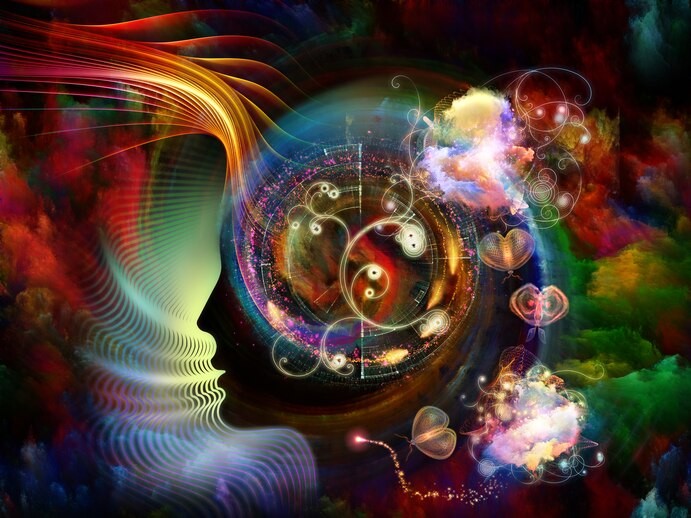
Business Decisions Transformation : AI meets Behavioral Sciences : New Strategic Approach
Add Your Heading Text Here
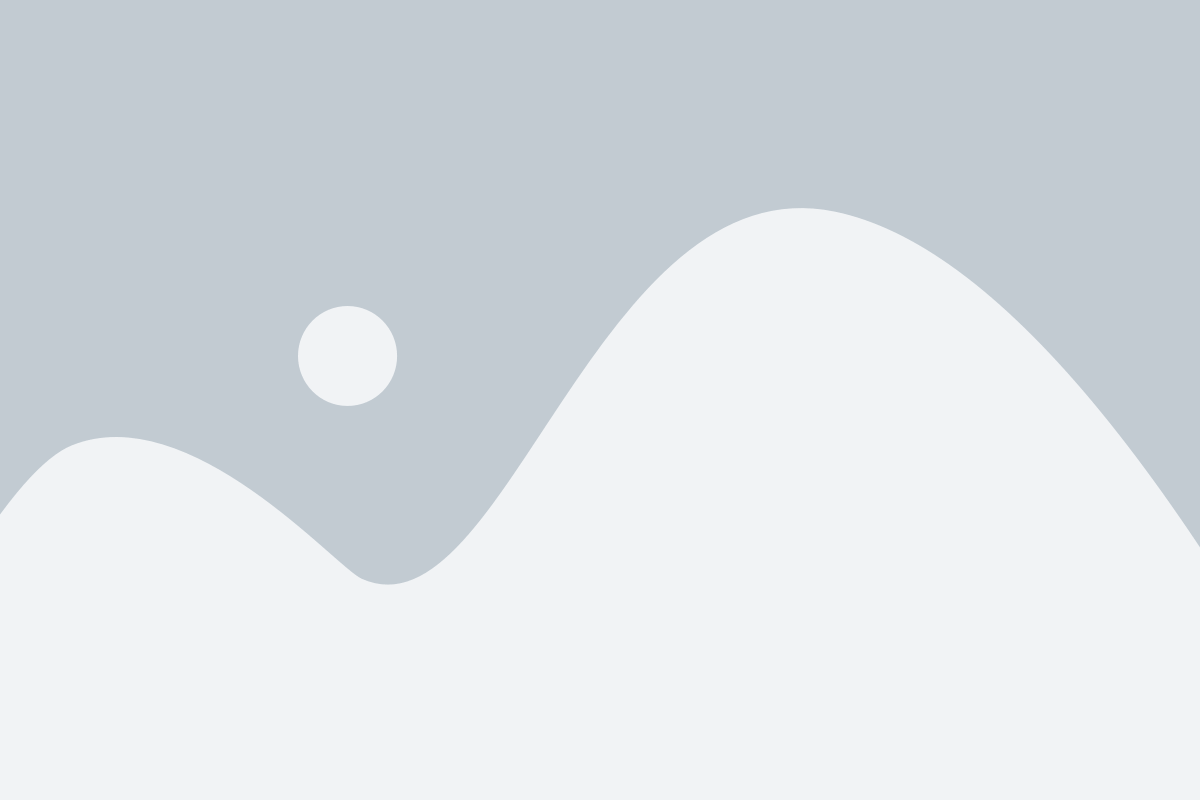
Ascendancy of artificial intelligence (AI) revolution has been made possible by the machine enabled , ensemble configured algorithm revolution. The machine learning algorithms researchers have been developing for decades, when cleverly applied to today’s web-scale data sets, can yield surprisingly good forms of intelligence. For instance, the United States Postal Service has long used neural network models to automatically read handwritten zip code digits. Today’s deep learning neural networks can be trained on millions of electronic photographs to identify faces, and similar algorithms may increasingly be used to navigate automobiles and identify tumors in X-rays.
But current AI technologies are a collection of big data-driven point solutions, and algorithms are reliable only to the extent that the data used to train them is complete and appropriate. One-off or unforeseen events that humans can navigate using common sense can lead algorithms to yield nonsensical outputs.
