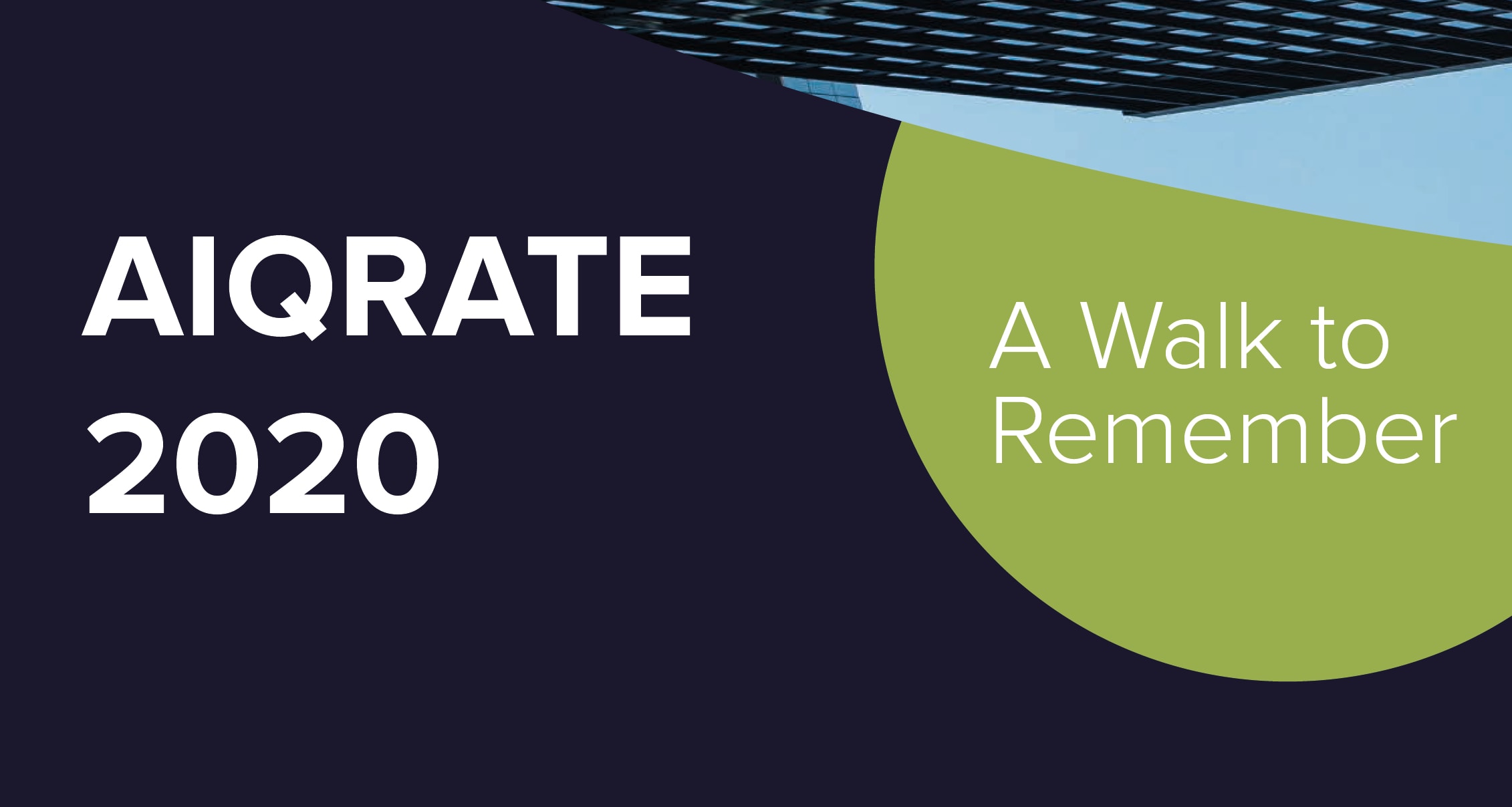
AIQRATE in 2020 ….A walk to remember
Add Your Heading Text Here
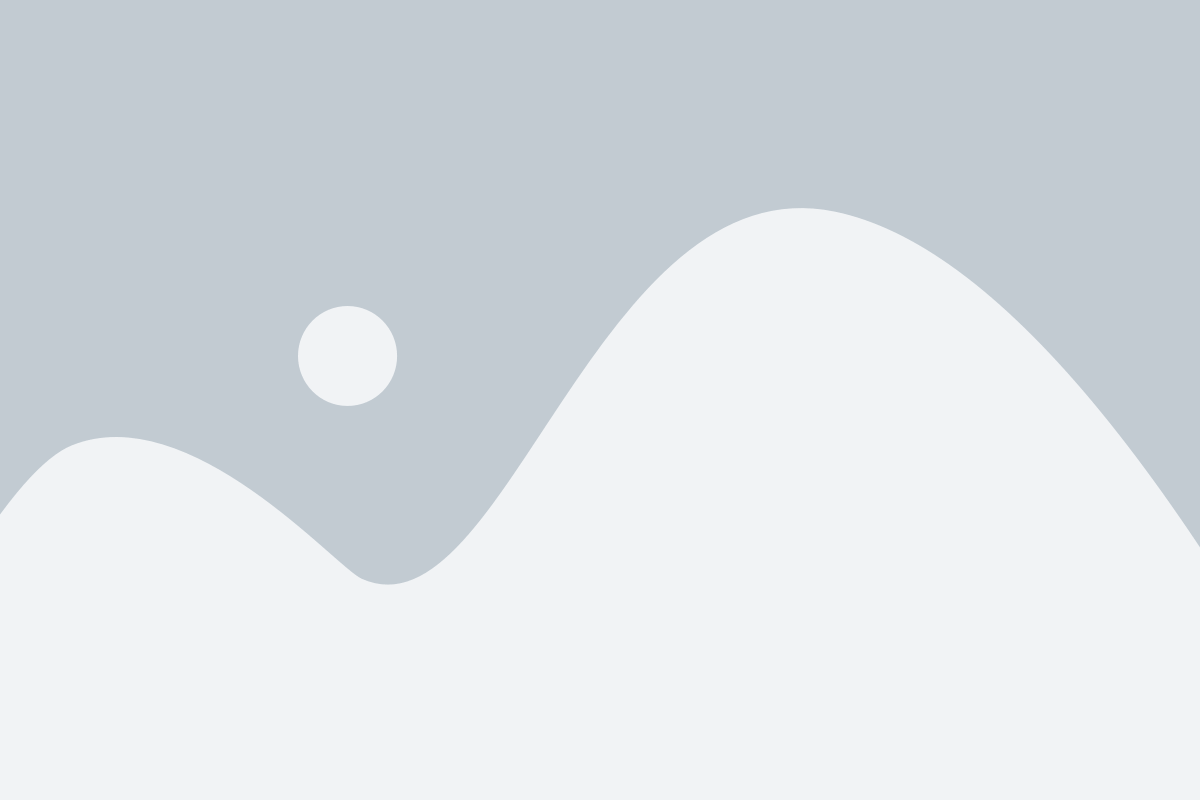
“Enabling clients reimagine their decision making & accentuate the business performance with AI strategy in a transformation, innovation and disruption driven world”
In today’s fast paced & volatile VUCA world, leaders face unprecedented challenges. They need to navigate through volatility while staying focused on strategy, business performance and culture. Artificial Intelligence is fast becoming a game changing catalyst and a strategic differentiator and almost a panacea to solve large, complex and unresolved problems. To be an AI powered organization, leaders not only need to have a broad understanding of AI strategy, they need to know how and where to use it. AIQRATE advisory services and consulting offerings are designed to enable leaders and decision makers from Enterprises, GCCs, Cloud Providers, Technology players, Startups, SMBs, VC/PE firms, Public Institutions and Academic Institutions to become AI ready and reduce the risk associated with curating, deploying AI strategy and ensuing interventions and increase the predictability of a durable leader’s success.
In the age of the bionic enterprises, AI continues to dominate the technology & business landscape. Under the aegis of transformation, disruption and innovation, AI has several applications and impact areas which usher a new change in how we make decisions in the enterprise and personal spheres. Traditionally, human decisions are to a large extent based on intuition, gut and historical data. In the age of AI, several of our decisions will be taken by algorithms. Leveraging AI, the ability to mimic the human brain and the ensuing ability to sense, comprehend and act will significantly go up and will result in emergence of augmented intelligence in decision making. Enterprises, GCCs, SMBs, Startups and Government Institutions are attempting to harness the power of AI to change the way they do business. All these industry segments are looking at AI becoming the secret sauce behind making them gain a competitive advantage. If you have not started yet, you are already behind the competition, however large or pedigreed you might be.
