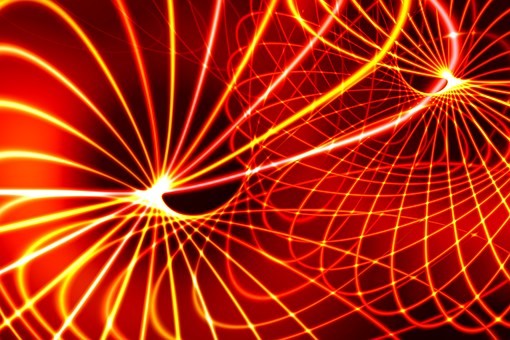
Experience the Algorithm Economy : Accentuating strategic value for the enterprises
Add Your Heading Text Here
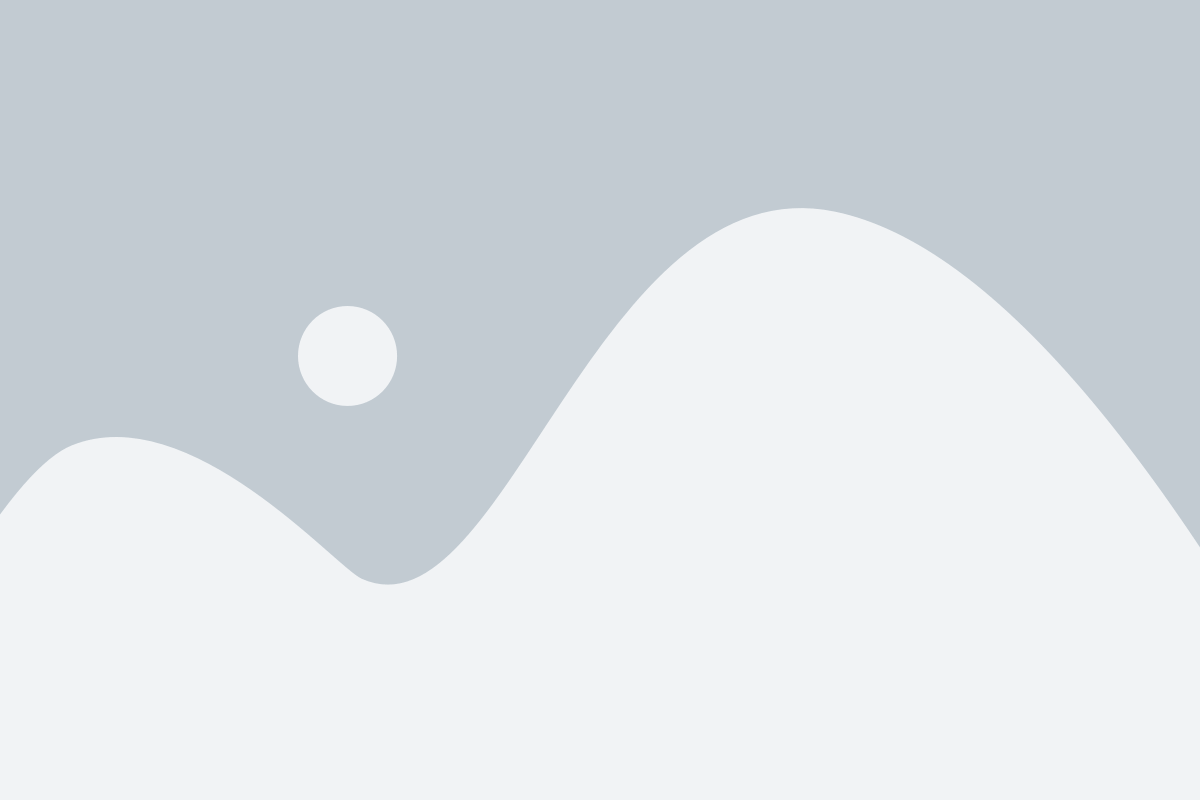
Algorithms will not only drive scores of business processes, but also build other self-intuitive algorithms, much as robots can build other robots. And rather than using apps, future users’ lives will revolve personalized algorithms to drive individual choices and behaviors .
Enterprises will license, trade, sell and even give away non-lynch pin algorithms and single-function software snippets that provide new opportunities for innovation by other enterprises. Enterprises will also partner with cloud-based, automated suppliers with the industry expertise to advice on ways to avoid future risk and adapt to technology trends.
