturing
Manufacturing companies are bringing in AI interventions to run leaner supply chains to cut the cost of transportation and wastage. AI also enables them to better anticipate demand by looking at historical sales, current uptake and other business environment factors to run on-demand production.
Some AI-led decisions are pervasive across multiple industries. For instance, digital personalisation, ie, serving targeted promotions to customers based on their key purchase drivers, is a multi-industry example of AI in action.
The other is for detecting security threats through anomaly detection and video analytics to identify unauthorised entry. Human Resources is another function that is rapidly changing, with companies using AI to speed up talent acquisition by scanning resumes for relevancy and reducing attrition by identifying key drivers that lead to employees leaving.
Successful AI-led Decisions
The business value of AI is significantly lowered when performed ad hoc, without a strong foundational strategy. It is important that the organisation clearly defines the decisions that should be powered by AI to maintain a high standard of outcomes. The responses will differ from company to company and from industry to industry, but it is important that corporations establish transparent standards for fair use.
We see enough examples of hastily implemented AI, leading to calamitous consequences and companies can no longer hide by saying, ‘The AI made me do it’. To demarcate the clear go and no-go zones for AI, here’s a handy questionnaire to ask yourself:
– Do we have enough superior quality data now and in the future for AI to make the best decision?
– Do we need to bring in insights from multiple sources to contribute to the decision-making process at a speed and scale, which cannot be efficiently handled by human cognition?
– Is human decision-fatigue or bias currently creating a sub-optimal outcome in this area?
– Could there be ethical or moral implications to an AI-led decision that might lead to disastrous consequences?
We also need to address the confidence issues. For instance, a lot of executives look down upon some of the black-box processes performed by AI algorithms. We need to find a way to address these issues by creating a transparent trail of AI decisions and the reasons why AI took a decision. Even in unsupervised learning scenarios, a trail of decisions will not only boost confidence but will also help build better AI and better businesses.
Re-imagined AI-powered decisions will become de rigueur only by the quality of the outcomes they deliver. According to Dr John Kelly, SVP — IBM Research and Solutions portfolio, “The success of cognitive computing will not be measured by Turing tests or a computer’s ability to mimic humans. It will be measured in more practical ways, like return on investment, new market opportunities, diseases cured and lives saved.” This is a crucial way to look at and measure the impact of AI on our businesses, society and lives.
Related Posts
AIQRATIONS
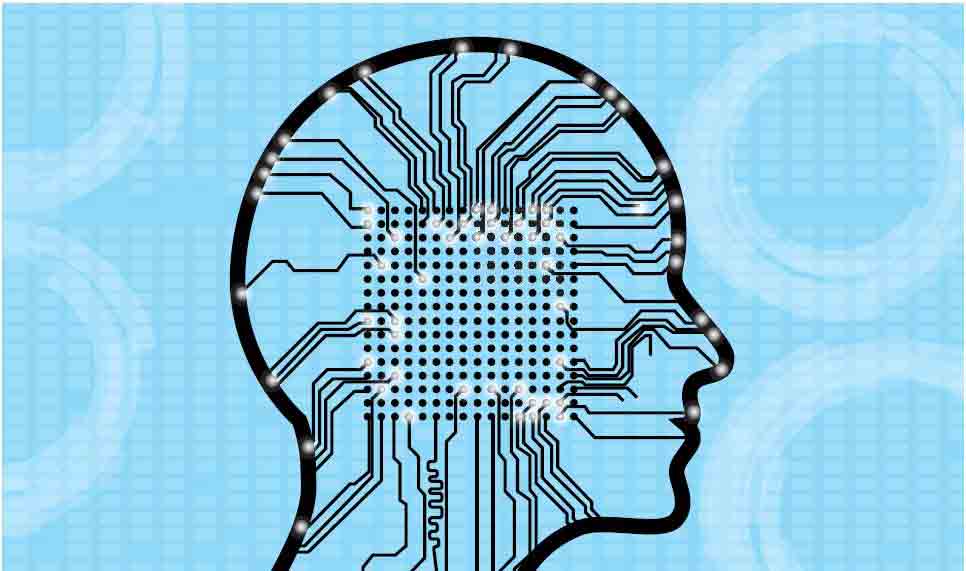
Detecting depression early with AI
Add Your Heading Text Here
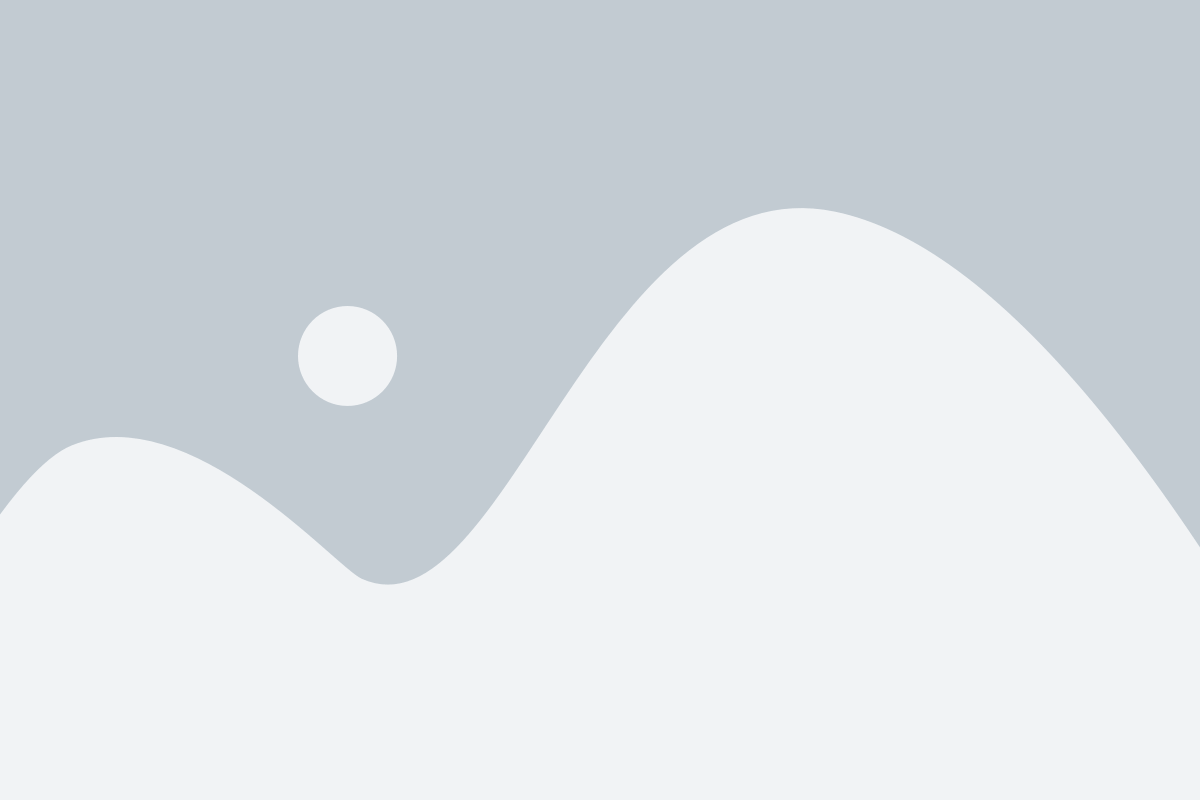
Can artificial intelligence be used in conjunction with mental health practitioners to better discover and address cases of depression and other mental illnesses? We have seen impactful examples of AI being applied to a range of health and wellness use cases, with the potential to help improve the lives of millions of people. Recent advances in this breakthrough technology have also shown the great promise that AI holds in helping individuals and doctors better manage cases and mitigate adverse health impacts of depression and other illnesses that plague the human mind.