So, where are you placed on your AI journey? At AIQRATE, we can guide you on your journey of understanding what AI can do for you, embedding it within your business strategy, functional areas and augmenting the decision-making process.
At AIQRATE, we are here to help you with the art of the possible with AI. Through our bespoke AI strategy frameworks, methodologies, toolkits, playbooks and assessments, we will bring seamless Transformation, Innovation and Disruption to your businesses. Leveraging our proven repository of consulting templates and artifacts, we will curate your AI strategic approach roadmap. Our advisory offerings and consulting engagements are designed in alignment with your strategic growth, vision and competitive scenarios.
We are at an inflection point where AI will revolutionize the way we do business. The paradigms of customer, products, offerings, services and competition will change dramatically; and being AI-ready will become a true differentiator. AIQRATE will be your strategic partner to help you to prepare for what’s next in order to stay relevant.
Enclosing here our journey walk through of 2020.
Wish you a great 2021!
Best,
Sameer Dhanrajani
Chief Executive Officer
AIQRATE
Bangalore , India
Related Posts
AIQRATIONS
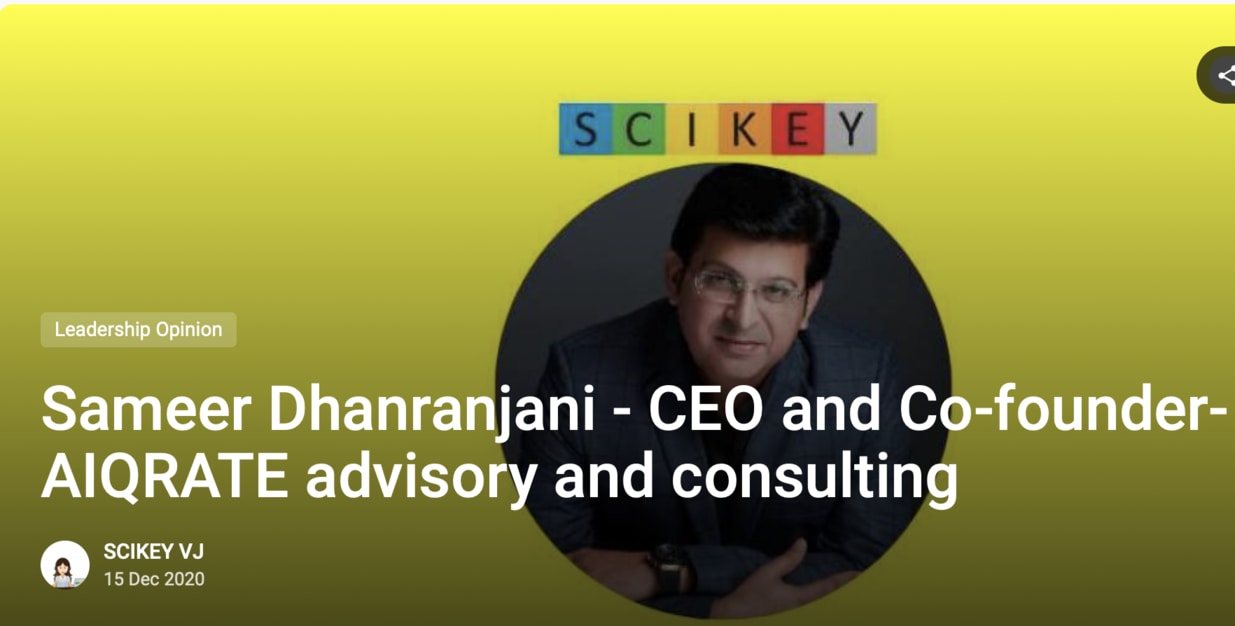
AI led Algorithms can decide on how we need to emote, behave, react, transact or interact with an individual – Sameer with SCIKEY
Add Your Heading Text Here
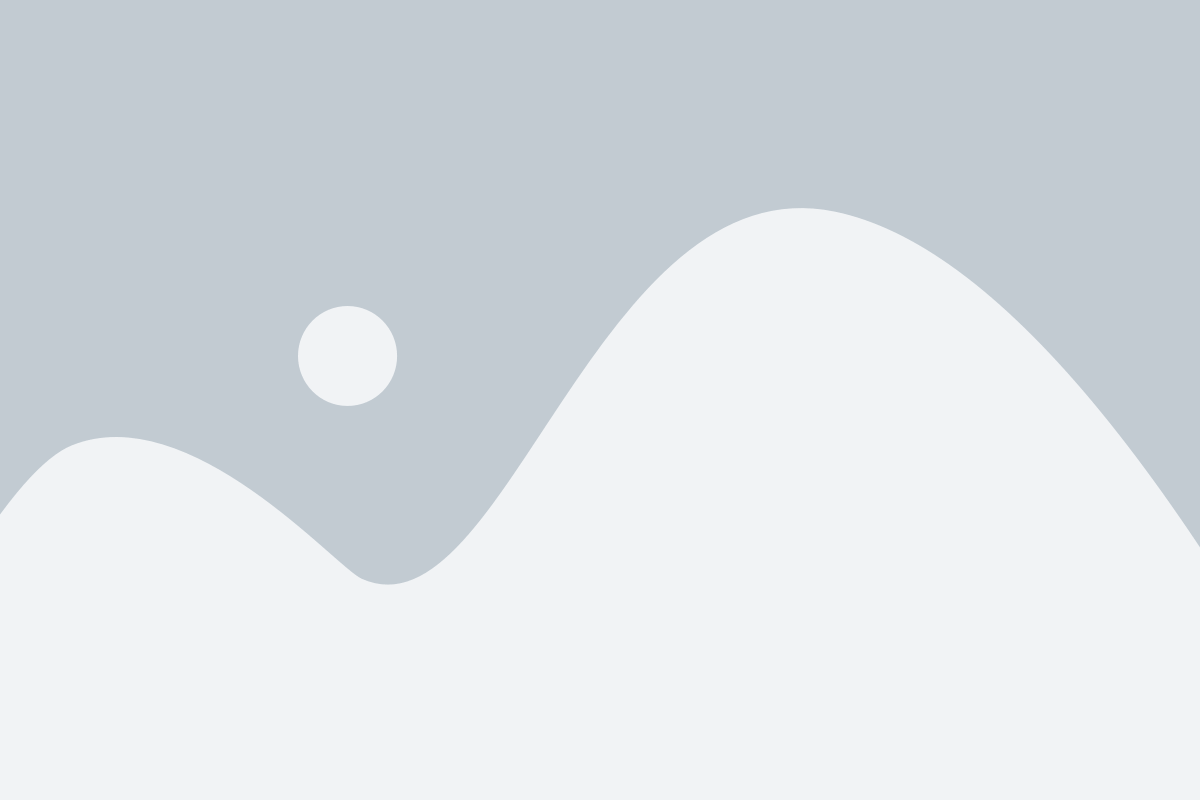
In an exclusive interaction with SCIKEY, Sameer Dhanrajani, CEO at AIQRATE Advisory & Consulting, speaks about how the future of work will look like enabled by AI, and it’s contribution in building productive teams and the emerging AI trends to watch out for in Post COVID scenario.
“AI led algorithms can decide on how we need to emote, behave, react, transact or interact with an individual,” Sameer Dhanranjani
Sameer is a globally recognized AI advisor, business builder, evangelist and thought leader known for his deep knowledge, strategic consulting approaches in AI space. Sameer has consulted with several Fortune 500 global enterprises, Indian corporations , GCCs, startups , SMBs, VC/PE firms, Academic Institutions in driving AI led strategic transformation and innovation strategies. Sameer is a renowned author, columnist, blogger and four times Tedx speaker. He is an author of bestselling book – AI and Analytics: accelerating business decisions.
In an exclusive interaction with SCIKEY, Sameer Dhanranjani, CEO at AIQRATE advisory consulting, speaks about how the future of work will look like enabled by AI, and it’s contribution in building productive teams and the emerging AI trends to watch out for in Post COVID scenario.
