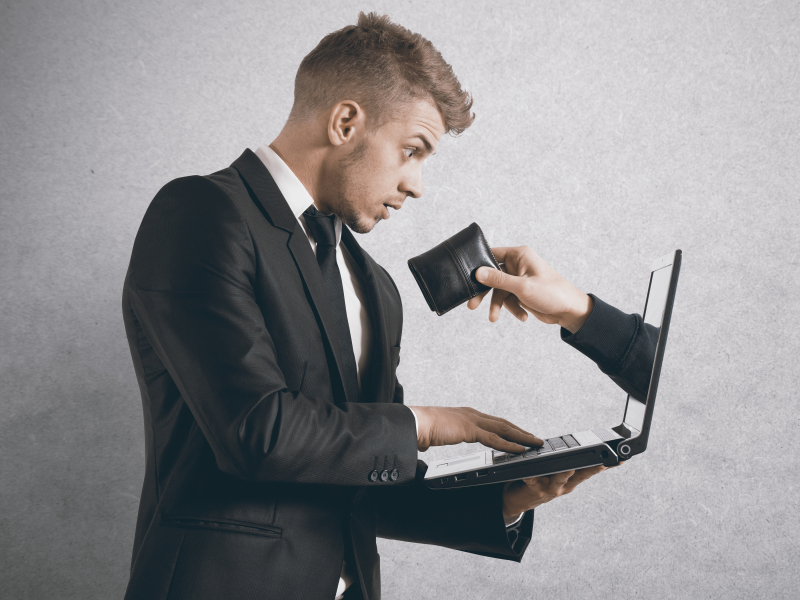
How AI is Enabling Mitigation of Fraud in the Banking, Insurance Enterprises
Add Your Heading Text Here
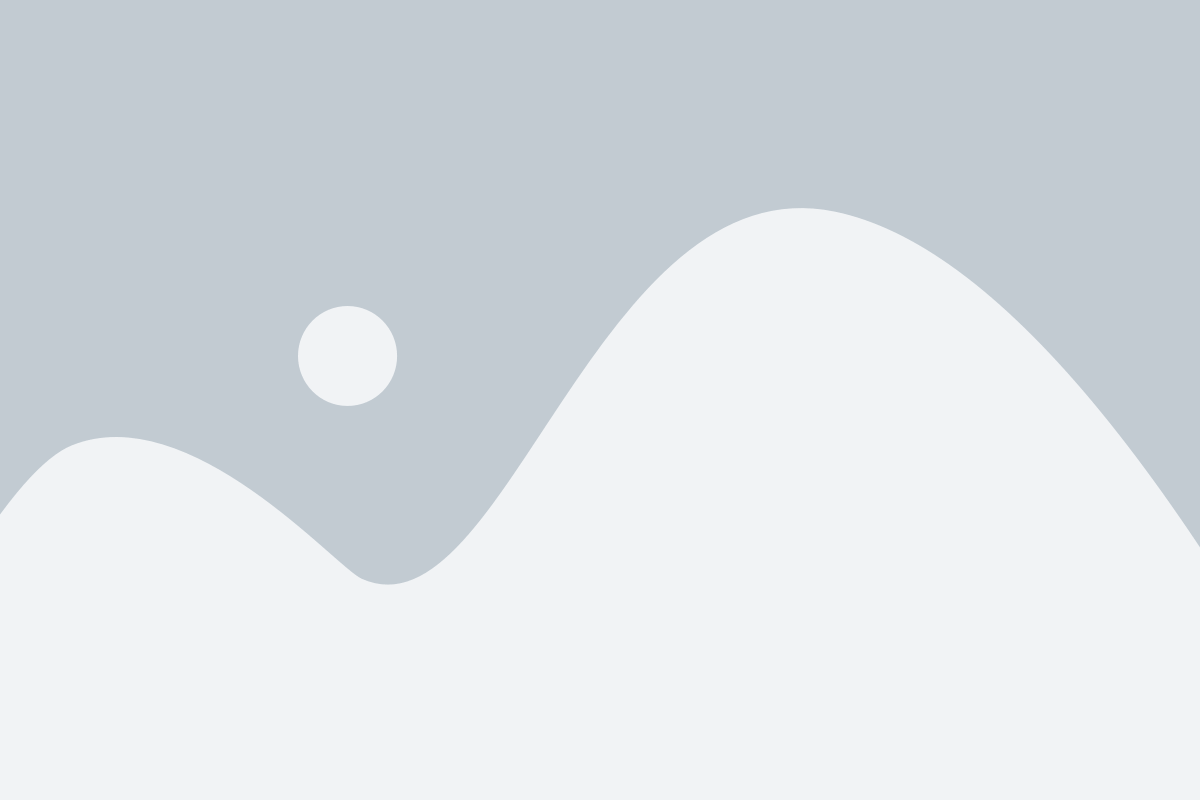
The Banking and Finance sector (BFSI) is witnessing one of its most interesting and enriching phases. Apart from the evident shift from traditional methods of banking and payments, technology has started playing a vital role in defining this change.
Mobile apps, plastic money, e-wallets and bots have aided the phenomenal swing from offline payments to online payments over the last two decades. Now, the use of Artificial Intelligence (AI) in BFSI is expediting the evolution of this industry.
But as the proliferation of digital continues, the number of ways one can commit fraud has also increased. Issuers, merchants, and acquirers of credit, debit, and prepaid general purpose and private label payment cards worldwide experienced gross fraud losses of US$11.27 billion in 2012, up 14.6% over the previous year1. Fraud losses on all general purpose and private label, signature and PIN payment cards reached US$5.33 billion in United States in the same period, up 14.5%1. These are truly big numbers, and present the single-biggest challenge to the trust reposed in banks by customers. Besides the risk of losing customers, direct financial impact for banks is also a significant factor.
Upon reporting of a fraudulent transaction by a customer, the bank is liable for the transaction cost, it has to refund merchant chargeback fee, as well as additional fee. Fraud also invites fines from regulatory authorities. The recently-passed Durbin Amendment caps processing fee that can be charged per transaction, and this increases the damage caused by unexpected fraud losses. The rapidly rising use of electronic payment modes has also increased the need for effective, efficient, and real-time methods to detect, deter, and prevent fraud.
Nuances of Banking Fraud Prevention Using AI
AI enables a computer to behave and take decisions like a human being. Coined in 1956 by John McCarthy at MIT, the term AI was little known to the layman and merely a subject of interest to academicians, researchers and technologists. However, over the past few years, it is more commonly seen in our everyday lives; in our smartphones, shopping experiences, hospitals, travel, etc.
Machine Learning, Deep Learning, NLP Platforms, Predictive APIs and Image and Speech Recognition are some core AI technologies used in BFSI today. Machine Learning recognises data patterns and highlights deviations in data observed. Data is analysed and then compared with existing data to look for patterns. This can help in fraud detection, prediction of spending patterns and subsequently, the development of new products.
Key Stroke Dynamics
Key Stroke Dynamics can be used for analysing transactions made by customers. They capture strokes when the key is pressed (dwell time) and released on a keyboard, along with vibration information.
As second factor authentication is mandatory for electronic payments, this can help detect fraud, especially if the user’s credentials are compromised. Deep Learning is a new area in Machine Learning research and consists of multiple linear and non-linear transformations. It is based on learning and improving representations of data. A common application of this can be found in the crypto-currency, Bitcoin.
Adaptive Learning
Adaptive Learning is another form of AI currently used by banks for fraud detection and mitigation. A model is created using existing rules or data in the bank’s system. Incremental learning algorithms are then used to update the models based on changes observed in the data patterns.
AI instances in Insurance for Fraud Prevention
Applying for Insurance
When a customer submits their application for insurance, there is an expectation that the potential policyholder provides honest and truthful information. However, some applicants choose to falsify information to manipulate the quote they receive.
To prevent this, insurers could use AI to analyse an applicant’s social media profiles and activity for confirmation that the information provided is not fraudulent. For example, in life insurance policies, social media pictures and posts may confirm whether an applicant is a smoker, is highly active, drinks a lot or is prone to taking risks. Similarly, social media may be able to indicate whether “fronting” (high-risk driver added as a named driver to a policy when they are in fact the main driver) is present in car insurance applications. This could be achieved by analysing posts to see if the named driver indicates that the car is solely used by them, or by assessing whether the various drivers on the policy live in a situation that would permit the declared sharing of the car.
Claims Management & Fraud Prevention
Insurance carriers can greatly benefit from the recent advances in artificial intelligence and machine learning. A lot of approaches have proven to be successful in solving problems of claims management and fraud detection. Claims management can be augmented using machine learning techniques in different stages of the claim handling process. By leveraging AI and handling massive amounts of data in a short time, insurers can automate much of the handling process, and for example fast-track certain claims, to reduce the overall processing time and in turn the handling costs while enhancing customer experience.
The algorithms can also reliably identify patterns in the data and thus help to recognize fraudulent claims in the process. With their self-learning abilities, AI systems can then adapt to new unseen cases and further improve the detection over time. Furthermore, machine learning models can automatically assess the severity of damages and predict the repair costs from historical data, sensors, and images.
Two companies tackling the management of claims are Shift Technology who offer a solution for claims management and fraud detection and RightIndem with the vision to eliminate friction on claims. Motionscloud offer a mobile solution for the claims handling process, including evidence collection and storage in various data formats, customer interaction and automatic cost estimation. ControlExpert handle claims for the auto insurance, with AI replacing specialized experts in the long-run. Cognotekt optimize business processes using artificial intelligence. Therefore the current business processes are analyzed to find the automation potentials. Applications include claims management, where processes are automated to speed up the circle time and for detecting patterns that would be otherwise invisible to the human eye, underwriting, and fraud detection, among others. AI techniques are potential game changers in the area of fraud. Fraudulent cases may be detected easier, sooner, more reliable and even in cases invisible to the human eye.
Conclusion
Those who wish to defraud insurance companies currently do so by finding ways to “beat” the system. For some uses of AI, fraudsters can simply modify their techniques to “beat” the AI system. In these circumstances, whilst AI creates an extra barrier to prevent and deter fraud, it does not eradicate the ability to commit insurance fraud. However, with other uses of AI, the software is able to create larger blockades through its use of “big data”. It can therefore provide more preventative assistance. As AI continues to develop, this assistance will become of greater use to the insurance industry in their fight against fraud.