Design thinking is defined as human-centric design that builds upon the deep understanding of our users (e.g., their tendencies, propensities, inclinations, behaviors) to generate ideas, build prototypes, share what you’ve made, embrace the art of failure (i.e., fail fast but learn faster) and eventually put your innovative solution out into the world. And fortunately for us humans (who really excel at human-centric things), there is a tight correlation between the design thinking and artificial intelligence.
Artificial intelligence technologies could reshape economies and societies, but more powerful algorithms do not automatically yield improved business or societal outcomes. Human-centered design thinking can help organizations get the most out of cognitive technologies.
Divergence from More Powerful Intelligence To More Creative Intelligence
Whilst algorithms can automate many routine tasks, the narrow nature of data-driven AI implies that many other tasks will require human involvement. In such cases, algorithms should be viewed as cognitive tools capable of augmenting human capabilities and integrated into systems designed to go with the grain of human—and organizational—psychology. We don’t want to ascribe to AI algorithms more intelligence than is really there. They may be smarter than humans at certain tasks, but more generally we need to make sure algorithms are designed to help us, not do an end run around our common sense.
Design Thinking at Enterprise Premise
Although cognitive design thinking is in its early stages in many enterprises, the implications are evident. Eschewing versus embracing design thinking can mean the difference between failure and success. For example, a legacy company that believes photography hinges on printing photographs could falter compared to an internet startup that realizes many customers would prefer to share images online without making prints, and embraces technology that learns faces and automatically generates albums to enhance their experience.
To make design thinking meaningful for consumers, companies can benefit from carefully selecting use cases and the information they feed into AI technologies. In determining which available data is likely to generate desired results, enterprises can start by focusing on their individual problems and business cases, create cognitive centers of excellence, adopt common platforms to digest and analyze data, enforce strong data governance practices, and crowd source ideas from employees and customers alike.
In assessing what constitutes proper algorithmic design, organizations may confront ethical quandaries that expose them to potential risk. Unintended algorithmic bias can lead to exclusionary and even discriminatory practices. For example, facial recognition software trained on insufficiently diverse data sets may be largely incapable of recognizing individuals with different skin tones. This could cause problems in predictive policing, and even lead to misidentification of crime suspects. If the training data sets aren’t really that diverse, any face that deviates too much from the established norm will be harder to detect. Accordingly, across many fields, we can start thinking about how we create more inclusive code and employ inclusive coding practices.
CXO Strategy for Cognitive Design Thinking & Behavioral Science
CIOs can introduce cognitive design thinking to their organizations by first determining how it can address problems that conventional technologies alone cannot solve. The technology works with the right use cases, data, and people, but demonstrating value is not always simple. However, once CIOs have proof points that show the value of cognitive design thinking, they can scale them up over time.
CIOs benefit from working with business stakeholders to identify sources of value. It is also important to involve end users in the design and conception of algorithms used to automate or augment cognitive tasks. Make sure people understand the premise of the model so they can pragmatically balance algorithm results with other information.
Enterprise Behavioral Science – From Insights to Influencing Business Decisions
Every January, how many people do you know say that they want to resolve to save more, spend less, eat better, or exercise more? These admirable goals are often proclaimed with the best of intentions, but are rarely achieved. If people were purely logical, we would all be the healthiest versions of ourselves.
However, the truth is that humans are not 100% rational; we are emotional creatures that are not always predictable. Behavioral economics evolved from this recognition of human irrationality. Behavioral economics is a method of economic analysis that applies psychological insights into human behavior to explain economic decision-making.
Decision making is one of the central activities of business – hundreds of billions of decisions are made every day. Decision making sits at the heart of innovation, growth, and profitability, and is foundational to competitiveness. Despite this degree of importance, decision making is poorly understood, and badly supported by tools. A study by Bain & Company found that decision effectiveness is 95% correlated with companies’ financial performance.
Enterprise Behavioral Science is not only about understanding potential outcomes, but to completely change outcomes, and more specifically, change the way in which people behave. Behavioral Science tells us that to make a fundamental change in behavior that will affect the long-term outcome of a process, we must insert an inflection point.
As an example, you are a sales rep and two years ago your revenue was $1 million. Last year it was $1.1 million, and this year you expect $1.2 million in sales. The trend is clear, and your growth has been linear and predictable. However, there is a change in company leadership and your management has increased your quota to $2 million for next year. What is going to motivate you to almost double your revenues? The difference between expectations ($2 million) and reality ($1.2 million) is often referred to as the “behavioral gap” . When the behavioral gap is significant, an inflection point is needed to close that gap. The right incentive can initiate an inflection point and influence a change in behavior. Perhaps that incentive is an added bonus, President’s Club eligibility, a promotion, etc.
Cognitive Design Thinking – The New Indispensable Reskilling Avenue
Artificial intelligence, machine learning, analytics and mobile and cloud engineering will be the top technology areas where the need for re-skilling will be the highest.whilst there is a high probability that machine learning and artificial intelligence will play an important role in whatever job you hold in the future, there is one way to “future-proof” your career…embrace the power of design thinking & behavioral science.