Mr Dhanranjani, you have consulted with several Fortune 500 enterprises, GCCs also start-ups in driving AI-led strategic transformation strategies. What according to you, are the topmost strategic considerations to weigh for managing accelerating business in Post COVID world for a start-up?
The unprecedented times of COVID-19 have brought the aspect of decision making under consideration. This includes tactical, strategic, and operational decision making that is crucial to make the venture more sustainable. Today the use of artificial intelligence is quite high amongst organizations. It can be used by start-up ventures and other outfits to make decisions irrespective of the area that needs decision making.
Most decisions that need to be made strategically are being passed on to artificial intelligence-enabled interventions. The algorithm makes similar decisions based on the previous decisions taken. Algorithms can decide how we need to emote, behave, react, transact or interact with the opposite individual This advancement in AI brings the challenge for organizations to create products and services specific to each customer through hyper-personalization and micro-segmenting. However, it can also be considered as an opportunity for organizations to emerge from the pandemic with newer business models and experiences for customers. Start-ups, especially, can make use of such advancements to reinvent and rejuvenate the organizational ecosystem.
You are known for your passion for Artificial Intelligence and are an author to the bestselling book – AI and Analytics: Accelerating Business Decisions. Tell us where how can AI be strategically significant while building productive teams.
My experience has led me to deal with engagements in the entire value chain of HR, ranging from hiring to engagement to incentivization that has leveraged using AI. It is phenomenal to see how AI can help build, engage, and sustain productive teams. AI can help in hiring through the detection emotions, facial expressions, tone modulations of the interviewee through computer vision and image classification techniques.
In the creation of productive teams, AI can gauge the engagement levels of an employee. It tries to look at the various interventions made by an employee regarding their attendance, participation in virtual meetings, and propensity to ask and engage themselves in conversations. It also keeps in check the number of pauses, intervals, and breaks taken by an employee. Every aspect of the employee is being marked to see how productive, inclusive, as an individual and in teams.
What are the top 5 AI trends to watch out for in Post COVID the scenario of the next one year?
When it comes to AI, the first trend emerging is that AI is not a tool or a technology, but it is now being touted as a strategic imperative for any organization. This means that AI strategies will become an intrinsic part and feature of every organisation.
The second trend is the democratization of AI. There is a possibility of the emergence of an AI marketplace where virtual exchanges related to business problems, demo runs etc. can be conducted. One would actually be able to figure out which algorithm is best for them in customer experience, supply chain etc.
The third trend being the cloud will act as a catalyst for AI proliferation. The propensity for cloud providers to enable AI companies with possible aspects of microservice API’s, Product Solutions will be created on the go. This means that the cloud enablers will have options to see various possibilities specific to their organisation when it comes to AI-specific use cases.
The fourth trend is linked to skilling. AI today is a part of a lot of course curriculums. But what is missing is the whole aspect of how does it get applied? The new courseware will be focused on how is AI implemented, adopted in the organization.
The last fifth trend is decision-making enabled by AI, which means humans will have no option but to upskill and reskill themselves to take a more rational, pragmatic and sanguine approach. So new models, new emerging realities of decision making will emerge.
How is AI powering the Future of Work, what are critical considerations for business and tech leaders considering the rapidly changing business dynamics due to COVID?
The future of work will be about AI and what we call AI plus a set of exponential technologies. This means that every aspect of our performance interaction and our responses will be gauged very manually through these technologies. This indicates that the level of performances in terms of how we go up-to-date needs to be worked upon. The future of work is an ecosystem where one particular employer cannot do it all.
This means that if learning must occur through an external player, it must come through the ecosystem of co-employees and the employer. In the future, we will not be caged as mere professionals doing our job but will be encouraged to push our boundaries to explore more at work. At the same time, transformation, innovation, and disruption will be a part of the future’s performance metrics. They will become a major parameter for the organization to create a mediocre versus proficient employee or a professional. This is where the onus will fall on the employees to ensure that they are not just doing what is being called out, but are going beyond to create what we call a value creation for the organisation.
About SCIKEY:
SCIKEY Market Network is a Digital Marketplace for Jobs, Work Business solutions, supported by a Professional Network and an integrated Services Ecosystem. It enables enterprises, businesses, job seekers, freelancers, and gig workers around the world. With its online events, learning certifications, assessments, ranking awards, content promotion tools, SaaS solutions for business, a global consulting ecosystem, and more, companies can get the best deals in one place.
‘SCIKEY Assured,’ a premium managed services offering by SCIKEY, delivers the best outcomes to enterprise customers globally for talent and technology solutions getting delivered offshore, remotely, or on-premise. We are super-proud to be working with some of the world’s most iconic Fortune1000 brands.
Better Work. Better Business. Better Life. Better World.
Related Posts
AIQRATIONS
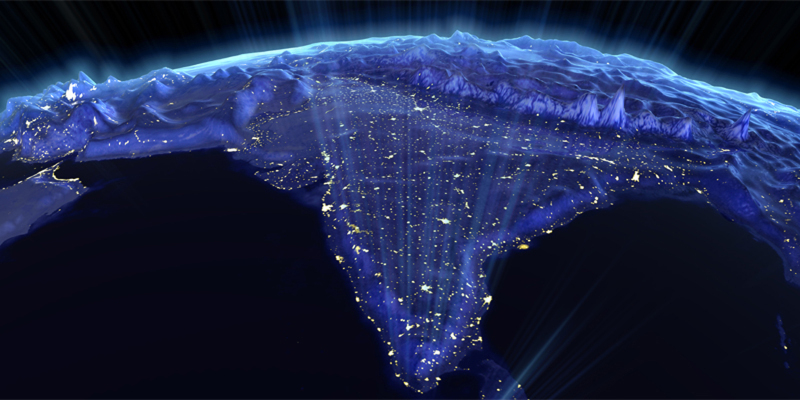
How India can Emerge as a Premier Destination for AI; Watch out China, USA…
Add Your Heading Text Here
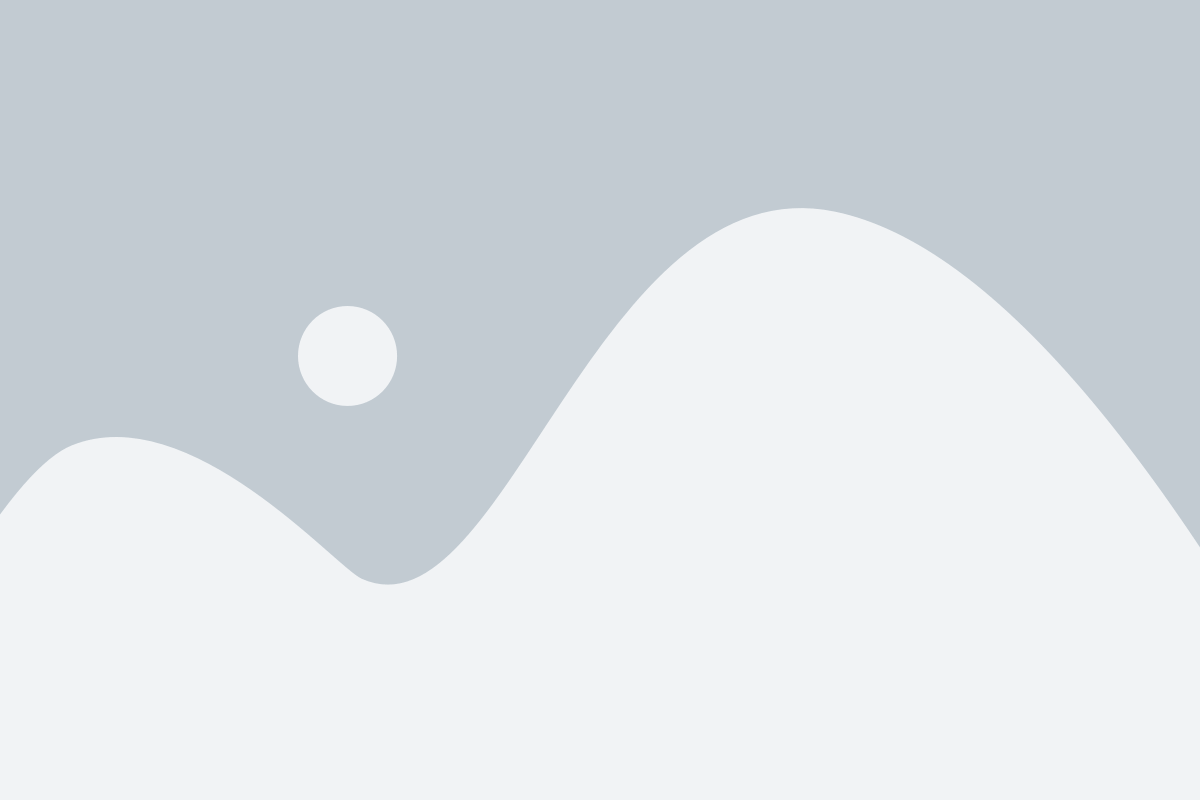
This detailed primer will provide you crucial overview of the AI’s increasing prevalence amongst Indian industry, government and peripheral ecosystem and the significant impact AI will have in your organizations to remodel strategic and business models accordingly. The ensuing details also highlights the relative comparison amongst India, China and USA on the steady progress being done in AI adoption.