Depression is a key problem faced by humans across the world — from milder forms of repetitive depressive mood states to more deeper-seated clinical depression. While we have seen significant improvements in our theoretical understanding of clinical depression, its causes and how to effectively mitigate it, the problem continues to affect millions of people. Psychiatric conditions such as this do not have vaccines or proven preventive medication. So much of the success in helping those with clinical depression hinges on early detection, continuous monitoring and scientific counselling and – in certain cases with a physician’s prescription – medication.
Health Data
At the macro-level, the downside of not managing clinical depression, especially for big populations, can be devastating. According to a study in 2015, it is estimated that approximately 216 million individuals across the globe (3% of total global population) have been affected with clinical depression – with a usual onset in the 20s and 30s. A lack of concerted focus on overall mental health and well-being can lead to outcomes as diverse as high suicide and crime rates to a reduction in national productivity and high levels of substance abuse and addiction.
Today we have a huge repository of health data. By applying machine learning and deep learning algorithms, AI can help individuals and physicians detect early indications of the onset of depression, monitor the progress of patients using medical and other therapeutic options and provide continuous support.
Early Detection
By using AI to understand and highlight speech patterns, use of specific words in text communication and even facial expression, we could possibly improve the speed at which such cases are uncovered. For achieving this, it is important that doctors and machine learning experts work together to identify patterns that have a strong correlation with clinical depression.
A research led by Dr Fei-Fei Li of Stanford University found that using facial and speech recognition data and algorithmic models, it might be possible to detect cases where there is an onset of clinical depression. By leveraging a combination of facial expressions, voice intonation and spoken words, their research was able to diagnose if an individual was suffering from depression with 80% accuracy.
Facebook too has begun piloting its own machine learning algorithm to go through posts to identify linguistic red flags that are indicative of depression. In early testing, the algorithm was said to perform just as well as existing questionnaires that are used to identify depression.
Therapist Interaction
Can AI inform therapists and help them do their job better? With the high and growing number of mental illnesses, the limited number of therapists need AI interventions to help make their job easier while helping improve health outcomes. AI could change the game in the therapy arena by augmenting therapists in identifying subtle signs showcased by patients – such as intonations in voice and facial expressions – which therapists might sometimes miss. Further, AI can also provide guided suggestions to them in providing effective treatment options.
