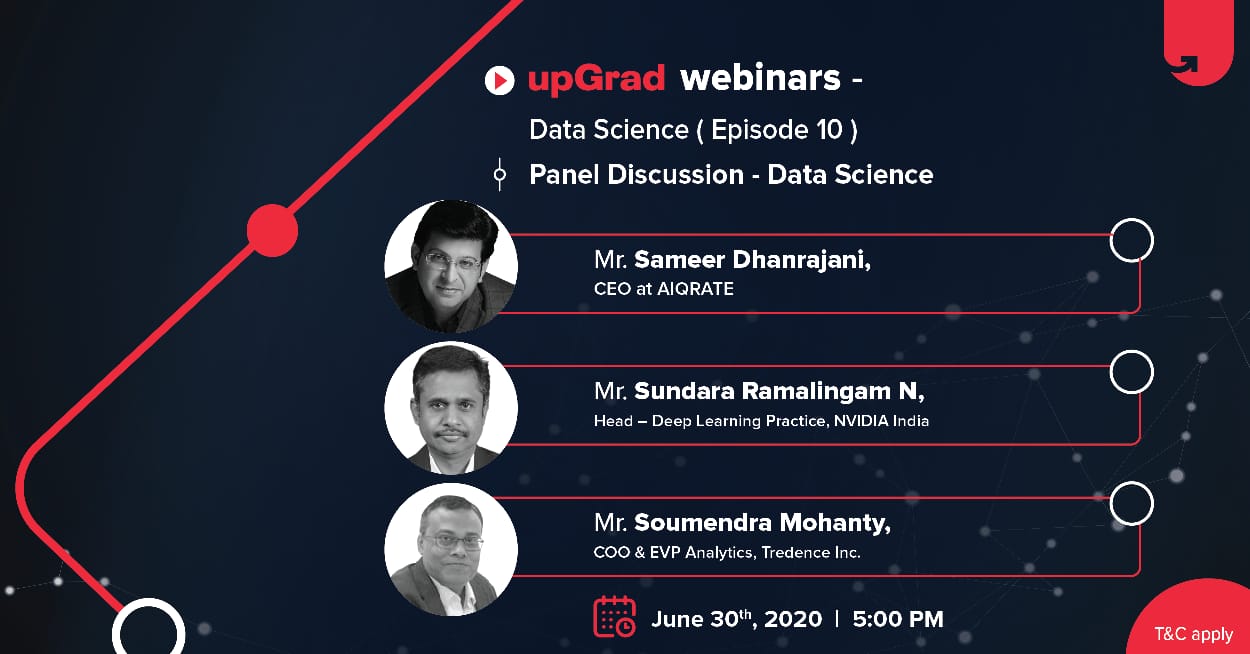
AIQRATE in upGrad’s Elite Panel Discussion on Data Science
Add Your Heading Text Here
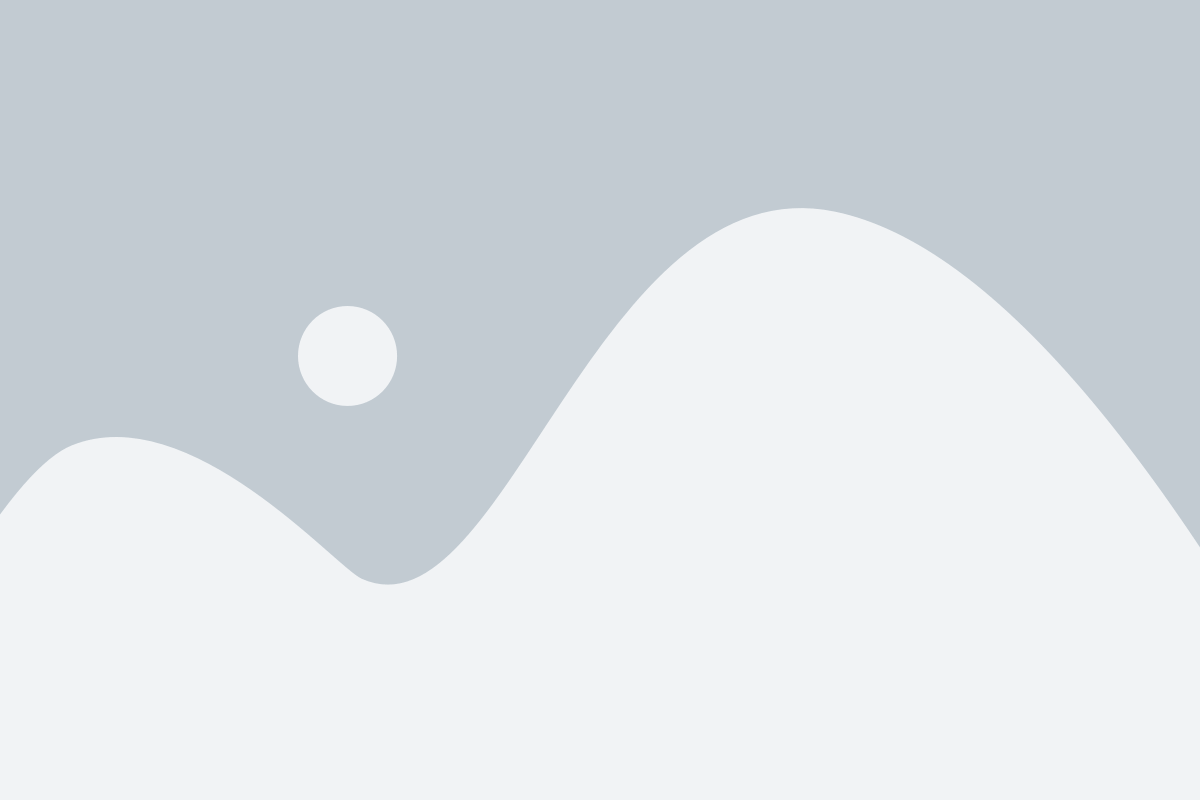
Panel Discussion Topic: Evolution of Data Science & AI
Tuesday, June 30th 2020
Panel: Sameer Dhanrajani, CEO & Co-founder, AIQRATE Advisory & Consulting, Sundara Ramalingam N, Head – Deep Learning Practice, NVIDIA & Soumendra Mohanty, COO & EVP Analytics, Tredence Inc.
The last 3 months, upGrad has organized webinars on Industry relevant topics by some of the most sought after speakers in varied different formats.
This was the Elite Panel Discussion on Data Science to end the quarter on a high.
To shed some light on the Why(s) and How(s), the impact it creates on each career path and the ways in which young aspirants should function in the Data Science industry, upGrad brought together for this panel discussion 3 Industry experts who’ve closely seen data becoming the mastermind behind everything.
Related Posts
AIQRATIONS
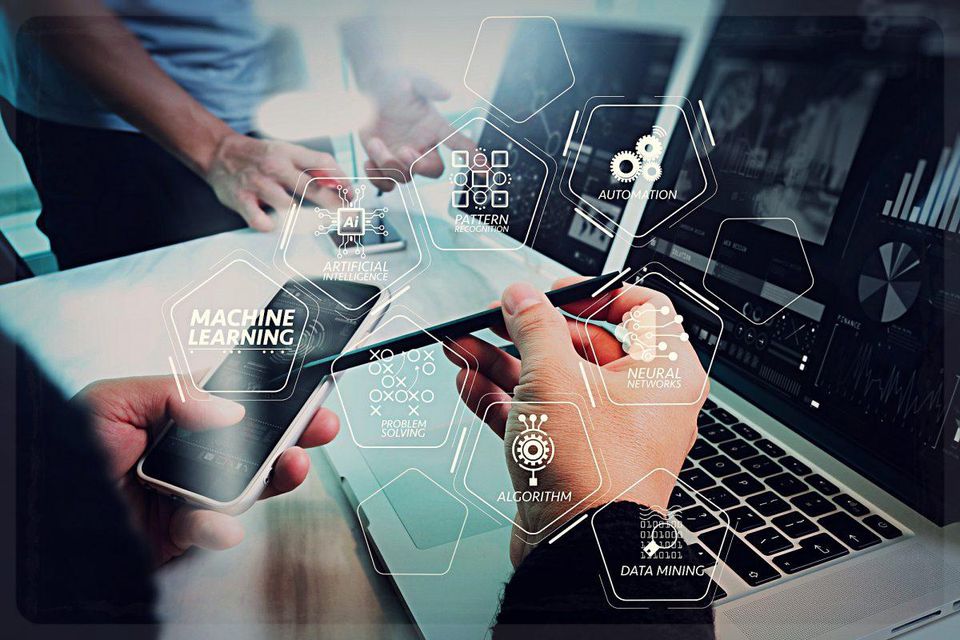
Reimagining Enterprise Decision-Making With Artificial Intelligence
Add Your Heading Text Here
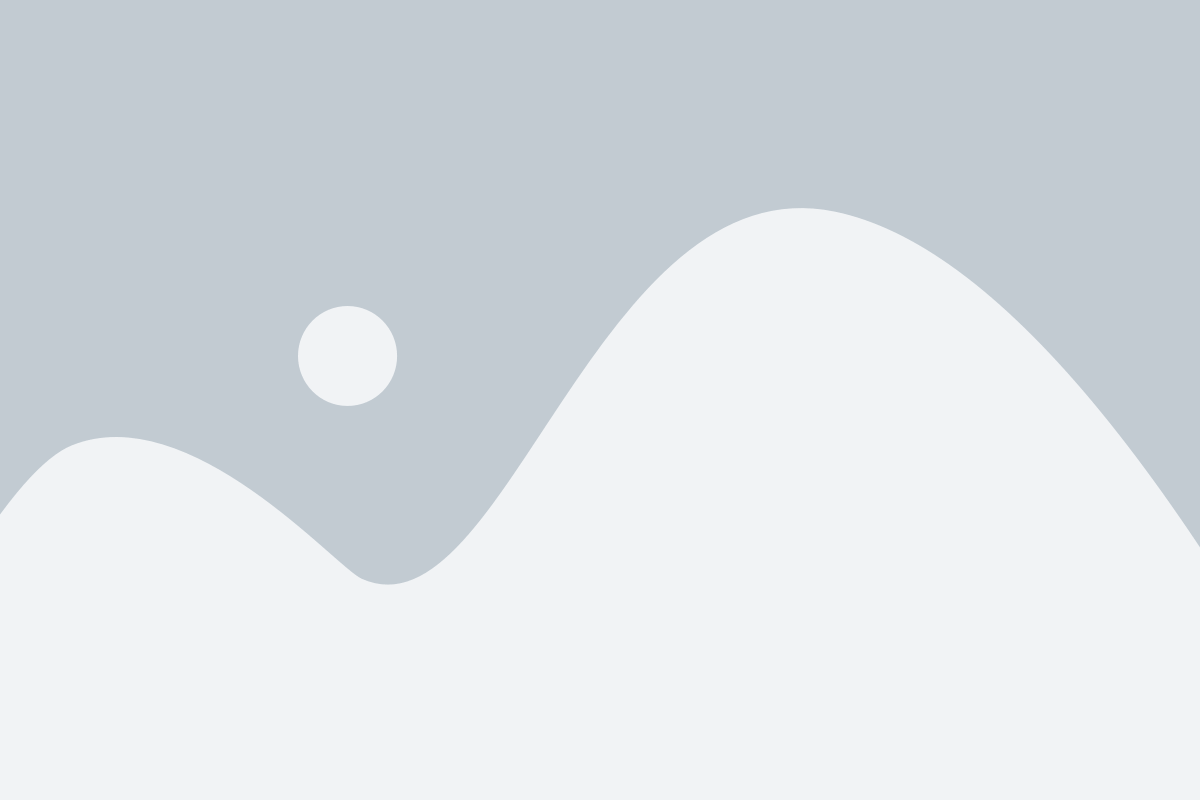