Imaginative thinking ! but it’s no surprise that future value will come from increased density of interactions, relationships and sharing between people, businesses and things ̶ or what I call “ Algorithm Economy “ .The greater the maturity of algorithms , the greater potential value you can reap. We’ve seen interconnection coming of age for a while now and have invested heavily in a platform to empower enterprises with fast, direct and secure interconnections with business partners and network and cloud service providers.
Redefining Business Architecture with Algorithms
The term “algorithm economy” is relatively new, but the practical use of algorithms is already well established in many industries. In my opinion , CXOs must begin designing their algorithmic business models, both to capitalize on their potential for business differentiation, and to mitigate the possible risks involved.
Established businesses need to adopt a “bi modal strategy” and build what I called an algorithmic platform, completely separate from legacy systems, that harnesses repository of algorithms, interconnections, the cloud and the Internet of Things (IoT) to innovate, share value, increase revenues and manage risk.
New platforms based on this bimodal model should be far simpler, more cloud-based and more flexible than in the past, with the ability to add and remove capabilities “like Velcro” to support new short- and long-term projects. At the same time, IT should start divesting itself of older systems and functions that are outliving their usefulness or could be better done by other methods. The significant development and growth of smart machines is a major factor in the way algorithms have emerged from the shadows, and become more easily accessible to every organization. We can already see their impact in today’s world, but there is much work ahead to harness the opportunities and manage the challenges of algorithmic business.
CXOs should examine how algorithms and intelligent machines are already used by competitors and even other enterprises to determine if there is relevance to their own needs. The retail sector has long been at the leading edge of using smart algorithms to improve business outcomes. Today, many retail analysts believe that the algorithms that automate pricing and merchandising may soon become the most valuable asset that a retailer can possess. In HR function, algorithms are already transforming talent acquisition as they are able to rapidly evaluate the suitability of candidates for specific roles, but the same technology could easily be applied within an enterprise to allocate workloads to the right talent. In healthcare, the open availability of advanced clinical algorithms is transforming the efficiency of healthcare delivery organizations and their ability to deliver care. The practice of sharing and co-developing algorithms between enterprises with mutual interests could be relevant to most enterprises.
The Challenges of Algorithm Economy
The advances and benefits of algorithm economy will come hand in hand with obstacles to navigate. Whether the problems are anticipated or unexpected, as quantum computing becomes more pervasive, the implications have the potential to make or break organizations. For example, an extreme point of view is that any beneficial effects of algorithms on humanity may be nullified by algorithmically driven systems that are antithetical to human interests. Or, while an algorithmic business model may be deployed with good intentions, it could be manipulated by malicious humans to achieve undesirable outcomes. Undesirable, at least, from the point of the view of the person or organization that owns or controls the algorithm. Algorithms rely on the data they are fed, and their decisions are only as good as the data they are based on. Moreover, tricky ethical problems that do not necessarily have a “correct” answer will be inevitable, as a greater complexity of decision making is left in the hands of automated systems.
The scale of change that is made possible by smart machines and algorithm economy warrants considerable planning and testing. Enterprises that fail to prepare risk being left behind or facing unexpected outcomes with negative implications.
The Transformation required in Algorithm Economy
Making sense of all the data about how customers behave, and what connected things tell an organization, will require algorithms to define business processes and create a differentiated customer experience. Algorithms will evaluate suppliers, define how our cars operate, and even determine the right mix of drugs for a patient. In the purely digital world, agents will act independently based on our algorithms, in the cloud. In the 2020s, we’ll move away from using apps to rely on virtual assistants – basically, algorithms in the cloud – to guide us through our daily tasks. People will trust personal algorithms that thinks and acts for them. Take this to another level and the algorithms themselves will eventually become smart by learning from experience and producing results their creators never expected.
The Final Frontier
Therefore, we have to get the architecture of algorithms robust and steady to derive meaningful objectives. In essence, algorithms spot the business moments, meaningful connections, and predict ill behaviors and threats. CXOs need to be the strategic voice on the use of information, to build the right set of intelligent insights. Experience the Algorithm Economy and the ensuing strategic value for your enterprise . Are you geared up ?
