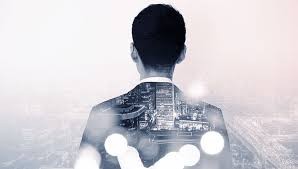
Cloud Platforms: Strategic Enabler for AI led Transformation
Add Your Heading Text Here
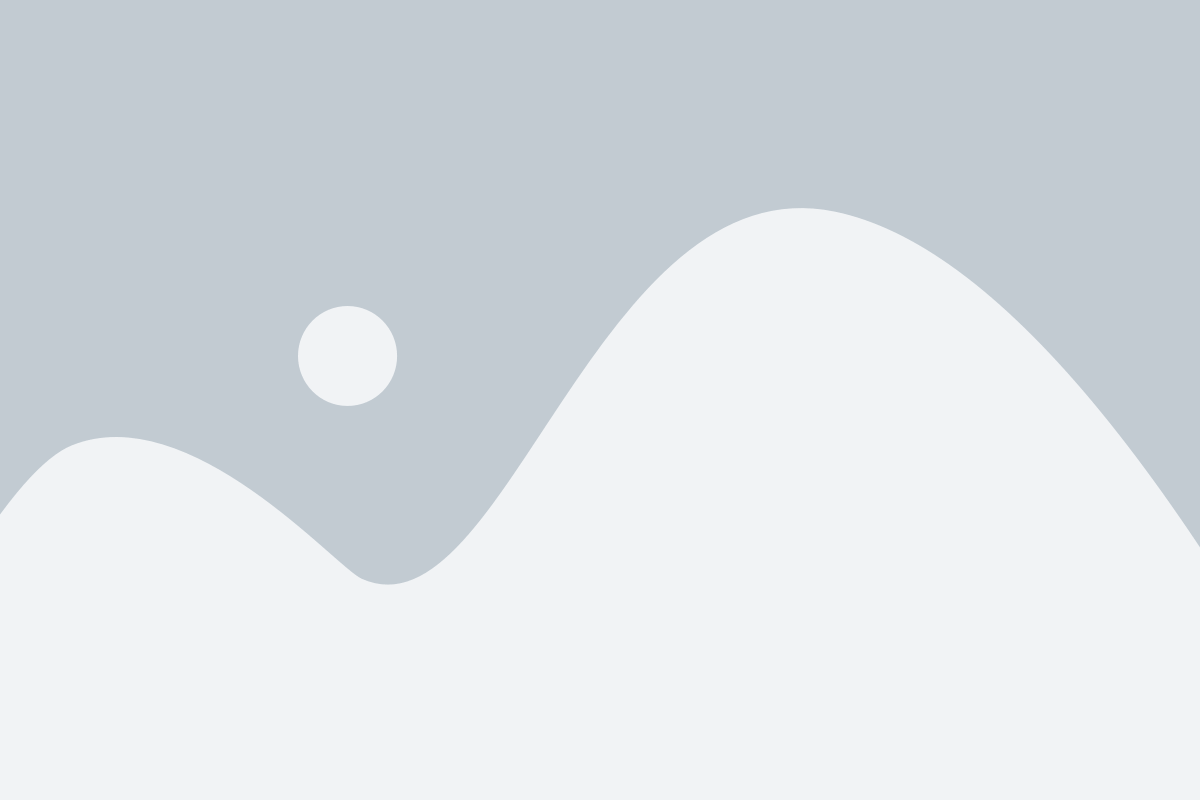
CIOs & CTOs have been toying with the idea of cloud adoption at scale for more than a decade since the first corporate experiments with external cloud platforms were conceptualized, and the verdict is long in on their business value. Companies that adopt the cloud well bring new capabilities to market more quickly, innovate more easily, and scale more efficiently—while also reducing technology risk.
Unfortunately, the verdict is still out on what constitutes a successful cloud implementation to actually capture that value. Most CIOs and CTOs default to traditional implementation models that may have been successful in the past but that make it almost impossible to capture the real value from the cloud. Defining the cloud opportunity too narrowly with siloed business initiatives, such as next-generation application hosting or data platforms, almost guarantees failure. That’s because no design consideration is given to how the organization will need to operate holistically in cloud, increasing the risk of disruption from nimbler attackers with modern technology platforms that enable business agility and innovation.
Companies that reap value from cloud platforms treat their adoption as a business- AI led transformation by doing three things:
- Focusing investments on business domains where cloud can enable increased revenues and improved margins
2. Selecting a technology and sourcing model that aligns with business strategy and risk constraints
3. Developing and implementing an operating model that is oriented around the cloud
CIOs and CTOs need to drive cloud adoption, but, given the scale and scope of change required to exploit this opportunity fully, they also need support and air cover from the rest of the management team.
Using cloud to enable AI led transformation : Only 14 percent of companies launching AI transformations have seen sustained and material performance improvements. Why? Technology execution capabilities are often not up to the task. Outdated AI technology environments make change expensive. Quarterly release cycles make it hard to tune AI capabilities to changing market demands. Rigid and brittle infrastructures choke on the data required for sophisticated analytics.