In fact, integrating design thinking , behavioral science and artificial intelligence can give you “immense synergies ” that future-proof whatever career you decide to pursue. To meld these three disciplines together, one must:
Understand where and how artificial intelligence and behavioral science can impact your business initiatives. While you won’t need to write machine learning algorithms, business leaders do need to learn how to “Think like a data scientist” in order understand how AI can optimize key operational processes, reduce security and regulatory risks, uncover new monetization opportunities.
Understand how design thinking techniques, concepts and tools can create a more compelling and emphatic user experience with a “delightful” user engagement through superior insights into your customers’ usage objectives, operating environment and impediments to success.
Design thinking & Behavioral Science is a mindset. IT firms are trying to move up the curve. Higher-end services that companies can charge more is to provide value and for that you need to know that end-customers’ needs. For example, to provide value services to banking customers is to find out what the bank’s customer needs are in that country the banking client is based. Latent needs come from a design thinking philosophy where you observe customer data, patterns and provide a solution that the customer does not know. Therefore, Companies will hire design thinkers as they can predict what the consumer does not know and hence charge for the product/service from their clients. Idea in design thinking is to provide agile product creation or solutions.
Without Design Thinking & Behavioral Science, AI Will be Only an Incremental Value
Though organizations understand the opportunity that big data presents, many struggles to find a way to unlock its value and use it in tandem with design thinking – making “AI an colossal waste of time & money.” Only by combining quantitative insights gathered using AI, machine/deep learning, and qualitative research through behavioral science, and finally design thinking to uncover hidden patterns and leveraging it to understand what the customer would want, will we be able to paint a complete picture of the problem at hand, and help drive towards a solution that would create value for all stakeholders.
(AIQRATE, A bespoke global AI advisory and consulting firm. A first in its genre, AIQRATE provides strategic AI advisory services and consulting offerings across multiple business segments to enable clients navigate their AI powered transformation, innovation & revival journey and accentuate their decision making and business performance.
AIQRATE works closely with Boards, CXOs and Senior leaders advising them on their Analytics to AI journey construct with the art of possible roadmap blended with a jump start to AI driven transformation with AI@scale centric strategy followed by consulting them on embedding AI as core to business strategy within business functions and augmenting the decision-making capabilities with AI. Our bespoke AI advisory services focuses on curating & designing building blocks of AI strategy, embed AI@scale interventions and create AI powered organizations.
AIQRATE’s path breaking 50+ AI consulting frameworks, methodologies, primers, toolkits and playbooks crafted by seasoned and proven AI strategy advisors enable Indian & global enterprises, GCCs, Startups, SMBs, VC/PE firms, and Academic Institutions enhance business performance & ROI and accelerate decision making capability.
Visit www.aiqrate.ai to experience our AI advisory services & consulting offerings. Write to us :consult@aiqrate.ai . Follow us on Linkedin | Facebook | Twitter | Instagram )
Related Posts
AIQRATIONS

3 Ways To Human Centric AI
Add Your Heading Text Here
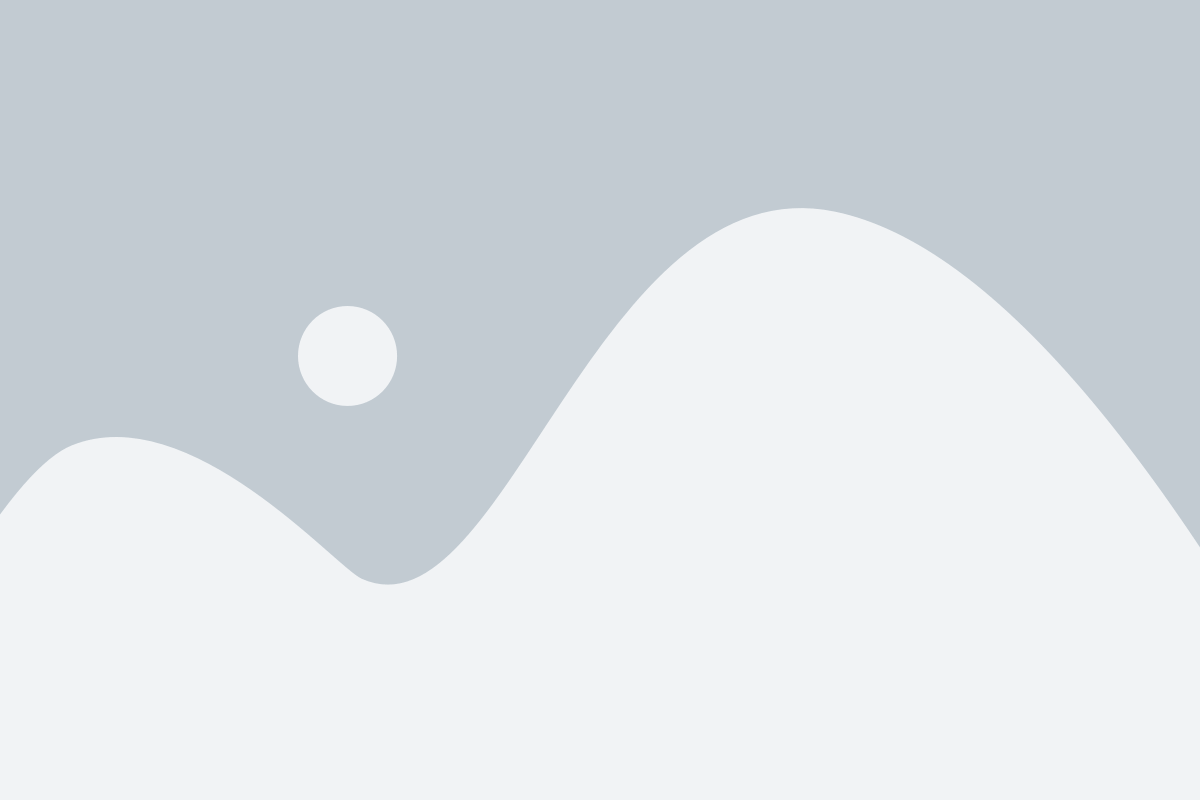
Three Ways to Facilitate a Symbiotic Relationship Between Cognitive Intelligence and Behavioral Sciences
After every conference I speak at about the transformative power of Artificial Intelligence and its potential to unlock untold business value, the one question that often crops up from the audience is.. if AI is expected to perform much of the grunt work in the enterprise world, what scope is there for the so-called ‘human’ qualities?