Artificial Intelligence will deliver revolutionary impact on how enterprises make decisions today. In the last few years alone, we have rapidly moved beyond heuristics-based decision-making to analytics-driven decision-support. In the VUCA phase, businesses globally are now pivoting to an AI-led, algorithm-augmented style of decision-making. With huge computing power and ever-increasing data storage and analytics prowess, we are entering a new paradigm, a probable and interesting scenario wherein, Artificial Intelligence will play a huge role in augmenting human intelligence and enabling decision-making with complete autonomy. The big hope is that this new paradigm will not only reduce human biases and errors that are common with heuristic decisions, but also reduce the time involved in making these critical decisions.
Here, I’ll attempt to focus on how we moved from simpler data driven decision-support to AI-powered decisions. The evolution of this technology has been breathtaking to behold and just might provide clues as to what we can expect in the future. Further, I’ll cover a few critical aspects that need to be inculcated by organizations on the AI transformation journey, and provide a few insightful cues that will make this journey exciting and fruitful.
Transformation of Decision-Making: From Analytics to AI
First, let us look at how we got here. Some truly pathbreaking events happened along the way while we were trying to make more accurate business decisions, leading us to reimagine how decisions will be made in the enterprise.
Organizations are Becoming Math Houses
With data deluge and digital detonation, combined with the appreciation of the fact that robust analytical capabilities lead to more informed decisions, we are witnessing AI savvy organizations rapidly maturing into ‘math houses.’ Data science – the ability to extract meaningful insights out of data has become de rigueur. Why? Because we now know that data, when seen in isolation, is inherently dumb. It is the ability to process this data and identify patterns and anomalies – using sophisticated algorithms and ensemble techniques – that makes all the difference. These self-intuitive algorithms are where real value resides – as they define the intelligence required to uncover insights and make smart recommendations. Organizations today are evolving into algorithm factories. There is a real understanding today that by enabling continuous advancement in mathematical algorithms, we can deliver consistent decisions based on prescribed as well as evolving business rules.
