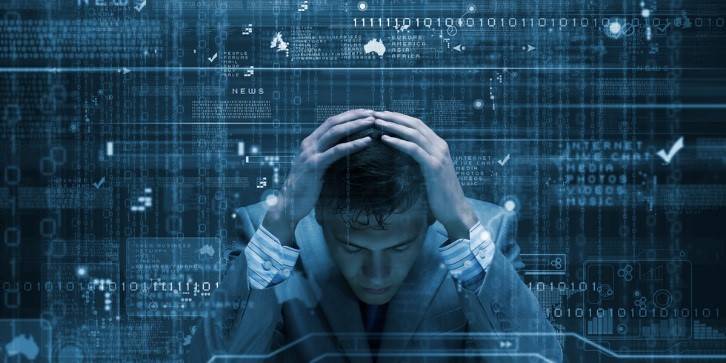
Add Your Heading Text Here
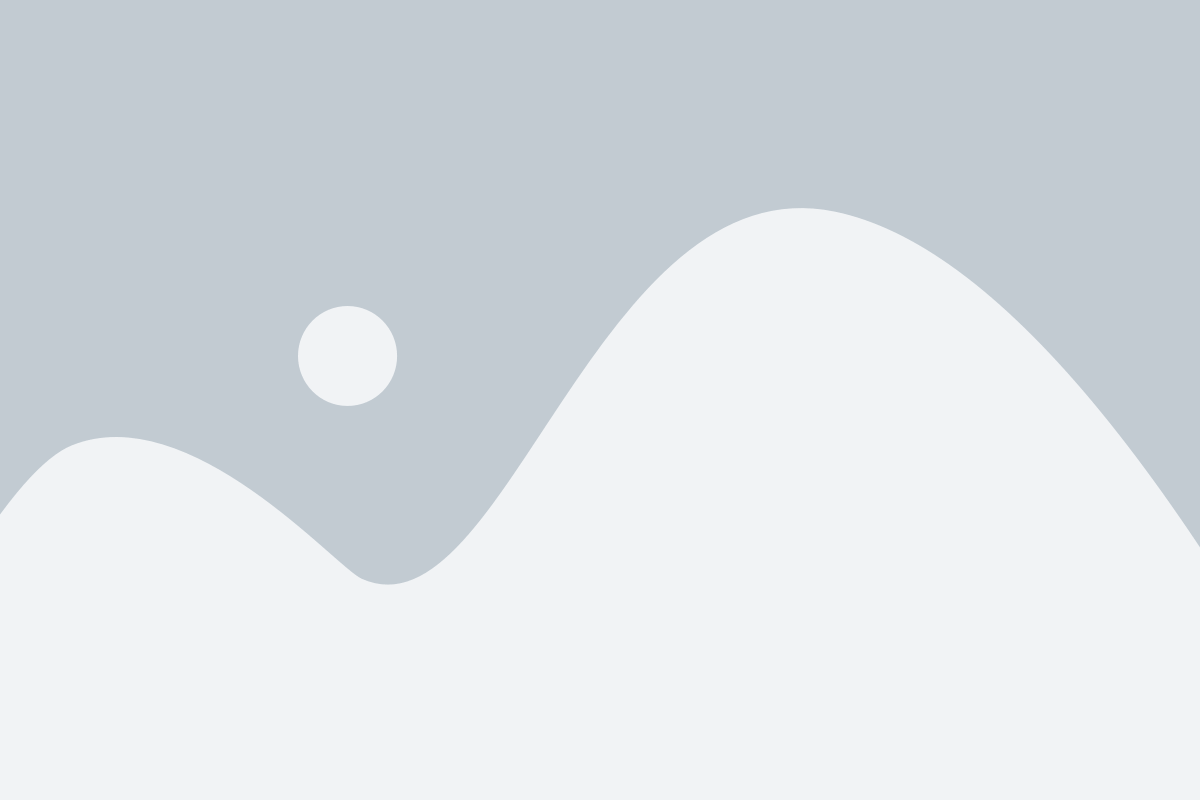
The definition of Data Breaches in current times have evolved from, happening under ‘malicious intent’, to also cover those which have been occurring as a consequences of bad data policies and regulation oversight. This means even policies that have been deemed legally screened might end up, in certain circumstances, in opening doors to some significant breach of data, user privacy and ultimately user trust.
For example, recently, Facebook banned data analytics company Cambridge Analytica from buying ads from its platform. The voter profiling firm allegedly procured 50 million physiological profiles of people through a research application developer Aleksandr Kogan, who broke Facebook’s data policies by sharing data from his personality-prediction app, that mined information from the social network’s users.
Kogan’s app, ‘thisisyourdigitallife’ harvested data not only from the individuals participating in the game, but also from everyone on their friend list. Since Facebook’s terms of services weren’t so clear back in 2014 the app allowed Kogan to share the data with third parties like Cambridge Analytica. This means policy wise it is a grey area whether the breach could be considered ‘unauthorized’, but it is clear that it happened without any express authorization from Facebook. This personal information was subsequently used to target voters and sway public opinion
This is different than the site hackings where credit card information was actually stolen at major retailers, the company in question, Cambridge Analytica, actually had the right to use this data. The problem is they used this information without permission in a way that was overtly deceptive to both Facebook users and Facebook itself.