Is the future of business and technology so deeply intertwined that it leaves virtually no scope in the future for the vagaries of human intelligence and behavior? The answer is quick and simple – absolutely not. Artificial Intelligence, while a great paradigm-shifter in the world of business, is still one of the tools that will be used by humans for making better decisions. At the end of the day, AI will still be developed and used by humans. And maintaining the ‘human element’ in the way it is made, delivered, used and improved will most certainly make it a lot more successful. AI exists to make human life simpler and richer – and hence it is critical that AI practitioners and data scientists adopt a human-centric approach to its development, deployment and adoption. Even the best AI will become quickly redundant without inputs from real humans on how to accelerate strategic decisions and processes.
How do we then build in that ‘human element’ into our Artificial Intelligence tools? This is where humanities-centric subjects of design and behavioral science come into the picture. What is behavioral science? Simply put, it is the study of internal cognitive processes of humans and societies and how these processes manifest into external perceptible and imperceptible actions and interactions. Behavioral science typically stands at a nexus of various subjects – borrowing aspects from sociology, anthropology, psychology and even economics and political science. Its interdisciplinary nature precludes the scale of impact it can have if applied correctly. In technology, and specifically in AI, behavioral science will and should impact how we build, use and interact with technology.
I see primarily three key areas where the symbiosis of AI-led cognitive intelligence systems and behavioral science can unlock massive value for enterprises that marry these two starkly different, but extremely complementary fields of study:
Appeal to the Non-Conscious
We have known for nearly a century now that a large majority of human biases, inferences, preferences and reactions are largely controlled by the dark recesses of our non-conscious brain. For technologists to build successful AI products, that are widely adopted and used they need to reach out inside the non-conscious parts of the human brain and orchestrate responses from there.
In AI technology specifically, user adoption is often the difference between make and break for products. Numerous AI products are mostly informed by the data they gather from human actions and their preferences. This data feeds the algorithms running in the background and makes them more sophisticated to better understand their human overlords. To that end, AI products need to have a strong underpinning in behavioral science, so that they can appeal to the non-conscious and improve adoption.
Take for example the work done by Nir Eyal for his book, ‘Hooked: How to Build Habit Forming Products.’ In the book, Eyal writes about multiple ways in which human subjects get applied to technology development. One of them is the Hook canvas – a loop comprising triggers, actions, rewards and investments – which are the cornerstone features of every addictive software you’ve used – from Facebook to Instagram, Snapchat and YouTube. Another is the idea of using the trinity of emotion, features and incentives – extremely relevant ideas to anyone working on building AI products. Another example comes from Worxogo – an Indian startup that employs behavioral design, neuroscience in tandem with predictive analytics to enhance employee performance through nudges to the non-conscious.
Build with Humans
Not only is AI built to serve humans, it is also built by humans. To that end, it becomes extremely important to consider what emotional triggers help define what we build and how we build it. Again, behavioral science practitioners have a key role to play in order to engage empathy in defining the requirements and going about the development of AI. Learnings from behavioral science can bring to light immeasurably important interventions for how we manage and lead teams, collaborate between a team and across multiple teams – all while maintaining a high level of motivation by appealing to a higher sense of purpose. It is worth examining how something as simple as empathy can be extremely valuable in how we build software. For instance, with improved self-awareness and empathy, developers can feel an intrinsic desire to write cleaner code while maintaining proper documentation. Also, given that AI is largely deployed using the DevOps methodology – empathy can be the difference between whether we can build a trust-based bridge between how we build, deploy and automate releases faster.
Beyond the ‘how’ of AI development, behavioral science can also contribute meaningfully to the ‘what’. Currently a lot of concern around AI is related to ethics – will AI lead to loss of meaningful work for humans? What data privacy issues can rear their head when we deploy large-scale data capture systems to improve our algorithms? We need to move the dial from apathy to empathy in the process of conceptualizing software – and knowledge of behavioral science will undoubtedly help AI practitioners develop more responsible AI.