It is now an established reality that companies with robust mathematical capabilities possess a huge advantage over those that don’t. Indeed, it’s this math-house orientation that separates companies like Amazon and Google from the ones they leave in their wake, with their ability to understand their customers better, identify anomalies and recognize key patterns.
AI: From Predictive to Prescriptive
We saw a similar evolution in the age of analytics – wherein the science and value veered from descriptive analytics, providing diagnostics of past events to prescriptive analytics, helping see and shape the future. We are seeing a similar evolution in how AI gets leveraged in the enterprise and where its maximum value lies.
In early implementations, it was common to see AI as just a tool to predict and forecast future conditions, while accounting for the dynamism seen in the external environment. Today, AI-enabled decision-making is more prescriptive, with AI providing enterprises not just a look into the future, but also key diagnostics and suggestions on potential decision options and their payoffs. Such evolved applications of AI can help businesses make decisions that can potentially exploit more business opportunities, while averting potential threats much earlier.
Mr. Algorithm to Drive Decision Making
The culmination of this AI-era advancement would be the introduction of smart algorithms in every walk of life and business. Algorithms will become further mainstream leading to what will be the most sweeping business change since the industrial revolution. Organizations – those that already aren’t – will start developing a suite of algorithmic IP’s that will de-bias most enterprise decisions.
If Mr. Algorithm is going to drive most enterprise decisions of tomorrow, we need to create some checks and balances to ensure that it does not go awry. It is more critical today than ever before that the algorithmic suite developed by enterprises has a strong grounding in ethics and can handle situations appropriately for which explicit training may not have been provided.
How to Enable this AI Era of Change
Ushering into an AI-centric era of decision-making will require organizational transformation from business, cultural and technical standpoints. The following facets will be the enablers of this change:
Developing an Engineering Mindset
Instrumenting AI in the enterprise requires a combination of data scientists and computer scientists. As AI matures in the enterprise, the users, use cases and data will increase exponentially. To deliver impactful AI applications, scale and extensibility is critically important. This is where having an engineering mindset comes in. Imbibing an engineering mindset will help standardize the use of these applications while ensuring that they are scalable and extensible.
Learning, Unlearning, Relearning
The other critical aspect to a culture where AI can thrive is creating an environment supporting continuous unlearning and relearning. AI can succeed if the people developing and operating it are rewarded for continuous experimentation and exploration. And just like AI, people should be encouraged to incorporate feedback loops and learn continuously. As technology matures it’s important that the existing workforce keeps up. For one, it’s critical that the knowledge of algorithm theory, applied math alongside training on AI library and developer tools, is imparted into the workforce – and is continuously updated to reflect new breakthroughs in this space.
Embedding Design-Thinking and Behavioral Science at the Center of this Transformation
Finally, given the nature of AI applications, it’s critical that they are consumed voraciously. User input very often activates the learning cycles of artificial intelligence applications. To ensure high usage of these applications, it’s very important that we put the user at the center while designing these applications. This is where the application of behavioral sciences and human-centered design will deliver impact. By imparting empathy in these applications for the user, we will be able to design better and more useful AI applications.
As we augment decision-making with algorithmic, AI-centered systems and platforms – the big expectation is that they will bring untold efficiencies in terms of cost, alongside improvement in the speed and quality with which decisions get made. It’s time to reimagine and deliver on enterprise decision-making that is increasingly shaped through artificial intelligence. These aspects – how the AI is progressing and how to exploit its potential are of paramount importance to keep in mind for an AI transformation.