Related Posts
AIQRATIONS

Lock in winning AI deals : Strategic recommendations for enterprises & GCCs
Add Your Heading Text Here
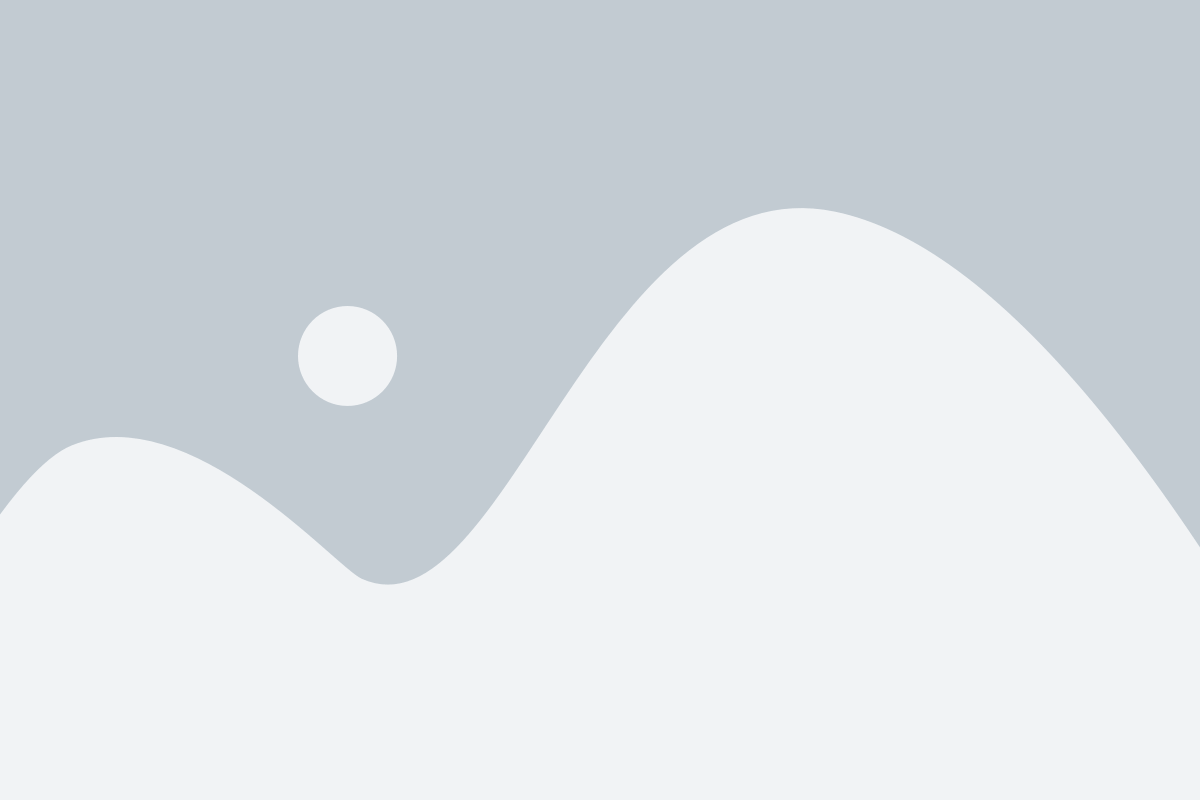
Artificial Intelligence is unleashing exciting growth opportunities for the enterprises & GCCs , at the same time , they also present challenges and complexities when sourcing, negotiating and enabling the AI deals . The hype surrounding this rapidly evolving space can make it seem as if AI providers hold the most power at the negotiation table. After all, the market is ripe with narratives from analysts stating that enterprises and GCCs failing to embrace and implement AI swiftly run the risk of losing their competitiveness. With pragmatic approach and acknowledgement of concerns and potential risks, it is possible to negotiate mutually beneficial contracts that are flexible, agile and most importantly, scalable. The following strategic choices will help you lock in winning AI deals :
Understand AI readiness & roadmap and use cases
It can be difficult to predict exactly where and how AI can be used in the future as it is constantly being developed, but creating a readiness roadmap and identifying your reckoner of potential use cases is a must. Enterprise and GCC readiness and roadmap will help guide your sourcing efforts for enterprises and GCCs , so you can find the provider best suited to your needs and able to scale with your business use cases. You must also clearly frame your targeted objectives both in your discussions with vendors as well as in the contract. This includes not only a stated performance objective for the AI , but also a definition of what would constitute failure and the legal consequences thereof.
Understand your service provider’s roadmap and ability to provide AI evolution to steady state
Once you begin discussions with AI vendors & providers, be sure to ask questions about how evolved their capabilities and offerings are and the complexity of data sets that were used to train their system along with the implementation use cases . These discussions can uncover potential business and security risks and help shape the questions the procurement and legal teams should address in the sourcing process. Understanding the service provider’s roadmap will also help you decide whether they will be able to grow and scale with you. Gaining insight into the service provider’s growth plans can uncover how they will benefit from your business and where they stand against their competitors. The cutthroat competition among AI rivals means that early adopter enterprises and GCCs that want to pilot or deploy AI@scale will see more capabilities available at ever-lower prices over time. Always mote that the AI service providers are benefiting significantly from the use cases you bring forward for trial as well as the vast amounts of data being processed in their platforms. These points should be leveraged to negotiate a better deal.