An interesting innovation in this area comes from Ginger. This technology company combines a strong clinician network with machine learning to provide patients with the emotional support that they need at the right time – offering 24/7 availability for cognitive behaviour therapy (CBT), alongside mindfulness and resilience training.
On the back-end, AI is using each patient’s progress to inform its capabilities and make it smarter and more scalable. AI also helps Ginger match its users with a team of three emotional support coaches that it determines to be best suited for the individual.
Continuous Support
The stigma associated with mental illnesses often acts as a bottleneck in uncovering which individuals are suffering from it. Having a non-human companion that can provide human-like compassion can be a possible game changer in helping counsel patients suffering from depression and help them in the rehabilitation process. There are also advantages associated with AI being that counsellor – as it offers higher availability at a much lower cost.
Woebot is an excellent example and a bellwether for what AI could accomplish in the future for this use case. Created by Dr Alison Darcy, Woebot is a computer program that can be integrated with Facebook and is aimed at replicating the typical conversation a patient would have with a therapist. The tool asks about a patient’s mood and thoughts and offers evidence-based CBT. Just like a therapist would in a real-life situation, Woebot ‘listens’ actively in a conversation and learns about the patient to offer interactions more tailored to an individual’s unique situation.
Last year, researchers from the Massachusetts Institute of Technology presented a paper detailing a neural-network model that can be run over raw text and audio data to discover speech patterns that could be indicative of depression, without the need for a pre-set questionnaire. This immense breakthrough will hopefully ripple into more similar research, which could help aid those who suffer from cognitive conditions such as dementia.
While the potential for AI in the field of mental health is massive, it is also imperative that we consider the aspect of protecting the privacy and identity of those included in studies pertaining to this domain. Maintaining data security norms is an extremely crucial part of any AI exercise and in this case, it is even more paramount. To protect the sanctity of AI interventions and participating patients providing the data, it is extremely important to address concerns around data and information security first, before addressing any future use cases in this technology.
Related Posts
AIQRATIONS
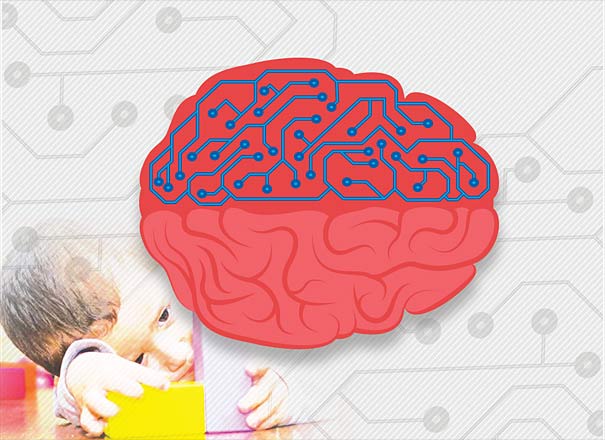
Autism care with AI
Add Your Heading Text Here
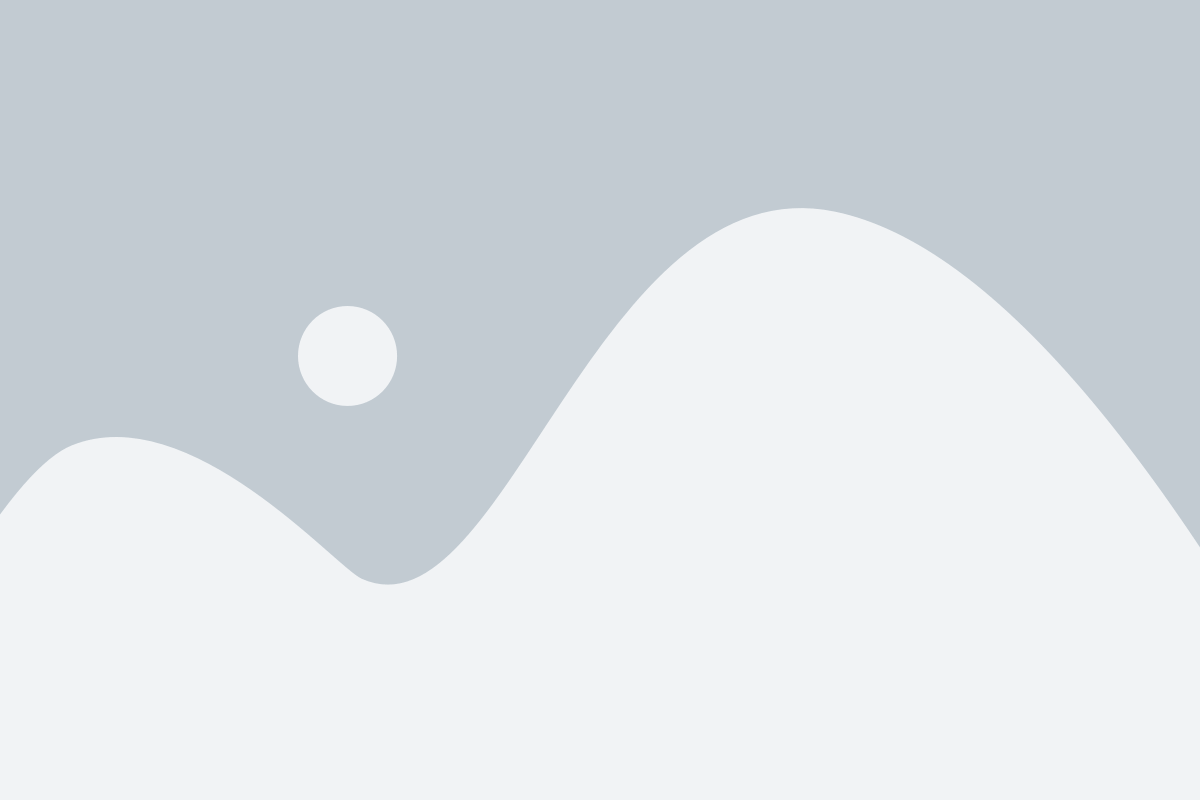
In these columns, I have earlier attempted to highlight the possibilities of multiple game-changing applications in the field of artificial intelligence (AI) that hold the potential to deliver positive benefits to humanity. AI can do much more beyond enabling a transformative business impact. Every technological advancement brings with it an opportunity to deliver positive changes to society. It is no different with AI. With massive increases in computing power and data deluge, it is possible for AI to be a harbinger of change for the society we live in.