Operating in the cloud can reduce or eliminate many of these issues. Exploiting cloud services and tooling, however, requires change across all of IT and many business functions as well—in effect, a different business-technology model.
AI led transformation success requires CIOs and tech leaders to do three things :
1. Focus cloud investments in business domains where cloud platforms can enable increased revenues and improved margins:
The vast majority of the value the cloud generates comes from increased agility, innovation, and resilience provided to the business with sustained velocity. In most cases, this requires focusing cloud adoption on embedding re usability and composability so investment in modernizing can be rapidly scaled across the rest of the organization. This approach can also help focus programs on where the benefits matter most, rather than scrutinizing individual applications for potential cost savings
Faster time to market: Cloud-native companies can release code into production hundreds or thousands of times per day using end-to-end automation. Even traditional enterprises have found that automated cloud platforms allow them to release new capabilities daily, enabling them to respond to market demands and quickly test what does and doesn’t work. As a result, companies that have adopted cloud platforms report that they can bring new capabilities to market about 20 to 40 percent faster.
Ability to create innovative business offerings: Each of the major cloud service providers offers hundreds of native services and marketplaces that provide access to third-party ecosystems with thousands more. These services rapidly evolve and grow and provide not only basic infrastructure capabilities but also advanced functionality such as facial recognition, natural-language processing, quantum computing, and data aggregation.
Reduced risk: Cloud clearly disrupts existing security practices and architectures but also provides a rare opportunity to eliminate vast operational overhead to those that can design their platforms to consume cloud securely. Taking advantage of the multi billion-dollar investments CSPs have made in security operations requires a cyber-first design that automatically embeds robust standardized authentication, hardened infrastructure, and a resilient interconnected data-center availability zone.
Efficient scalability: Cloud enables companies to automatically add capacity to meet surge demand (in response to increasing customer usage, for example) and to scale out new services in seconds rather than the weeks it can take to procure additional on-premises servers. This capability has been particularly crucial during the COVID-19 pandemic, when the massive shift to digital channels created sudden and unprecedented demand peaks.
2. Select a technology, sourcing, and migration model that aligns with business and risk constraints
Decisions about cloud architecture and sourcing carry significant risk and cost implications—to the tune of hundreds of millions of dollars for large companies. The wrong technology and sourcing decisions will raise concerns about compliance, execution success, cyber security, and vendor risk—more than one large company has stopped its cloud program cold because of multiple types of risk. The right technology and source decisions not only mesh with the company’s risk appetite but can also “bend the curve” on cloud-adoption costs, generating support and excitement for the program across the management team.
If CIOs or CTOs make those decisions based on the narrow criteria of IT alone, they can create significant issues for the business. Instead, they must develop a clear picture of the business strategy as it relates to technology cost, investment, and risk.
3. Change operating models to capture cloud value
Capturing the value of migrating to the cloud requires changing both how IT works and how IT works with the business. The best CIOs and CTOs follow a number of principles in building a cloud-ready operating model:
Make everything a product : To optimize application functionality and mitigate technical debt,CIOs need to shift from “IT projects” to “products”—the technology-enabled offerings used by customers and employees. Most products will provide business capabilities such as order capture or billing. Automated as-a-service platforms will provide underlying technology services such as data management or web hosting. This approach focuses teams on delivering a finished working product rather than isolated elements of the product. This more integrated approach requires stable funding and a “product owner” to manage it.
Integrate with business. Achieving the speed and agility that cloud promises requires frequent interaction with business leaders to make a series of quick decisions. Practically, business leaders need to appoint knowledgeable decision makers as product owners for business-oriented products. These are people who have the knowledge and authority to make decisions about how to sequence business functionality as well as the understanding of the journeys of their “customers.”
Drive cloud skill sets across development teams. Traditional centers of excellence charged with defining configurations for cloud across the entire enterprise quickly get overwhelmed. Instead, top CIOs invest in delivery designs that embed mandatory self-service and co-creation approaches using abstracted, unified ways of working that are socialized using advanced training programs (such as “train the trainer”) to embed cloud knowledge in each agile tribe and even squad.
How Technology Leaders can join forces with leadership to drive AI led transformation
Given the economic and organizational complexity required to get the greatest benefits from the cloud, heads of infrastructure, CIOs, and CTOs need to engage with the rest of the leadership team. That engagement is especially important in the following areas:
Technology funding. Technology funding mechanisms frustrate cloud adoption—they prioritize features that the business wants now rather than critical infrastructure investments that will allow companies to add functionality more quickly and easily in the future. Each new bit of tactical business functionality built without best-practice cloud architectures adds to your technical debt—and thus to the complexity of building and implementing anything in the future. CIOs and CTOs need support from the rest of the management team to put in place stable funding models that will provide resources required to build underlying capabilities and remediate applications to run efficiently, effectively, and safely in the cloud.
Business-technology collaboration. Getting value from cloud platforms requires knowledgeable product owners with the power to make decisions about functionality and sequencing. That won’t happen unless the CEO and relevant business-unit heads mandate people in their organizations to be product owners and provide them with decision-making authority.
