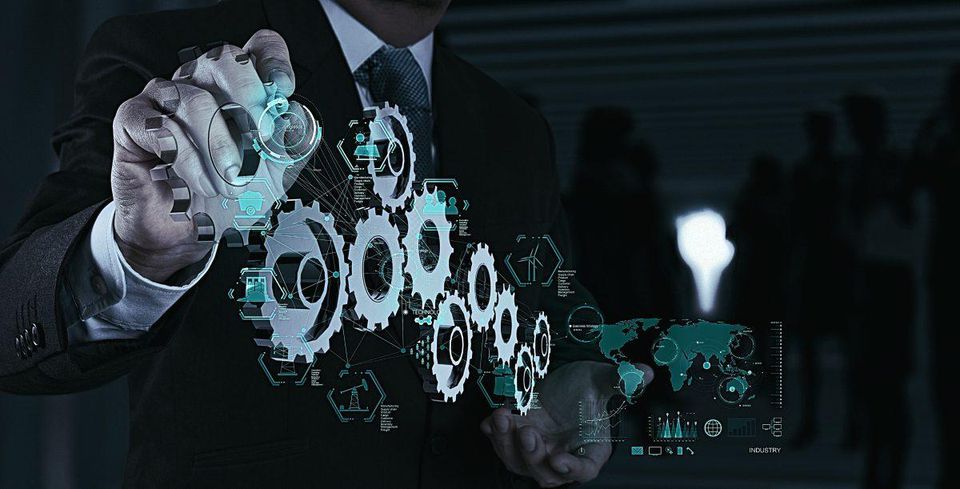
Building AI-enabled organisations
Add Your Heading Text Here
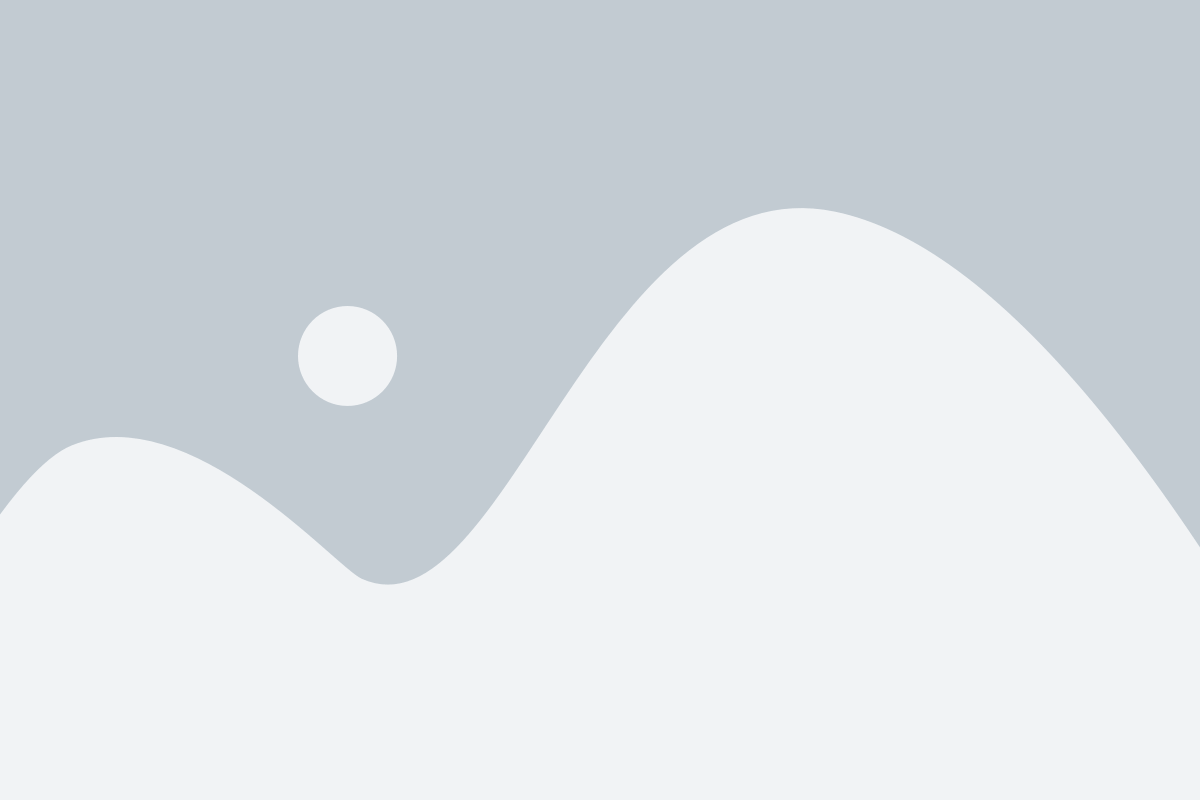
The adoption and benefit realisation from cognitive technologies is gaining increasing momentum. According to a PwC report, 72% of business executives surveyed believe that artificial intelligence (AI) will be a strong business advantage and 67% believe that a combination of human and machine intelligence is a more powerful entity than each one on its own.