Related Posts
AIQRATIONS
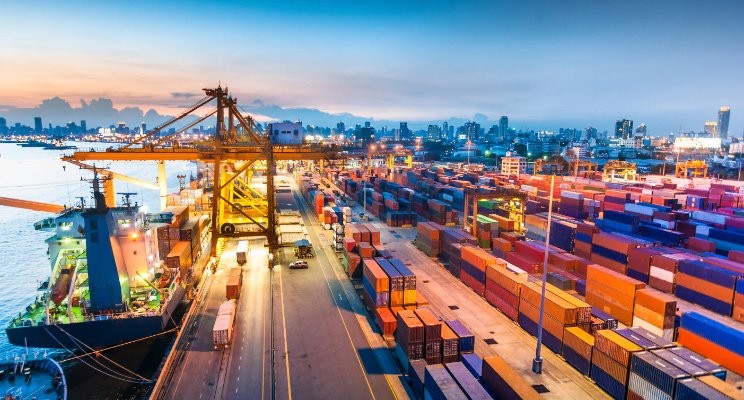
Fluid Supply Chain Transformation = AI + Automation
Add Your Heading Text Here
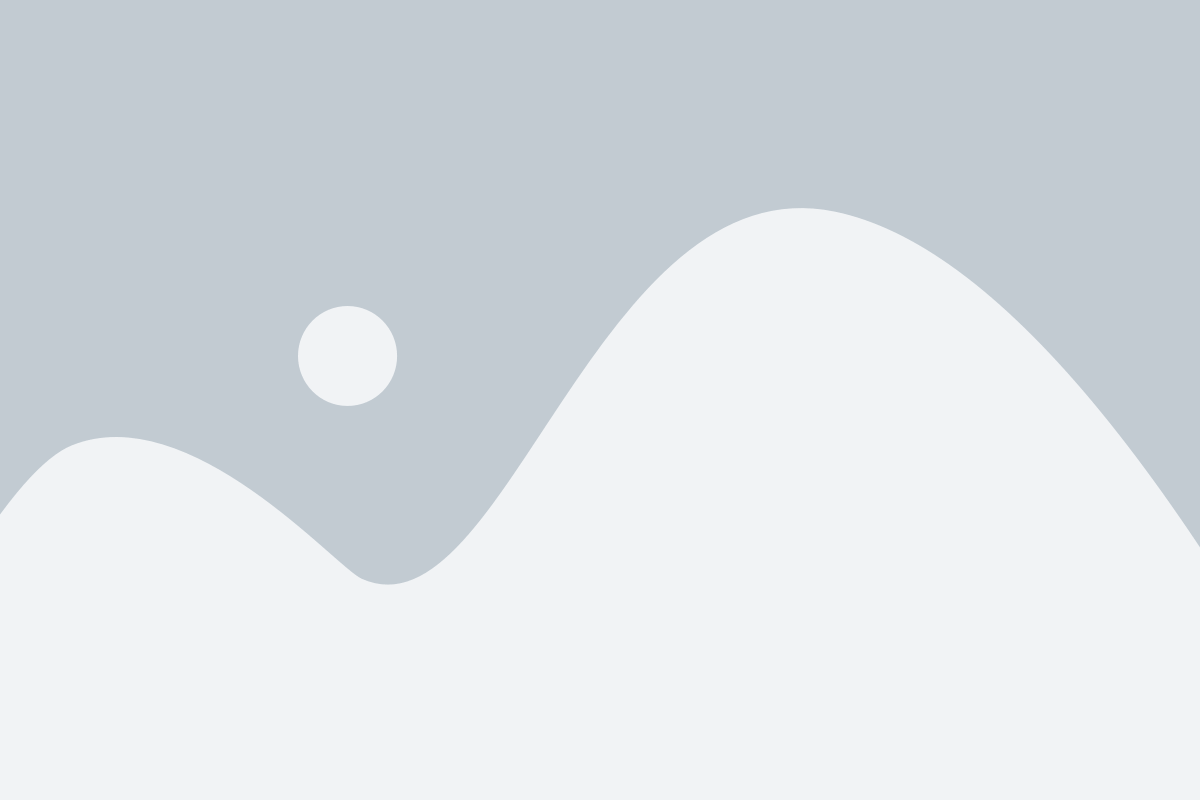
Rapidly evolving technology and a digitally focused world have opened the door for a new wave of automation to enter the workforce. Robots already stand side-by-side with their human counterparts on many manufacturing floors, adding efficiency, capacity (robots don’t need to sleep!) and dependability. Add in drones and self-driving vehicles and it’s no wonder many are questioning the role of humans going forward.
Supply chains, although automated to a degree, still face challenges brought about by the amount of slow, manual tasks required, and the daily management of a complex web of interdependent parts. The next generation of process efficiency gains and visibility could be on your doorstep with artificial intelligence in supply chain management, if only you’d let the robots automatically open it for you.
Robotic Process Automation
RPA works by automating the end-to-end supply chain, enabling the management of all tasks and sections in tandem. It allows you to spend less time on low value, high frequency activities like managing day-to-day processes, and provides more time to work on high value, exception-based requirements, which ultimately drives value for the entire business.
PwC estimates businesses could automate up to 45% of current work, saving $2 trillion in annual wages. “In addition to the cost and efficiency advantages, RPA can take a business to the next level of productivity optimization,” the firm says. Those ‘lights out’ factories and warehouses are becoming closer to a reality.
Four key elements need to be in place for you to take full advantage of robotic process automation in your supply chain:
- robots for picking orders and moving them through the facility;
- sensors to ensure product quality and stock;
- cognitive learning systems;
- and, artificial intelligence to turn processes into algorithms to guide the entire operation.
In addition, you’ll need strong collaboration internally and among suppliers and customers to tie all management systems back to order management and enterprise resource planning platforms.
Artificial Intelligence In Supply Chain Automation
AI is changing the traditional way in which companies are operating. Siemens in its “lights out” manufacturing plant, has automated some of its production lines to a point where they are run unsupervised for several weeks.
Siemens is also taking a step towards a larger goal of creating Industrie 4.0 or a fully self-organizing factory which will automate the entire supply chain. Here, the demand and order information would automatically get converted into work orders and be incorporated into the production process.
This would streamline manufacturing of highly customized products.
Artificial Intelligence In Supplier Management And Customer Service
Organizations are also increasingly leveraging AI for supplier management and customer management. IPsoft’s AI platform, Amelia automates work knowledge and is able to speak to the customers in more than 20 languages. A global oil and gas company has trained Amelia to help provide prompt and more efficient ways of answering invoicing queries from its suppliers. A large US-based media services organization taught Amelia how to support first line agents in order to raise the bar for customer service.
Artificial Intelligence In Logistics & Warehousing
Logistics function will undergo a fundamental change as artificial intelligence gets deployed to handle domestic and international movement of goods. DHL has stated that its use of autonomous fork lifts is “reaching a level of maturity” in warehouse operations. The next step would be driver less autonomous vehicles undertaking goods delivery operations.
Artificial Intelligence In Procurement
AI is helping drive cost reduction and compliance agenda through procurement by generating real time visibility of the spend data. The spend data is automatically classified by AI software and is checked for compliance and any exceptions in real time. Singapore government is carrying out trials of using artificial intelligence to identify and prevent cases of procurement fraud.
The AI algorithm analyzes HR and finance data, procurement requests, tender approvals, workflows, non-financial data like government employee’s family details and vendor employee to identify potentially corrupt or negligent practices. AI will also take up basic procurement activities in the near future thereby helping improve the procurement productivity.
Artificial Intelligence in new product development
AI has totally overhauled the new product development process.by reducing the time to market for new products. Instead of developing physical prototypes and testing the same, innovators are now creating 3D digital models of the product. AI facilitates interaction of the product developers in the digital space by recognizing the gestures and position of hand. For example, the act of switching on a button of a digital prototype can be accomplished by a gesture.
AI In Demand Planning And Forecasting
Getting the demand planning right is a pain point for many companies. A leading health food company leveraged analytics with machine learning capabilities to analyze their demand variations and trends during promotions.
The outcome of this exercise was a reliable, detailed model highlighting expected results of the trade promotion for the sales and marketing department. Gains included a rapid 20 percent reduction in forecast error and a 30 percent reduction in lost sales.
AI in Smart Logistics
The impact of data-driven and autonomous supply chains provides an opportunity for previously unimaginable levels of optimization in manufacturing, logistics, warehousing and last mile delivery that could become a reality in less than half a decade despite high set-up costs deterring early adoption in logistics.
Changing consumer behavior and the desire for personalization are behind two other top trends Batch Size One and On-demand Delivery: Set to have a big impact on logistics, on-demand delivery will enable consumers to have their purchases delivered where and when they need them by using flexible courier services.
A study by MHI and Deloitte found more than half (51%) of supply chain and logistics professionals believe robotics and automation will provide a competitive advantage. That’s up from 39% last year. While only 35% of the respondents said they’ve already adopted robotics, 74% plan to do so within the next 10 years. And that’s likely in part to keep up with key players like Amazon, who have been leading the robotics charge for the past few years.
What is the mantra ?
These examples showcase that in today’s dynamic world, AI embedded supply chains offer a competitive advantage. AI armed with predictive analytics can analyze massive amounts of data generated by the supply chains and help organizations move to a more proactive form of supply chain management.
Thus, in this digital age where the mantra is “evolve or be disrupted”, companies are leveraging AI to reinvent themselves and scale their businesses quickly. AI is becoming a key enabler of the changes that businesses need to make and is helping them manage complexity of the constant digital change.