A complex problem that we see is Autism Spectrum Disorder. As of 2015, this neurodevelopment disorder is estimated to have affected the lives of 24.8 million people globally. Worse, despite all the advances in medical sciences, there is no conclusive understanding of its causes and cures. While we do see cases where autism gets resolved, we are still unable to point concretely to any medical option that works better than others.
At best, autism can be managed – possibly through a mix of early diagnosis and continuous therapy. Thankfully, we live in an era where the awareness of autism is on the rise and the associated stigma is declining. By harnessing the power of AI to detect and manage cases of autism, we could potentially help those suffering from it lead a fulfilling life of dignity and respect.
While not conclusively proven, it is likely that an early diagnosis of autism is hugely beneficial for managing it in the future. An early detection would also help the people around these children be better attuned to their condition and enable them to set an environment that is conducive to their development.
One such application is applying AI to analyse vocal and behavioural cues exhibited by children. Stephen Sheinkopf — an autism researcher and psychologist at the Brown University Center for the Study of Children at Risk — believes anomalous crying patterns of babies might serve as an early warning sign of autism.
Neurological cues present in the acoustic features of cries — pitch, energy and resonance — might hold the key to early detection. Combining vocal, behavioural and physiological data, we might be able to piece together a pathway to early detection. That’s where AI and machine learning can add really great value, in integrating these disparate pieces of information that might otherwise be hard to make sense of.
An excellent example of this in action is Chatterbaby — conceptualised and developed by Ariana Anderson, a computational neuropsychologist at UCLA. Earlier, functionality enabled identification of potential causes for why a baby is crying by monitoring crying patterns. In addition, Chatterbaby is also attempting to identify if there are discernible differences in the crying patterns of autistic children and neurotypical ones — ultimately aiming to isolate the characteristics of each group to detect autism early.
Early ‘Testing’
Researchers in the UK and Italy have turned to AI for developing what might be the world’s first ‘test’ for autism. In their study, they leveraged AI to compare the protein levels in the blood samples of two groups of children — one group comprising 38 children diagnosed with autism, and the other with 31 children without the diagnosis. Their findings helped develop an algorithm that could test autism — with a 90% accuracy for which children have autistic spectrum disorder and an 87% accuracy for which children do not have it.
Another example is through tracking changes in brain function of six-month-old babies, which researchers from UNC Chapel Hill and Washington University’s School of Medicine believe can help early detection. They recently published a paper wherein they examined the brain scans of 59 high-risk babies to understand the connections and interactions between different regions of the brain. Post this, they analysed the brain scans of the 11 babies that were eventually diagnosed with autism.
By combining the data with AI and deep learning, they developed an algorithm able to detect the possibility of autism with an accuracy of 9 out of 11.
Chatbots and Virtual Assistants
Across studies, we see children with ASD have high levels of comfort with computers due to their predictable and logical nature. Autistic children can perceive humans to be emotional and unpredictable but computer-based systems (even those with human expression) to be rational and non-judgmental.
Systems such as chatbots and social robots can help capture and track the progress of autistic children, continuously monitor their social behaviours and make quick, informal assessments in school and at home. The big promise of these systems is allowing autistic children the opportunity to navigate social interactions, unfamiliar environments while aiding them to reach the developmental goals usually set for neurotypical children.
An example of this is an app called Companion, featuring a virtual assistant named Abby. Abby helps identify interests and needs of autistic children and provides support throughout the day. Beyond this app, Identifier identifies talents of autistic children through interactive game-play; eventual results are compiled onto a dashboard detailing the skills and shortcomings of the child.
A Strict No-No
While AI can bring these benefits, it is also important to ensure that these systems are designed with empathy for the autistic population. For instance, the use of bright, jarring colours is an absolute no-no for the design of such applications. Secondly, virtual agents should be enabled to speak in simple language, without the use of idioms, euphemisms or figures of speech to help such people understand instructions much better.