Related Posts
AIQRATIONS
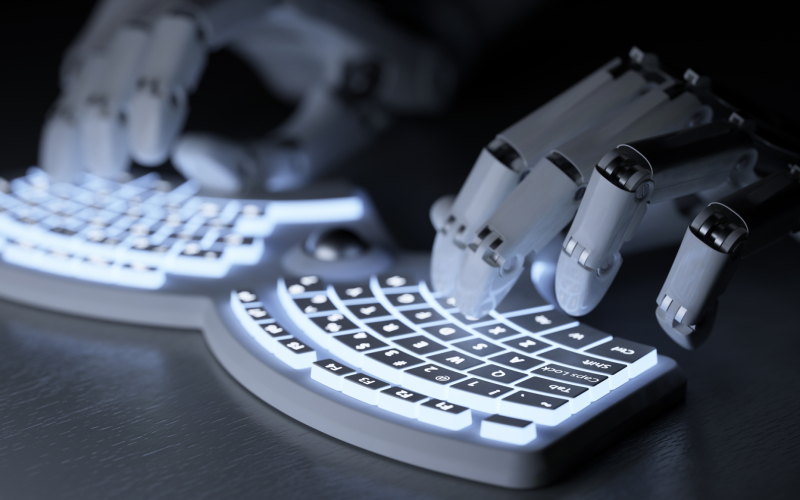
Chatbots – The Protege of AI & Data Sciences
Add Your Heading Text Here
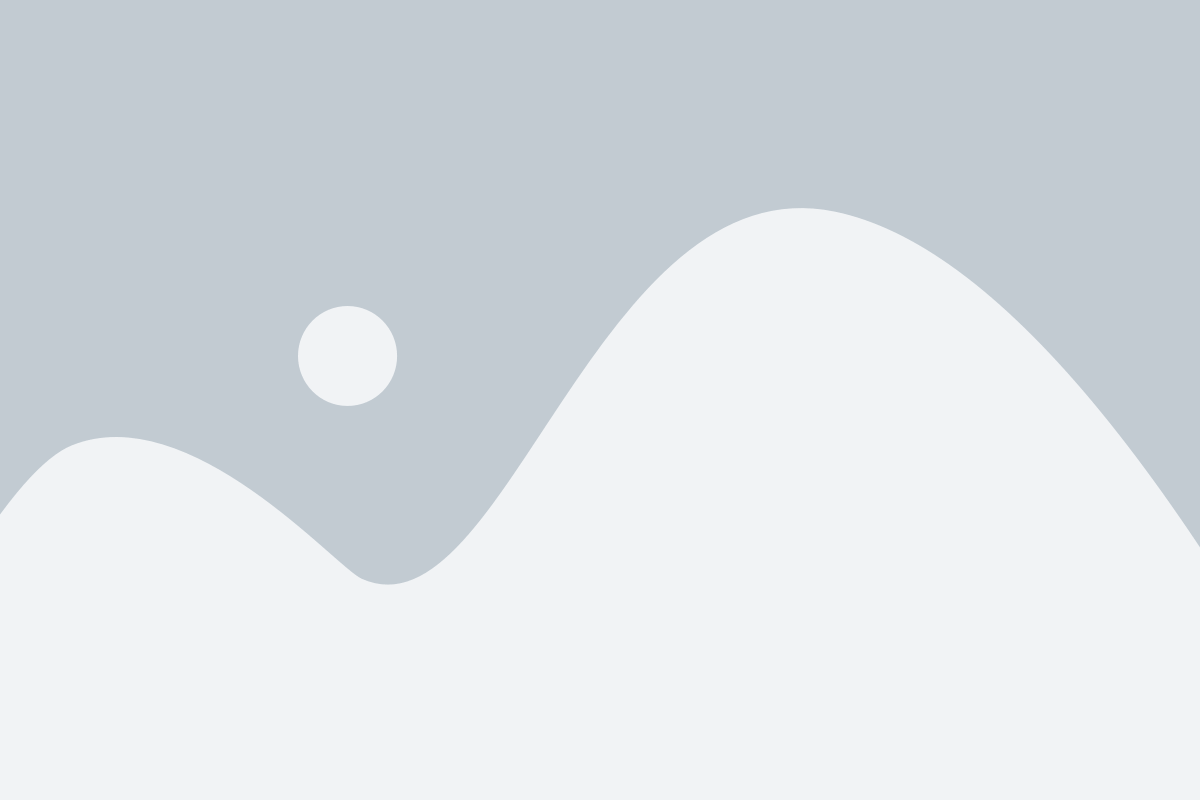
There has been a great deal of talk about the use of Artificial Intelligence chatbots in the last few weeks, especially given the news that Facebook are looking to implement AI into their Messenger and WhatsApp platforms, which are currently used by more than 1.8 billion people worldwide. However, does this bode well for the relationship between humans and Artificial Intelligence programs? Would you rather speak to an intelligent algorithm rather than a fellow human being?
The Sales and Customer Support Bot-ler ?
Chatbots, done right, are the cutting-edge form of interactive communications that captivate and engage users. But what kind of potential do they have for sales & customer support ?
To answer this, I should emphasize that customer service can be a delicate field. A lot of consumer engagement with a company happens when something goes wrong — such as a recently-purchased broken product or an incorrect bill or invoice.
By nature, these situations can be highly emotional. And as a business, you want to be responsive to potentially problematic customer inquiries like these. So relying on a chatbot to resolve issues that require a human touch might not be the best idea.
This is especially true if you let your bot “learn” from interactions it sees (say, in user forums) with no or minimal supervision. Things can easily go wrong, as the disaster around Microsoft’s Twitter bot “Tay” showed.
On the other hand, with the right supervision and enough training data, machine learning as an A.I. technique can help build very responsive and accurate informational chatbots — for example those that are meant to help surface data from large text collections, such as manuals.