Related Posts
AIQRATIONS
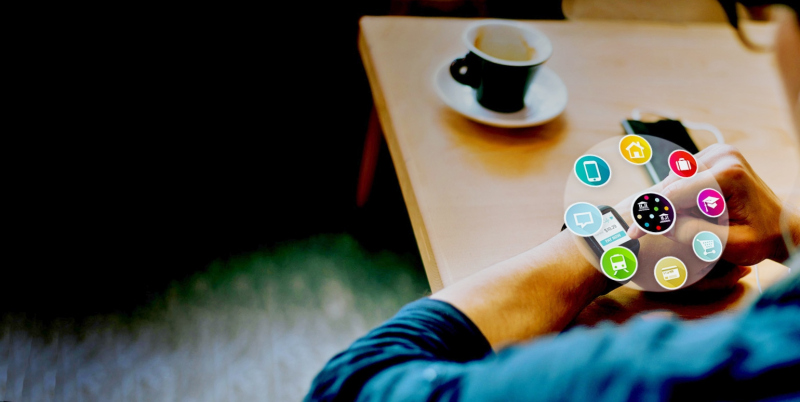
ACCELERATED DECISION MAKING AMPLIFIED BY REAL TIME ANALYTICS – A PERSPECTIVE
Add Your Heading Text Here
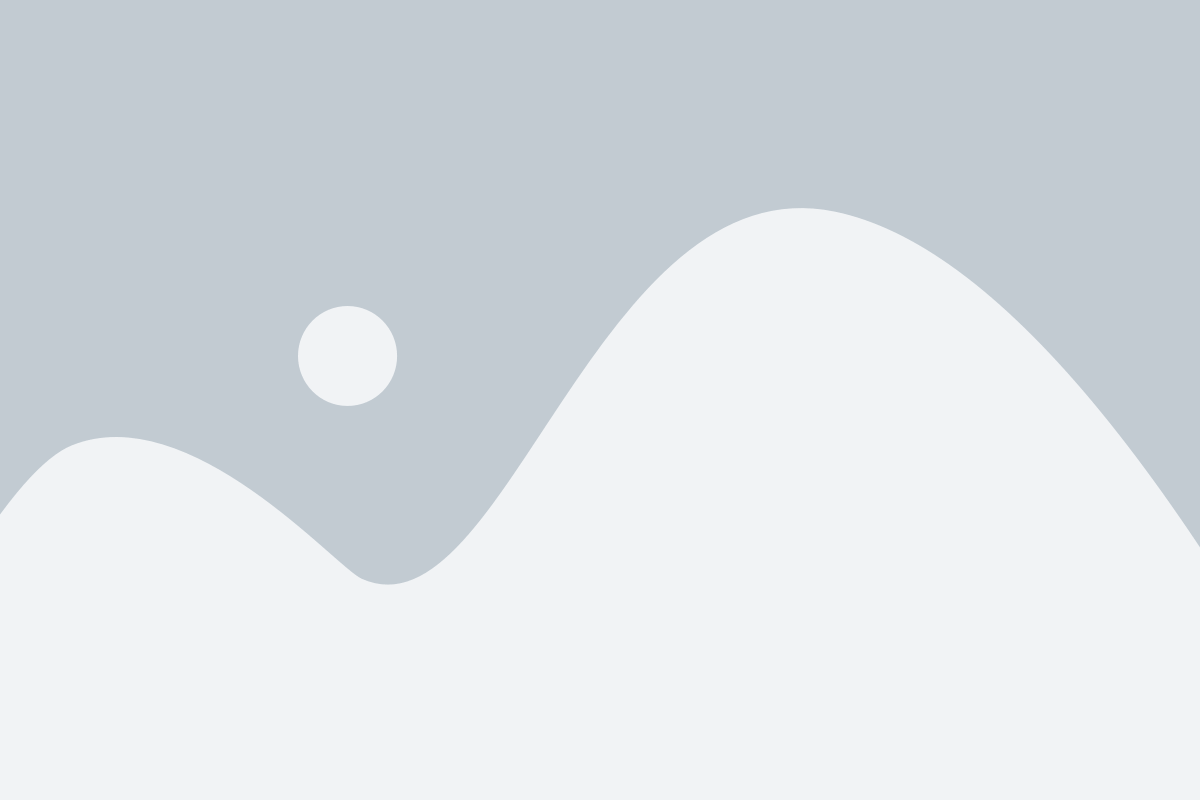
Companies are using more real-time analytics, because of the pressure to increase the speed and accuracy of business processes — particularly for digital business and the Internet of Things (IoT). Although data and analytics leaders intuitively understand the value of fast analytical insights, many are unsure how to achieve them.
Every large company makes thousands of real-time decisions each minute. For example, when a potential customer calls the contact center or visits the company’s website to gather product information, the company has a few seconds to figure out the best-next-action offer to propose to maximize the chance of making a sale. Or, when a customer presents a credit card to buy something or submits a withdrawal transaction request to an automated teller machine, a bank has one second or less to determine if the customer is who they say they are and whether they are likely to pay the bill when it is due. Of course, not all real-time decisions are tied to customers. Companies also make real-time decisions about internal operations, such as dynamically rerouting delivery trucks when a traffic jam forms; calling in a mechanic to replace parts in a machine when it starts to fail; or adjusting their manufacturing schedules when incoming materials fail to arrive on time.
Many decisions will be made in real time, regardless of whether real-time analytics are available, because the world is event-driven and the company has to respond immediately as events unfold. Improved real-time responses that are informed by fact-based, real-time analytics are optional, but clearly desirable.
Real-time analytics can be confusing, because different people may be thinking of different concepts when they use the term “real time.” Moreover, it isn’t always simple to determine where real-time analytics are appropriate, because the “right time” for analytics in a given business situation depends on many considerations; real-time is not always appropriate, or even possible. Finally, data and analytics leaders and their staff typically know less about real-time analytics than about traditional business intelligence and analytics.
Find the certain concept of “Real Time” Relevant to Your Business Problem
Real-time analytics is defined as “the discipline that applies logic and mathematics to data to provide insights for making better decisions quickly.” Real time means different things to different people.
When engineers say “real time” they mean that a system will always complete the task within a specified time frame.
Each component and subtask within the system is carefully designed to provide predictable performance, avoiding anything that could take longer to occur than is usually the case. Real-time systems prevent random delays, such as Java garbage collection, and may run on real-time operating systems that avoid nondeterministic behavior in internal functions such as scheduling and dispatching. There is an implied service-level agreement or guarantee. Strictly speaking, a real-time system could take hours or more to do its work, but in practice, most real-time systems act in seconds, milliseconds or even microseconds.
The concept of engineering real time is most relevant when dealing with machines and fully automated applications that require a precise sequence and timing of interactions among multiple components. Control systems for airplanes, power plants, self-driving cars and other machines often use real-time design. Time-critical software applications, such as high-frequency trading (HFT), also leverage engineering real-time concepts although they may not be entirely real time.
Use Different Technology and Design Patterns for Real-Time Computation Versus Real-Time Solutions
Some people use the term real-time analytics to describe fast computation on historical data from yesterday or last year. It’s obviously better to get the answer to a query, or run a model, in a few seconds or minutes (business real time) rather than waiting for a multihour batch run. Real-time computation on small datasets is executed in-memory by Excel and other conventional tools. Real-time computation on large datasets is enabled by a variety of design patterns and technologies, such as:
- Preloading the data into an in-memory database or in-memory analytics tool with large amounts of memory
- Processing in parallel on multiple cores and chips
- Using faster chips or graphics processing units (GPUs)
- Applying efficient algorithms (for example, minimizing context switches)
- Leveraging innovative data architectures (for example, hashing and other kinds of encoding)
Most of these can be hidden within modern analytics products so that the user does not have to be aware of exactly how they are being used.
Real-time computation on historical data is not sufficient for end-to-end real-time solutions that enable immediate action on emerging situations. Analytics for real-time solutions requires two additional things:
- Data must be real time (current)
- Analytic logic must be predefined
If conditions are changing from minute to minute, a business needs to have situation awareness of what is happening right now. The decision must reflect the latest sensor readings, business transactions, web logs, external market data, social computing postings and other current information from people and things.
Real-time solutions use design patterns that enable them to access the input data quickly so that data movement does not become the weak link in the chain. There is no time to read large amounts of data one row or message at a time across a wide-area network. Analytics are run as close as possible to where the data is generated. For example, IoT applications run most real-time solutions on or near the edge, close to the devices. Also, HFT systems are co-located with the stock exchanges to minimize the distance that data has to travel. In some real-time solutions, including HFT systems, special high-speed networks are used to convey streaming data into the system.
Match the Speed of Analytics to the Speed of the Business Decision
Decisions have a range of natural timing, so “right time” is not always real time. Business analysts and solution architects should work with managers and other decision makers to determine how fast to make each decision. The two primary considerations are:
- How quickly will the value of the decision degrade?
- Decisions should be executed in real time if a customer is waiting on the phone for an answer; resources would be wasted if they sit idle; fraud would succeed; or physical processes would fail if the decision takes more than a few milliseconds or minutes. On the other hand, a decision on corporate strategy may be nearly as valuable in a month as it would be today, because its implementation will take place over months and years so starting a bit earlier may not matter much.
- How much better will a decision be if more time is spent?
- Simple, well-understood decisions on known topics, and for which data is readily available, can be made quickly without sacrificing much quality.
Lastly, Automate Decisions if Algorithms Can Represent the Entire Decision Logic
Algorithms offers the “last mile” of the decision. However, automating algorithms requires a well described process to code against. According to Gartner, “Decision automation is possible only when the algorithms associated with the applicable business policies can be fully defined.”
Final Word
Performing some analytics in real time is a goal in many analytics and business intelligence modernization programs. To operate in real time, data and analytics leaders must leverage predefined analytical models, rather than ad hoc models, and use current input data rather than just historical data.