For a problem that has no apparent cause or cure, AI could be a breakthrough in improving the quality of life of those who suffer from autism and the people around them. By using AI for early diagnosis, disease management and people enablement, we would be able to help bring dignity to the lives of affected people.
Related Posts
AIQRATIONS
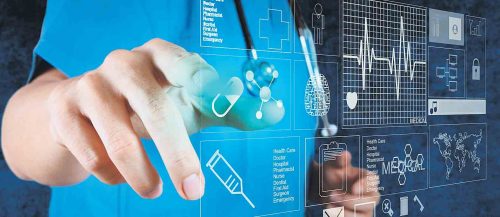
Get AI to Solve Systemic Problems
Add Your Heading Text Here
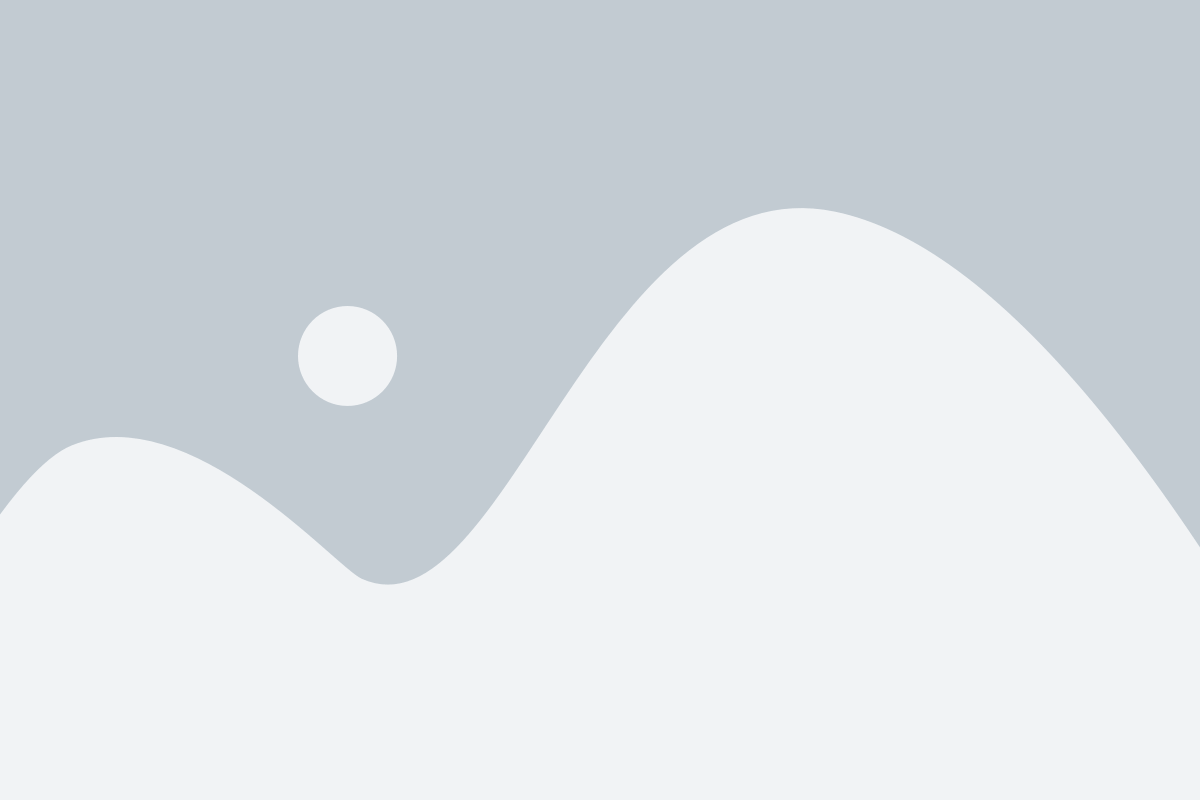
It is critical that public services ramp up their data sets, identify partners for ideation and leverage technology
For all its growth and development since independence, India faces many systemic problems. From our complex and labyrinthine legal system to the inefficiencies in our agricultural sector, large-scale problems still abound.
We need to better connect our burgeoning population with basic facilities. While Artificial Intelligence may not be the panacea in itself, we need to harness its potential to improve living conditions. Thankfully, we have the intellectual capital – our information technology peers – that can bring substantial dividend in this arena. By combining our inherent technological prowess and the keenness of our government in promoting technology-led interventions, AI can truly be a game-changer for India. Here’s an India-specific perspective on how AI can be a force for good for our country.