Engineering talent. Adopting the cloud requires specialized and sometimes hard-to-find technical talent—full-stack developers, data engineers, cloud-security engineers, identity and access-management specialists, cloud engineers, and site-reliability engineers. Unfortunately, some policies put in place a decade ago to contain IT costs can get in the way of on boarding cloud talent. Companies have adopted policies that limit costs per head and the number of senior hires, for example, which require the use of outsourced resources in low-cost locations. Collectively, these policies produce the reverse of what the cloud requires, which is a relatively small number of highly talented and expensive people who may not want to live in traditionally low-cost IT locations. CIOs and CTOs need changes in hiring and location policies to recruit and retain the talent needed for success in the cloud.
The recent COVID-19 pandemic has only heightened the need for companies to adopt AI led business models. Only cloud platforms can provide the required agility, scalability, and innovative capabilities required for this transition. While there have been frustrations and false starts in the enterprise cloud journey, companies can dramatically accelerate their progress by focusing cloud investments where they will provide the most business value and building cloud-ready operating models.
Related Posts
AIQRATIONS
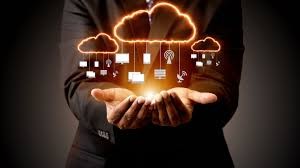
Best Practices to Accelerate & Transform Analytics Adoption in the Cloud
Add Your Heading Text Here
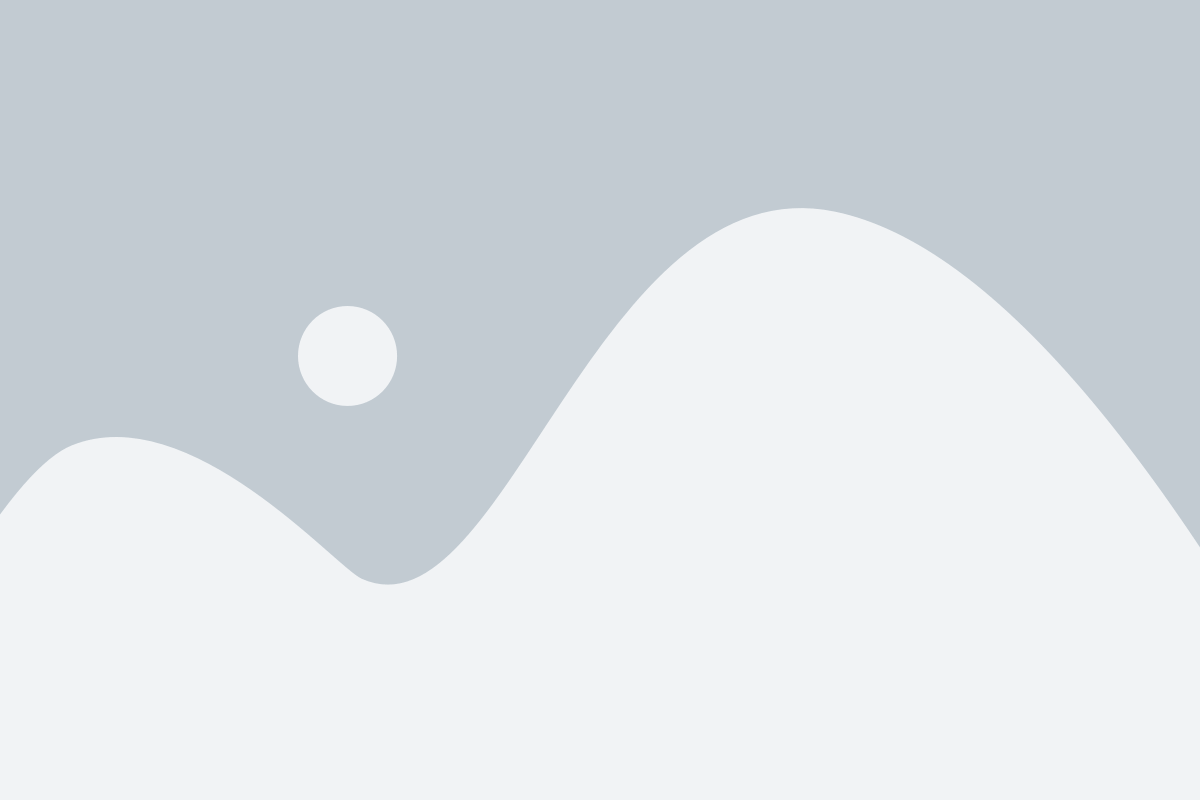
Reimagining analytics in the cloud enables enterprises to achieve greater agility, increase scalability and optimize costs. But organizations take different paths to achieving their goals. The best way to proceed will depend on data environment and business objectives. There are two best practices to maximize analytics adoption in the cloud:
• Cloud Data Warehouse, Data Lake, and Lakehouse Transformation: Strategically moving data warehouse and data lake to the cloud over time and adopting a modern, end-to-end data infrastructure for AI, and machine learning projects.
• New Cloud Data Warehouse and Data Lake: Start small and fast and grow as needed by spinning up a new cloud data warehouse or cloud data lake. The same guidance applies whether implementing new data warehouses and data lakes in the cloud for the first time, or doing so for an individual department or line of business.