VC’s, PE funds and investors attempting to understand where to target investment, what offerings and capabilities would lead to better performance and gains, and how to capitalize on AI opportunities, it’s crucial for them to understand the International economic potential of AI for now and projections in the coming years.
Cutting across all these considerations is how to build responsible AI operating models and keep it transparent enough to maintain the confidence of customers and wider stakeholders.
International AI Capitalization Report – China & NA Leads, India hot in the heels
Without doubt, AI is going to be a big game changer in the international setting. A recent PwC report concludes that AI could contribute up to $15.7 trillion to the global economy in 2030, more than the current output of China and India combined. Of this, $6.6 trillion is likely to come from increased productivity and $9.1 trillion is likely to come from consumption-side effects.
Global GDP will be up to 14% higher in 2030 as a result of the accelerating development and take-up of AI
from the standpoint of direct economic impact of AI, China and North America will have greatest gains in GDP. Even though NA will reach its peak of AI led growth faster due to huge opportunities in parallel technologies implementations and advanced customer readiness for AI. NA is supposed to reach the peak of macroeconomic gains by 2020, following which there would be a relative slowdown in the growth.
Figure 1: Souce – PwC Analysis
China, on the other hand will have a slower but stable rise in GDP gains, even post mid 2020s because a large portion of Chinese GDP comes from manufacturing, a sector which is highly susceptible to AI disruption in its operation, and also a higher rate of capital re-investment within Chinese economy compared to EU and NA. As productivity in China eventually catches up with North America, NA will focus more on importing AI-enabled products from China due to economically cheap alternative China provides. Hence by 2030, China will see much larger impact in its GDP.
Sector-wise AI Consumption Impact Index – The sector-wise impact of AI and its constituent offerings space will give crucial overview for investors to get a clear understanding of opportunities and threats in AI investment space. AI is set to be the key source of transformation, disruption and competitive advantage in today’s fast changing economy. Drawing on the findings of our AI Impact Index, we look at how quickly change is coming and where your business can expect the greatest return.
Figure 2: AI Consumption Impact (Src: PwC Analysis)
Deriving from the detailed PwC analysis report, which includes an AI impact index rating which gives an indication of enhancing quality and personalization for consumers and freeing up their time to invest their preferences in other endeavours.
The areas with the biggest potential representation will help your business target investment in the short to medium term. Some aspects could be even more disruptive or even revolutionary, such as robotic doctors, but they would be further off in the timeline of mainstream implementation.
Is the Differential for Developing countries like India too steep in catching up with AI? – AI is still at its early stages, which means that irrespective of the fact that the technology landscape is skewed towards the developed economies as compared to developing, the developing economies and their markets could still lead the developed markets from AI standpoint. This makes countries like India, the second largest economy with a strong focus in IT sector, a strong contender.
The economic impact of AI in GDP for developing countries, will be driven by:
- Productivity gains from businesses automating processes (including use of robots and autonomous vehicles).
- Productivity gains from businesses augmenting their existing labour force with AI technologies (assisted and augmented intelligence).
- Increased consumer demand resulting from the availability of personalised and/or higher-quality AI-enhanced products and services.
The consumer revolution set off by AI opens the way for massive disruption as both established businesses and new entrants drive innovation and develop new business models. A key part of the impact of AI will come from its ability to make the most of parallel developments such as IoT connectivity.
India’s Macroeconomic Landscape of AI
India is already way ahead of many other countries in implementing artificial intelligence (AI). More than half of the companies are going beyond pilot and test projects and adopting the technology at a larger scale. This statistic is largely driven by American firms such as Accenture, Microsoft, the successful implementers’ toolkit, said. Last year, India was the second-largest global site for new centres, after the US.
Well over 58% of the companies that are using AI in India are working with the technology at significant scale
The Indian government’s Digital India initiative, too, has created a favourable regulatory environment for increased use of AI.
Recipe for AI Success in India – Digital & Data Bedrock
As India undergoes rapid digital transformation, data centres and the intelligence behind the data collected will enable the government and industry to make effective decisions based on algorithms. This means increasing opportunities for using (and investing over) AI in the country.
For example, Intel is betting on Artificial Intelligence (AI) to drive demand for its electronic chips, for which it is aiming to train 15,000 scientists, developers, engineers and students on AI in India over the next one year. The company will host 60 courses under its ‘AI Developer Education Program’. These will train people on ways they can adopt AI for better research, testing or even building of products. Intel is looking at India due to the country’s large base of technical talent. The country is the third largest global site for AI companies.
As India’s largest e-commerce marketplace Flipkart closes in on completing a decade in the business, it is looking to put in use its mammoth pile of data to predict sales of products months in advance. The company is working on an artificial intelligence (AI) solution that will give it an edge over rivals by helping it make smarter decisions in ordering, distribution and pricing products on its platform. Ultimately, the AI system will allow Flipkart to boost efficiency and reduce the cost of products for customers.
While rival Amazon, which has around a 10-year headstart over Flipkart, is known to have some of the most advanced sales prediction engines, the Indian company has the advantage of having a bigger data set of the country’s online consumer market.
AI Inroads in the Private Sector
AI has now a significant impact in the day to day lives of the regular mass of the country. Now that the Indian IT sector has reached a certain intermediary peak of digitization, the focus, now , is more on automating the repetitive problems and finding more optimized, efficient or refined methods of performing the same tasks, with less time duration and lesser manpower. The result is the standardization of some very critical app based services like virtual assistants, cab aggregators, shopping recommendations etc. This will eventually lead to AI solutions to real world problems.
The AI Startups Sphere of India- Startups are clearly playing a major role in innovating faster than corporates, which has led to several curious partnerships. SAP India has invested in Niki.ai, a bot that improves the ordering experience. Then there’s Ractrack.AI, where a bot improves customer engagement and provides insights; it functions as a virtual communications assistant to convert the customer into a client. Racetrack is helping companies turn leads into meaningful engagements by using AI. Another startup, LUCEP, converts all potential queries into leads with their AI engine.
The objective is to generate insights from data and simplify customer interaction with a business and also convert them into leads.
Indian startups saw $4 billion in risk capital being deployed across 1,040 angel and VC/PE deals between January and December 2016.
Disclosed funding announcements have shown a decreased value of 55 percent from the same period last year (2015) and a decrease of 20 percent from 2014. About $9 billion in VC/PE capital had been invested in 2015. The number of deals in 2016, however, has increased by 3 percent over the last year. On an average, four startup deals were announced every weekday throughout 2016. VCs predict that going forward machine learning and AI would be key themes to invest in.
AI in Public Sector– Ripe for Digital Revamp and AI Adoption
A Blue Ocean for AI Investment due to Digital India Initiatives – Though both corporates and startups are making significant inroads in instituting AI in their service architecture and product offerings, and sometimes as part of their core business strategy itself, the challenges in the public sector in instituting AI can be quickly overcome due to huge Digital Movements instituted by the Indian Govt. like Digital India, Skill India and Make in India. This will create a solid bedrock of Data and Digital Footprint which will act as a foundational infrastructure to base AI implementation on, opening a huge blue ocean in public sector, rich for AI investment.
A New Workaround for Regulatory Challenges in Public Sector AI Implementation – One of the peculiar problems the public sector faces in mainstream implementation of AI is the fact that since AI is a continuously self-learning system, capable of analytical or creative decision making and autonomous implementation of actions, who will then be accountable in taking responsibility for its actions, should they turn out to be not so favourable. This is because of the fact that since AI has a degree of autonomous decision making, it makes it difficult to pre-meditate its consequence. The AI systems are meant to augment and enrich the life of the consumers. In such a situation, deciding liability of AI system’s actions will be difficult. Therefore, a lot of deliberation will be required to carefully come to a precise conclusion surrounding implementing these systems with ethical foundation and propriety.