I’d say that machine learning as a technique has been shown to work best on image processing. The advancements that Google, Facebook, and innovative startups such as Moodstocks (just acquired by Google) are showing in that space are truly amazing. Part of the amazement however, comes from the fact that we now see software take on another cognitive task that we thought could only be managed by humans.
What can bots do for the bottom line?
In my opinion, a bot’s primary application lies in customer service since most companies unfortunately continue to rely on an ancient methodology to manage customer interaction. And this is to be expected as most consumers themselves are still “hard-wired” to pick up a phone and dial a number when they want to engage with a company.
Companies haven’t necessarily made it easy for consumers to transition to digital-first interaction. Consumers are forced to either download a mobile app, browse websites, or use voice, the “dumbest” channel the smartphone has to offer, to retrieve information or perform transactions.
This is truly unfortunate because when it comes to paying a bill, checking on an order status, or reviewing account transactions, nothing is easier than sending a simple message. And with 900 million users now on Facebook Messenger, 1 billion on WhatsApp, and hundreds of millions more on basic SMS, companies have a consumer-preferred new medium for engaging with customers.
With messaging, a simple question can be posed in a simple message such as “Where is my order?”
Contrast this to the conventional options of being forced to shepherding that question through a maze of web or mobile app menus, or with IVR systems over the phone. Now imagine how a consumer-adopted, digital and automated interaction for simple questions vs. agent interaction over the phone could impact customer service and its cost. When chatbots handle the most commonly-asked questions, agent labor is reduced or redeployed to manage more complex and time-consuming interactions. Simple and moderate issues are resolved faster, leading to greater customer satisfaction and long-term loyalty. Bots can help deflect calls from the contact center and your IVR, which further reduces speech recognition license and telephony cost.
Could there be Bot-tle-necks?
There is also the question of whether these chatbots will take jobs from humans; a subject of fierce debate for all industries and levels in the last few months. Facebook itself has been quick to clarify that these chatbots are not going to replace the people in their organisation, but instead to work alongside them. For example, Facebook have said that the customer service executives will be required to train the AI bots, and to step in when the AI comes unstuck, which is likely to be fairly frequently in the early stages! Chinese messenger service WeChat has taken the chatbot idea on, with companies having official accounts through which they are able to communicate with their customers. However, the platform is still in its early stages, and is reported to be incredibly frustrating to use, so those in the customer service sector needn’t worry that their jobs are under threat quite yet!
While we might see chatbots starting to appear through the likes of Facebook Messenger and WhatsApp platforms in the coming 12 months, and will be dedicating teams of engineers to train the platforms, rather than relying on the general public. There are three main factors on which their success depends.
The first is with how much freedom AI in general is allowed to be developed, especially given the hesitation that the likes of Elon Musk and Bill Gates have about a potential ‘Singularity’, with Musk recently being quoted as saying that ‘Artificial Intelligence is our biggest existential threat’.
The second is arguably more important; how willing the general public are to help develop the chatbots, by having conversations with them, in the knowledge that they are talking to an autonomous entity.
More important still, are these chatbots going to be safe from cyberattacks? How will you know if your financial information will be secure if you disclose it to a chatbot, especially if there are unlikely to be the same multi-stage security checks that are the hallmark of P2P customer service interactions?
The Road Ahead?
Many companies are already launching bots for customer acquisition or customer service. We will see failures, and in parts, have already seen some. Bots are not trivial to build: you need people with experience in man-machine interface design. But to quote Amara’s Law: “We tend to overestimate the effect of a technology in the short run and underestimate the effect in the long run.”
Bots are here to stay, and will be a great new platform and make things easier for all of us. But bots that try to do too much or set unreasonable expectations will slow consumer confidence and acceptance of them. What might help us now is maybe to calm down a bit with the hype, and focus on building good bots that have value — then share our experiences, and show the world where the true value lies.