As cloud adoption grows, most organizations will eventually want to modernize their enterprise analytics infrastructure entirely in the cloud. With the transformation pathway, rebuild everything to take advantage of the most modern cloud-based enterprise data warehouse, data lake, and lake house technology to end up in the strongest position long term. But migrate data and workloads from existing on-premises enterprise data warehouse and data lake to the cloud incrementally, over time. This approach allows enterprises to be strategic while minimizing disruption. Enterprises can take the time to carefully evaluate data and bring over only what is needed, which makes this a less risky approach. It also enables more complex analysis of data, using artificial intelligence, machine learning. The combination of a cloud data warehouse and data lake allows to manage the data necessary for analytics by providing economical scalability across compute and storage that is not possible with an on-premises infrastructure. And it enables to incorporate new types of data, from IoT sensors, social media, text, and more, into your analysis to gain new insights.
For this pathway ,enterprises need an intelligent, automated data platform that delivers a number of critical capabilities. It should handle new data sources, accommodate AI and machine learning projects, support new processing engines, deliver performance at a massive scale, and offer serverless scale up/scale down capabilities. As with a brand-new cloud data warehouse or data lake, enterprises need cloud-native, best-of-breed data integration, data quality, and metadata management to ensure maximizing the value of cloud analytics. Once the data is in the cloud, organization can provide users with self-service access to this data so they can more easily and seamlessly create reports or take swift decision. Subsequently , this transformation pathway gives organizations an end-to-end modern infrastructure for next-generation cloud analytics
Lines of business increasingly rely on analytics to improve processes and business impact. For example, sales and marketing no longer ask, “How many leads did we generate?” They want to know how many sales-ready leads we gathered from Global 500 accounts as evidenced by user time spent consuming content on the web. But individual lines of business may not have the time or resources to create and maintain an on-premises data warehouse to answer these questions. With a new cloud data warehouse and data lake, departments can get analytics projects off the ground quickly and cost effectively. Departments simply spin up their own cloud data warehouses, populate them with data, and make sure they’re connected to analytics and BI tools. For data science projects, a team may want to quickly add a cloud data lake. In some cases, this approach enables the team to respond to requests for sophisticated analysis faster than centralized teams can normally handle. Whatever the purpose of new cloud data warehouse and data lake, enterprises need intelligent, automated cloud data management with best of-breed, cloud-native data integration, data quality, and metadata management all built on a cloud-native platform in order to deliver value and drive ROI. And note that while this approach allows enterprises to start small and scale as needed, the downside is that data warehouse and data lake may only benefit a particular department inside the enterprise.
Some organizations with significant investments in on-premises enterprise data warehouses and data lakes are looking to simply replicate their existing systems to the cloud. By lifting and shifting their data warehouse or data lake “as is” to the cloud, they seek to improve flexibility, increase scalability, and lower data center costs while migrating quickly to minimize disruption. Lifting and shifting an on-premises system to the cloud may seem fast and safe. But in reality, it’s an inefficient approach, one that’s like throwing everything you own into a moving van instead of packing strategically for a plane trip. In the long run, reducing baggage and traveling by air delivers greater agility and faster results because you are not weighed down by unnecessary clutter. Some organizations may need to do a lift and shift, but most will find it’s not the best course of action because it simply persists outdated or inefficient legacy systems and offers little in the way of innovation.