Related Posts
AIQRATIONS
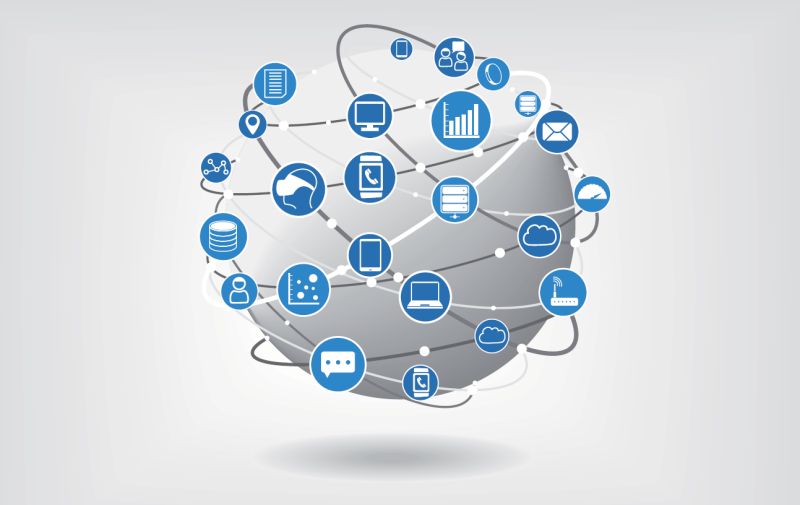
THE BEST PRACTICES FOR INTERNET OF THINGS ANALYTICS
Add Your Heading Text Here
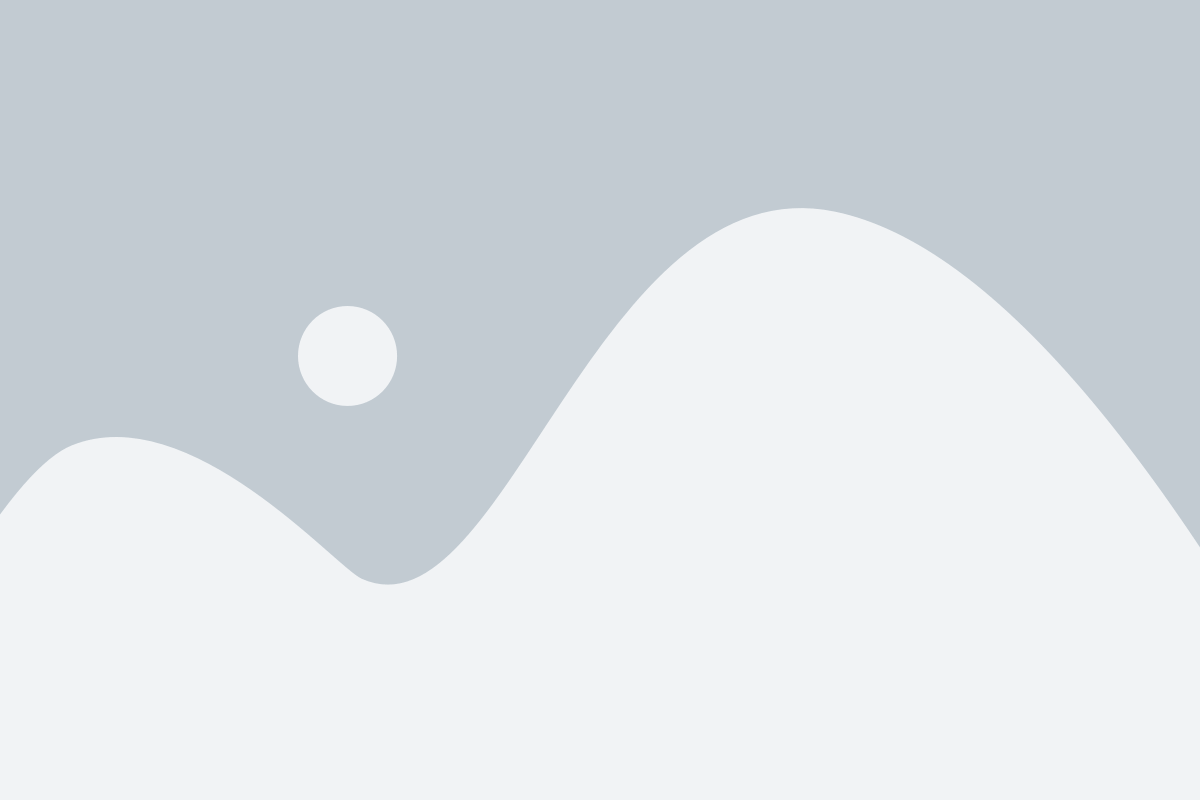
In most ways, Internet of Things analytics are like any other analytics. However, the need to distribute some IoT analytics to edge sites, and to use some technologies not commonly employed elsewhere, requires business intelligence and analytics leaders to adopt new best practices and software.
There are certain prominent challenges that Analytics Vendors are facing in venturing into building a capability. IoT analytics use most of the same algorithms and tools as other kinds of advanced analytics. However, a few techniques occur much more often in IoT analytics, and many analytics professionals have limited or no expertise in these. Analytics leaders are struggling to understand where to start with Internet of Things (IoT) analytics. They are not even sure what technologies are needed.
Also, the advent of IoT also leads to collection of raw data in a massive scale. IoT analytics that run in the cloud or in corporate data centers are the most similar to other analytics practices. Where major differences appear is at the “edge” — in factories, connected vehicles, connected homes and other distributed sites. The staple inputs for IoT analytics are streams of sensor data from machines, medical devices, environmental sensors and other physical entities. Processing this data in an efficient and timely manner sometimes requires event stream processing platforms, time series database management systems and specialized analytical algorithms. It also requires attention to security, communication, data storage, application integration, governance and other considerations beyond analytics. Hence it is imperative to evolve into edge analytics and distribute the data processing load all across.
Hence, some IoT analytics applications have to be distributed to “edge” sites, which makes them harder to deploy, manage and maintain. Many analytics and Data Science practitioners lack expertise in the streaming analytics, time series data management and other technologies used in IoT analytics.
Some visions of the IoT describe a simplistic scenario in which devices and gateways at the edge send all sensor data to the cloud, where the analytic processing is executed, and there are further indirect connections to traditional back-end enterprise applications. However, this describes only some IoT scenarios. In many others, analytical applications in servers, gateways, smart routers and devices process the sensor data near where it is generated — in factories, power plants, oil platforms, airplanes, ships, homes and so on. In these cases, only subsets of conditioned sensor data, or intermediate results (such as complex events) calculated from sensor data, are uploaded to the cloud or corporate data centers for processing by centralized analytics and other applications.
The design and development of IoT analytics — the model building — should generally be done in the cloud or in corporate data centers. However, analytics leaders need to distribute runtime analytics that serve local needs to edge sites. For certain IoT analytical applications, they will need to acquire, and learn how to use, new software tools that provide features not previously required by their analytics programs. These scenarios consequently give us the following best practices to be kept in mind:
Develop Most Analytical Models in the Cloud or at a Centralized Corporate Site
When analytics are applied to operational decision making, as in most IoT applications, they are usually implemented in a two-stage process – In the first stage, data scientists study the business problem and evaluate historical data to build analytical models, prepare data discovery applications or specify report templates. The work is interactive and iterative.
A second stage occurs after models are deployed into operational parts of the business. New data from sensors, business applications or other sources is fed into the models on a recurring basis. If it is a reporting application, a new report is generated, perhaps every night or every week (or every hour, month or quarter). If it is a data discovery application, the new data is made available to decision makers, along with formatted displays and predefined key performance indicators and measures. If it is a predictive or prescriptive analytic application, new data is run through a scoring service or other model to generate information for decision making.
The first stage is almost always implemented centrally, because Model building typically requires data from multiple locations for training and testing purposes. It is easier, and usually less expensive, to consolidate and store all this data centrally. Also, It is less expensive to provision advanced analytics and BI platforms in the cloud or at one or two central corporate sites than to license them for multiple distributed locations.
The second stage — calculating information for operational decision making — may run either at the edge or centrally in the cloud or a corporate data center. Analytics are run centrally if they support strategic, tactical or operational activities that will be carried out at corporate headquarters, at another edge location, or at a business partner’s or customer’s site.
Distribute the Runtime Portion of Locally Focused IoT Analytics to Edge Sites
Some IoT analytics applications need to be distributed, so that processing can take place in devices, control systems, servers or smart routers at the sites where sensor data is generated. This makes sure the edge location stays in operation even when the corporate cloud service is down. Also, wide-area communication is generally too slow for analytics that support time-sensitive industrial control systems.
Thirdly, transmitting all sensor data to a corporate or cloud data center may be impractical or impossible if the volume of data is high or if reliable, high-bandwidth networks are unavailable. It is more practical to filter, condition and do analytic processing partly or entirely at the site where the data is generated.
Train Analytics Staff and Acquire Software Tools to Address Gaps in IoT-Related Analytics Capabilities
Most IoT analytical applications use the same advanced analytics platforms, data discovery tools as other kinds of business application. The principles and algorithms are largely similar. Graphical dashboards, tabular reports, data discovery, regression, neural networks, optimization algorithms and many other techniques found in marketing, finance, customer relationship management and advanced analytics applications also provide most aspects of IoT analytics.
However, a few aspects of analytics occur much more often in the IoT than elsewhere, and many analytics professionals have limited or no expertise in these. For example, some IoT applications use event stream processing platforms to process sensor data in near real time. Event streams are time series data, so they are stored most efficiently in databases (typically column stores) that are designed especially for this purpose, in contrast to the relational databases that dominate traditional analytics. Some IoT analytics are also used to support decision automation scenarios in which an IoT application generates control signals that trigger actuators in physical devices — a concept outside the realm of traditional analytics.
In many cases, companies will need to acquire new software tools to handle these requirements. Business analytics teams need to monitor and manage their edge analytics to ensure they are running properly and determine when analytic models should be tuned or replaced.
Increased Growth, if not Competitive Advantage
The huge volume and velocity of data in IoT will undoubtedly put new levels of strain on networks. The increasing number of real-time IoT apps will create performance and latency issues. It is important to reduce the end-to-end latency among machine-to-machine interactions to single-digit milliseconds. Following the best practices of implementing IoT analytics will ensure judo strategy of increased effeciency output at reduced economy. It may not be suffecient to define a competitive strategy, but as more and more players adopt IoT as a mainstream, the race would be to scale and grow as quickly as possible.
Related Posts
AIQRATIONS
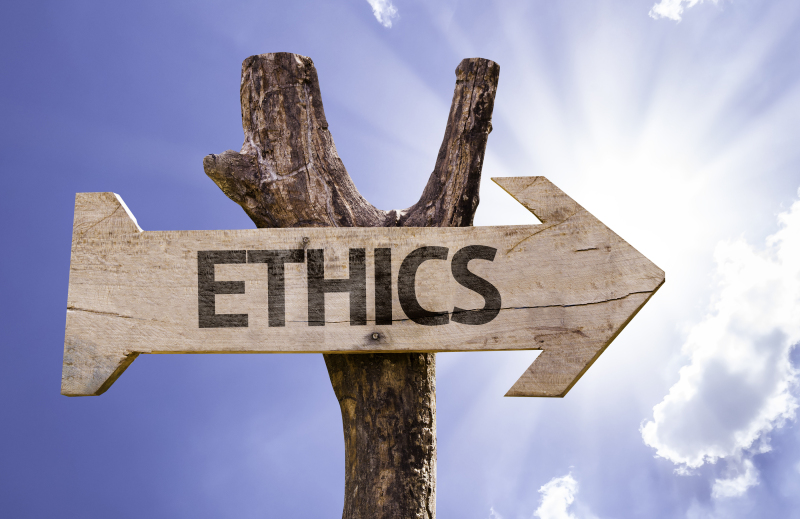
Ethics and Ethos in Analytics – Why is it Imperative for Enterprises to Keep Winning the Trust from Customers?
