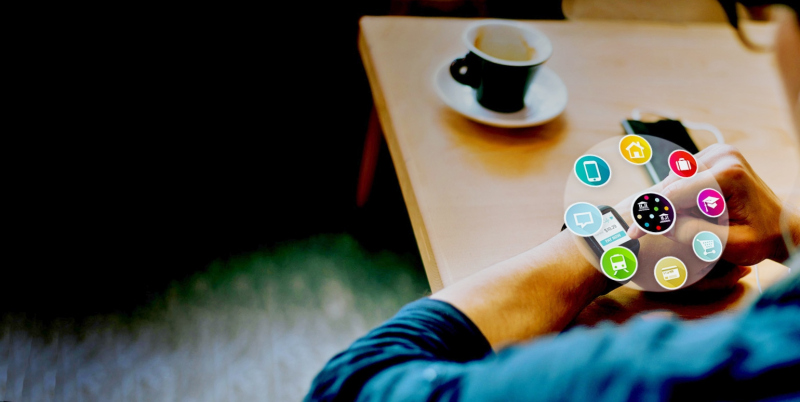
ACCELERATED DECISION MAKING AMPLIFIED BY REAL TIME ANALYTICS – A PERSPECTIVE
Add Your Heading Text Here
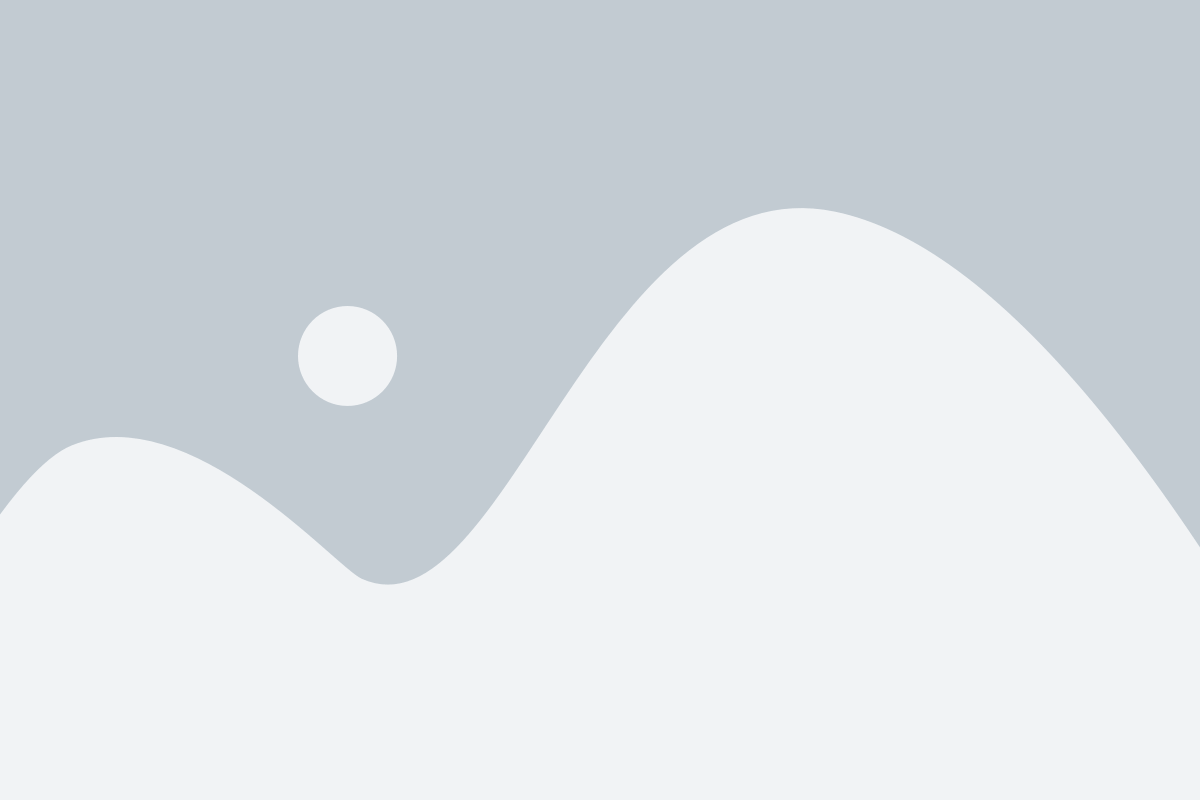
Companies are using more real-time analytics, because of the pressure to increase the speed and accuracy of business processes — particularly for digital business and the Internet of Things (IoT). Although data and analytics leaders intuitively understand the value of fast analytical insights, many are unsure how to achieve them.