Artificial Emotional Intelligence
The third key application of behavioral science – and possibly the most game-changing of the lot is – how can we apply behavioral science to make our systems more ‘human’? Is it possible to add a dash of EQ to these high IQ systems?
I certainly think there is a huge scope for developing AI that has a strong human bent. Consider the applications we are building today with AI and robotics – companions for the elderly, coaching apps for autistic children, even something as comparatively mundane is chatbots for customer service. Behavioral science holds the key to achieving the holy grail of how we can better balance the human-machine equation, by infusing human qualities into artificial systems.
To enable this, it is important to know who we are building for and what are their intrinsic and non-conscious needs. Behavioral science holds the clues that can complement AI’s ability to eliminate biases, while serving the emotional needs of humans. For example, StressSense tracks when people are highly stressed and helps them avoid anxious situations. This kind of breakthrough research can help in multiple AI applications, teaching them how to behave with humans, while ensuring a strong impact.
As technology providers and businesses work together to build transformational artificial intelligence systems and data science teams, it is very important to consider the human element. These teams would do well to develop a better understanding of whom the AI is built for and how it is used – through techniques offered by behavioral science. Balancing the human-machine equation and powering a complementary relationship between AI systems and the people who use them necessitates an infusion of behavioral science into the process. Ultimately, for AI to succeed, we need both – the foresight of technology as well as the insight of humans.
Related Posts
AIQRATIONS
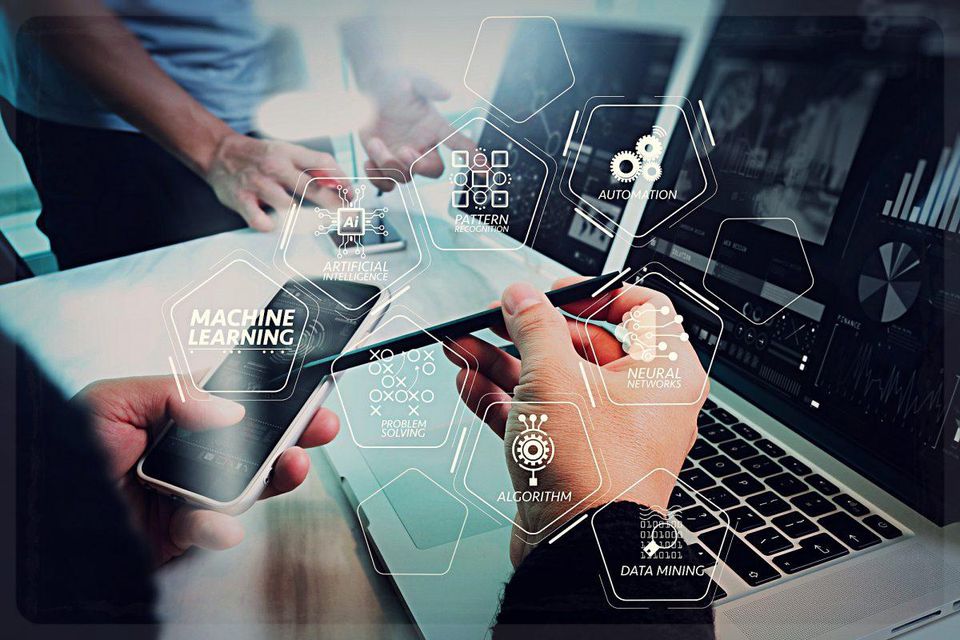
Reimagining Enterprise Decision-Making With Artificial Intelligence
Add Your Heading Text Here
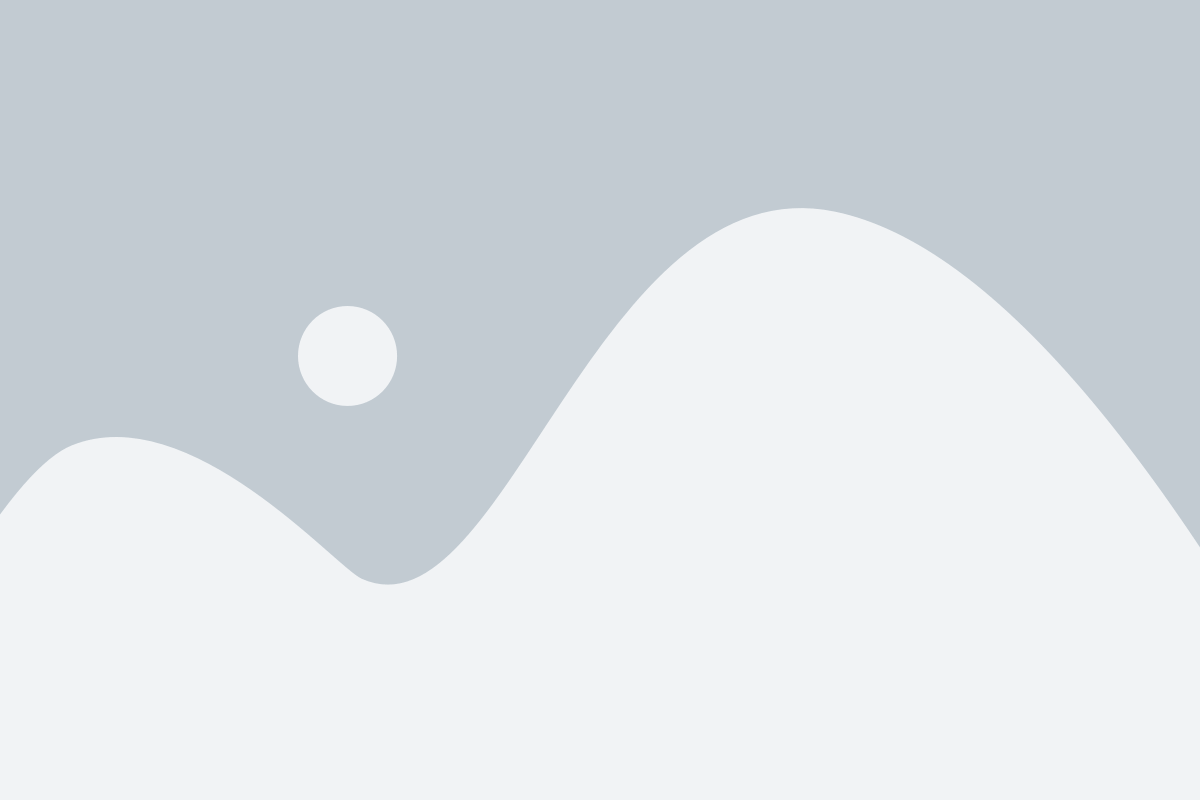
Artificial Intelligence will deliver revolutionary impact on how enterprises make decisions today. In the last few years alone, we have rapidly moved beyond heuristics-based decision-making to analytics-driven decision-support. In the VUCA phase, businesses globally are now pivoting to an AI-led, algorithm-augmented style of decision-making. With huge computing power and ever-increasing data storage and analytics prowess, we are entering a new paradigm, a probable and interesting scenario wherein, Artificial Intelligence will play a huge role in augmenting human intelligence and enabling decision-making with complete autonomy. The big hope is that this new paradigm will not only reduce human biases and errors that are common with heuristic decisions, but also reduce the time involved in making these critical decisions.
Here, I’ll attempt to focus on how we moved from simpler data driven decision-support to AI-powered decisions. The evolution of this technology has been breathtaking to behold and just might provide clues as to what we can expect in the future. Further, I’ll cover a few critical aspects that need to be inculcated by organizations on the AI transformation journey, and provide a few insightful cues that will make this journey exciting and fruitful.