Related Posts
AIQRATIONS
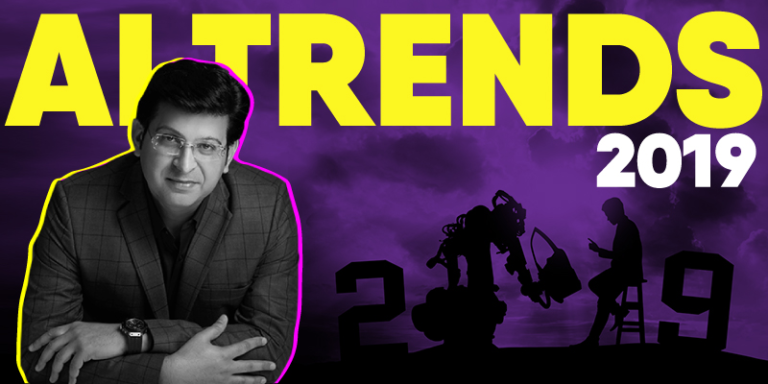
Here are the top 10 AI trends to watch out for in 2019
Add Your Heading Text Here
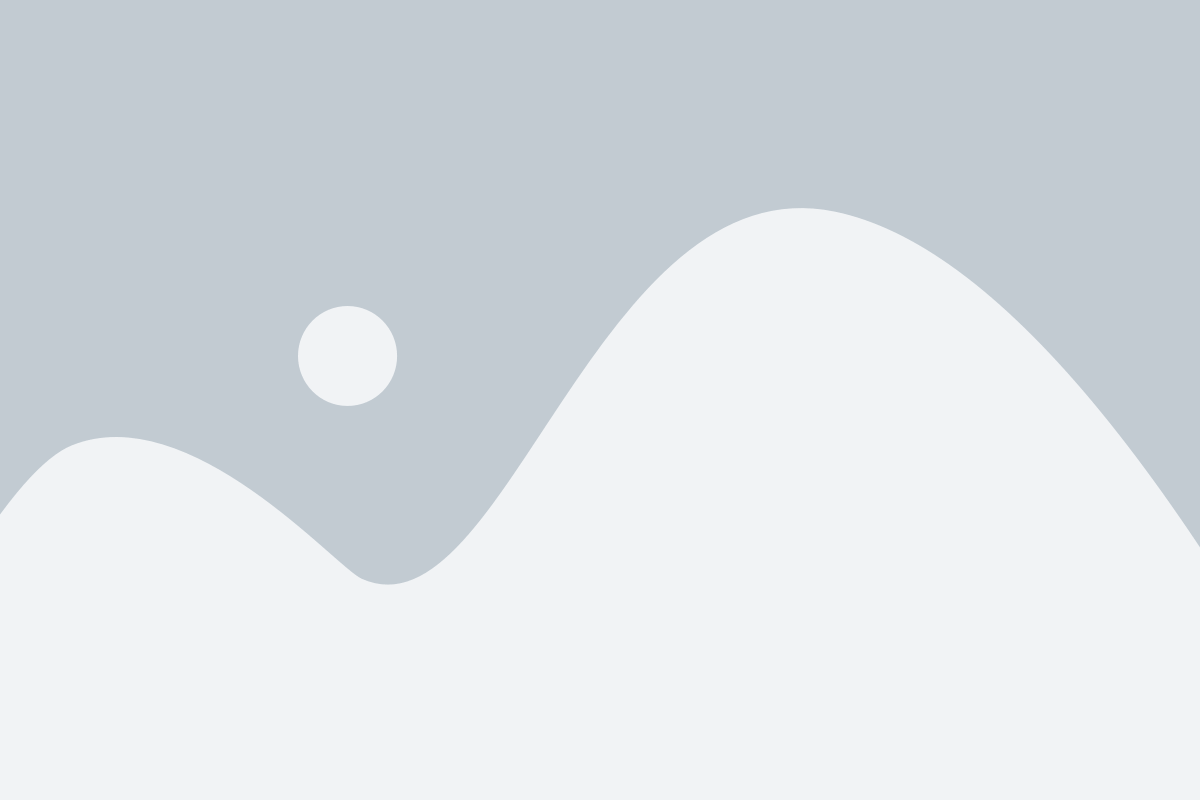
The year 2018 will be remembered as the year that artificial intelligence stopped being on the periphery of business and entered the mainstream realm. With increasing awareness and capability of AI among the numerous stakeholders, including tech buyers, vendors, investors, governments, and academia, I expect AI will go beyond just tinkering and experiments and will become the mainstay in the business arena.
With an increasing percentage of these stakeholders professing their commitment to leveraging this technology within their organisations, AI has arrived on the world scene. We are sure to see transformative business value being derived through AI in the coming years. As we come to the close of 2018, let us gaze into the crystal ball to see what 2019 will hold for this game-changing technology:
The rise of topical business applications
Currently, we have a lot of general purposes AI frameworks such as Machine Learning and Deep Learning that are being used by corporations for a plethora of use cases. We will see a further evolution of such technology into niche, topical business applications as the demand for pre-packaged software with lower time-to-value increases. We will see a migration from the traditional AI services paradigm to very specific out-of-the-box applications geared to serve particular use cases. Topical AI applications in this space that serve such use cases will be monumentally useful for furthering the growth of AI, rather than bespoke services that require longer development cycles and may cause bottlenecks that enterprises cannot afford.
The merger of AI, Blockchain, cloud, and IoT
Could a future software stack comprise AI, Blockchain, and IoT running on the cloud? It is not too hard to imagine how these exponential technologies can come together to create great value. IoT devices will largely be the interface with which consumers and other societal stakeholders will interact. Voice-enabled and always connected devices – such as Google Home and Amazon’s Alexa – will augment the customer experience and eventually become the primary point of contact with businesses. AI frameworks such as Speech Recognition and Natural Language Processing will be the translation layer between the sensor on one end and the deciphering technology on the other end. Blockchain-like decentralised databases will act as the immutable core for managing contracts, consumer requests, and transactions between various parties in the supply chain. The cloud will be the mainstay for running these applications, requiring huge computational resources and very high availability.
Focus on business value rather than cost efficiency
2019 will finally be the year that majority of the executive and boardroom conversations around AI will move from reducing headcount and cost efficiency to concrete business value. In 2019, more and more businesses will realise that focusing on AI solutions that reduce cost is a criminal waste of wonderful technology. Ai can be used to identify revenues lost, plug leakages in customer experience, and entirely reinvent business models. I am certain that businesses that focus only on the cost aspect will stand to lose ground to competitors that have a more cogent strategy to take the full advantage of the range of benefits that AI offers.