Related Posts
AIQRATIONS
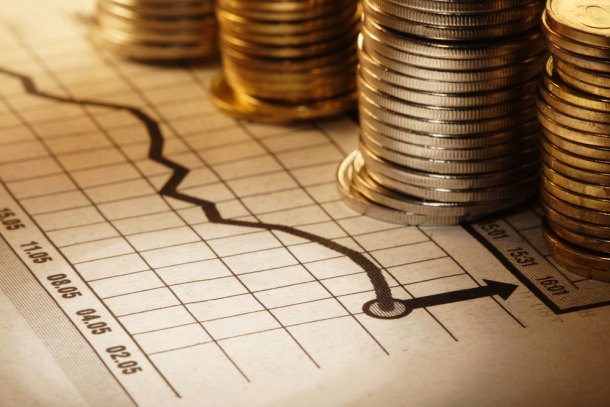
Data Sciences @ Fintech Companies for Competitive Disruption & Advantage
Add Your Heading Text Here
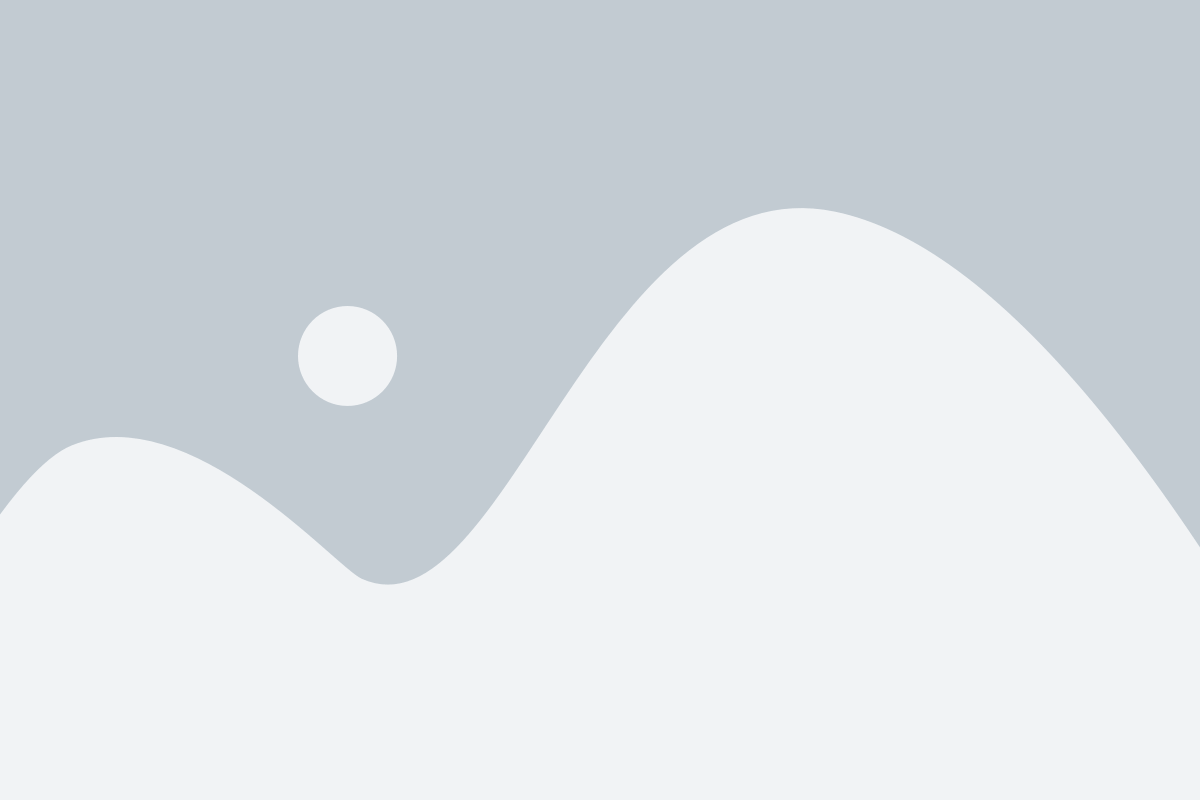
Long considered an impenetrable fortress dominated by a few well-known names, the banking and financial services industry is currently riding a giant wave of entrepreneurial disruption, disinter-mediation, and digital innovation. Everywhere, things are in flux. New, venture-backed arrivals are challenging the old powerhouses. Banks and financial services companies are caught between increasingly strict and costly regulations, and the need to compete through continuous innovation.
How does an entire industry remain relevant, authoritative, and trustworthy while struggling to surmount inflexible legacy systems, outdated business models, and a tired culture? Is there a way for banks and other traditional financial services companies to stay on budget while managing the competitive threat of agile newcomers and startups that do business at lower costs and with better margins? The threat is real. Can established institutions evolve in time to avoid being replaced? What other strategies can protect their extensive infrastructures and win the battle for the customer’s mind, heart, and wallet?
Financial technology, or fintech, is on fire with innovation and investment. The movement is reshaping entrepreneurial businesses and shaking the financial industry, reimagining the methods and tools consumers use to manage, save, and spend money. Agile fintech companies and their technology-intensive offerings do not shy away from big data, analytics, cloud computing, and machine learning, and they insist on a data-driven culture.
Consider a recent Forbes article by Chance Barnett, which quantifies fintech startup investments at $12 billion in 2014, quadrupling the $3 billion level achieved a year earlier. Adding to the wonderment, crowdfunding is likely to surpass venture capital in 2016 as a primary funding source. And people are joining the conversation. Barnett writes, “According to iQ Media, the number of mentions for ‘fintech’ on social media grew four times between 2013 and 2014, and will probably double again in 2015.” All of this activity underscores how technology is rattling the financial status quo and changing the very nature of money.