Another survey conducted by Deloitte reports that on an average, 83% of respondents who have actively deployed AI in the enterprise see moderate to substantial benefits through AI – a number that goes further up with the number of AI deployments.
These studies make it abundantly clear that AI is occupying a high and increasing mindshare among business executives – who have a strong appreciation of the bottom line impact delivered by cognitive systems, through improved efficiencies.
AI-first Mindset
Having said that, with AI becoming more and more mainstream in an organisational setup, piecemeal implementations will deliver a lower marginal impact to organisations’ competitive advantage. While once early adopters were able to realise transformational benefits through siloed AI deployments, now that it is fast maturing as a must-have in the enterprise and we will need a different approach.
To realise true competitive advantage, organisations need to have an AI-first mindset. It is the new normal in accelerating business decisions. It was once said that every company is a technology company – meaning that all companies were expected to have mature technology backbones to deliver business impact and customer satisfaction. That dictum is now being amended to say – every company is a cognitive company.
To deliver on this promise, companies need to weave AI into the very fabric of their strategy. To realise competitive advantage tomorrow, we need to embed AI across the organisation today, with a strong, stable and scalable foundation. Here are three building blocks that are needed to create that robust foundation.
1. Enrich Data & Algorithm Repositories
If data is indeed the new oil (which it is), organisations that hold the deepest reserves and the most advanced refinery will be the ones that win in this new landscape. Companies having the most meaningful repository of data, along with fit-for-purpose proprietary algorithms will most likely enjoy a sizeable competitive advantage.
So, companies need to improve and re-invent their data generation and collection mechanisms. Data generation will help reduce their reliance on external data providers and help them own the data for conducting meaningful, real-time analysis by continuously enriching the data set.
Alongside, corporations also need to build an ‘algorithm factory’ – to speed up the development of accurate, fit-for-purpose and meaningful algorithms. The algorithm factory would need to push out data models in an iterative process in a way that improves the speed and accuracy.
This would enable the data and analysis capabilities of companies to grow in a scalable manner. While this task would largely fall under the aegis of data science teams, business teams would be required to provide timely interventions and feedback – to validate impact delivered by these models, and suggest course-corrections where necessary.
Another key aspect of this process is to enable a transparent cross-organisation view into these repositories. This will allow employees to collaborate and innovate rapidly by learning what is already been done and will reduce needless time and effort spent in developing something that’s already there.
2. AI Education for Workforce
Operationalising AI requires a convergence of different skill sets. According to the above-cited Deloitte survey, 37% of respondents felt that their managers didn’t understand cognitive technology – which was a hindrance to their AI deployments.
We need to mix different streams of people to build a scalable AI-centric organisation. For instance, business teams need to be continuously trained on the operational aspects of AI, its various types, use cases and benefits – to appreciate how AI can impact their area of business.
Technology teams need to be re-skilled around the development and deployment of AI applications. Data processing and analyst teams need to better understand how to build scalable computational models, which can run more autonomously and improve fast.
Unlike a typical technology transformation, AI transformation is a business reengineering exercise and requires cross-functional teams to collaborate and enrich their understanding of AI and how it impacts their functions, while building a scalable AI programme.
The implicit advantage of developing topical training programmes and involving a larger set of the workforce is to mitigate the FUD that is typically associated with automation initiatives. By giving employees the opportunity to learn and contribute in a meaningful way, we can eliminate bottlenecks, change-aversion and enable a successful AI transformation.
3. Ethical and Security Measures
The 4th Industrial Revolution will require a re-assessment of ethical and security practices around data, algorithms and applications that use the former two.
