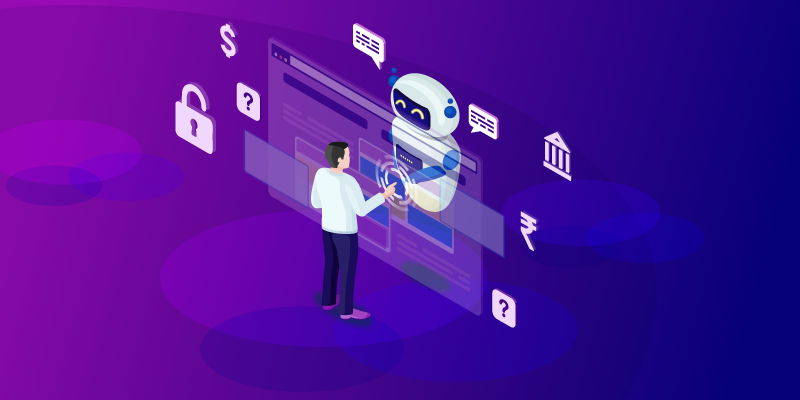
Add Your Heading Text Here
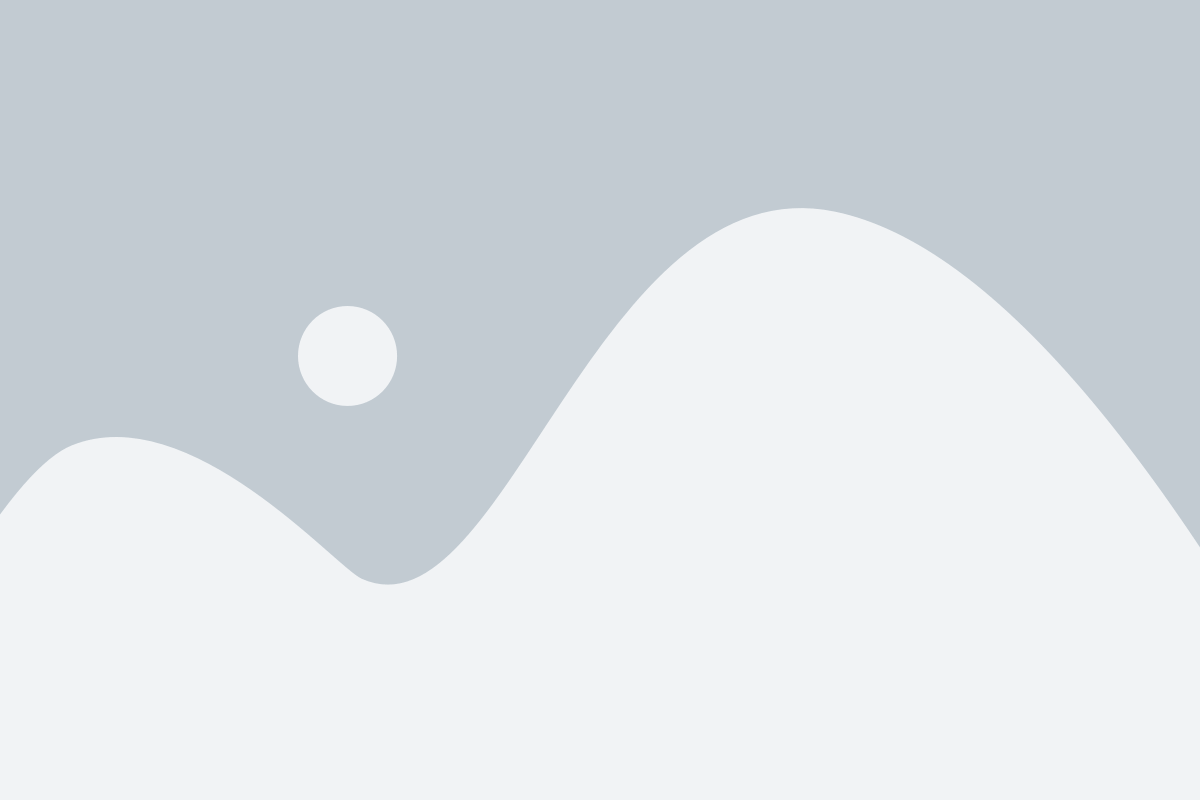
Artificial intelligence (AI) will empower banking organisations to completely redefine how they operate, establish innovative products and services, and most importantly impact customer experience interventions. In this second machine age, banks will find themselves competing with upstart fintech firms leveraging advanced technologies that augment or even replace human workers with sophisticated algorithms. To maintain a sharp competitive edge, banking corporations will need to embrace AI and weave it into their business strategy.