Development of AI-optimised hardware and software
Ubiquitous and all-pervasive availability of AI will require paradigm shifts in the design of the hardware and software that runs it. In 2019, we will see an explosion of hardware and software designed and optimised to run artificial intelligence. With the increasing size and scale of data fueling AI applications and even more complex algorithms, we will see a huge demand for specialised chipsets that can effectively run AI applications with minimal latency. Investors are showing heavy interest in companies developing GPUs, NPUs, and the like – as demonstrated by Chinese startup Cambricon, which stands valued at a whopping $2.5 billion since its last round of funding this year. End-user hardware such as smart assistants and wearables will also see a massive increase in demand. Traditional software paradigms will also continue to be challenged. Today’s novel frameworks such as TensorFlow will become de rigueur. Architectural components such as edge computing will ensure that higher processing power is more locally available to AI-powered applications.
‘Citizen AI’ to be the new normal
One of the reasons we saw widespread adoption of analytics and data-driven decision-making is because we built applications that democratised the power of data. No longer was data stuck in a remote silo, accessible only to the most sophisticated techies. With tools and technology frameworks we brought data into the mainstream and made it the cornerstone of how enterprises plan and execute strategy. According to Gartner, the number of citizen data scientists will grow five times faster than the number of expert data scientists. In 2019, I expect Citizen AI to gain traction as the new normal. Highly advanced AI-powered development environments that automate functional and non-functional aspects of applications will bring forward to a new class of “citizen application developers”, allowing executives to use AI-driven tools to automatically generate new solutions.
Policies to foster and govern AI
Following China’s blockbuster announcement of a National AI Policy in 2017, other countries have rushed to share their take on policy level interventions around AI. I expect to see more countries come forward with their versions of a policy framework for AI – from overarching vision to allaying concerns around ethical breaches. At the same time, countries will also be asked to temper their enthusiasm of widespread data proliferation by releasing their own versions of GDPR-like regulations. For enabling an ecosystem where data can be used to enrich AI algorithms, the public will need to be convinced that this is for the overall good, and they have nothing to fear from potential data misuse and theft.
Speech Recognition will revolutionise NLP
In the last few years, frameworks for Natural Language Understanding (NLU) and Natural Language Generation (NLG) have made huge strides. NLP algorithms are now able to decipher emotions, sarcasm, and figures of speech. Going forward, voice assistants will use data from voice and combine that with deep learning to associate the words spoken with emotions, enriching the overall library that processes speech and text. This will be a revolutionary step forward for fields such as customer service and customer experience where many bots have typically struggled with the customer’s tone of voice and intonation.
The growth of explainable AI
And finally, with numerous decisions powered by AI – and specifically unsupervised learning models – we will see enterprises demand “explainable” AI. In simplified terms, explainable AI helps executives “look under the hood” to understand the “what” and “why” of the decisions and recommendations made by artificial intelligence. Development of explainable AI will be predicated on the need for increased transparency and trust. Explainable AI will be essential to ensure that there is some level of transparency (and potentially, learning) that is gleaned from unsupervised systems.
Convergence of AI and analytics
This is a trend that is a logical consequence of the decisive power of data in business today. In 2019, we will see a merger of analytics and AI – as the one-stop for uncovering and understanding insights from data. With advancements in AI seen so far, the algorithms are more than capable of taking up tasks that involve complex insight generation from multi-source, voluminous data. This convergence of AI and analytics will lead to automation that will improve the speed and accuracy of the decisions that power business planning and strategy. AI-powered forecasting will help deliver faster decisions, with minimal human interventions and create higher cost savings for the business.
Focus on physical and cybersecurity paradigms
Two of the domains ripe for an AI transformation are the fields of physical and cybersecurity. As intrusions into physical and virtual environments become commonplace and threats become hugely pervasive, AI will be a massive boost to how we secure these environments. Advances in fields such as ML-powered anomaly detection will drastically reduce the time required to surface potential intrusions into secure environments. This will enable organisations to better protect user data. When combined with Blockchain, AI will give cybersecurity a huge boost through decentralised, traceable databases containing valuable client and strategic information. On the physical security side, Computer Vision is rapidly gaining currency in the fields of physical intruder detection. Surveillance cameras, originally manned by security guards, will soon be replaced by AI-powered systems that will be able to react faster and more proactively to intruders that pose a threat to physical premises. When you combine that with face recognition, working with a database of known offenders, we will see a quantum drop in the time required to adjudicate and address cases of theft and unauthorised entry by law enforcement agencies.
In summary, the broad directions that I predict AI will take include interventions to make it more embedded, responsible, and explainable; convergence with other exponential technologies such as cloud, Blockchain, and IoT; cybersecurity; a greater proliferation and development of use cases; and great strides in the technology and its supporting infrastructure. Enterprises would do well to adopt this revolutionary technology and ensure a strong availability of talent to conceptualise, develop, and unleash value from AI applications.