Fallouts of Data Breaches: Developers left to deal with Tighter Controls
Facebook will become less attractive to app developers if it tightens norms for data usage as a fallout of the prevailing controversy over alleged misuse of personal information mined from its platform, say industry members.
India has the second largest developer base for Facebook, a community that builds apps and games on the platform and engage its users. With 241 million users, the country last July over took the US as the largest userbase for the social network platform.
There will be more scrutiny now. When you do, say, a sign on. The basic data (you can get) is the user’s name and email address, even which will undergo tremendous scrutiny before they approve it. That will have an impact on the timeline. The viral effect) could decrease. Now, without explicit rights from users, you cannot reach out to his/her contacts. Thus, the overhead goes on to the developers because of such data breaches, which shouldn’t have occurred in the first place had the policies surrounding user data were more distinct and clear.
Renewed Focus to Conflicting Data Policies and Human Factors
These kinds of passive breaches that happen because of unclear and conflicting policies instituted by Facebook provides us a very clear example of how active breaches (involving malicious attacks) and passive breaches (involving technically authorized but legally unsavoury data sharing) need to be given equal priority and should both be considered pertinent focus of data protection.
While Facebook CEO Mark Zuckerberg has vowed to make changes to prevent these types of information grabs from happening in the future, many of those tweaks will be presumably made internally. Individuals and companies still need to take their own action to ensure their information remains as protected and secure as possible.
Humans are the weakest link in data protection, and potentially even the leading cause for the majority of incidents in recent years. This debacle demonstrates that cliché to its full extent. Experts believe that any privacy policy needs to take into account all third parties who get access to the data too. While designing a privacy policy one needs to keep the entire ecosystem in mind. For instance, a telecom player or a bank while designing their privacy policy will have to take into account third parties like courier agencies, teleworking agencies, and call centers who have access to all their data and what kind of access they have.
Dealing with Privacy in Analytics: Privacy-Preserving Data Mining Algorithms
The problem of privacy-preserving data mining has become more important in recent years because of the increasing ability to store personal data about users, and the increasing sophistication of data mining algorithms to leverage this information. A number of algorithmic techniques such as randomization and k-anonymity, have been suggested in recent years in order to perform privacy-preserving data mining. Different communities have explored parallel lines of work in regards to privacy preserving data mining:
Privacy-Preserving Data Publishing: These techniques tend to study different transformation methods associated with privacy. These techniques include methods such as randomization, k-anonymity, and l-diversity. Another related issue is how the perturbed data can be used in conjunction with classical data mining methods such as association rule mining.
Changing the results of Data Mining Applications to preserve privacy: In many cases, the results of data mining applications such as association rule or classification rule mining can compromise the privacy of the data. This has spawned a field of privacy in which the results of data mining algorithms such as association rule mining are modified in order to preserve the privacy of the data.
Query Auditing: Such methods are akin to the previous case of modifying the results of data mining algorithms. Here, we are either modifying or restricting the results of queries.
Cryptographic Methods for Distributed Privacy: In many cases, the data may be distributed across multiple sites, and the owners of the data across these different sites may wish to compute a common function. In such cases, a variety of cryptographic protocols may be used in order to communicate among the different sites, so that secure function computation is possible without revealing sensitive information.
Privacy Engineering with AI
Privacy by Design is a policy concept was introduced the Data Commissioner’s Conference in Jerusalem, and over 120 different countries agreed they should contemplate privacy in the build, in the design. That means not just the technical tools you buy and consume, [but] how you operationalize, how you run your business; how you organize around your business and data.
Privacy engineering is using the techniques of the technical, the social, the procedural, the training tools that we have available, and in the most basic sense of engineering to say, “What are the routinized systems? What are the frameworks? What are the techniques that we use to mobilize privacy-enhancing technologies that exist today, and look across the processing lifecycle to build in and solve for privacy challenges?”
It’s not just about individual machines making correlations; it’s about different data feeds streaming in from different networks where you might make a correlation that the individual has not given consent to with personally identifiable information. For AI, it is just sort of the next layer of that. We’ve gone from individual machines, networks, to now we have something that is looking for patterns at an unprecedented capability, that at the end of the day, it still goes back to what is coming from what the individual has given consent to? What is being handed off by those machines? What are those data streams?
Also, there is the question of ‘context’. The simplistic policy of asking users if an application can access different venues of their data is very reductive. This does not, in an measure give an understanding of how that data is going to be leveraged and what other information about the users would the application be able to deduce and mine from the said data? The concept of privacy is extremely sensitive and not only depends on what data but also for what purpose. Have you given consent to having it used for a particular purpose? So, I think AI could play a role in making sense of whether data is processed securely.
The Final Word: Breach of Privacy as Crucial as Breach of Data
It is undeniably so that we are slowly giving equal, if not more importance to breach of privacy as compared to breach of data, which will eventually target even the policies which though legally acceptable or passively mandated but resulted in compromise of privacy and loss of trust. Because there is no point claiming one is legally safe in their policy perusal if the end result leads to the users being at the receiving end.
This would require a comprehensive analysis of data streams, not only internal to an application ecosystem, like Facebook, but also the extended ecosystem involving all the players it is channeling the data sharing to, albeit in a policy-protected manner. This will require AI enabled contextual decision making to come to terms as what policies could be considered as eventually breaching the privacy in certain circumstances.
Longer-term, though, you’ve got to write that ombudsman. We need to be able to engineer an AI to serve as an ombudsman for the AI itself.