Add Your Heading Text Here
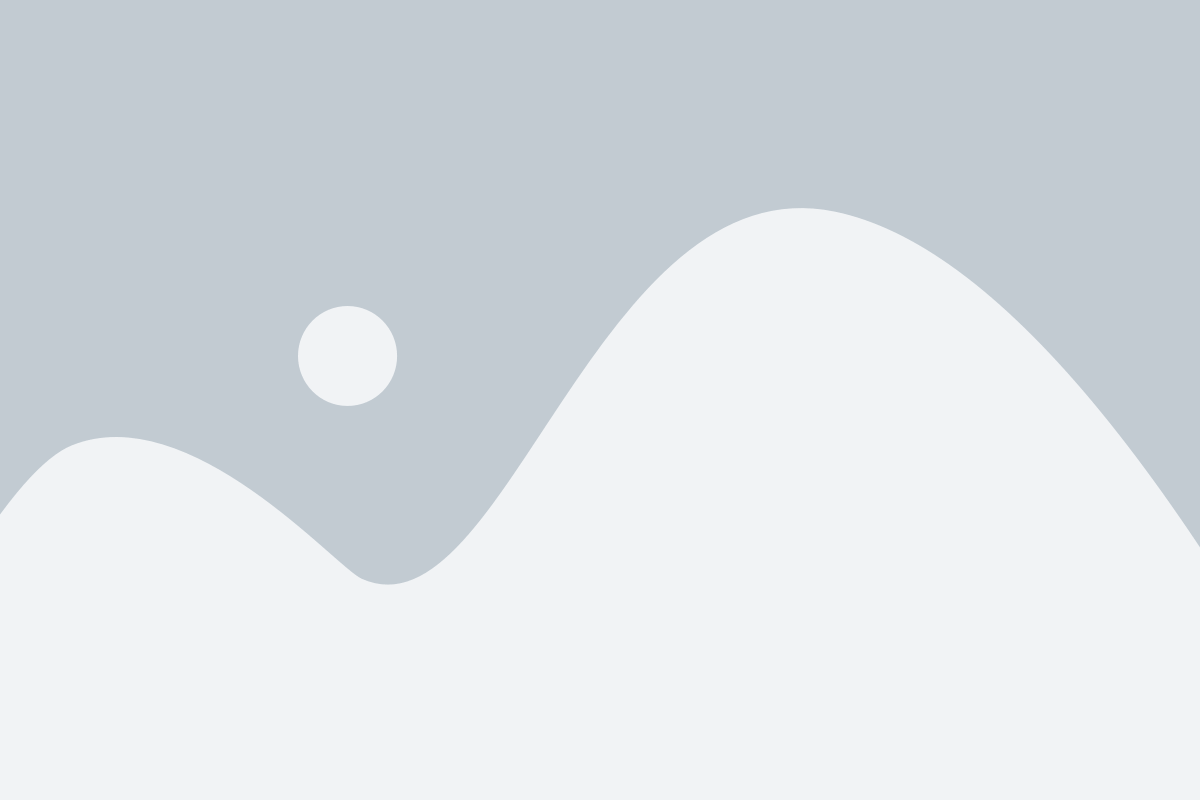
Data Sciences and analytics technology can reap huge benefits to both individuals and organizations – bringing personalized service, detection of fraud and abuse, efficient use of resources and prevention of failure or accident. So why are there questions being raised about the ethics of analytics, and its superset, Data Sciences?
Ethical Business Processes in Analytics Industry
At its core, an organization is “just people” and so are its customers and stakeholders. It will be individuals who choose what to organization does or does not do and individuals who will judge its appropriateness. As an individual, our perspective is formed from our experience and the opinions of those we respect. Not surprisingly, different people will have different opinions on what is appropriate use of Data Sciences and analytics technology particularly – so who decides which is “right”? Customers and stakeholders may have different opinions on to the organization about what is ethical.
This suggests that organizations should be thoughtful in their use of this Analytics; consulting widely and forming policies that record the decisions and conclusions they have come to. They will consider the wider implications of their activities including:
Context – For what purpose was the data originally surrendered? For what purpose is the data now being used? How far removed from the original context is its new use? Is this appropriate?
Consent & Choice – What are the choices given to an affected party? Do they know they are making a choice? Do they really understand what they are agreeing to? Do they really have an opportunity to decline? What alternatives are offered?
Reasonable – Is the depth and breadth of the data used and the relationships derived reasonable for the application it is used for?
Substantiated – Are the sources of data used appropriate, authoritative, complete and timely for the application?
Owned – Who owns the resulting insight? What are their responsibilities towards it in terms of its protection and the obligation to act?
Fair – How equitable are the results of the application to all parties? Is everyone properly compensated? Considered – What are the consequences of the data collection and analysis?
Access – What access to data is given to the data subject?
Accountable – How are mistakes and unintended consequences detected and repaired? Can the interested parties check the results that affect them?
Together these facets are called the ethical awareness framework. This framework was developed by the UK and Ireland Technical Consultancy Group (TCG) to help people to develop ethical policies for their use of analytics and Data Sciences. Examples of good and bad practices are emerging in the industry and in time they will guide regulation and legislation. The choices we make, as practitioners will ultimately determine the level of legislation imposed around the technology and our subsequent freedom to pioneer in this exciting emerging technical area.
Designing Digital Business for Transparency and Trust
With the explosion of digital technologies, companies are sweeping up vast quantities of data about consumers’ activities, both online and off. Feeding this trend are new smart, connected products—from fitness trackers to home systems—that gather and transmit detailed information.
Though some companies are open about their data practices, most prefer to keep consumers in the dark, choose control over sharing, and ask for forgiveness rather than permission. It’s also not unusual for companies to quietly collect personal data they have no immediate use for, reasoning that it might be valuable someday.
In a future in which customer data will be a growing source of competitive advantage, gaining consumers’ confidence will be key. Companies that are transparent about the information they gather, give customers control of their personal data, and offer fair value in return for it will be trusted and will earn ongoing and even expanded access. Those that conceal how they use personal data and fail to provide value for it stand to lose customers’ goodwill—and their business.
At the same time, consumers appreciate that data sharing can lead to products and services that make their lives easier and more entertaining, educate them, and save them money. Neither companies nor their customers want to turn back the clock on these technologies—and indeed the development and adoption of products that leverage personal data continue to soar. The consultancy Gartner estimates that nearly 5 billion connected “things” will be in use in 2015—up 30% from 2014—and that the number will quintuple by 2020.
Resolving this tension will require companies and policy makers to move the data privacy discussion beyond advertising use and the simplistic notion that aggressive data collection is bad. We believe the answer is more nuanced guidance—specifically, guidelines that align the interests of companies and their customers, and ensure that both parties benefit from personal data collection
Understanding the “Privacy Sensitiveness” of Customer Data
Keeping track of the “privacy sensitiveness” of customer data is also crucial as data and its privacy are not perfectly black and white. Some forms of data tend to be more crucial for customers to protect and maintained private. To see how much consumers valued their data , a conjoint analysis was performed to determine what amount survey participants would be willing to pay to protect different types of information. Though the value assigned varied widely among individuals, we are able to determine, in effect, a median, by country, for each data type.
The responses revealed significant differences from country to country and from one type of data to another. Germans, for instance, place the most value on their personal data, and Chinese and Indians the least, with British and American respondents falling in the middle. Government identification, health, and credit card information tended to be the most highly valued across countries, and location and demographic information among the least.
This spectrum doesn’t represents a “maturity model,” in which attitudes in a country predictably shift in a given direction over time (say, from less privacy conscious to more). Rather, our findings reflect fundamental dissimilarities among cultures. The cultures of India and China, for example, are considered more hierarchical and collectivist, while Germany, the United States, and the United Kingdom are more individualistic, which may account for their citizens’ stronger feelings about personal information.
Adopting Data Aggregation Paradigm for Protecting Privacy
If companies want to protect their users and data they need to be sure to only collect what’s truly necessary. An abundance of data doesn’t necessarily mean that there is an abundance of useable data. Keeping data collection concise and deliberate is key. Relevant data must be held in high regard in order to protect privacy.
It’s also important to keep data aggregated in order to protect privacy and instill transparency. Algorithms are currently being used for everything from machine thinking and autonomous cars, to data science and predictive analytics. The algorithms used for data collection allow companies to see very specific patterns and behavior in consumers all while keeping their identities safe.
One way companies can harness this power while heeding privacy worries is to aggregate their data…if the data shows 50 people following a particular shopping pattern, stop there and act on that data rather than mining further and potentially exposing individual behavior.
Things are getting very interesting…Google, Facebook, Amazon, and Microsoft take the most private information and also have the most responsibility. Because they understand data so well, companies like Google typically have the strongest parameters in place for analyzing and protecting the data they collect.
Finally, Analyze the Analysts
Analytics will increasingly play a significant role in the integrated and global industries today, where individual decisions of analytics professionals may impact the decision making at the highest levels unimagined years ago. There’s a substantial risk at hand in case of a wrong, misjudged model / analysis / statistics that can jeopardize the proper functioning of an organization.
Instruction, rules and supervisions are essential but that alone cannot prevent lapses. Given all this, it is imperative that Ethics should be deeply ingrained in the analytics curriculum today. I believe, that some of the tenets of this code of ethics and standards in analytics and data science should be:
- These ethical benchmarks should be regardless of job title, cultural differences, or local laws.