By introducing renewed standards and ethical codes, enterprises can address two important concerns people typically raise – how much power can/should AI exercise and how can we stay protected in cases of overreach.
We are already witnessing teething trouble – with accidents involving self-driving cars resulting in pedestrian deaths, and the continuing Facebook-Cambridge Analytica saga.
Building a strong grounding for AI systems will go a long way in improving customer and social confidence – that personal data is in safe hands and is protected from abuse – enabling them to provide an informed consent to their data. To that end, we need to continue refining our understanding around the ethical standards of AI implementations
AI and other cyber-physical systems are key components of the next generation of business. According to a report by semiconductor manufacturer, ARM, 61% of respondents believe that AI can make the world a better place. To increase that sentiment even further, and to make AI business-as-usual, and power the cognitive enterprise, it is critical that we subject machine intelligence to the same level of governance, scrutiny and ethical standards that we would apply to any core business process.
Related Posts
AIQRATIONS
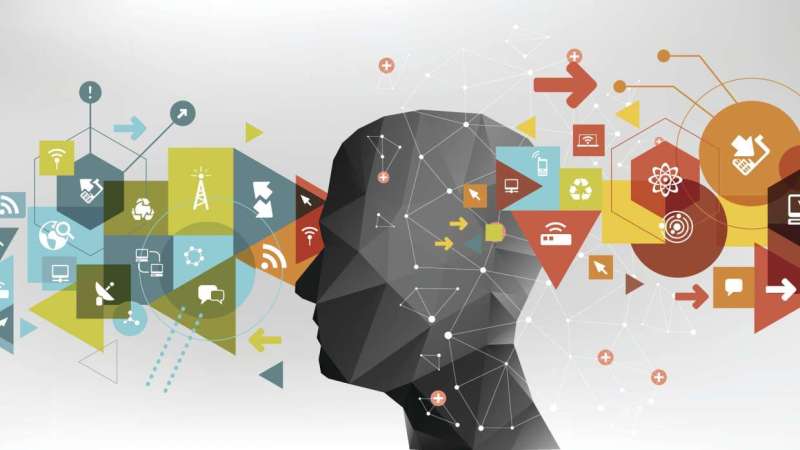
Design Thinking | Behavioural Sciences: Strategic Elements to Building a Successful AI Enterprise
Add Your Heading Text Here
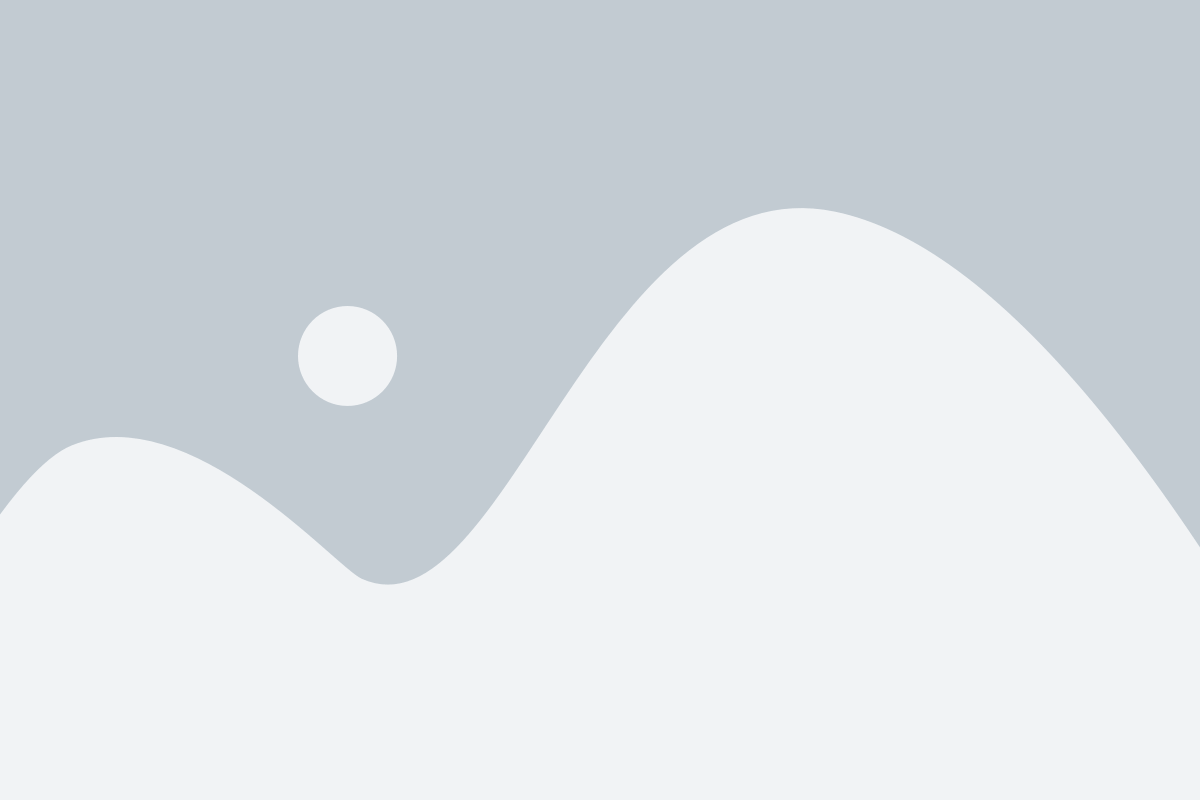
Today’s artificial intelligence (AI) revolution has been made possible by the algorithm revolution. The machine learning algorithms researchers have been developing for decades, when cleverly applied to today’s web-scale data sets, can yield surprisingly good forms of intelligence. For instance, the United States Postal Service has long used neural network models to automatically read handwritten zip code digits. Today’s deep learning neural networks can be trained on millions of electronic photographs to identify faces, and similar algorithms may increasingly be used to navigate automobiles and identify tumors in X-rays. The IBM Watson information retrieval system could triumph on the game show “Jeopardy!” partly because most human knowledge is now stored electronically.
But current AI technologies are a collection of big data-driven point solutions, and algorithms are reliable only to the extent that the data used to train them is complete and appropriate. One-off or unforeseen events that humans can navigate using common sense can lead algorithms to yield nonsensical outputs.
Design thinking is defined as human-centric design that builds upon the deep understanding of our users (e.g., their tendencies, propensities, inclinations, behaviours) to generate ideas, build prototypes, share what you’ve made, embrace the art of failure (i.e., fail fast but learn faster) and eventually put your innovative solution out into the world. And fortunately for us humans (who really excel at human-centric things), there is a tight correlation between the design thinking and artificial intelligence.