Although many countries like US and some European countries are in the verge of implementing regulations and laws surrounding concepts like driverless vehicles, India still don’t have the regulations sanctioned. This, but need not be a bad news. India is cut to establish a completely revamped legal infrastructure, thereby completely circumventing the need for continuous regulatory intervention. Also, there is a favourable atmosphere in India as far as AI is concerned which will foster a spike in activities in that avenue.
Indian Governance Initiatives – Huge Scope for Investment of AI – As India emerges as a premier destination for AI, scope for investment opens in the governance aspect, in several ways. Governance schemes have a unique trait of the baggage of large volume and large scale implementation need, which can be tackled with Deep learning. For example, in Swachh Bharat Initiative, specifically construction of toilets in rural India, public servants are tasked with uploading images of these toilet constructions to a central server for assessment. Image recognition can be used to target unfinished toilets. It can also be used to identify whether the same official appears in multiple images or if photos were uploaded from a different location other than the intended place.
Other initiatives such as the Make in India, Digital India & Skill India can be augmented with AI to deal with scale. The range of application for AI techniques could range from crop insurance schemes, tax fraud detection, and detecting subsidy leakage and defence and security strategy.
An AI system can improve and enrich the agriculture of India by enhancing the bodies like The Department of Agriculture Cooperation and Farmers Welfare, Ministry of Agriculture runs the Kisan Call Centers across the country etc. It can help assist the call centre by linking various available information like soil reports from government agencies and link them to the environmental conditions. It will then provide advice on the optimal crop that can be sown in that land pocket.
As the need for large scale implementation and monitoring of governance initiative becomes more pronounced, the need for AI becomes absolute and it will open doors to considerable AI investment in the future of India.
Finally, Looking Ahead – A Collaborative Innovation Environment due to AI
AI innovations which fall under assisted, augmented and autonomous intelligence will help users understand and decide which level of intelligence is helpful and required in their context, thereby making AI Acceptance easier for the people. At the same time, this AI continuum can be used to understand economic ramifications, usage complexity and decision-making implications. While academia and the private sector conduct research on various AI problems with diverse implications in mind, the public sector with its various digital initiatives (Digital India, Make in India, etc.) can identify areas where parts of the AI continuum can be utilised to increase reach, effectiveness and efficiency, thereby giving direction to AI Innovative Research.
A collaborative innovation environment between academia and the private and public sectors will help provide holistic and proactive advisory delivery to the population, for eg. through public call centres, linking information from various government sources. At the same time, the rich data generated from these interactions can be used to draw deep conclusions. Collaboration between the three pillars could further help get a comprehensive view of problems and find intelligent and innovative ways to increase the efficiency and effectiveness of services delivered to society.
Related Posts
AIQRATIONS
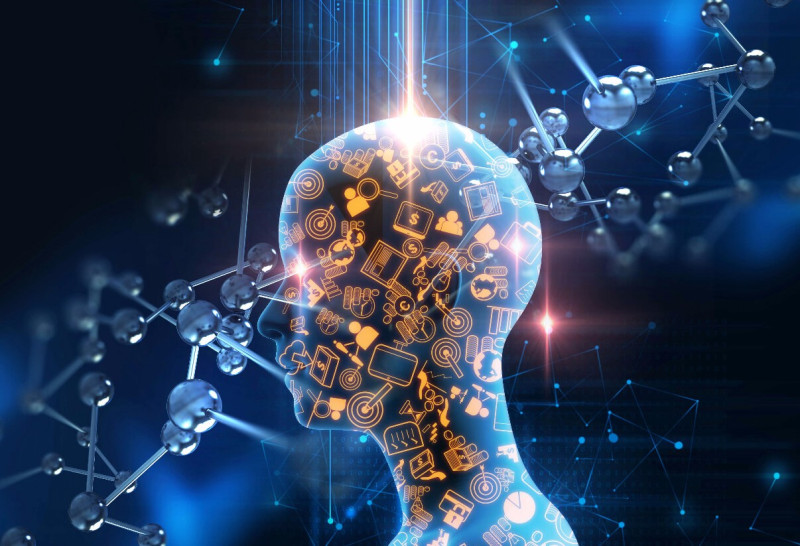
Understanding Burgeoning AI Landscape; A Perspective for PE/VC & Investment Funds
Add Your Heading Text Here
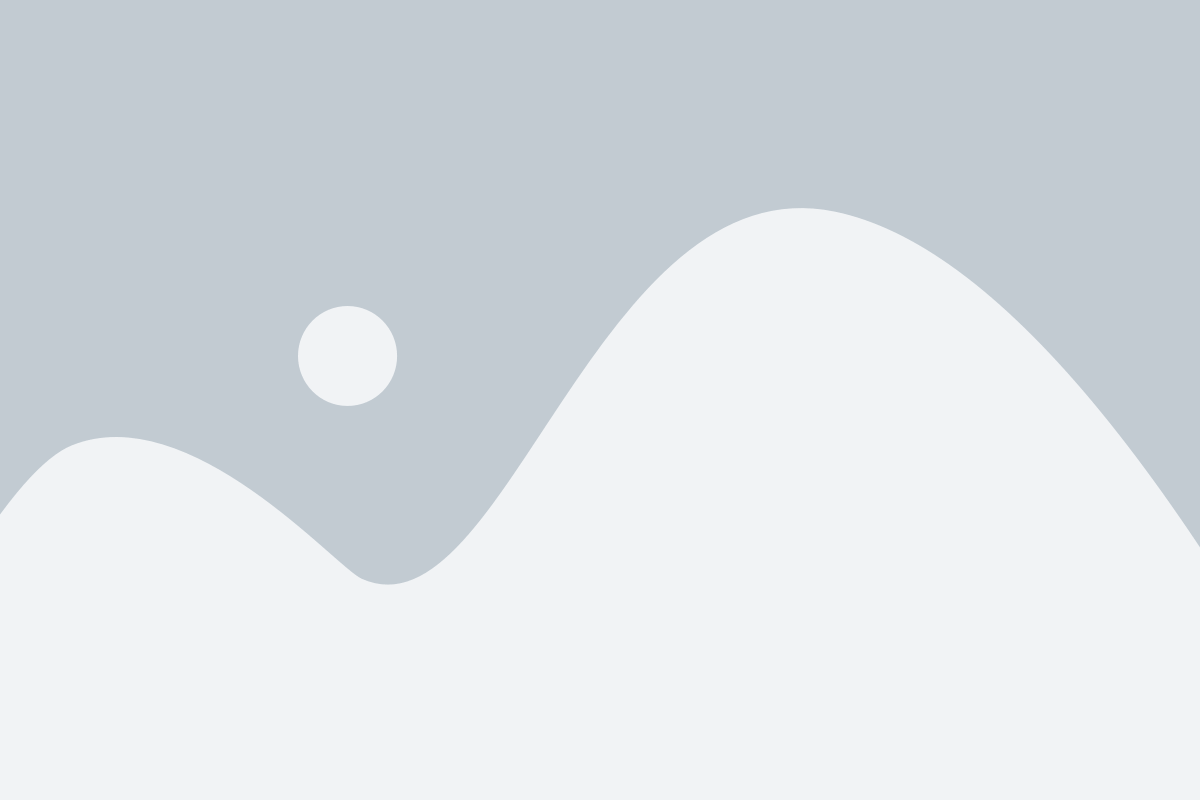
There seems to be a glaring ambiguity as to exactly what artificial intelligence (AI) is, and how the discipline of AI should be categorized. Is AI a form of analytics or is it a totally new discipline that is distinct from analytics? I firmly believe that AI is more closely related to predictive analytics and data sciences than to any other discipline. One might even argue that AI is the next generation of predictive analytics and is born out of sophistication of analytics . Additionally, AI is often utilized in situations where it is necessary to operationalize the analytics process. So, in that sense, AI is also often pushing the envelope of prescriptive, operationalized analytics. It would be a mistake to say that AI is not a form of analytics.
I’ve seen AI applied to some of the most obscure topics you can imagine, ranging from industrial energy usage all the way to finding the right GIFs. Using Artificial Intelligence to improve and create solutions to today’s pressing business and social problems is one of the defining trends of the tech world for me.