Transformation of Decision-Making: From Analytics to AI
First, let us look at how we got here. Some truly pathbreaking events happened along the way while we were trying to make more accurate business decisions, leading us to reimagine how decisions will be made in the enterprise.
Organizations are Becoming Math Houses
With data deluge and digital detonation, combined with the appreciation of the fact that robust analytical capabilities lead to more informed decisions, we are witnessing AI savvy organizations rapidly maturing into ‘math houses.’ Data science – the ability to extract meaningful insights out of data has become de rigueur. Why? Because we now know that data, when seen in isolation, is inherently dumb. It is the ability to process this data and identify patterns and anomalies – using sophisticated algorithms and ensemble techniques – that makes all the difference. These self-intuitive algorithms are where real value resides – as they define the intelligence required to uncover insights and make smart recommendations. Organizations today are evolving into algorithm factories. There is a real understanding today that by enabling continuous advancement in mathematical algorithms, we can deliver consistent decisions based on prescribed as well as evolving business rules.
It is now an established reality that companies with robust mathematical capabilities possess a huge advantage over those that don’t. Indeed, it’s this math-house orientation that separates companies like Amazon and Google from the ones they leave in their wake, with their ability to understand their customers better, identify anomalies and recognize key patterns.
AI: From Predictive to Prescriptive
We saw a similar evolution in the age of analytics – wherein the science and value veered from descriptive analytics, providing diagnostics of past events to prescriptive analytics, helping see and shape the future. We are seeing a similar evolution in how AI gets leveraged in the enterprise and where its maximum value lies.
In early implementations, it was common to see AI as just a tool to predict and forecast future conditions, while accounting for the dynamism seen in the external environment. Today, AI-enabled decision-making is more prescriptive, with AI providing enterprises not just a look into the future, but also key diagnostics and suggestions on potential decision options and their payoffs. Such evolved applications of AI can help businesses make decisions that can potentially exploit more business opportunities, while averting potential threats much earlier.
Mr. Algorithm to Drive Decision Making
The culmination of this AI-era advancement would be the introduction of smart algorithms in every walk of life and business. Algorithms will become further mainstream leading to what will be the most sweeping business change since the industrial revolution. Organizations – those that already aren’t – will start developing a suite of algorithmic IP’s that will de-bias most enterprise decisions.
If Mr. Algorithm is going to drive most enterprise decisions of tomorrow, we need to create some checks and balances to ensure that it does not go awry. It is more critical today than ever before that the algorithmic suite developed by enterprises has a strong grounding in ethics and can handle situations appropriately for which explicit training may not have been provided.