Artificial intelligence technologies could reshape economies and societies, but more powerful algorithms do not automatically yield improved business or societal outcomes. Human-centered design thinking can help organizations get the most out of cognitive technologies.
Divergence from More Powerful Intelligence To More Creative Intelligence
While algorithms can automate many routine tasks, the narrow nature of data-driven AI implies that many other tasks will require human involvement. In such cases, algorithms should be viewed as cognitive tools capable of augmenting human capabilities and integrated into systems designed to go with the grain of human—and organizational—psychology. We don’t want to ascribe to AI algorithms more intelligence than is really there. They may be smarter than humans at certain tasks, but more generally we need to make sure algorithms are designed to help us, not do an end run around our common sense.
Design Thinking at Enterprise Premise
Although cognitive design thinking is in its early stages in many enterprises, the implications are evident. Eschewing versus embracing design thinking can mean the difference between failure and success. For example, a legacy company that believes photography hinges on printing photographs could falter compared to an internet startup that realizes many customers would prefer to share images online without making prints, and embraces technology that learns faces and automatically generates albums to enhance their experience.
To make design thinking meaningful for consumers, companies can benefit from carefully selecting use cases and the information they feed into AI technologies. In determining which available data is likely to generate desired results, enterprises can start by focusing on their individual problems and business cases, create cognitive centres of excellence, adopt common platforms to digest and analyze data, enforce strong data governance practices, and crowdsource ideas from employees and customers alike.
In assessing what constitutes proper algorithmic design, organizations may confront ethical quandaries that expose them to potential risk. Unintended algorithmic bias can lead to exclusionary and even discriminatory practices. For example, facial recognition software trained on insufficiently diverse data sets may be largely incapable of recognizing individuals with different skin tones. This could cause problems in predictive policing, and even lead to misidentification of crime suspects. If the training data sets aren’t really that diverse, any face that deviates too much from the established norm will be harder to detect. Accordingly, across many fields, we can start thinking about how we create more inclusive code and employ inclusive coding practices.