Every large company makes thousands of real-time decisions each minute. For example, when a potential customer calls the contact center or visits the company’s website to gather product information, the company has a few seconds to figure out the best-next-action offer to propose to maximize the chance of making a sale. Or, when a customer presents a credit card to buy something or submits a withdrawal transaction request to an automated teller machine, a bank has one second or less to determine if the customer is who they say they are and whether they are likely to pay the bill when it is due. Of course, not all real-time decisions are tied to customers. Companies also make real-time decisions about internal operations, such as dynamically rerouting delivery trucks when a traffic jam forms; calling in a mechanic to replace parts in a machine when it starts to fail; or adjusting their manufacturing schedules when incoming materials fail to arrive on time.
Many decisions will be made in real time, regardless of whether real-time analytics are available, because the world is event-driven and the company has to respond immediately as events unfold. Improved real-time responses that are informed by fact-based, real-time analytics are optional, but clearly desirable.
Real-time analytics can be confusing, because different people may be thinking of different concepts when they use the term “real time.” Moreover, it isn’t always simple to determine where real-time analytics are appropriate, because the “right time” for analytics in a given business situation depends on many considerations; real-time is not always appropriate, or even possible. Finally, data and analytics leaders and their staff typically know less about real-time analytics than about traditional business intelligence and analytics.
Find the certain concept of “Real Time” Relevant to Your Business Problem
Real-time analytics is defined as “the discipline that applies logic and mathematics to data to provide insights for making better decisions quickly.” Real time means different things to different people.
When engineers say “real time” they mean that a system will always complete the task within a specified time frame.
Each component and subtask within the system is carefully designed to provide predictable performance, avoiding anything that could take longer to occur than is usually the case. Real-time systems prevent random delays, such as Java garbage collection, and may run on real-time operating systems that avoid nondeterministic behavior in internal functions such as scheduling and dispatching. There is an implied service-level agreement or guarantee. Strictly speaking, a real-time system could take hours or more to do its work, but in practice, most real-time systems act in seconds, milliseconds or even microseconds.
The concept of engineering real time is most relevant when dealing with machines and fully automated applications that require a precise sequence and timing of interactions among multiple components. Control systems for airplanes, power plants, self-driving cars and other machines often use real-time design. Time-critical software applications, such as high-frequency trading (HFT), also leverage engineering real-time concepts although they may not be entirely real time.
Use Different Technology and Design Patterns for Real-Time Computation Versus Real-Time Solutions
Some people use the term real-time analytics to describe fast computation on historical data from yesterday or last year. It’s obviously better to get the answer to a query, or run a model, in a few seconds or minutes (business real time) rather than waiting for a multihour batch run. Real-time computation on small datasets is executed in-memory by Excel and other conventional tools. Real-time computation on large datasets is enabled by a variety of design patterns and technologies, such as:
- Preloading the data into an in-memory database or in-memory analytics tool with large amounts of memory
- Processing in parallel on multiple cores and chips
- Using faster chips or graphics processing units (GPUs)
- Applying efficient algorithms (for example, minimizing context switches)
- Leveraging innovative data architectures (for example, hashing and other kinds of encoding)
Most of these can be hidden within modern analytics products so that the user does not have to be aware of exactly how they are being used.
Real-time computation on historical data is not sufficient for end-to-end real-time solutions that enable immediate action on emerging situations. Analytics for real-time solutions requires two additional things:
- Data must be real time (current)
- Analytic logic must be predefined
If conditions are changing from minute to minute, a business needs to have situation awareness of what is happening right now. The decision must reflect the latest sensor readings, business transactions, web logs, external market data, social computing postings and other current information from people and things.