Identify business risk cycles & inherent bias
As with any implementation, it is important to assess the various risks involved. As technologies become increasingly interconnected, entry points for potential data breaches and risk of potential compliance claims from indirect use also increase. What security measures are in place to protect your data and prevent breaches? How will indirect use be measured and enforced from a compliance standpoint? Another risk AI is subject to is unintentional bias from developers and the data being used to train the technology. Unlike traditional systems built on specific logic rules, AI systems deal with statistical truths rather than literal truths. This can make it extremely difficult to prove with complete certainty that the system will work in all cases as expected.
Develop a sourcing and negotiation plan
Using what you gained in the first three steps, develop a sourcing and negotiation plan that focuses on transparency and clearly defined accountability. You should seek to build an agreement that aligns both your enterprise’s and service provider’s roadmaps and addresses data ownership and overall business and security related risks. For the development of AI , the transparency of the algorithm used for AI purposes is essential so that unintended bias can be addressed. Moreover, it is appropriate that these systems are subjected to extensive testing based on appropriate data sets as such systems need to be “trained” to gain equivalence to human decision making. Gaining upfront and ongoing visibility into how the systems will be trained and tested will help you hold the AI provider accountable for potential mishaps resulting from their own erroneous data and help ensure the technology is working as planned.
Develop a deep understanding of your data, IP, commercial aspects
Another major issue with AI is the intellectual property of the data integrated and generated by an AI product. For an artificial intelligence system to become effective, enterprises would likely have to supply an enormous quantity of data and invest considerable human and financial resources to guide its learning. Does the service provider of the artificial intelligence system acquire any rights to such data? Can it use what its artificial intelligence system learned in one company’s use case to benefit its other customers? In extreme cases, this could mean that the experience acquired by a system in one company could benefit its competitors. If AI is powering your business and product, or if you start to sell a product using AI insights, what commercial protections should you have in place?