Related Posts
AIQRATIONS
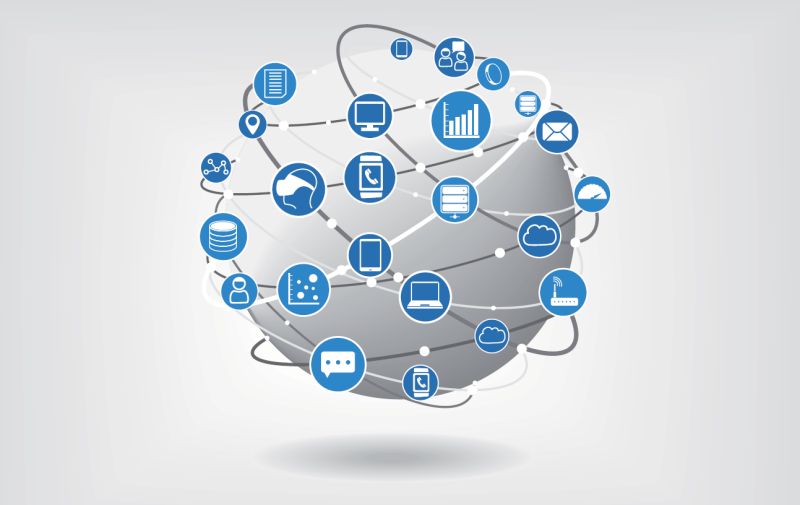
THE BEST PRACTICES FOR INTERNET OF THINGS ANALYTICS
Add Your Heading Text Here
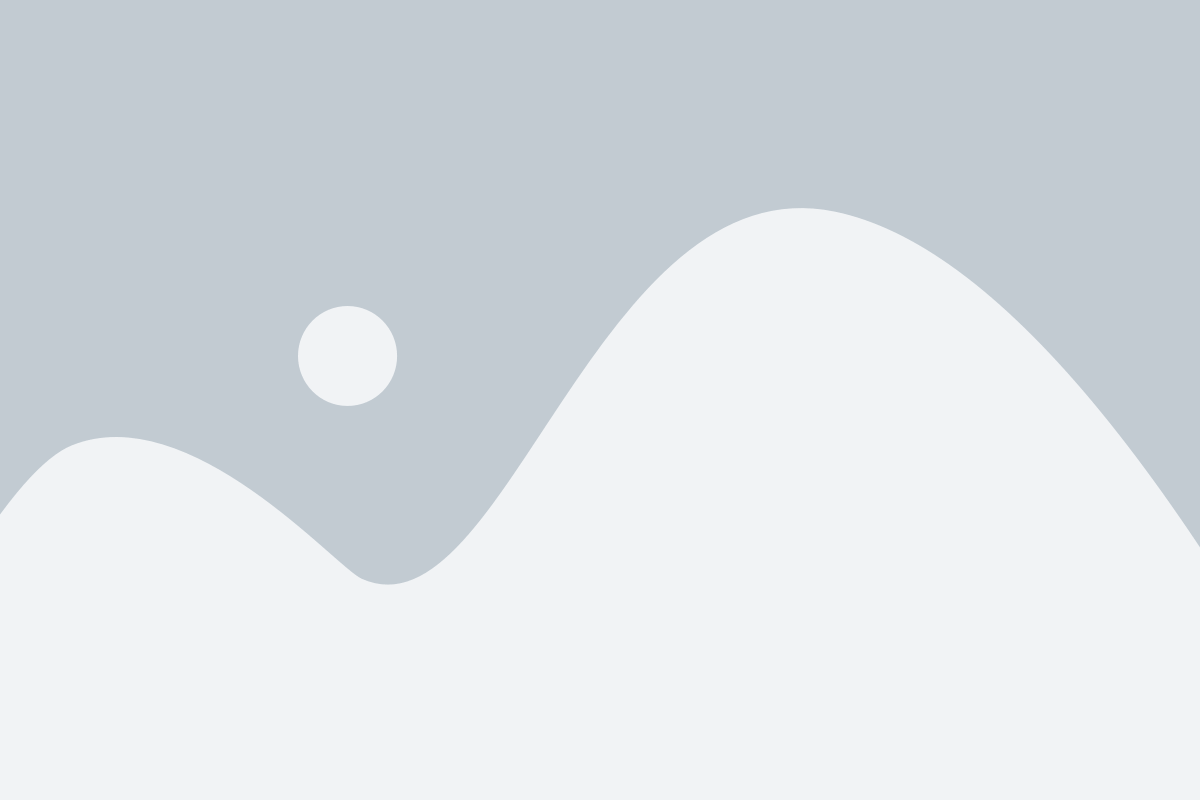
In most ways, Internet of Things analytics are like any other analytics. However, the need to distribute some IoT analytics to edge sites, and to use some technologies not commonly employed elsewhere, requires business intelligence and analytics leaders to adopt new best practices and software.
There are certain prominent challenges that Analytics Vendors are facing in venturing into building a capability. IoT analytics use most of the same algorithms and tools as other kinds of advanced analytics. However, a few techniques occur much more often in IoT analytics, and many analytics professionals have limited or no expertise in these. Analytics leaders are struggling to understand where to start with Internet of Things (IoT) analytics. They are not even sure what technologies are needed.