- Places integrity of analytics profession above own interests
- Maintains governance & standards mechanism that data scientists adhere to
- Maintain and develop professional competence
- Top managers create a strong culture of analytics ethics at their firms, which must filter throughout their entire analytics organization
Related Posts
AIQRATIONS
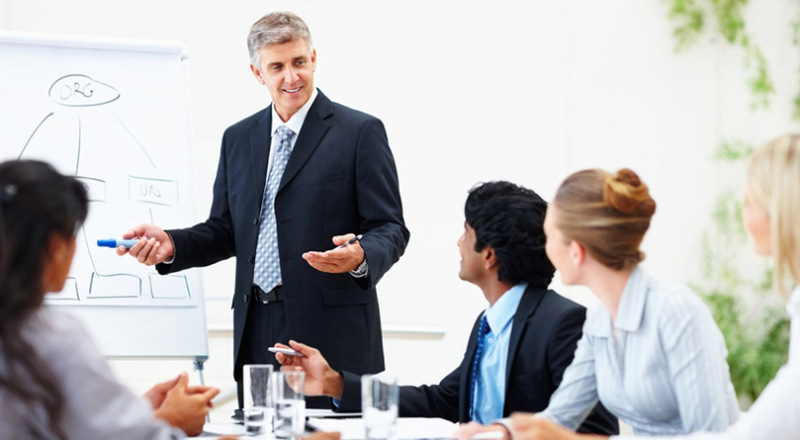
Analytics Business Leaders are a Scant Community
Add Your Heading Text Here
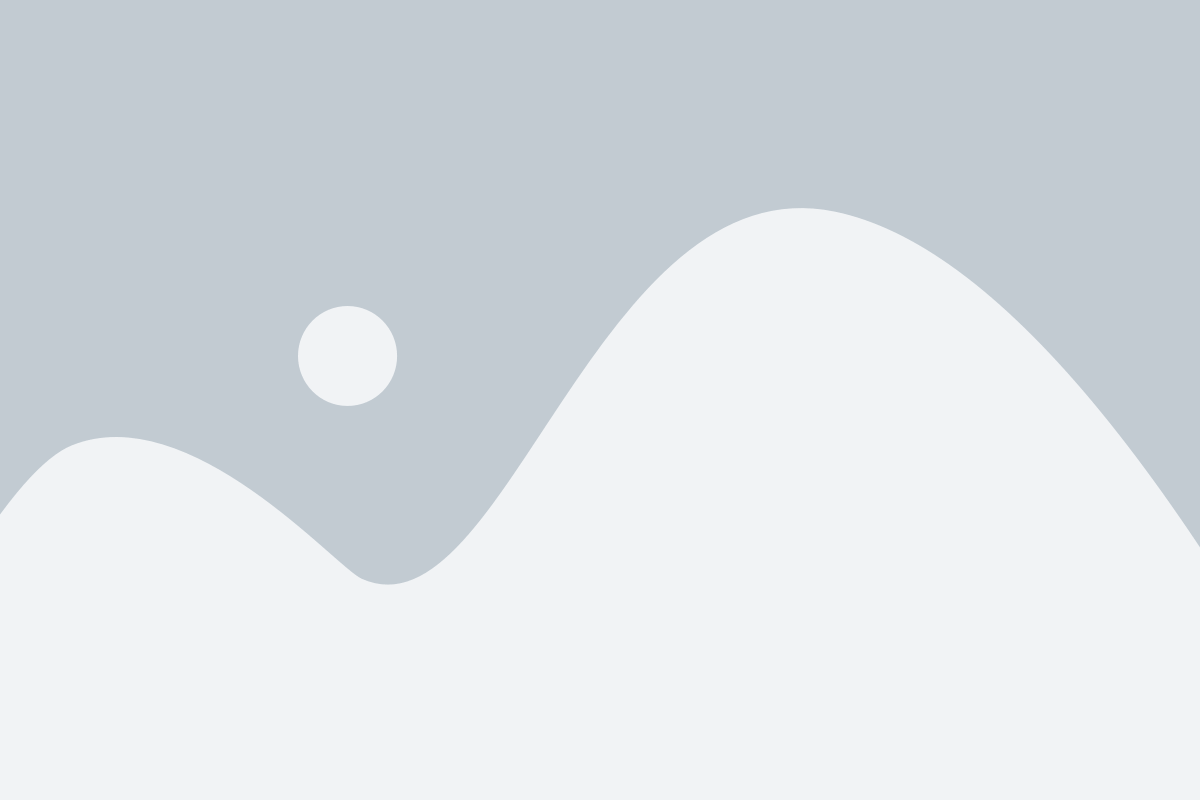
A considerable amount of current conversation in the area of data science and analytics focuses on the virtues of solving all the challenges that organizations face when using this new paradigm in the business world. There is also a lot of discussion around the technology-related issues that impact achieving data science and analytics goals.
What hasn’t gotten the attention that it merits, however, is the role of business leadership and how thought leaders need to raise the stakes to become not only well versed in analytics, but to build data science and analytics literacy throughout their organizations. They need a heightened awareness of analytics if they are going to effectively drive analytics strategies and outcomes for their organizations and become true leaders in this area by all relevant measures.
The following three significant findings from align with this point of view:
- Create a culture for making fact-based decisions.
- Establish a common data science and analytics vision—and strategy—to focus everyone on the outcomes.
- Instill analytics expertise across the entire organization, from the top down.
Senior executives and business managers should aspire to create the core competencies and to develop analytical insights that enable them to become data science and analytics leaders within their industries. Education, mentorship, and consultation with outside advisors should be implemented to gain the knowledge necessary to attain a leadership role. When selecting a consultancy, business leaders should choose one that can advise, mentor, and support based on specific needs and levels of maturity, as opposed to those that may take a force-fit approach that essentially attempts to force a round peg methodology into a square pegenvironment. And a good grasp of numbers in respect to numerical literacy is also important in this age of fact-based decision making based on insights derived from large volumes of data.
Numerical literacy means acceptable levels of working knowledge and experience in decision science in which analytical techniques such as statistical and descriptive analysis, forecasting, and performance management can be applied. In addition, moving from a gut-based decision model to a fact-based one requires both cultural change as well as the tools and know-how required to create and manage the facts themselves. Finance teams can typically be the source of such competencies, and they can be used as a center for fostering and developing these competencies across the enterprise. It is ultimately at the mercy of top level management on how they are going to leverage their business acumen to instil a cultural change to foster data thinking.
Bringing In Data Mentors At The Very Top
Today’s executives and managers are trained primarily in operations, finance, marketing, and sales, along with a bit of strategy thrown in for good measure. While a significant number of senior executives in the US have advanced degrees in their field of expertise, few have been formally trained in information management, analytics, or decision science. Yet, virtually none have been schooled in decision science, information theory, analytics, or risk management. Lack of training in these areas creates a dilemma for those organizations that want to focus on data science and analytics but do not have experienced leaders who can lead from a position of domain expertise. But that wouldn’t mean stacking up data workers at the TLM (top level management). Leadership drive , domain insight and People Skills will still be the most coveted virtues at the very top. But the lack of ground level data-sifting skills need not be only plugged at the lower levels. To achieve these competencies without formal education or hands-on experience requires consultation with outside data mentors and advisors who can work hand in hand with the entire senior executive team. These advisors help ground the team in both the science and the pragmatics required to achieve successful data science and analytics outcomes that can be applied pervasively across the organization. This approach—some call it the “charm school” approach—can be characterized by a close collaboration among all parties involved. It can rapidly accelerate the process of nondisruptively developing the senior executive team’s data science and analytics expertise and competency to maximize strategic outcomes.
Data science and analytics success should be driven by the business, and more importantly from the ranks of its senior executives and managers—not from the bottom up or from the IT function. The inherent accountability for all strategic initiatives is at the very top of the organization and cascades down and across to business managers at various levels who then have responsibilities for its execution within their area of control. Organizations today remain hierarchical in both structure and cultural behavior. To change either of these structures requires engaged and competent senior executive teams that are committed to the outcome and can influence and align behaviors to support it.
Strategic Thinking in Data Monetization
A number of organizations have come to this realization already. They’re now engaging with management consultancies and analytics boutiques to address their shortcomings and accelerate results from their data science and analytics strategies and successfully monetize them. Alongside these mentoring activities, organizational leadership is strongly advised to consider organizational structures and change readiness as complementary endeavors. They can help illuminate the revisions to structure and Organizational Change Management (OCM) activities required to bring the entire organization to a level that they could start assessing the monetizing estimates of data thinking. These accompanying measures can also bring cohesion to the entire data science and analytics strategy and the pursuit of its outcomes.
Firms that are looking to monetize Data and their Data Science strategies, must look beyond the data and into the economic questions that the data can answer. Often the data can help answer questions about the value, use, risk, or future value or risk of a specific asset. Or the data can say something about an overall market and how asset classes perform and how customers behave generally. Such insights are understood to have great economic value to asset owners and market participants. However, not all data will offer these features or value. The temperature readings from inside our refrigerators are unlikely to alter markets. However, the temperature readings of our furnaces and air conditioners could, in aggregate, drive new energy conservation and policy decisions.
Transforming data into economic insights will be the focus of top level executives who will monetize data. This transformation will require the creation of data products. It may be that such data products can be sold or traded to clients. It can also be that giving away data products will drive other related monetization strategies. Hence creating the data product will not only require technical expertise with manipulating data but a clear vision on how data can be leveraged to answer economical questions and how the future market of the various domains will pan out.
Related Posts
AIQRATIONS
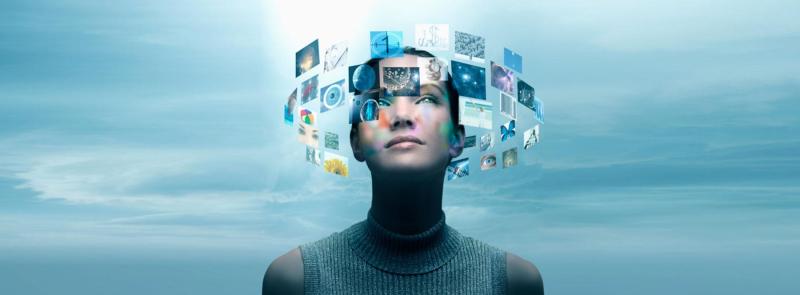
2017 Digital Trends
Add Your Heading Text Here
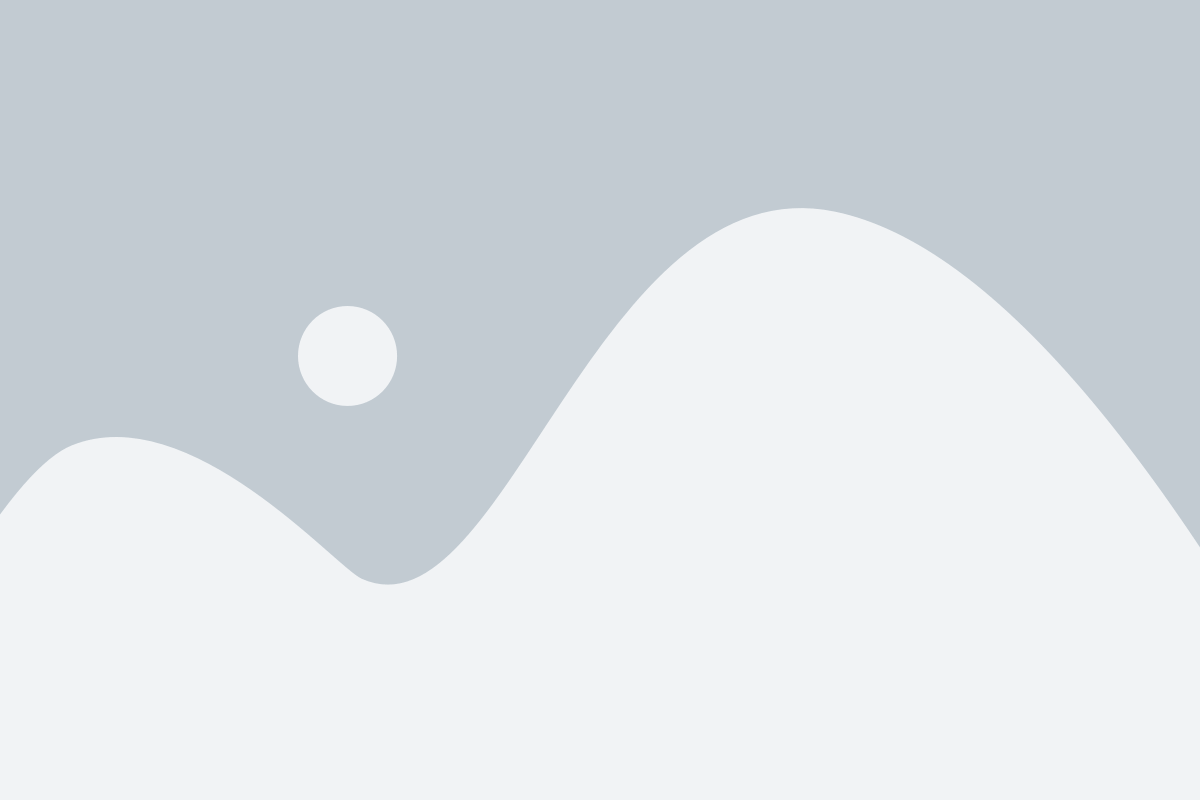
Digital transformation reshapes every aspect of a business. As digital technology continues to evolve, I believe that successful digital transformation will require careful collaboration, thoughtful planning, and the inclusion of every department.