How to Enable this AI Era of Change
Ushering into an AI-centric era of decision-making will require organizational transformation from business, cultural and technical standpoints. The following facets will be the enablers of this change:
Developing an Engineering Mindset
Instrumenting AI in the enterprise requires a combination of data scientists and computer scientists. As AI matures in the enterprise, the users, use cases and data will increase exponentially. To deliver impactful AI applications, scale and extensibility is critically important. This is where having an engineering mindset comes in. Imbibing an engineering mindset will help standardize the use of these applications while ensuring that they are scalable and extensible.
Learning, Unlearning, Relearning
The other critical aspect to a culture where AI can thrive is creating an environment supporting continuous unlearning and relearning. AI can succeed if the people developing and operating it are rewarded for continuous experimentation and exploration. And just like AI, people should be encouraged to incorporate feedback loops and learn continuously. As technology matures it’s important that the existing workforce keeps up. For one, it’s critical that the knowledge of algorithm theory, applied math alongside training on AI library and developer tools, is imparted into the workforce – and is continuously updated to reflect new breakthroughs in this space.
Embedding Design-Thinking and Behavioral Science at the Center of this Transformation
Finally, given the nature of AI applications, it’s critical that they are consumed voraciously. User input very often activates the learning cycles of artificial intelligence applications. To ensure high usage of these applications, it’s very important that we put the user at the center while designing these applications. This is where the application of behavioral sciences and human-centered design will deliver impact. By imparting empathy in these applications for the user, we will be able to design better and more useful AI applications.
As we augment decision-making with algorithmic, AI-centered systems and platforms – the big expectation is that they will bring untold efficiencies in terms of cost, alongside improvement in the speed and quality with which decisions get made. It’s time to reimagine and deliver on enterprise decision-making that is increasingly shaped through artificial intelligence. These aspects – how the AI is progressing and how to exploit its potential are of paramount importance to keep in mind for an AI transformation.
Related Posts
AIQRATIONS
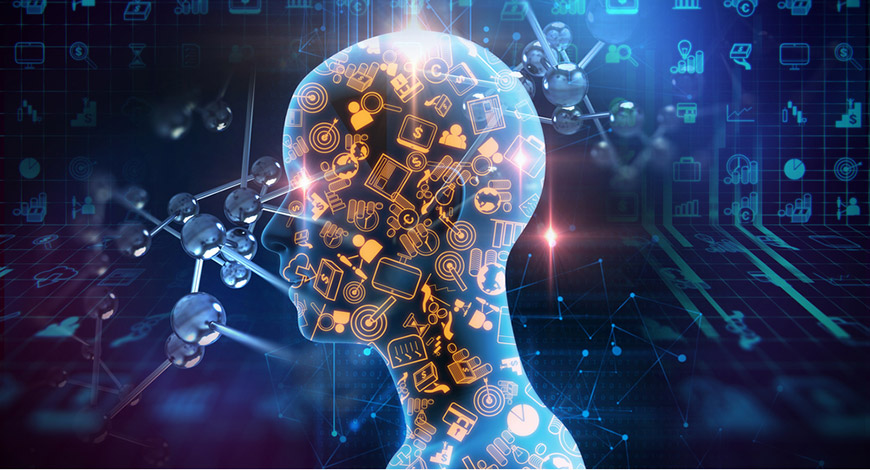
Training Programs Can Enhance The Skills And Employability Of The Existing IT Workforce
Add Your Heading Text Here
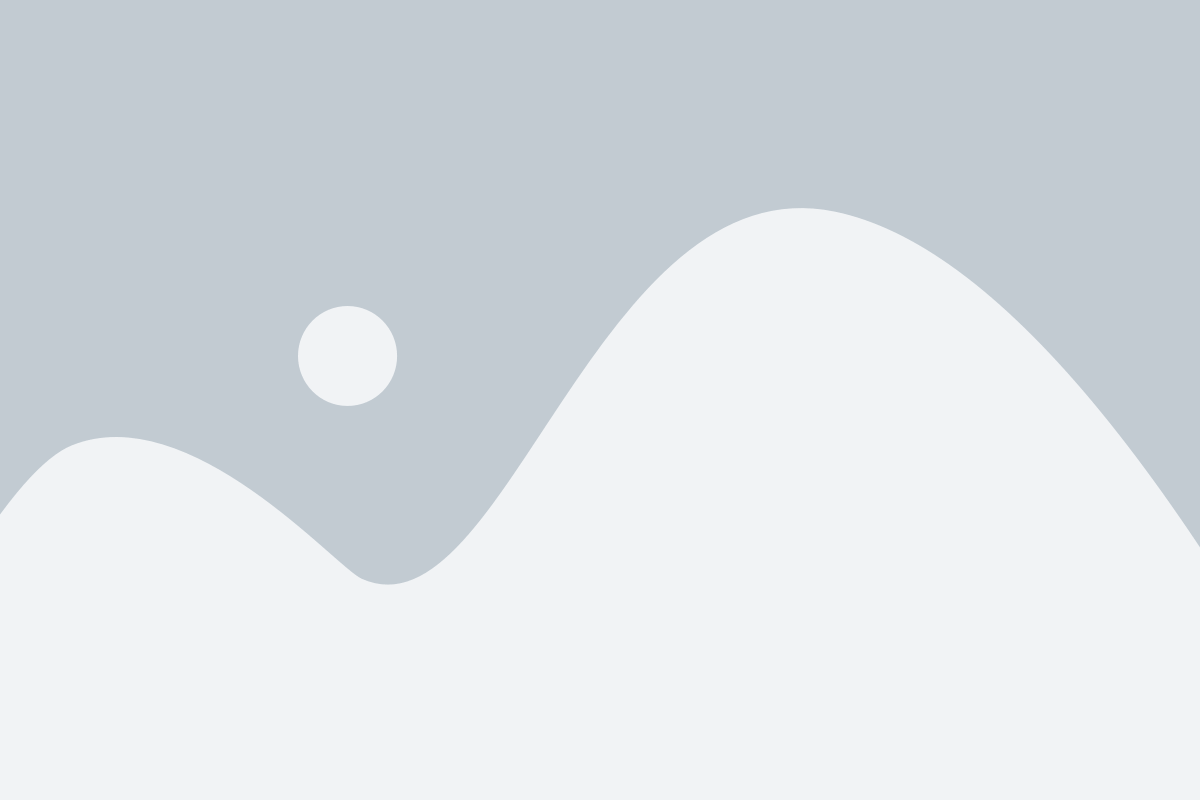
Several pieces of research, studies and content have been published about the exponential growth witnessed in emerging technologies – AI, blockchain, RPA, cybersecurity, IoT, AR/VR. There is no doubt that these are the emerging technologies of the future, which will help catapult the next growth spurt of enterprises. Professionals across the information technology landscape are queuing up to upskill, reskill themselves across these mentioned areas to continue to be relevant in the workforce for tomorrow. But for those who already have learning, skills and experience in these emerging technologies, how can they take their career to the next level?
The flux of deployment-ready and value-generating use cases across industries suggest that a cross-technology expertise across these emerging technology areas would be the next big source of career growth for incumbent professionals. We witnessed few years ago, software developers were keen to reinvent themselves as full-stack developers. High performing technologists wanted to develop proficiency across the software architecture and the development life-cycle – from database to UI, and from infrastructure set-up to deployment. Similarly, IT professionals today should seek the synergistic benefits of combining areas across emerging technologies. This article will focus on what emerging technology areas are being effectively combined by enterprises.