So, if you are a PE / VC entity and are looking for investment opportunity in AI space, you will have to understand what kinds of AI companies exist and how this AI practice has evolved from Analytics practice.
There are three types of AI companies — core, applied, and industry
1. Core AI Companies
Core AI companies develop technology that improves parts of the AI creation or deployment process itself. Here are a few selected parts of that process and a few companies that are innovating in each:
Data scrubbing and cleaning: Trifacta, Paxata, Wealthport, Datalogue
Modeling: Sentient, Petuum, MLJar
Deployment: Yhat, Seldon
These companies all innovate in some specific, industry-agnostic part of the AI pipeline. Some of them are specific tools, while others purport to have an entirely new approach to AI that will revolutionize how it’s done (see Geometric Intelligence circa 2015).
If you’re investing in Core AI companies, you should probably have a good understanding of how this pipeline works. If you’re founding one of these companies, you should probably have experience deploying Machine Learning and AI at scale.
2. Applied AI Companies
A bit on the more specific side, Applied AI start-ups develop technology that helps companies across different industries perform a specific task using AI. As with the above, here are some examples of those applications and a few interesting companies in each:
Analysing and understanding text: Indico, Synapsify, Lexalytics
Analysing and understanding images and videos: Clarifai, Kairos, Imagry, Affectiva, Deepomatic
Bots / Voice: Init.ai, MindMeld
While investors can get away with not having experience in one of these specific applications, founders will likely have done projects involving this stuff in the past.
The implementation of AI in this scenario corresponds to the implementation of predictive analytics. At its core, predictive analytics is, naturally, about predicting something. Who will buy? Will certain equipment break? Which price will maximize profits? Each of these questions can be addressed by following a familiar workflow – First, we identify a metric or state that we want to predict and gather historical information on that metric or state. Next, we gather additional data that we believe could be relevant to predicting our target. Then, we pass the data through one or more algorithms that attempt to find a relationship between the target and the additional data. Through this process, a model is created that produces a prediction if new data is fed to it. If a customer had this profile, how likely would she be to respond? If we priced at this point, how much profit might we expect?
The goals and steps followed within an AI process are the same. Let’s look at two examples:
Take image recognition. First, we identify a bunch of cat pictures. Then, we grab a bunch of non-cat pictures. We pass a deep learning algorithm over the images to learn to accurately predict whether an image is a cat. When provided with a new image, the model will answer with the probability that the image is a cat. Sounds a lot like predictive analytics, doesn’t it?
Let’s now consider natural language processing (NLP). We gather a wide range of statements that have specific meanings we care about. We also gather a wide range of other statements. We run NLP procedures against the data to try to tease out how to tell what is important and how to tell what is being asked. As we feed a new line of text to the process, it will identify what the point of the statement is in probabilistic terms. The NLP process will assign probabilities to various possible interpretations and send those back (think Watson playing jeopardy). This also sounds a lot like predictive analytics.
3. Industry AI Companies
The final category of ML/AI companies apply these techniques to specific business problems in specific verticals. This is undoubtedly the lion’s share of the actual number of companies being founded, and in many ways, represents the true promise of AI — solving actual and immediate problems with new techniques. Here, it’s easier to give companies as examples. The format is always “AI for ________”:
DigitalGenius: AI for customer support
Cylance: AI for cyber threat prevention
X.ai: AI for scheduling meetings
Drive.ai: AI for autonomous vehicles
The implementation of AI in this scenario corresponds to industrialized embedded analytics. A major trend today is to embed analytics into business applications so that the models are utilized in an automated, embedded, prescriptive fashion at the point of a business decision. For example, as a person navigates a web page, models are utilized to predict what offers should appear on the next page. There is no human intervention once the process is in place. The process makes offers until told to stop.
Many applications of AI today also require industrialization. For example, as an image is posted on social media, it is immediately analysed to identify who is present in the image. As I make a statement to Siri or Alexa, it attempts to determine what I said and what the best answer is. While this qualifies as a more advanced application of predictive analytics that moves into embedded, prescriptive, automated processes, it is still very much in line with how industrialized embedded analytics are being used today.
The common theme among these companies is that they take Machine Learning / AI and use it on a specific problem or space. When researching investments like this, investors should look at both the AI itself (if it works well) and the business case (whether it’s compelling). In x.ai’s case, investors need to know if the AI works, but they should also consider whether AI is the best way to solve the scheduling problem, and whether scheduling is a problem worth solving at all. With the other two types of companies, this is rarely a consideration. Founders of these types of companies can often not have AI experience and can even be non-technical (with the right supporting team and CTO, of course).
Final Word
Your journey to a fruitful AI investment will be far easier if you recognize and embrace AI as sophistication of analytics and understand the true categorization, and then task your analysts with leading the charge. Don’t cause confusion and redundancy by considering AI to be something completely different.
Related Posts
AIQRATIONS
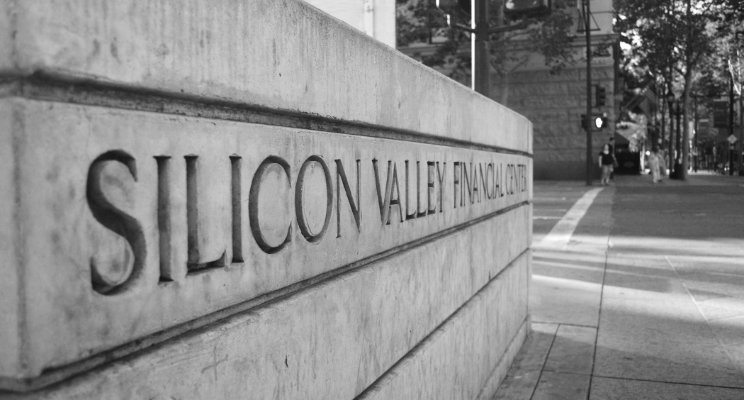
The gold rush for AI – silicon valley vs. China – a perspective hard to ignore
Add Your Heading Text Here
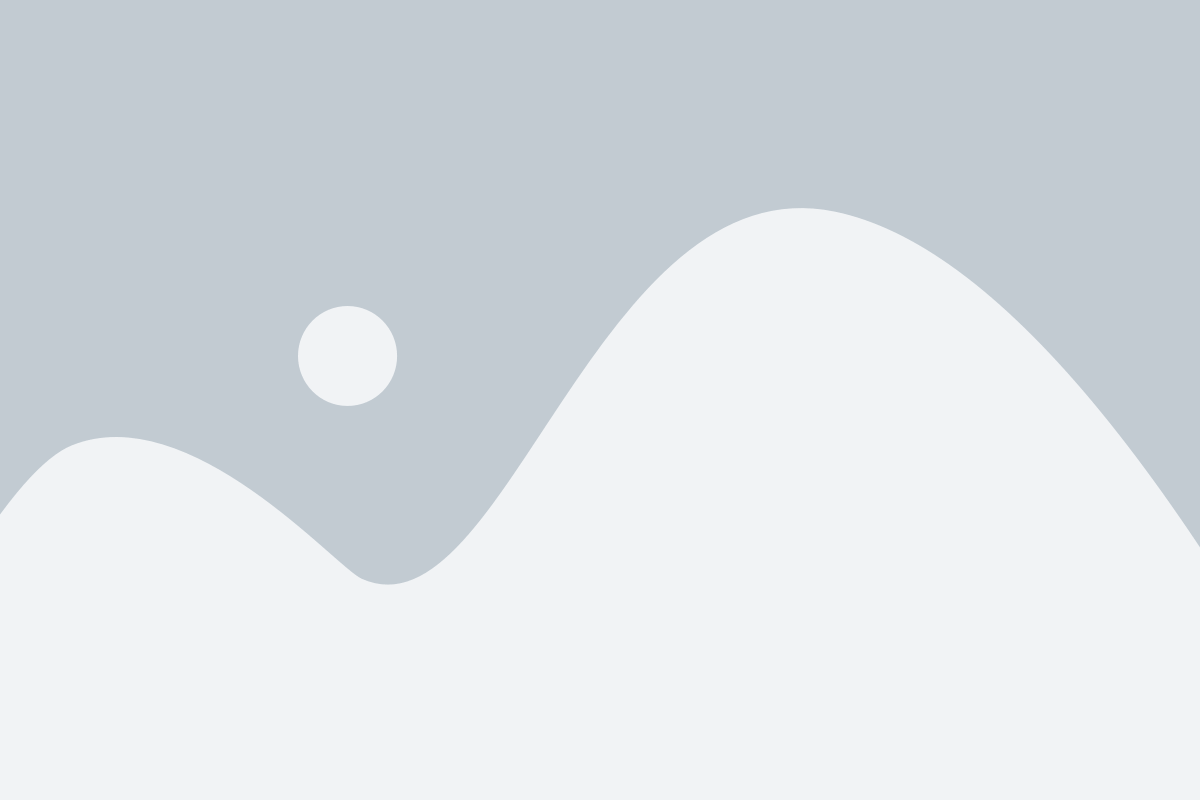
The buzzword among the business and tech communities in China for the past year has been ‘AI’, or artificial intelligence. Artificial intelligence, which allows software to “learn” human ways of thinking, is being incorporated into the largest e-commerce platforms, including Baidu, Alibaba, and Tencent, as well as into data-intensive traditional sectors. With strong government backing and concentrated research in this area, AI is poised to drive China’s economy forward toward higher levels of growth.