Real-time solutions use design patterns that enable them to access the input data quickly so that data movement does not become the weak link in the chain. There is no time to read large amounts of data one row or message at a time across a wide-area network. Analytics are run as close as possible to where the data is generated. For example, IoT applications run most real-time solutions on or near the edge, close to the devices. Also, HFT systems are co-located with the stock exchanges to minimize the distance that data has to travel. In some real-time solutions, including HFT systems, special high-speed networks are used to convey streaming data into the system.
Match the Speed of Analytics to the Speed of the Business Decision
Decisions have a range of natural timing, so “right time” is not always real time. Business analysts and solution architects should work with managers and other decision makers to determine how fast to make each decision. The two primary considerations are:
- How quickly will the value of the decision degrade?
- Decisions should be executed in real time if a customer is waiting on the phone for an answer; resources would be wasted if they sit idle; fraud would succeed; or physical processes would fail if the decision takes more than a few milliseconds or minutes. On the other hand, a decision on corporate strategy may be nearly as valuable in a month as it would be today, because its implementation will take place over months and years so starting a bit earlier may not matter much.
- How much better will a decision be if more time is spent?
- Simple, well-understood decisions on known topics, and for which data is readily available, can be made quickly without sacrificing much quality.
Lastly, Automate Decisions if Algorithms Can Represent the Entire Decision Logic
Algorithms offers the “last mile” of the decision. However, automating algorithms requires a well described process to code against. According to Gartner, “Decision automation is possible only when the algorithms associated with the applicable business policies can be fully defined.”
Final Word
Performing some analytics in real time is a goal in many analytics and business intelligence modernization programs. To operate in real time, data and analytics leaders must leverage predefined analytical models, rather than ad hoc models, and use current input data rather than just historical data.
Related Posts
AIQRATIONS
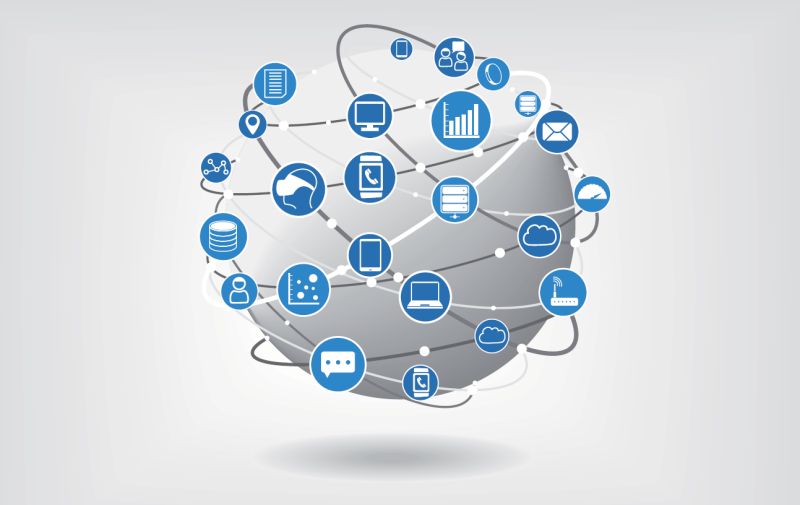
THE BEST PRACTICES FOR INTERNET OF THINGS ANALYTICS
Add Your Heading Text Here
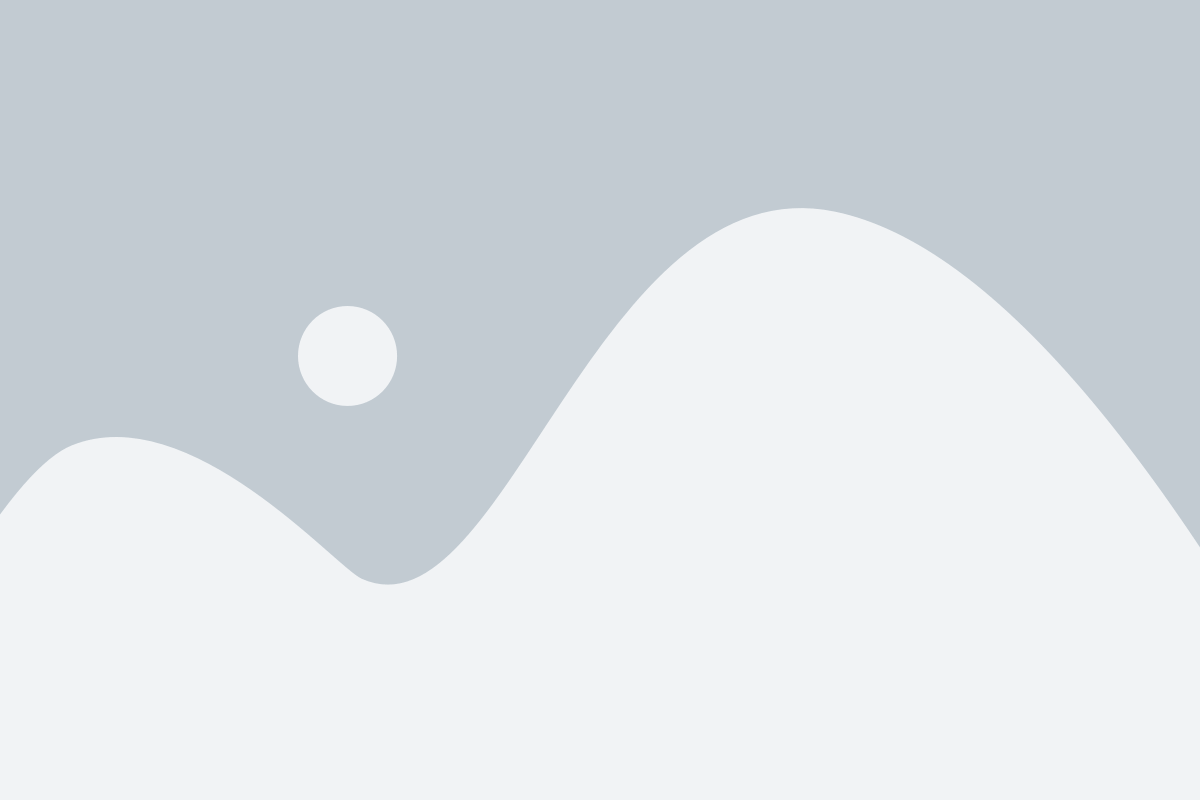