Yesterday’s Bank: A Rigid Culture, Strapped for Funds
Established banking institutions are strapped. The financial meltdown in 2008 questioned their operations, eroded trust, and invited punitive regulation designed to command, control, and correct the infractions of the past. Regulatory requirements have drained budgets, time, and attention, locking the major firms into constant compliance reporting. To the chagrin of some, these same regulations have also opened the door for new market entrants, technologies, platforms, and modalities—all of which are transforming the industry.
For traditional banking institutions, focus and energy for innovation are simply not there, nor are the necessary IT budgets. Gartner’s Q3 2015 forecast for worldwide IT spending growth (including all devices, data center systems, enterprise software, IT and Telecom services) hints at the challenge banks face: global IT spending is now down to -4.9%, even further from the -1.3% originally forecast, evidence of the spending and investment restraint we see across the financial landscape.
With IT budgets limited, it is hard to imagine banking firms easily reinventing themselves. Yet some are doing just that. Efficient spending is a top strategic priority for banking institutions. Many banks are moving away from a heavy concentration on compliance spending to instead focus on digital transformation, innovation, or collaboration with fintech firms. There is a huge amount of activity on all fronts. To begin, let’s review the competitive landscape of prominent fintech startups.
Data Sciences Intervention
Digital data has snowballed, with the proliferation of the internet, smartphones and other devices. Companies and governments alike recognize the massive potential in using this information – also known as Big Data – to drive real value for customers, and improve efficiency.
Big Data could transform businesses and economies, but the real game changer is data science.
Data science goes beyond traditional statistics to extract actionable insights from information – not just the sort of information you might find in a spreadsheet, but everything from emails and phone calls to text, images, video, social media data streaming, internet searches, GPS locations and computer logs.
“Data sciences enables us to process data better, faster and cheaper than ever
With powerful new techniques, including complex machine-learning algorithms, data science enables us to process data better, faster and cheaper than ever before.
We’re already seeing significant benefits of this – in areas such as national security, business intelligence, law enforcement, financial analysis, health care and disaster preparedness. From location analytics to predictive marketing to cognitive computing, the array of possibilities is overwhelming, sometimes even life-saving. The New York City Fire Department, for example, was one of the earlier success stories of using data science to proactively identify buildings most at risk from fire.
Unleashing the power of Advanced Data Mining using Data Sciences
For banks – in an era when banking is becoming commoditized – the data mining provides a massive opportunity to stand out from the competition. Every banking transaction is a nugget of data, so the industry sits on vast stores of information.
By using data science to collect and analyses Data, banks can improve, or reinvent, nearly every aspect of banking. Data science can enable hyper-targeted marketing, optimized transaction processing, personalized wealth management advice and more – the potential is endless.
A large proportion of the current Data Mining projects in banking revolve around customers – driving sales, boosting retention, improving service, and identifying needs, so the right offers can be served up at the right time.
“Data sciences can help strengthen risk management such as cards fraud detection
Banks can model their clients’ financial performance on multiple data sources and scenarios. Data science can also help strengthen risk management in areas such as cards fraud detection, financial crime compliance, credit scoring, stress-testing and cyber analytics.
The promise of Big Data is even greater than this, however, potentially opening up whole new frontiers in financial services.
Seamless experience for customers
Over 1.7 billion people with mobile phones are currently excluded from the formal financial system. This makes them invisible to credit bureaus, but they are increasingly becoming discoverable through their mobile footprint. Several innovative Fintech firms have already started building predictive models using this type of unconventional data to assess credit risk and provide new types of financing.
While banks have historically been good at running analytics at a product level, such as credit cards, or mortgages, very few have done so holistically, looking across inter-connected customer relationships that could offer a business opportunity – say when an individual customer works for, supplies or purchases from a company that is also a client of the bank. The evolving field of data science facilitates this seamless view.
Blockchain as the new database
Much more is yet to come. Blockchain, the underlying disruptive technology behind cryptocurrency Bitcoin, could spell huge change for financial services in the future. Saving information as ‘hash’, rather than in its original format, the blockchain ensures each data element is unique, time-stamped and tamper-resistant.
The semi-public nature of some types of blockchain paves the way for an enhanced level of security and privacy for sensitive data – a new kind of database where the information ‘header’ is public but the data inside is ‘private’.
As such, the blockchain has several potential applications in financial markets – think of trade finance, stock exchanges, central securities depositories, trade repositories or settlements systems.
Data analytics using blockchain, distributed ledger transactions and smart contracts will become critical in future, creating new challenges and opportunities in the world of data science.