During recent years, we’ve seen shifts in how traditional leadership roles operate, as silos break down and the scopes of various roles widen and change. Digital transformation has morphed from a trend to a central component of modern business strategy. Following are the enlisted major trends that will capture the gist of what is to come in 2017.
DIGITAL PLATFORM VIEW OF BUSINESS
A platform provides the business with a foundation where resources can come together — sometimes quickly and temporarily, sometimes in a relatively fixed way — to create value. The value comes largely from connecting the resources, and the network effects between them. As digitalization moves from an innovative trend to a core competency, enterprises will understand and exploit platform effects throughout all aspects of their businesses.
- The deepening of digital means that lines are becoming increasingly blurred, and boundaries semi porous — both inside and outside the enterprise — as multiple networks of stakeholders bring value to each other by exploiting and exploring platform dynamics
- CIOs are clearly being given the opportunity to lead a digital transformation that exploits platform effects majorly in managing delivery, talent and executing leadership
Detailed Analysis can be found here:
https://sameerdhanrajani.wordpress.com/2016/12/18/sameer-dhanrajani-key-win-themes-to-master-in-digital-business/
THE ADVENT OF IMMERSIVE CONTENT: AUGMENTED REALITY AND VIRTUAL REALITY
The booming success of the Pokémon GO AR app is a wakeup call to any business that hasn’t evaluated the potential of AR and VR. These technologies were once limited to the gaming realm, but they’re now easier to implement than ever before. The mainstream shift toward AR and VR provides new ways to connect with customers and offer unique, memorable interactions.
- The AR and VR resurgence will open up the gates for workplace gamification in a big way into a core business strategy
- 2017 is also going to mark a turning point in the way audiences interact with and consume video content through the releases of the HTC Vive, Oculus Rift, PSVR etc.
- Significant improvements in immersive devices as well as software is anticipated
Detailed Analysis can be found here:
https://sameerdhanrajani.wordpress.com/2016/05/27/sameer-dhanrajani-retail-industry-redefined-through-data-sciences/
SMART MACHINES AND ARTIFICIAL INTELLIGENCE ARE TAKING OFF IN A BIG WAY
Our relationships to technology continue to evolve. Soon machines will be able to learn and adapt to their environments. While advanced learning machines may replace low-skill jobs, AIs will be able to work collaboratively with human professionals to solve intensely complex problems.
- Data complexity is the top challenge standing in the way of digital transformation
- AI tools will evolve to read, review and analyze vast quantities of disparate data, providing insight into how customers feel about a company’s products or services and why they feel the way they do
- using AI to expedite knowledge-based activities to improve efficiency and performance will spread from reducing costs through automation, to transforming customer experience
Detailed Analysis can be found here:
https://sameerdhanrajani.wordpress.com/2016/11/18/sameer-dhanrajani-banking-evolution-using-ai/
GROWING IMPORTANCE OF THE USER EXPERIENCE
The customer experience (including employees) is the ultimate goal of any digital transformation. Customers are more cautious than ever; they’ll turn away from brands that don’t align with their values and needs. A top-notch user experience is a fantastic way to keep customers involved and engaged with your brand.
- Every touch point matters, and those leading the transformation will strive to constantly ask how they are removing friction and enhancing the experience for every customer regardless of where they are in the journey
- Understanding digital consumers’ biases, behaviors and expectations at each point along the customer journey will be at the heart of every successful digital transformation
Detailed Analysis can be found here:
https://sameerdhanrajani.wordpress.com/2015/07/24/sameer-dhanrajani-how-to-bring-about-a-customer-experience-focused-digital-transformation/
https://sameerdhanrajani.wordpress.com/2016/12/18/sameer-dhanrajani-key-win-themes-to-master-in-digital-business/
BLOCKCHAIN’S DISRUPTIVE GROWTH
What Uber did for on-demand auto transformation, Blockchain will to do for financial transactions. And with $1.4 billion in venture-capital money in the past three years, 24 countries investing in Blockchain technology for government services, 90-plus central banks engaged in related discussions, and 10 percent of global GDP to be traded via Blockchain technology by 2025-2027, it is important that marketers understand the potential implications for their business.
- Blockchain technology will majorly be a part of the next great flattening and removal of middle-layer institutions
- The semi-public nature of some types of Blockchain paves the way for an enhanced level of security and privacy for sensitive data – a new kind of database where the information ‘header’ is public but the data inside is ‘private’
- Data analytics using Blockchain, distributed ledger transactions and smart contracts will become critical in future, creating new challenges and opportunities in the world of data science
Detailed Analysis can be found here:
https://sameerdhanrajani.wordpress.com/2016/06/21/sameer-dhanrajani-data-sciences-fintech-companies-for-competitive-disruption-advantage/
DIGITAL TRANSFORMATION DRIVEN BY THE INTERNET OF THINGS (IOT).
Speaking of how invaluable big data is to marketers, the IoT offers immeasurable insight into customer’s mind. Businesses and customers alike will continue to benefit from the IoT. With an estimated 50 billion IoT Sensors by 2020 and more than 200 billion “Things” on the Internet by 2030, it is no question that IoT will be not only transformative, but disruptive to business models.
- IoT will change how daily life operates by helping create more efficient cities and leaner enterprises
- The staple tech for autonomous systems would be the Internet of Things (IoT) which would be the infrastructure, as well as the customers, since they work, interact, negotiate and decide with zero human intervention
- Real-time streaming analytics will collection, integration, analysis, and visualization of IoT data in real-time without disrupting the working of existing sources, storage, and enterprise systems
Detailed Analysis can be found here:
https://sameerdhanrajani.wordpress.com/2015/09/25/sameer-dhanrajani-real-time-streaming-analytics/
API ECONOMY
We live in an API economy, a set of business models and channels based on secure access of functionality and exchange of data. APIs will continue to make it easier to integrate and connect people, places, systems, data, things and algorithms, create new user experiences, share data and information, authenticate people and things, enable transactions and algorithms, leverage third-party algorithms, and create new product/services and business models.
- An industry vision seeks using APIs to turn a business into a platform involving digital business models
- As the Internet of Things (IoT) gets smarter, things using an application programming interface (API) to communicate, transact and even negotiate with one another will become the norm
Detailed Analysis can be found here:
https://sameerdhanrajani.wordpress.com/2016/02/12/mr-algorithms-the-new-member-in-the-board-room-to-discuss-algorithm-economy/
http://www.gartner.com/smarterwithgartner/welcome-to-the-api-economy/
Related Posts
AIQRATIONS
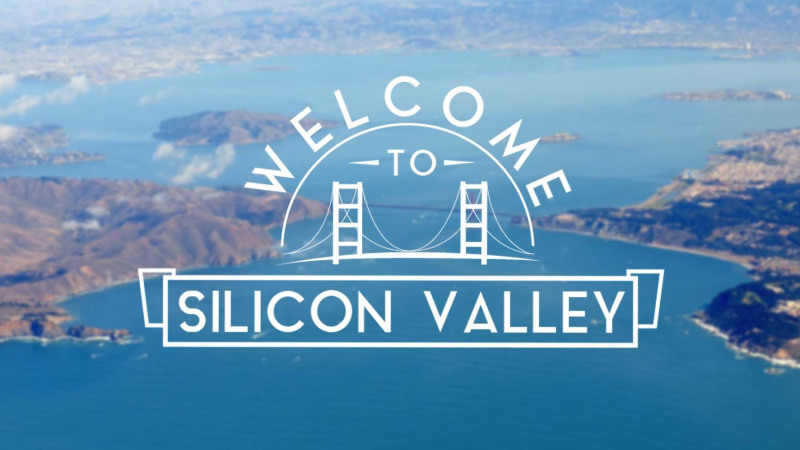
The Rush for Artificial Intelligence in Silicon Valley…Is This Here to Stay?
Add Your Heading Text Here
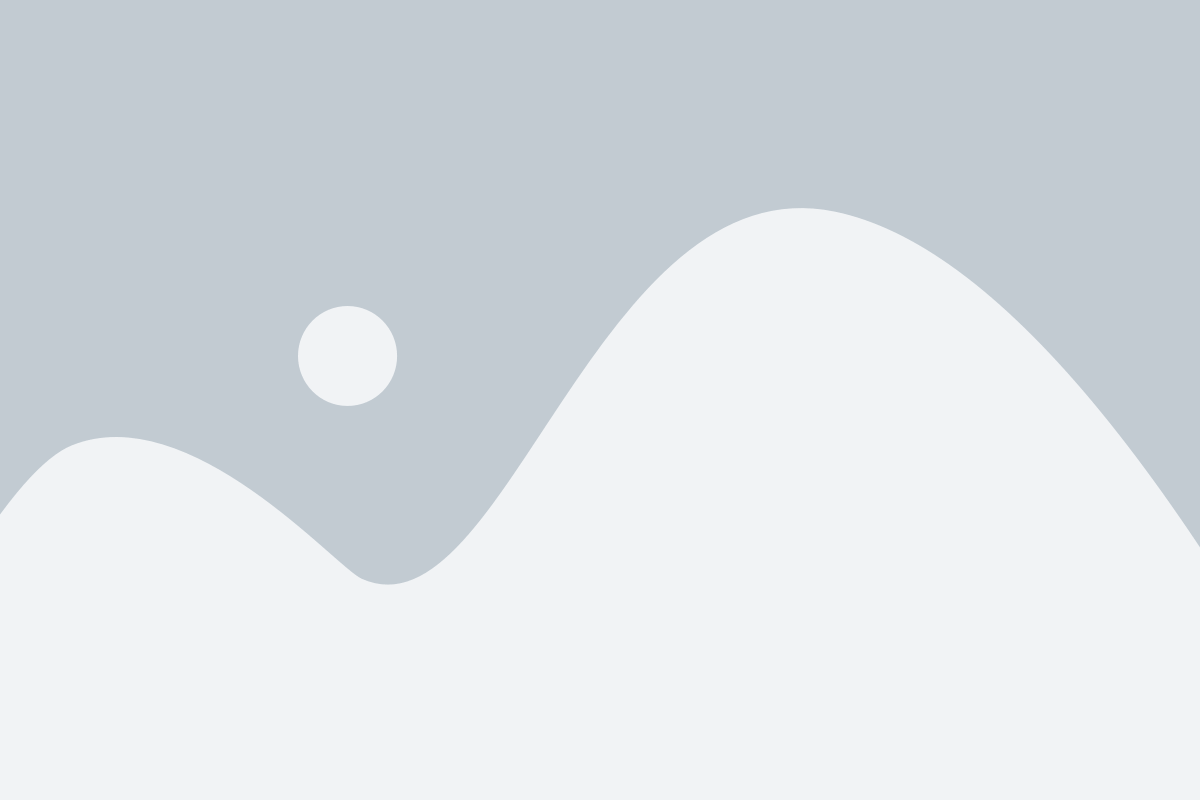
For more than a decade, Silicon Valley’s technology investors and entrepreneurs obsessed over social media and mobile apps that helped people do things like find new friends, fetch a ride home or crowdsource a review of a product or a movie.