In most ways, Internet of Things analytics are like any other analytics. However, the need to distribute some IoT analytics to edge sites, and to use some technologies not commonly employed elsewhere, requires business intelligence and analytics leaders to adopt new best practices and software.
There are certain prominent challenges that Analytics Vendors are facing in venturing into building a capability. IoT analytics use most of the same algorithms and tools as other kinds of advanced analytics. However, a few techniques occur much more often in IoT analytics, and many analytics professionals have limited or no expertise in these. Analytics leaders are struggling to understand where to start with Internet of Things (IoT) analytics. They are not even sure what technologies are needed.
Also, the advent of IoT also leads to collection of raw data in a massive scale. IoT analytics that run in the cloud or in corporate data centers are the most similar to other analytics practices. Where major differences appear is at the “edge” — in factories, connected vehicles, connected homes and other distributed sites. The staple inputs for IoT analytics are streams of sensor data from machines, medical devices, environmental sensors and other physical entities. Processing this data in an efficient and timely manner sometimes requires event stream processing platforms, time series database management systems and specialized analytical algorithms. It also requires attention to security, communication, data storage, application integration, governance and other considerations beyond analytics. Hence it is imperative to evolve into edge analytics and distribute the data processing load all across.
Hence, some IoT analytics applications have to be distributed to “edge” sites, which makes them harder to deploy, manage and maintain. Many analytics and Data Science practitioners lack expertise in the streaming analytics, time series data management and other technologies used in IoT analytics.
Some visions of the IoT describe a simplistic scenario in which devices and gateways at the edge send all sensor data to the cloud, where the analytic processing is executed, and there are further indirect connections to traditional back-end enterprise applications. However, this describes only some IoT scenarios. In many others, analytical applications in servers, gateways, smart routers and devices process the sensor data near where it is generated — in factories, power plants, oil platforms, airplanes, ships, homes and so on. In these cases, only subsets of conditioned sensor data, or intermediate results (such as complex events) calculated from sensor data, are uploaded to the cloud or corporate data centers for processing by centralized analytics and other applications.