In the end , do realize the enormous value of your data, participate in AI readiness, maturity workshops and immersion sessions and identification of new and practical AI use cases. All of this is hugely beneficial to the service provider’s success as well and will enable you to strategically source and win the right AI deal.
(AIQRATE advisory & consulting is a bespoke global AI advisory & consulting firm and provides strategic advisory services to boards, CXOs, senior leaders to curate , design building blocks of AI strategy , embed AI@scale interventions & create AI powered enterprises . Visit www.aiqrate.ai , reach out to us at consult@aiqrate.ai )
Related Posts
AIQRATIONS
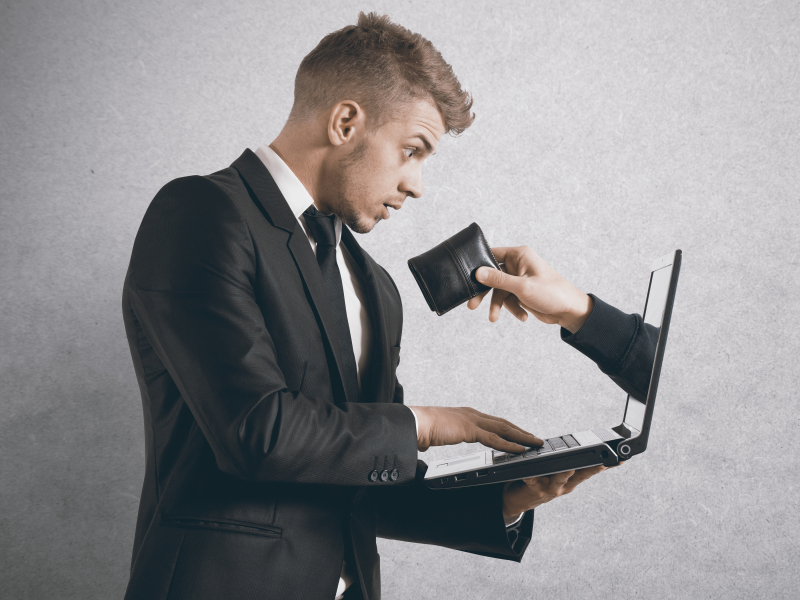
How AI is Enabling Mitigation of Fraud in the Banking, Insurance Enterprises
Add Your Heading Text Here
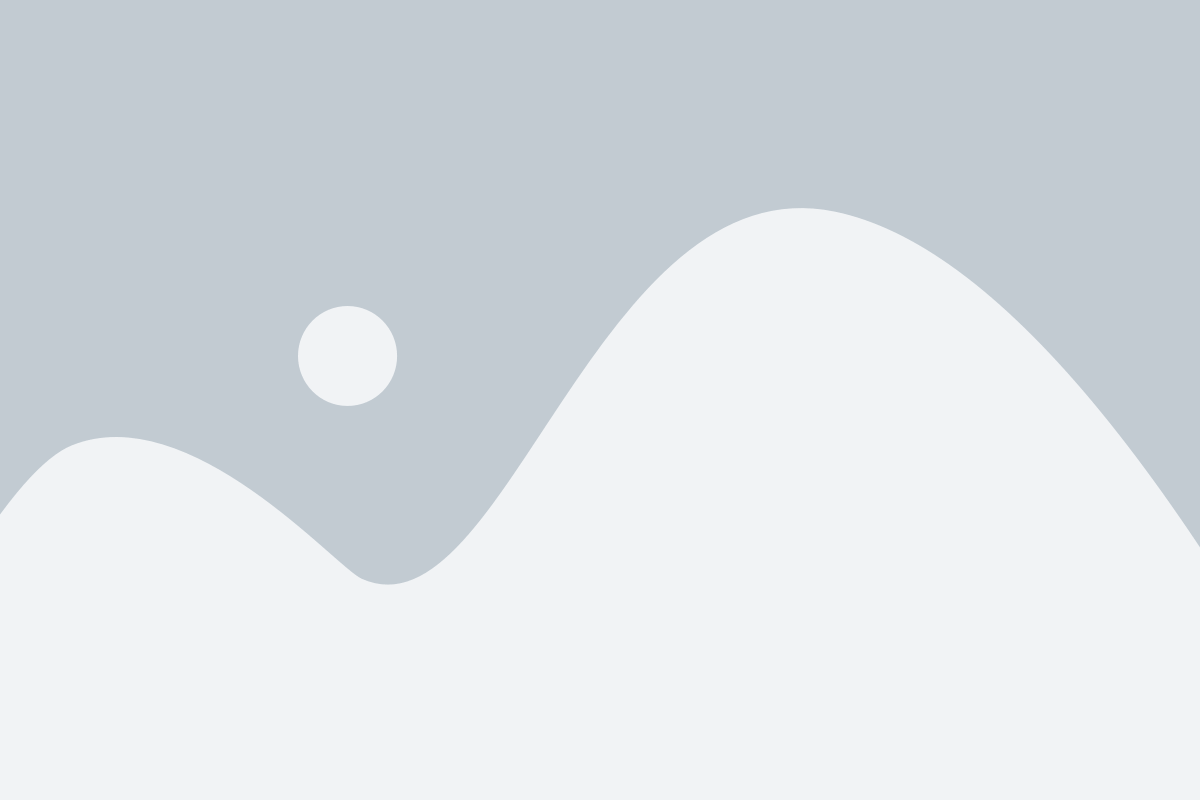
The Banking and Finance sector (BFSI) is witnessing one of its most interesting and enriching phases. Apart from the evident shift from traditional methods of banking and payments, technology has started playing a vital role in defining this change.
Mobile apps, plastic money, e-wallets and bots have aided the phenomenal swing from offline payments to online payments over the last two decades. Now, the use of Artificial Intelligence (AI) in BFSI is expediting the evolution of this industry.
But as the proliferation of digital continues, the number of ways one can commit fraud has also increased. Issuers, merchants, and acquirers of credit, debit, and prepaid general purpose and private label payment cards worldwide experienced gross fraud losses of US$11.27 billion in 2012, up 14.6% over the previous year1. Fraud losses on all general purpose and private label, signature and PIN payment cards reached US$5.33 billion in United States in the same period, up 14.5%1. These are truly big numbers, and present the single-biggest challenge to the trust reposed in banks by customers. Besides the risk of losing customers, direct financial impact for banks is also a significant factor.
Upon reporting of a fraudulent transaction by a customer, the bank is liable for the transaction cost, it has to refund merchant chargeback fee, as well as additional fee. Fraud also invites fines from regulatory authorities. The recently-passed Durbin Amendment caps processing fee that can be charged per transaction, and this increases the damage caused by unexpected fraud losses. The rapidly rising use of electronic payment modes has also increased the need for effective, efficient, and real-time methods to detect, deter, and prevent fraud.