CXO Strategy for Cognitive Design Thinking
CIOs can introduce cognitive design thinking to their organizations by first determining how it can address problems that conventional technologies alone cannot solve. The technology works with the right use cases, data, and people, but demonstrating value is not always simple. However, once CIOs have proof points that show the value of cognitive design thinking, they can scale them up over time.
CIOs benefit from working with business stakeholders to identify sources of value. It is also important to involve end users in the design and conception of algorithms used to automate or augment cognitive tasks. Make sure people understand the premise of the model so they can pragmatically balance algorithm results with other information.
Enterprise Behavioral Science – From Insights to Influencing Business Decisions
Every January, how many people do you know say that they want to resolve to save more, spend less, eat better, or exercise more? These admirable goals are often proclaimed with the best of intentions, but are rarely achieved. If people were purely logical, we would all be the healthiest versions of ourselves.
However, the truth is that humans are not 100% rational; we are emotional creatures that are not always predictable. Behavioral economics evolved from this recognition of human irrationality. Behavioral economics is a method of economic analysis that applies psychological insights into human behavior to explain economic decision-making.
Decision making is one of the central activities of business – hundreds of billions of decisions are made everyday. Decision making sits at the heart of innovation, growth, and profitability, and is foundational to competitiveness. Despite this degree of importance, decision making is poorly understood, and badly supported by tools. A study by Bain & Company found that decision effectiveness is 95% correlated with companies’ financial performance.
Enterprise Behavioral Science is not only about understanding potential outcomes, but to completely change outcomes, and more specifically, change the way in which people behave. Behavioral Science tells us that to make a fundamental change in behavior that will affect the long-term outcome of a process, we must insert an inflection point.
As an example, you are a sales rep and two years ago your revenue was $1 million. Last year it was $1.1 million, and this year you expect $1.2 million in sales. The trend is clear, and your growth has been linear and predictable. However, there is a change in company leadership and your management has increased your quota to $2 million for next year. What is going to motivate you to almost double your revenues? The difference between expectations ($2 million) and reality ($1.2 million) is often referred to as the “behavioral gap” . When the behavioral gap is significant, an inflection point is needed to close that gap. The right incentive can initiate an inflection point and influence a change in behavior. Perhaps that incentive is an added bonus, President’s Club eligibility, a promotion, etc.
Cognitive Design Thinking – The New Indispensable Reskilling Avenue
Artificial intelligence, machine learning, big data analytics and mobile and software development will be the top technology areas where the need for re-skilling will be the highest. India will need 700 million skilled workforce by 2022 to meet the demands of a growing economy. Hence, while there is a high probability that machine learning and artificial intelligence will play an important role in whatever job you hold in the future, there is one way to “future-proof” your career…embrace the power of design thinking.
In fact, integrating design thinking and artificial intelligence can give you “super powers” that future-proof whatever career you decide to pursue. To meld these two disciplines together, one must:
- Understand where and how artificial intelligence and behavioural science can impact your business initiatives. While you won’t need to write machine learning algorithms, business leaders do need to learn how to “Think like a data scientist” in order understand how AI can optimize key operational processes, reduce security and regulatory risks, uncover new monetization opportunities.
- Understand how design thinking techniques, concepts and tools can create a more compelling and emphatic user experience with a “delightful” user engagement through superior insights into your customers’ usage objectives, operating environment and impediments to success.
Design thinking is a mindset. IT firms are trying to move up the curve. Higher-end services that companies can charge more is to provide value and for that you need to know that end-customers needs. For example, to provide value services to banking customers is to find out what the bank’s customer needs are in that country the banking client is based. Latent needs come from a design thinking philosophy where you observe customer data, patterns and provide a solution that the customer does not know. Therefore, Companies will hire design thinkers as they can predict what the consumer does not know and hence charge for the product/service from their clients. Idea in design thinking is to provide agile product creation or solutions.
Without Design Thinking & Behavioural Science, AI Will be Only an Incremental Value
Though organizations understand the opportunity that big data presents, many struggles to find a way to unlock its value and use it in tandem with design thinking – making “big data a colossal waste of time & money.” Only by combining quantitative insights gathered using AI, machine/deep learning, and qualitative research through behavioural science, and finally design thinking to uncover hidden patterns and leveraging it to understand what the customer would want, will we be able to paint a complete picture of the problem at hand, and help drive towards a solution that would create value for all stakeholders.