Also, the advent of IoT also leads to collection of raw data in a massive scale. IoT analytics that run in the cloud or in corporate data centers are the most similar to other analytics practices. Where major differences appear is at the “edge” — in factories, connected vehicles, connected homes and other distributed sites. The staple inputs for IoT analytics are streams of sensor data from machines, medical devices, environmental sensors and other physical entities. Processing this data in an efficient and timely manner sometimes requires event stream processing platforms, time series database management systems and specialized analytical algorithms. It also requires attention to security, communication, data storage, application integration, governance and other considerations beyond analytics. Hence it is imperative to evolve into edge analytics and distribute the data processing load all across.
Hence, some IoT analytics applications have to be distributed to “edge” sites, which makes them harder to deploy, manage and maintain. Many analytics and Data Science practitioners lack expertise in the streaming analytics, time series data management and other technologies used in IoT analytics.
Some visions of the IoT describe a simplistic scenario in which devices and gateways at the edge send all sensor data to the cloud, where the analytic processing is executed, and there are further indirect connections to traditional back-end enterprise applications. However, this describes only some IoT scenarios. In many others, analytical applications in servers, gateways, smart routers and devices process the sensor data near where it is generated — in factories, power plants, oil platforms, airplanes, ships, homes and so on. In these cases, only subsets of conditioned sensor data, or intermediate results (such as complex events) calculated from sensor data, are uploaded to the cloud or corporate data centers for processing by centralized analytics and other applications.
The design and development of IoT analytics — the model building — should generally be done in the cloud or in corporate data centers. However, analytics leaders need to distribute runtime analytics that serve local needs to edge sites. For certain IoT analytical applications, they will need to acquire, and learn how to use, new software tools that provide features not previously required by their analytics programs. These scenarios consequently give us the following best practices to be kept in mind:
Develop Most Analytical Models in the Cloud or at a Centralized Corporate Site
When analytics are applied to operational decision making, as in most IoT applications, they are usually implemented in a two-stage process – In the first stage, data scientists study the business problem and evaluate historical data to build analytical models, prepare data discovery applications or specify report templates. The work is interactive and iterative.
A second stage occurs after models are deployed into operational parts of the business. New data from sensors, business applications or other sources is fed into the models on a recurring basis. If it is a reporting application, a new report is generated, perhaps every night or every week (or every hour, month or quarter). If it is a data discovery application, the new data is made available to decision makers, along with formatted displays and predefined key performance indicators and measures. If it is a predictive or prescriptive analytic application, new data is run through a scoring service or other model to generate information for decision making.
The first stage is almost always implemented centrally, because Model building typically requires data from multiple locations for training and testing purposes. It is easier, and usually less expensive, to consolidate and store all this data centrally. Also, It is less expensive to provision advanced analytics and BI platforms in the cloud or at one or two central corporate sites than to license them for multiple distributed locations.
The second stage — calculating information for operational decision making — may run either at the edge or centrally in the cloud or a corporate data center. Analytics are run centrally if they support strategic, tactical or operational activities that will be carried out at corporate headquarters, at another edge location, or at a business partner’s or customer’s site.
Distribute the Runtime Portion of Locally Focused IoT Analytics to Edge Sites
Some IoT analytics applications need to be distributed, so that processing can take place in devices, control systems, servers or smart routers at the sites where sensor data is generated. This makes sure the edge location stays in operation even when the corporate cloud service is down. Also, wide-area communication is generally too slow for analytics that support time-sensitive industrial control systems.
Thirdly, transmitting all sensor data to a corporate or cloud data center may be impractical or impossible if the volume of data is high or if reliable, high-bandwidth networks are unavailable. It is more practical to filter, condition and do analytic processing partly or entirely at the site where the data is generated.
Train Analytics Staff and Acquire Software Tools to Address Gaps in IoT-Related Analytics Capabilities
Most IoT analytical applications use the same advanced analytics platforms, data discovery tools as other kinds of business application. The principles and algorithms are largely similar. Graphical dashboards, tabular reports, data discovery, regression, neural networks, optimization algorithms and many other techniques found in marketing, finance, customer relationship management and advanced analytics applications also provide most aspects of IoT analytics.
However, a few aspects of analytics occur much more often in the IoT than elsewhere, and many analytics professionals have limited or no expertise in these. For example, some IoT applications use event stream processing platforms to process sensor data in near real time. Event streams are time series data, so they are stored most efficiently in databases (typically column stores) that are designed especially for this purpose, in contrast to the relational databases that dominate traditional analytics. Some IoT analytics are also used to support decision automation scenarios in which an IoT application generates control signals that trigger actuators in physical devices — a concept outside the realm of traditional analytics.
In many cases, companies will need to acquire new software tools to handle these requirements. Business analytics teams need to monitor and manage their edge analytics to ensure they are running properly and determine when analytic models should be tuned or replaced.
Increased Growth, if not Competitive Advantage
The huge volume and velocity of data in IoT will undoubtedly put new levels of strain on networks. The increasing number of real-time IoT apps will create performance and latency issues. It is important to reduce the end-to-end latency among machine-to-machine interactions to single-digit milliseconds. Following the best practices of implementing IoT analytics will ensure judo strategy of increased effeciency output at reduced economy. It may not be suffecient to define a competitive strategy, but as more and more players adopt IoT as a mainstream, the race would be to scale and grow as quickly as possible.