AI + Blockchain
Artificial intelligence is the set of technologies that help machines mimic human functions. Blockchain is the emergent technology paradigm that helps build a distributed, immutable sequence of financial events and transactions. With a strong uptick in the dispersion of blockchain use cases, enterprises are also looking for a robust way to surface potential fraud and other anomalous events in real-time. The events of fraud attempt and security threats to blockchain systems are often very high-speed and require immediate attention and analysis to ensure that the perceived anomalies are rooted out. Using AI, specifically machine learning, we can rapidly parse through a log of events to find anomalous situations and flag them off in real-time, protecting the integrity of the blockchain.
AI +IOT
Internet of Things is the network of physical devices that exchange data. This very definition makes the case for combining artificial intelligence and IOT plainly clear. IOT-enabled sensors usually are a source of multitudinous data – based on the use case employed – which is increasingly being sent to the central controlling server in real-time, rather than in batches. Picking out key inferences from voluminous data, sent in real-time by numerous sensors is a task that is again handed over to AI systems – typically machine learning systems. While IOT systems can ably sense, transmit and store data, ML systems are required for making sense of the data and providing input on whether any action is required to be taken, and potentially even suggesting what best-case action could be taken.
IOT + Smart Cities
While the concept of a Smart City is a sub-segment of IOT, it’s the other way around. IOT forms only one of the component of powering a smart city. In addition to IOT, the Smart City stack would typically also include cloud (for running processes and storing data), Artificial Intelligence (for data analysis and learning) and an element of urban planning (for deciding the what and how of a Smart City design). By combining knowledge of IOT with these other ancillary areas can help IOT professionals become valuable and irreplaceable resources in this fast-growing technology area.
AI + Behavioral Sciences
This final combination may sound surprising, but one of the most valuable and high-impact grouping of skills might just be the combination of data science and behavioral science. While AI and data science can provide the what (‘What happened?’ And ‘What should we do now?’) of a business scenario, behavioral sciences inform the how part. Consider the example of Amazon, which has numerous examples of the coming together of behavioral sciences and AI. The recommendation engine uses artificial intelligence to answer the what part of ‘what are other people buying’. But, the idea that it will lead to continued stickiness on the website, serve as a wide showcase of SKUs available on it, while promoting product bundling and larger cart sizes is a clear behavioral sciences intervention and contributes massively to the success of AI applications.
If you are a professional already conversant with one of the emerging technology areas, combining that with another emerging area can be hugely beneficial. IT professionals today in these areas should strongly consider leveraging synergies across multiple technology areas – which can help them be better-rounded, high-value practitioners in an ever evolving areas of technology.