China is developing artificial intelligence in improving the capabilities of robotics, developing driverless cars, divining consumer preferences, inventory forecasting, selling enhanced products, and marketing goods and services. According to Liu Lihua, Vice Minister of Industry and Information Technology, China has thus far applied for 15,745 AI patents.
China plans to launch a national AI plan, which will strengthen AI development and application, introduce policies to contain risks associated with AI, and work toward international cooperation. The plan will also provide funds to back these endeavors. Some municipalities also support AI research programs. Beijing, for example, is home to the CAS Institute of Automation, a consortium of universities and firms that provides venture capital funding of 1 billion RMB ($150 million) to AI development. Zhejiang province has also embraced AI programs. Already, Geely Automobile in Zhejiang is using intelligent manufacturing and internet marketing services based on AI to boost sales.
BAT – Chinese AI Frontier Giants
China’s BAT, or Baidu, Alibaba and Tencent, is leading the way for AI in China. Baidu was the first Chinese company to embark upon research in AI, using a system known as Duer to be used in home devices and driverless cars. Driverless auto software provided by Baidu will be made available to car manufacturers under the Apollo Project. Alibaba is using AI to forecast regional order quantities and to improve logistics efficiency, while Tencent has released a platform for deep learning using social data.
Baidu, Alibaba and Tencent have been vying for top talent in AI in order to become leaders in this area. Making headlines several days ago, Alibaba lured Ren Xiaofeng from Amazon.com to lead its own technology lab, which aims to make headway in artificial intelligence. Tencent brought Baidu’s AI expert Zhang Tong on board in March. In 2014, Baidu poached Andrew Ng from the Google Brain project to lead the Baidu Research Institute (though he recently stepped down).
Bay Area dominates this year’s AI funding
Venture investment in startups that are applying artificial intelligence or machine learning has more than tripled in the U.S. since 2013, according to PitchBook Data, with about 60 percent of that coming to founders in the Silicon Valley Bay Area.
The Seattle investment research firm put together a ranking of the top 20 AI deals done around the world this year for me while I was researching this week’s Silicon Valley Business Journal cover story. Almost half of the startups that were funded and nearly three-quarters of the investors involved were from San Francisco and the Silicon Valley region.
The new era in Silicon Valley centers on artificial intelligence and robots, a transformation that many believe will have a payoff on the scale of the personal computing industry or the commercial internet, two previous generations that spread computing globally. Computers have begun to speak, listen and see, as well as sprout legs, wings and wheels to move unfettered in the world.
Silicon Valley’s financiers and entrepreneurs are digging into artificial intelligence with remarkable exuberance. The region now has at least 19 companies designing self-driving cars and trucks, up from a handful five years ago. There are also more than a half-dozen types of mobile robots, including robotic bellhops and aerial drones, being commercialized.
Funding in A.I. start-ups has increased more than fourfold to $681 million in 2015, from $145 million in 2011, according to the market research firm CB Insights. The firm estimates that new investments will reach $1.2 billion this year, up 76 percent from last year.
Even Silicon Valley’s biggest social media companies are now getting into artificial intelligence, as are other tech behemoths. Facebook is using A.I. to improve its products. Google will soon compete with Amazon’s Echo and Apple’s Siri, which are based on A.I., with a device that listens in the home, answers questions and places e-commerce orders. Satya Nadella, Microsoft’s chief executive, recently appeared at the Aspen Ideas Conference and called for a partnership between humans and artificial intelligence systems in which machines are designed to augment humans.
The auto industry has also set up camp in the valley to learn how to make cars that can do the driving for you. Both technology and car companies are making claims that increasingly powerful sensors and A.I. software will enable cars to drive themselves with the push of a button as soon as the end of this decade — despite recent Tesla crashes that have raised the question of how quickly human drivers will be completely replaced by the technology.
AI is in it for the long-haul
Whenever there is a new idea, the valley swarms it. But you have to wait for a good idea, and good ideas don’t happen every day. Silicon Valley’s new A.I. era underscores the region’s ability to opportunistically reinvent itself and quickly follow the latest tech trend. This is at the heart of the region’s culture that goes all the way back to the Gold Rush. The valley is built on the idea that there is always a way to start over and find a new beginning.
Related Posts
AIQRATIONS
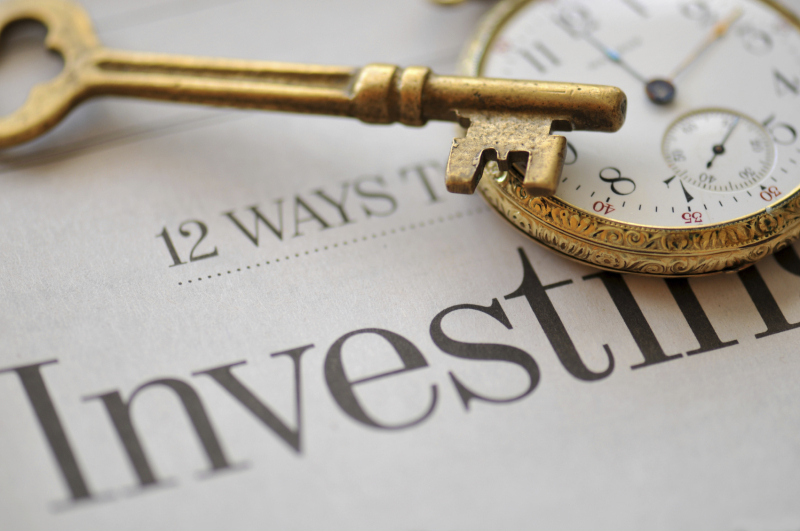
Investment Analytics for VC & PE Firms
Add Your Heading Text Here
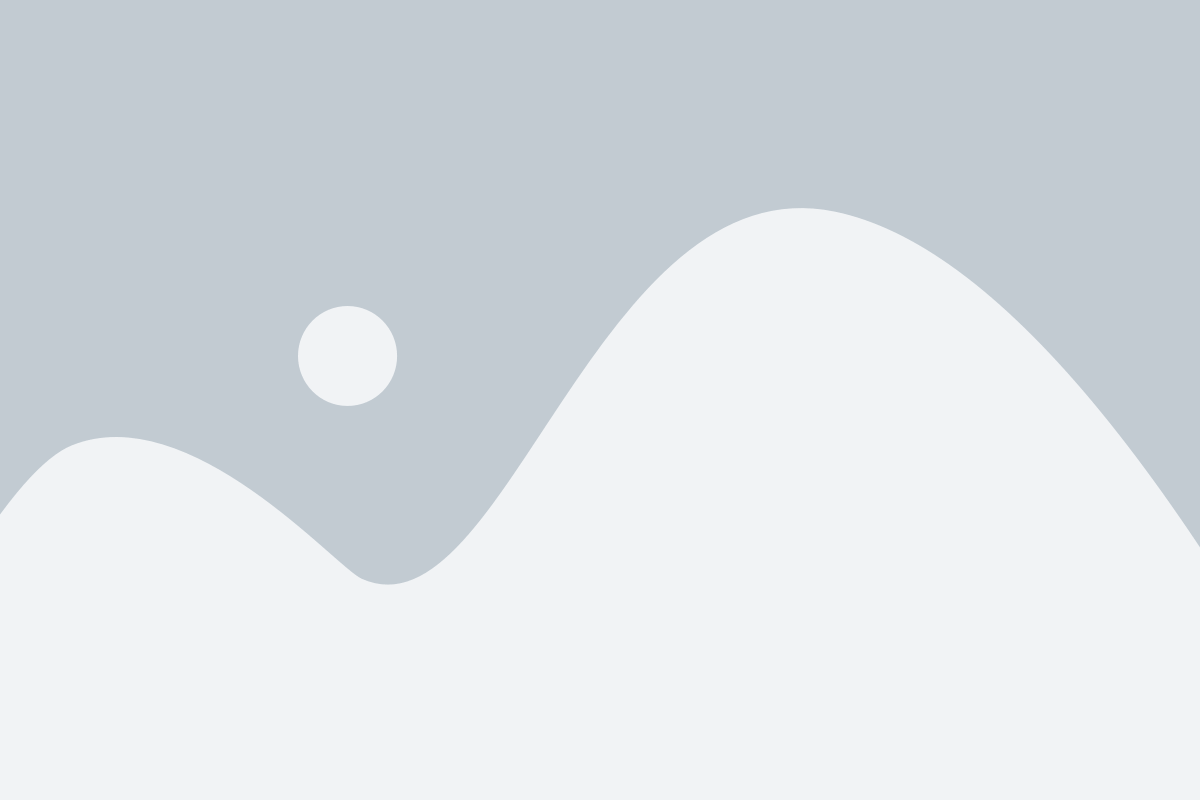
In the venture capital world, it’s all about the “hits.” A hit is a startup that makes it big, returning many multiples of a venture fund’s initial investment. Hits are great for everyone—investors, entrepreneurs, job seekers—but the problem is they don’t happen very often. The odds of a big hit are about one in 10.