Agriculture Sector
Though the agricultural sector sees piecemeal improvements, numerous problems go unresolved – from low yield, low predictability of yield, poor access to institutional credit and financing to lack of transparency around pricing for produce. Using AI, agriculture can be transformed by:
• Provision of on-demand information on quality of seeds, fertilizers, pesticides and the track record of providers and opportunities for mechanisation through better equipment. This can be done through bot-enabled ‘Kisan Helplines’ that can provide guided advice for improving productivity
• Improving predictability of yield by ingesting data on soil health, equipment quality, farmer activity and weather conditions
• Improving visibility of market price trends for crops produced (domestic and international) so that they can make informed decisions on pricing, while exploring going to market without intermediary interference
• Leveraging data from productivity, yield and forecasts and potential prices to assess creditworthiness of individual farmers. This will speed up disbursement of finance and ensure farmers get better rates for crop insurance
Smart Cities
Indian cities have grown in an extremely unplanned manner, with public infrastructure and services struggling to catch up. Consider this – the cost of traffic congestion alone in just four major cities is estimated to be $22 billion annually. With AI, urban planners can:
• Track movement of traffic and people to identify opportunities for ‘decentralising’ major hubs and develop future-ready public infrastructure to facilitate smoother movement of people, vehicles and goods
• Model population density and availability of sanitation facilities to improve access. Additionally, by applying image analytics on drone surveilled images can help determine quality of sanitation facilities and accelerating their upkeep
• Identify and improve access to current and emergent residential and commercial hubs by creating more optimal public transport networks
• Align consumption of resources – energy, water, cooking gas – to actual needs
• Crowdsource, store and take action to improve infrastructure by directly soliciting participation from citizens
• Improve planning and forecasting for infrastructure development through better coordination between public works departments, leveraging traffic data and streamlining supply chains
Education System
The education system in India is among the most outdated and unequitable when compared with the developed world. Problems abound from a prominent level of student dropouts, to quality and methodology of teaching, lack of workforce readiness among students and outdated curricula. Here’s how AI can help improve certain facets:
• Track the demand for skills in the market and the educational infrastructure available to supply those skills through a National Skills Repository. This will help keep education concurrent with current market demands
• Automate routine, time-consuming tasks – from creating and grading test papers, developing personalised benchmarks for each student, identifying gaps in student development, tracking aptitude and attentiveness within each subject – and enabling teachers to focus on curriculum development, coaching and mentoring and improving behavioural and personality aspects of students
• Identify potential dropouts and root-causes, enabling educational institutions to take proactive steps to ensure student retention and course completion
Healthcare
The doctor-to-patient ratio in India is quite poor – with 0.62 doctors available per 1,000 people (WHO recommends a ratio of 1:1,000). When you add to that the inadequate spread of doctors across the country, we have a poorly served population, ranking 125th in the world for life expectancy. Using AI, we can:
• Identify areas with a high population density, which are underserved by public hospitals. Further, improve the deployment and availability of doctors, medical equipment and medication to better serve the population
• Track patient histories and clinical notes to prescribe evidence-based treatment
• Speed up routine processes such as scanning X-rays and CT-scans for malignancies using image analytics
• Improve public health studies by identifying early warning signals through alternative methods such as social media tracking
• Identify individuals without health insurance and incentivise their usage to improve patient medical adherence
Legal Challenges
When adjusted for VIP protection, India claims an extremely poor police-to-people ratio with 1 police for every 663 people. There are 27 million cases pending with courts, of which six million have been pending for over five years. AI can be a crucial enabler for our crumbling governance system and can help:
• Speed up review and summary writing of long drawn cases and their
history using natural language processing and voice recognition
• Use image analytics for surveillance and identification of wrong-doers in areas recognised for high criminal activity
• Surface fraudulent deals – especially among land deals – using anomaly detection frameworks to speed up delivery of justice
• Improve public services and transparency by routing RTI requests through intelligent bots, thus making it more efficient to get critical information
With a population of over 1.3 billion people, distributed across a huge landmass, public services urgently need technology-centric solutions that are both intelligent and scalable. AI will effectively address a number of these problems. To this end, it is critical that public services act sooner than later and ramp up their data sets, identify technology partners for ideation and apply AI techniques to power the India’s next leap forward.