In this post, I will examine the dynamics of AI ecosystems in the banking industry and how it is fast becoming a major disrupter by looking at some of the critical unsolved problems in this area of business. AI’s potential can be looked at through multiple lenses in this sector, particularly its implications and applications across the operating landscape of banking. Let us focus on some of the key artifiicial intelligence technology systems: robotics, computer vision, language, virtual agents, and machine learning (including deep learning) that underlines many recent advances made in this sector.
Industry Changes
Banks entering the intelligence age are under intense pressure on multiple fronts. Rapid advances in AI are coming at a time of widespread technological and digital disruption. To manage this impact, many changes are being triggered.
- Leading banks are aggressively hiring Chief AI Officers while investing in AI labs and incubators
- AI-powered banking bots are being used on the customer experience front.
- Intelligent personal investment products are available at scale
- Multiple banks are moving towards custom in-house solutions that leverage sophisticated ontologies, natural language processing, machine learning, pattern recognition, and probabilistic reasoning algorithms to aid skilled employees and robots with complex decisions
Some of the key characteristics shaping this industry include:
- Decision support and advanced algorithms allow the automation of processes that are more cognitive in nature
- Solutions incorporate advanced self-learning capabilities
- Sophisticated cognitive hypothesis generation/advanced predictive analytics
Surge of AI in Banking
Banks today are struggling to reduce costs, meet margins, and exceed customer expectations through personal experience. To enable this, implementing AI is particularly important. And banks have started embracing AI and related technologies worldwide. According to a survey by the National Business Research Institute, over 32 percent of financial institutions use AI through voice recognition and predictive analysis. The dawn of mobile technology, data availability and the explosion of open-source software provides artificial intelligence huge playing field in the banking sector. The changing dynamics of an app-driven world is enabling the banking sector to leverage AI and integrate it tightly with the business imperatives.
AI in Banking Customer Services
Automated AI-powered customer service is gaining strong traction. Using data gathered from users’ devices, AI-based relay information using machine learning by redirecting users to the source. AI-related features also enable services, offers, and insights in line with the user’s behaviour and requirements. The cognitive machine is trained to advise and communicate by analysing users’ data. Online wealth management services and other services are powered by integrating AI advancements to the app by capturing relevant data.
The tested example of answering simple questions that the users have and redirecting them to the relevant resource has proven successful. Routine and basic operations i.e. opening or closing the account, transfer of funds, can be enabled with the help of chatbots.
Fraud and risk management
Online fraud is an area of massive concern for businesses as they digitise at scale. Risk management at internet scale cannot be managed manually or by using legacy information systems. Most banks are looking to deploy machine or deep learning and predictive analytics to examine all transactions in real-time. Machine learning can play an extremely critical role in the bank’s middle office.
The primary uses include mitigating fraud by scanning transactions for suspicious patterns in real-time, measuring clients for creditworthiness, and enabling risk analysts with right recommendations for curbing risk.
Trading and Securities
Robotic Process Automation (RPA) plays a key role in security settlement through reconciliation and validation of information in the back office with trades enabled in the front office. Artificial intelligence facilitates the overall process of trade enrichment, confirmation and settlement.
Credit Assessment
Lending is a critical business for banks, which directly and indirectly touches almost all parts of the economy. At its core, lending can be seen as a big data problem. This makes it an effective case for machine learning. One of the critical aspects is the validation of creditworthiness of individuals or businesses seeking such loans. The more data available about the borrower, the better you can assess their creditworthiness.
Usually, the amount of a loan is tied to assessments based on the value of the collateral and taking future inflation into consideration. The potential of AI is that it can analyse all of these data sources together to generate a coherent decision. In fact, banks today look at creditworthiness as one of their everyday applications of AI.
Portfolio Management
Banks are increasingly relying on machine learning to make smarter, real-time investment decisions on behalf of their investors and clients.
These algorithms can progress across distinct ways. Data becomes an integral part of their decision-making tree, this enables them to experiment with different strategies on the fly to broaden their focus to consider a more diverse range of assets.
Banks are focussed to leverage an AI and machine learning-based technology platforms that make customised portfolio profiles of customers based on their investment limits, patterns and preferences.
Banking and artificial intelligence are at a vantage position to unleash the next wave of digital disruption. A user-friendly AI ecosystem has the potential for creating value for the banking industry, but the desire to adopt such solutions across all spectrums can become roadblocks. Some of the issues can be long implementation timelines, limitations in the budgeting process, reliance on legacy platforms, and the overall complexity of a bank’s technology environment.
To overcome the above challenges of introducing and building an AI-enabled environment. Banks need to enable incremental adoption methods and technologies. The critical part is ensuring that the transition allows them to overcome the change management/behavioural issues. The secret sauce of successful deployment is to ensure a seamless fit into the existing technology architecture landscape, making an effective AI enterprise environment.