The design and development of IoT analytics — the model building — should generally be done in the cloud or in corporate data centers. However, analytics leaders need to distribute runtime analytics that serve local needs to edge sites. For certain IoT analytical applications, they will need to acquire, and learn how to use, new software tools that provide features not previously required by their analytics programs. These scenarios consequently give us the following best practices to be kept in mind:
Develop Most Analytical Models in the Cloud or at a Centralized Corporate Site
When analytics are applied to operational decision making, as in most IoT applications, they are usually implemented in a two-stage process – In the first stage, data scientists study the business problem and evaluate historical data to build analytical models, prepare data discovery applications or specify report templates. The work is interactive and iterative.
A second stage occurs after models are deployed into operational parts of the business. New data from sensors, business applications or other sources is fed into the models on a recurring basis. If it is a reporting application, a new report is generated, perhaps every night or every week (or every hour, month or quarter). If it is a data discovery application, the new data is made available to decision makers, along with formatted displays and predefined key performance indicators and measures. If it is a predictive or prescriptive analytic application, new data is run through a scoring service or other model to generate information for decision making.
The first stage is almost always implemented centrally, because Model building typically requires data from multiple locations for training and testing purposes. It is easier, and usually less expensive, to consolidate and store all this data centrally. Also, It is less expensive to provision advanced analytics and BI platforms in the cloud or at one or two central corporate sites than to license them for multiple distributed locations.
The second stage — calculating information for operational decision making — may run either at the edge or centrally in the cloud or a corporate data center. Analytics are run centrally if they support strategic, tactical or operational activities that will be carried out at corporate headquarters, at another edge location, or at a business partner’s or customer’s site.
Distribute the Runtime Portion of Locally Focused IoT Analytics to Edge Sites
Some IoT analytics applications need to be distributed, so that processing can take place in devices, control systems, servers or smart routers at the sites where sensor data is generated. This makes sure the edge location stays in operation even when the corporate cloud service is down. Also, wide-area communication is generally too slow for analytics that support time-sensitive industrial control systems.
Thirdly, transmitting all sensor data to a corporate or cloud data center may be impractical or impossible if the volume of data is high or if reliable, high-bandwidth networks are unavailable. It is more practical to filter, condition and do analytic processing partly or entirely at the site where the data is generated.
Train Analytics Staff and Acquire Software Tools to Address Gaps in IoT-Related Analytics Capabilities
Most IoT analytical applications use the same advanced analytics platforms, data discovery tools as other kinds of business application. The principles and algorithms are largely similar. Graphical dashboards, tabular reports, data discovery, regression, neural networks, optimization algorithms and many other techniques found in marketing, finance, customer relationship management and advanced analytics applications also provide most aspects of IoT analytics.
However, a few aspects of analytics occur much more often in the IoT than elsewhere, and many analytics professionals have limited or no expertise in these. For example, some IoT applications use event stream processing platforms to process sensor data in near real time. Event streams are time series data, so they are stored most efficiently in databases (typically column stores) that are designed especially for this purpose, in contrast to the relational databases that dominate traditional analytics. Some IoT analytics are also used to support decision automation scenarios in which an IoT application generates control signals that trigger actuators in physical devices — a concept outside the realm of traditional analytics.
In many cases, companies will need to acquire new software tools to handle these requirements. Business analytics teams need to monitor and manage their edge analytics to ensure they are running properly and determine when analytic models should be tuned or replaced.
Increased Growth, if not Competitive Advantage
The huge volume and velocity of data in IoT will undoubtedly put new levels of strain on networks. The increasing number of real-time IoT apps will create performance and latency issues. It is important to reduce the end-to-end latency among machine-to-machine interactions to single-digit milliseconds. Following the best practices of implementing IoT analytics will ensure judo strategy of increased effeciency output at reduced economy. It may not be suffecient to define a competitive strategy, but as more and more players adopt IoT as a mainstream, the race would be to scale and grow as quickly as possible.