Related Posts
AIQRATIONS
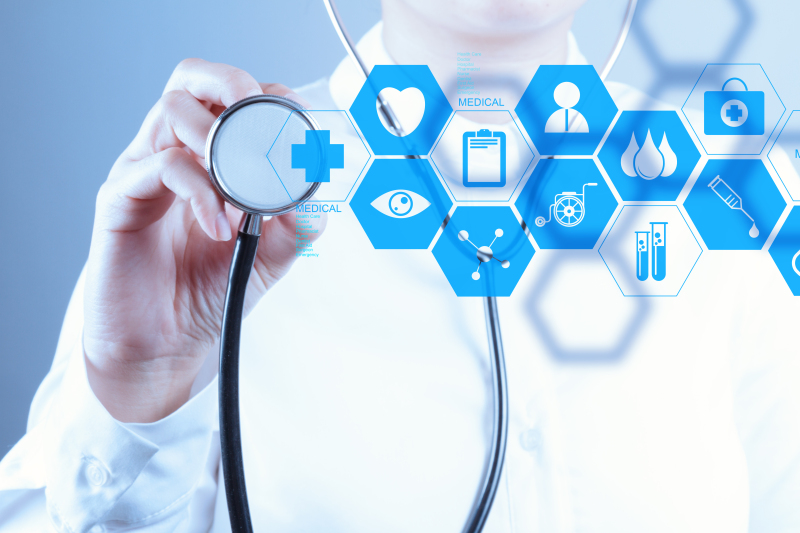
How Healthcare Industry will Benefit by Embracing Data Sciences
Add Your Heading Text Here
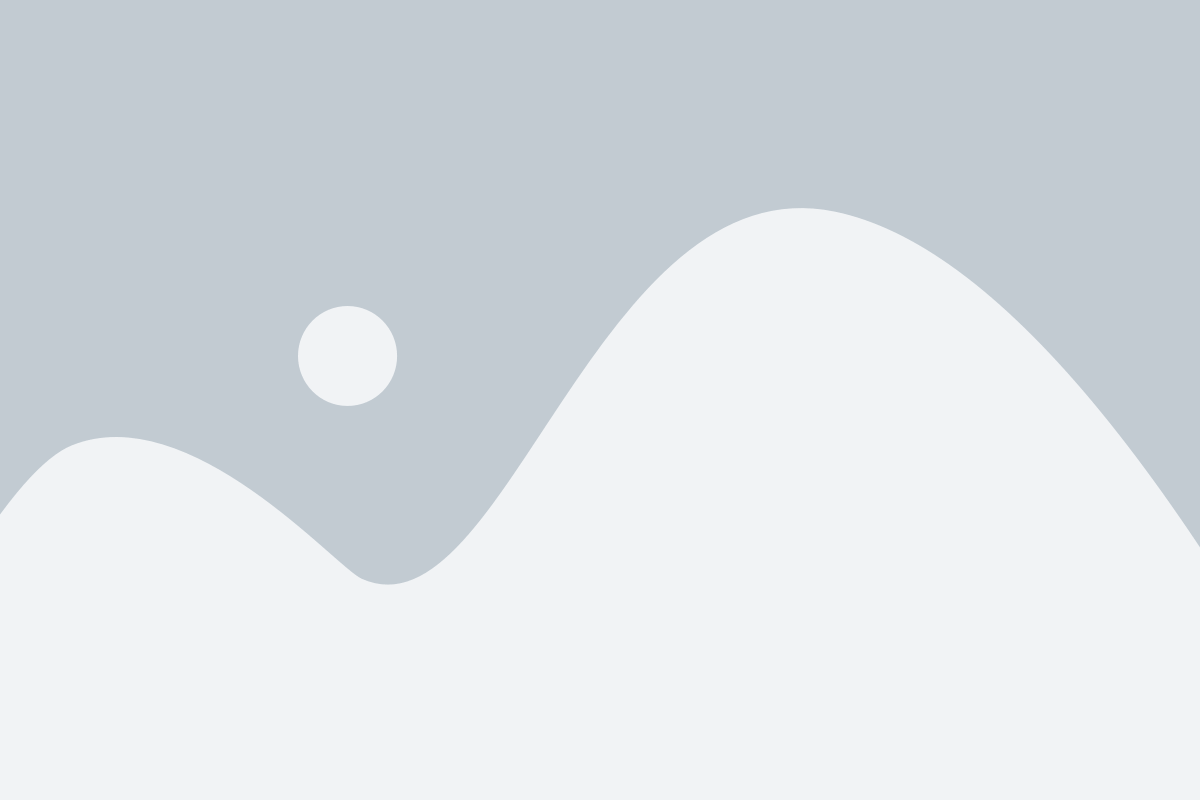
In the healthcare industry, what could be more important than having better healthcare outcomes? Each and every day healthcare workers around the globe are striving hard to find more ways of improving our lives. However, the world is changing, and frankly, at a faster rate than most of us can keep up. Intuition alone will no longer be enough for quality patient outcomes. The amount of healthcare data continues to mound every second, making it harder and harder to find any form of helpful information. Big Data is not to be romanticized; it can be a blessing and a curse. It can contribute to both the insight and the fog of visibility.
In truth, data science is proving invaluable to improving outcomes due to its ability to automate so much of the heavy lifting – in fast, scalable, and precise ways. All one has to do is look at our ability to predict epidemics, advance cures, and make patient stays in hospitals safer and more pleasant. In healthcare, data science should be seen as a beneficial intelligence rather than only artificial intelligence, providing an augmentation of services to the healthcare experts already in play.
Hospital Claims Data
In 2010, there were 35.1 million discharges with an average length of stay of 4.8 days according to the National Hospital Discharge Survey. That same survey went on to note that there were 51.4 million procedures performed. The National Hospital Ambulatory Medical Care Survey in 2011 stated the number of outpatient department visits were 125.7 million with 136.3 million emergency department visits. These are some of the basic figures showing the amount of care the U.S. health care system has provided. Using Data Science to annualize this sort of data allows healthcare providers to start building a new intuition built on a data narrative that could possibly help avoid the spread of diseases or address specific health threats. Using a combination of descriptive statistics, exploratory data analysis, and predictive analytics, it becomes relatively easy to identify the most cost-effective treatments for specific ailments and allows for a process to help reduce the number of duplicate or unnecessary treatments. The power in predicting a future state is in using that knowledge to change the behavior patterns of today.