Nuances of Banking Fraud Prevention Using AI
AI enables a computer to behave and take decisions like a human being. Coined in 1956 by John McCarthy at MIT, the term AI was little known to the layman and merely a subject of interest to academicians, researchers and technologists. However, over the past few years, it is more commonly seen in our everyday lives; in our smartphones, shopping experiences, hospitals, travel, etc.
Machine Learning, Deep Learning, NLP Platforms, Predictive APIs and Image and Speech Recognition are some core AI technologies used in BFSI today. Machine Learning recognises data patterns and highlights deviations in data observed. Data is analysed and then compared with existing data to look for patterns. This can help in fraud detection, prediction of spending patterns and subsequently, the development of new products.
Key Stroke Dynamics
Key Stroke Dynamics can be used for analysing transactions made by customers. They capture strokes when the key is pressed (dwell time) and released on a keyboard, along with vibration information.
As second factor authentication is mandatory for electronic payments, this can help detect fraud, especially if the user’s credentials are compromised. Deep Learning is a new area in Machine Learning research and consists of multiple linear and non-linear transformations. It is based on learning and improving representations of data. A common application of this can be found in the crypto-currency, Bitcoin.
Adaptive Learning
Adaptive Learning is another form of AI currently used by banks for fraud detection and mitigation. A model is created using existing rules or data in the bank’s system. Incremental learning algorithms are then used to update the models based on changes observed in the data patterns.
AI instances in Insurance for Fraud Prevention
Applying for Insurance
When a customer submits their application for insurance, there is an expectation that the potential policyholder provides honest and truthful information. However, some applicants choose to falsify information to manipulate the quote they receive.
To prevent this, insurers could use AI to analyse an applicant’s social media profiles and activity for confirmation that the information provided is not fraudulent. For example, in life insurance policies, social media pictures and posts may confirm whether an applicant is a smoker, is highly active, drinks a lot or is prone to taking risks. Similarly, social media may be able to indicate whether “fronting” (high-risk driver added as a named driver to a policy when they are in fact the main driver) is present in car insurance applications. This could be achieved by analysing posts to see if the named driver indicates that the car is solely used by them, or by assessing whether the various drivers on the policy live in a situation that would permit the declared sharing of the car.
Claims Management & Fraud Prevention
Insurance carriers can greatly benefit from the recent advances in artificial intelligence and machine learning. A lot of approaches have proven to be successful in solving problems of claims management and fraud detection. Claims management can be augmented using machine learning techniques in different stages of the claim handling process. By leveraging AI and handling massive amounts of data in a short time, insurers can automate much of the handling process, and for example fast-track certain claims, to reduce the overall processing time and in turn the handling costs while enhancing customer experience.
The algorithms can also reliably identify patterns in the data and thus help to recognize fraudulent claims in the process. With their self-learning abilities, AI systems can then adapt to new unseen cases and further improve the detection over time. Furthermore, machine learning models can automatically assess the severity of damages and predict the repair costs from historical data, sensors, and images.
Two companies tackling the management of claims are Shift Technology who offer a solution for claims management and fraud detection and RightIndem with the vision to eliminate friction on claims. Motionscloud offer a mobile solution for the claims handling process, including evidence collection and storage in various data formats, customer interaction and automatic cost estimation. ControlExpert handle claims for the auto insurance, with AI replacing specialized experts in the long-run. Cognotekt optimize business processes using artificial intelligence. Therefore the current business processes are analyzed to find the automation potentials. Applications include claims management, where processes are automated to speed up the circle time and for detecting patterns that would be otherwise invisible to the human eye, underwriting, and fraud detection, among others. AI techniques are potential game changers in the area of fraud. Fraudulent cases may be detected easier, sooner, more reliable and even in cases invisible to the human eye.
Conclusion
Those who wish to defraud insurance companies currently do so by finding ways to “beat” the system. For some uses of AI, fraudsters can simply modify their techniques to “beat” the AI system. In these circumstances, whilst AI creates an extra barrier to prevent and deter fraud, it does not eradicate the ability to commit insurance fraud. However, with other uses of AI, the software is able to create larger blockades through its use of “big data”. It can therefore provide more preventative assistance. As AI continues to develop, this assistance will become of greater use to the insurance industry in their fight against fraud.