Boosting the odds for VCs
But what if venture capital could boost its odds to 50-50, or even two out of three? With $48 billion in VC investment in 2014, such an improvement would prevent huge amounts of money from being lost on startups that never had much of a chance of surviving the harsh competitive environment. The challenge is to identify those likely laggards well before the market rejects their idea and, perhaps more importantly, to see the big hits before anyone else. Venture capital has long relied on subjective, intuitive methods of assessing startups, but that’s changing as more firms are bringing data science and consistency into their decision-making.
The next is to try to do the science, build the tools, and do the research all around this one question: How can we better predict when innovations will survive or fail, both for startups and when corporations launch new products or do acquisitions? There’s no human subjectivity involved anywhere along the line. All the algorithms converge on a discrete yes or no. That yes or no depends on majorly two areas – those inside the startup, and those external to the startup. Only around 20% of the predictive value to come from details specific to the startup itself whereas 80% comes from things outside of the startup, such as the market, customers, competitors, technology trends, and timing.
In this scenario, managers are trying to predict the future before investing money in it. According to analysis of data from the Small Business Administration, and data on startups, between 20% and 30% of new businesses survive to their 10th birthday. Startups with VC-backing, aren’t doing much better than spin-offs from large corporations. Has the entrepreneurship economy gotten any better at predicting any business? Data suggest no, not in any statistically significant amount. But Investment Analytics is turning that around. When it comes to predicting survivorship of companies after a 10-year period, implementing investment analytics at present setup can point you to the right answer 67% of the time, and totally wrong on the remaining third. So, If investment analytics has vastly improved the odds of investing in new technologies and businesses, why isn’t the entire VC world knocking on its door? Because A lot of venture capitalists, like the scouts in Moneyball reacting to sabermetrics, are skeptical. Most of the people don’t see quants taking over VC, even in the distant future, but they do see the potential of using data to help venture capitalists make decisions.
Data analytics are undoubtedly creeping into venture capital—Google Ventures uses an algorithm to help with investment decisions, and a Silicon Valley firm called Correlation Ventures is built upon an algorithmic investing strategy. But the old-fashioned process of detailed research and human judgment still has a lot going for it. Just ask the people at Lux Research, an emerging-technology consulting firm in Boston. For the past 10 years, Lux’s science-trained analysts have been scouring the business landscape for new technology firms, interviewing employees of those firms, and slowly compiling their own database of companies that succeeded or failed. Lux rates each company it profiles according to nine key factors, which are available to the public on its website in a report called “Measuring and Quantifying Success in Innovation. The result of that rating is a company profile with a “Lux Take,” which ranges from “strong positive” to “strong caution.”
The company recently looked back at five years’ worth of profiles and found that 50% of the companies that earned a “positive” rating went on to be successful, an outcome which Lux defines as an IPO, acquisition, or transition to standalone profitability. Given the usual odds of new business survival, the Lux system seems to inject a significant amount certainty into the process of evaluating startups. The company’s high rate of accuracy is attributable to two things: capability and methodology.
People talk a lot about the importance of innovation to economic growth. In a recent survey of voters in swing states by the Economic Innovation Group, 75% of those surveyed agreed that America needs more entrepreneurs and investors in order to improve long-standing economic problems. The innovation economy has an information problem. The information that drives it isn’t good. How can countries become innovation economies in a more efficient way? Let’s get better at funding the startup companies that will grow and drive employment. For every dollar that goes in the wrong place, that’s a bad dollar.
Better Investment Strategy for PEs
Data, and specifically the analysis of data is becoming a critical component in enabling private equity firms to create and maintain value for their investors. I know some of you are thinking, “What does a data management geek know about private equity?” One thing to note about private equity firms is that there has been a greater focus on IT and a growing need for data analytics services recently, especially for deals that involve middle-market companies and/or are add-ons to existing platforms. Increasing acquisition valuations and the dearth of available debt financing are making it increasingly difficult for private equity firms to generate outsized returns for their investors. This is causing private equity firms to focus more on operational improvements within their portfolio companies as a means of driving growth and value creation. Given the strong correlation between operational improvement and higher returns for limited partners, private equity managers’ hands-on involvement is, and will continue to be, critical to the future success of the fund. For private equity managers, this means identifying key drivers of EBITDA, understanding customer behavior, developing competitor analyses, determining the best place to invest capital, managing budgets, and controlling costs—for each of their portfolio companies. And to do it effectively and efficiently, managers must leverage data.
Nobody said it was going to be easy and the fact is: it’s not. Unfortunately, most of the private equity managers are not in a position to dig deep into the recesses of their portfolio company systems and extract the data tables necessary to produce key operational metrics. Therefore, managers will have to rely on the current workforce at each of their portfolio companies or hire an outside party to do the ‘dive into the data’ for them. Many of them struggle with capturing, extracting, and ultimately analyzing operational data within their businesses. This is largely due to the fact that, historically, middle-market companies have rarely made technology a priority, leaving them with an unsophisticated IT platform. As a result, when dealing with legacy and perhaps “home-grown” solutions, there are a variety of challenges that arise when attempting to harmonize, integrate, and perform data analytics. Additionally, many middle-market companies are experiencing data volumes that are growing exponentially and are held in disparate systems throughout the organization. In short, private equity managers are faced with massive amounts of “dirty” data that must be converted into meaningful metrics. This leaves private equity firm managers asking themselves, “How much time and money do I need to commit to information technology and data analytics?” Answer: more than you have in the past. The key is to invest wisely and in a way that will get you the greatest returns in the shortest amount of time. There are an infinite number of technology solutions out there. From off-the-shelf, in-the-box solutions to massive enterprise resource planning (ERP) solutions, software companies have done their best to provide an answer to your “big data” problems. Although there are many great tools out there, there is no cookie-cutter solution when trying to capitalize on the presumed synergies of integration. Private equity managers must understand the specific business needs and work to develop strategic analytical solutions driven from the data that already exists in their organization. It is critical, especially in today’s competitive market, to “leverage your data” and apply these targeting solutions in a way that will provide the greatest returns for your investors.
Big data may indeed be able to help, but it’s more likely to be a piece of the puzzle, not the solution. For instance, academic studies have shown that serial entrepreneurs successful in the past are more likely to do well in new ventures. That implies there is some explanatory power in looking backwards for guidance on what’s ahead. “But the nature of entrepreneurship is always changing,” says Josh Lerner, the Jacob H. Schiff Professor of Investment Banking at Harvard Business School. Most regressions predicting entrepreneurial success in the literature have very low goodness of fit, which suggests the limits of a ‘Moneyball’ approach here. Predicting which startup is going to be successful is much harder than [predicting] which baseball player is. It is as if the baseball rules are being changed every year in unpredictable ways.”