Electronic Health Record (EHR)
Interoperable electronic health records (EHRs) for patient care hold tremendous potential to reduce the growth in costs. EHRs can help healthcare organizations improve chronic disease management, increase operating efficiencies, transform their finances, and improve patient outcomes. However, EHR implementations are in various stages of maturity across the country, and their benefits have not been fully realized. One of the primary challenges healthcare decision-makers face is how to make meaningful use of the data collected, available, and accessible in EHRs.
By optimizing the use of data accessible in EHRs, we can uncover hidden relationships and identify patterns and trends in this diverse and complex information to improve chronic disease management, increase operating efficiencies, and transform healthcare organizations’ finances.
Patient Behavior and Sentiment Data
A study by AMI Research suggests that “wearables” are expected to reach $52 million by 2019. Wearables monitor heart rates, sleep patterns, walking, and much more while providing new dimensions of context, geolocation, behavioral pattern, and biometrics. Combine this with the unstructured “lifestyle” data that comes across social media and you have a potent combination that is more than just numbers and tweets.
It is obvious that we will experience huge benefits from analyzing the in’s and out’s of healthcare data. In my judgment, we will continue to see a push for prevention over cure which puts predicting outcomes front and center. After all, catching things in the earlier stages is easier to treat and outbreaks can be more easily contained.
It may not resonate as widely today, but in the future we will look back on data science as something significant for healthcare. It is reasonable to expect that we will likely recover more quickly from illness and injury, live longer because of newly discovered drugs, and benefit from more efficient hospital surgeries – and in large part this will be because of how we analyze Big Data.
What makes living in the era of Big Data such a delight is that the healthcare industry is being pressed to find better tools, skills, and techniques to deal competently with the deluge of patient data and its inherent insights? When healthcare makes the choice to fully embrace data science, it will change the future for everyone.
Genomics
Inexpensive DNA sequencing and next-generation genomic technologies are changing the way healthcare providers do business. We now have the ability to map entire DNA sequences and measure tens of thousands of blood components to assess health.
Next-generation genomic technologies allow data scientists to drastically increase the amount of genomic data collected on large study populations. When combined with new informatics approaches that integrate many kinds of data with genomic data in Healthcare applications such as disease research, Prescription Effectiveness etc, we will better understand the genetic bases of drug response and disease. Researchers aim to achieve ultra-personalized healthcare. As a beginning, the FDA has already begun to issue medicine labels that specify different dosages for patients with particular genetic variants.
Predictive Analytics and Preventive Measures
Prevention is always better than cure. For the health-care industry, it also happens to save a lot of money. (The Centers for Medicaid and Medicare Services, for instance, can penalize hospitals that exceed average rates of readmission – indicating that they could be doing more to prevent medical problems.)
Take, for example, the partnership between Mount Sinai Medical Center and former Facebook guru Jeff Hammerbach. Mount Sinai’s problem was how to reduce readmission rates. Hammerbach’s solution was predictive analytics:
- In a pilot study, Hammerbach and his team combined data on disease, past hospital visits and other factors to determine a patient’s risk of readmission.
- These high-risk patients would then receive regular communication from hospital staff to help them avoid getting sick again.
Sinai isn’t alone. In 2008, Texas Health partnered with Healthways to merge and analyze clinical and insurance claims information. Their goal was the same – identify high-risk patients and offer them customized interventions.
Meanwhile, in 2013, data scientists at Methodist Health System are looking at accountable-care organization claims from 14,000 Medicare beneficiaries and 6,000 employees. Their aim? You guessed it. Predict which patients will need high-cost care in the future.
Patient Monitoring and Home Devices
Doctors can do a lot, but they can’t follow a patient around every minute of the day. Wearable body sensors – sensors tracking everything from heart rate to testosterone to body water – can.
Sensors are just one way in which medical technology is moving beyond the hospital bed. Home-use, medical monitoring devices and mobile applications are cropping up daily. A scanner to diagnose melanomas? A personal EEG heart monitor? No problem.
These gadgets are designed to help the patient, naturally, but they’re also busy harvesting data.
For example:
- Asthmapolis’s GPS-enabled tracker, already available by 2011, records inhaler usage by asthmatics. This information is collated, analyzed and merged with data on asthma catalysts from the CDC (e.g., high pollen counts in New England) to help doctors learn how best to prevent attacks.
- With Ginger.io’s mobile application, out in 2012, patients consent to have data about their calls, texts, location and movements monitored. These are combined with data on behavioral health from the NIH and other sources to pinpoint potential problems. Too many late-night phone calls, for instance, might signal a higher risk of anxiety attack.
- To improve patient drug compliance, Eliza, a Boston-based company, monitors which types of reminders work on which types of people. Smarter targeting means more compliance.
The Challenges Ahead
There are plenty of hurdles to creating a data-driven health care industry. Some are technical, some emotional. Health care providers have had decades to accumulate paper records, inefficiencies and entrenched routines. A remedy will not be quick.
And some say it shouldn’t. At least, not without a hard look at patient privacy, data ownership and the overall direction of U.S. health care.