Robots after the “Like” Button
Now Silicon Valley has found its next shiny new thing. And it does not have a “Like” button.
The new era in Silicon Valley centers on artificial intelligence and robots, a transformation that many believe will have a payoff on the scale of the personal computing industry or the commercial internet, two previous generations that spread computing globally. Computers have begun to speak, listen and see, as well as sprout legs, wings and wheels to move unfettered in the world.
The shift was evident in a Lowe’s home improvement store here this month, when a prototype inventory checker developed by Bossa Nova Robotics silently glided through the aisles using computer vision to automatically perform a task that humans have done manually for centuries.
The robot, which was skilled enough to autonomously move out of the way of shoppers and avoid unexpected obstacles in the aisles, alerted people to its presence with soft birdsong chirps. Gliding down the middle of an aisle at a leisurely pace, it can recognize bar codes on shelves, and it uses a laser to detect which items are out of stock.
Silicon Valley’s financiers and entrepreneurs are digging into artificial intelligence with remarkable exuberance. The region now has at least 19 companies designing self-driving cars and trucks, up from a handful five years ago. There are also more than a half-dozen types of mobile robots, including robotic bellhops and aerial drones, being commercialized.
The Surge after the Static – The Social Way
There has been a slow trickle in investments in robotics all this while, and suddenly, there seem to be a dozen companies securing large investment rounds focusing on specific robotic niches. Funding in A.I. start-ups has increased more than fourfold to $681 million in 2015, from $145 million in 2011, according to the market research firm CB Insights. The firm estimates that new investments will reach $1.2 billion this year, up 76 percent from last year.
By contrast, funding for social media start-ups peaked in 2011 before plunging. That year, venture capital firms made 66 social media deals and pumped in $2.4 billion. So far this year, there have been just 10 social media investments, totaling $6.9 million, according to CB Insights. Last month, the professional social networking site LinkedIn was sold to Microsoft for $26.2 billion, underscoring that social media has become a mature market sector.
Even Silicon Valley’s biggest social media companies are now getting into artificial intelligence, as are other tech behemoths. Facebook is using A.I. to improve its products. Google will soon compete with Amazon’s Echo and Apple’s Siri, which are based on A.I., with a device that listens in the home, answers questions and places e-commerce orders. Satya Nadella, Microsoft’s chief executive, recently appeared at the Aspen Ideas Conference and called for a partnership between humans and artificial intelligence systems in which machines are designed to augment humans.
The auto industry has also set up camp in the valley to learn how to make cars that can do the driving for you. Both technology and car companies are making claims that increasingly powerful sensors and A.I. software will enable cars to drive themselves with the push of a button as soon as the end of this decade — despite recent Tesla crashes that have raised the question of how quickly human drivers will be completely replaced by the technology.
AI Outdoes the Silicon Valley Reset Trend
Silicon Valley’s new A.I. era underscores the region’s ability to opportunistically reinvent itself and quickly follow the latest tech trend. This is at the heart of the region’s culture that goes all the way back to the Gold Rush. The valley is built on the idea that there is always a way to start over and find a new beginning.
The change spurred a rush for talent in A.I. that has become intense. It is unusual that the number of people trying to get the students to drop out of the class halfway through because now they know a little bit of this stuff is crazy. The valley’s tendency toward reinvention dates back to the region’s initial emergence from the ashes of a deep aerospace industry recession as a consumer-electronics manufacturing center producing memory chips, video games and digital watches in the mid-1970s. A malaise in the personal computing market in the early 1990s was followed by the World Wide Web and the global expansion of the consumer internet.
A decade later, in 2007, just as innovation in mobile phones seemed to be on the verge of moving away from Silicon Valley to Europe and Asia, Apple introduced the first iPhone, resetting the mobile communications marketplace and ensuring that the valley would — for at least another generation — remain the world’s innovation center.
In the most recent shift, the A.I. idea emerged first in Canada in the work of cognitive scientists and computer scientists like Geoffrey Hinton, Yoshua Bengio and Yann LeCun during the previous decade. The three helped pioneer a new approach to deep learning, a machine learning method that is highly effective for pattern recognition challenges like vision and speech. Modeled on a general understanding of how the human brain works, it has helped technologists make rapid progress in a wide range of A.I. fields.
The Road Ahead
How far the A.I. boom will go is hotly debated. For some technologists, today’s technical advances are laying the groundwork for truly brilliant machines that will soon have human-level intelligence. Yet Silicon Valley has faced false starts with A.I. before. During the 1980s, an earlier generation of entrepreneurs also believed that artificial intelligence was the wave of the future, leading to a flurry of start-ups. Their products offered little business value at the time, and so the commercial commercial enthusiasm ended in disappointment, leading to a period now referred to as the “A.I. Winter.” The current resurgence will not fall short this time, and the economic potential in terms of new efficiency and new applications is strong.
Related Posts
AIQRATIONS
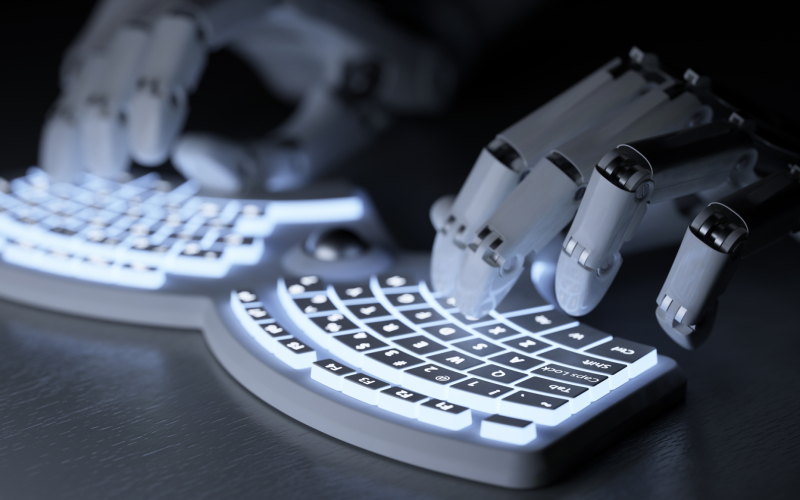
Chatbots – The Protege of AI & Data Sciences
Add Your Heading Text Here
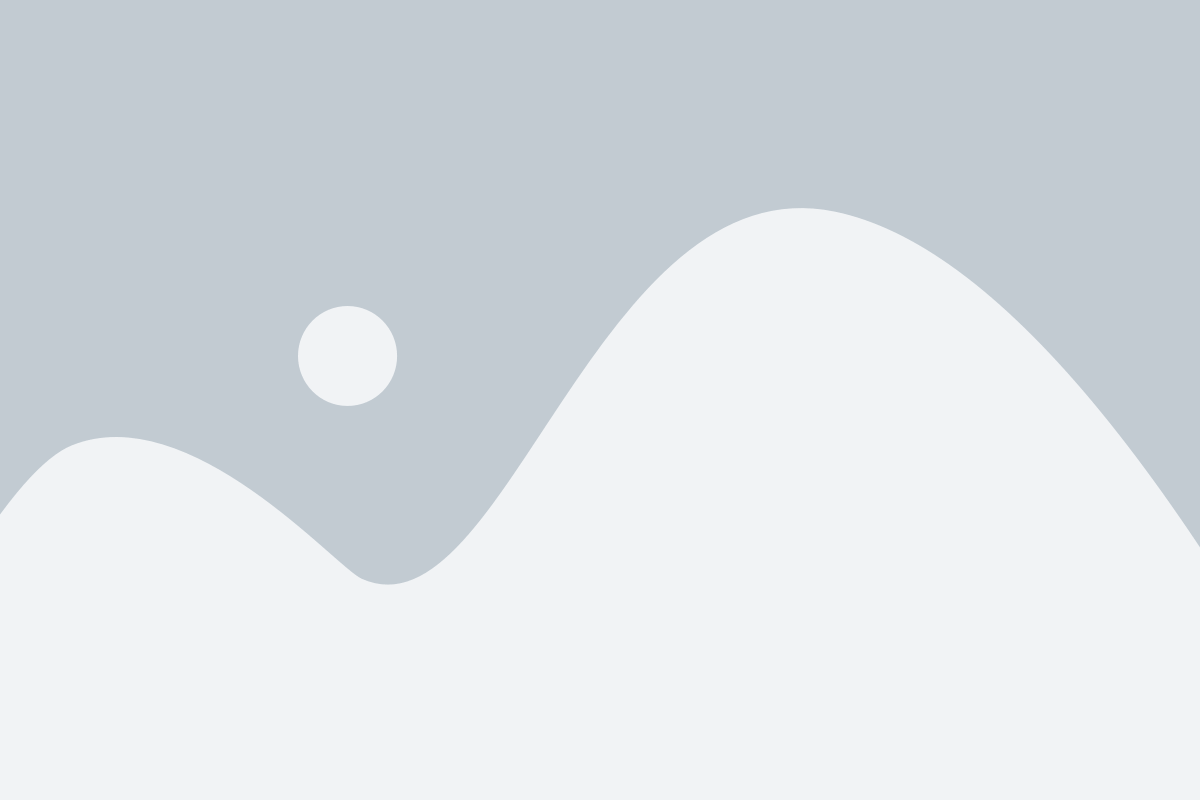
There has been a great deal of talk about the use of Artificial Intelligence chatbots in the last few weeks, especially given the news that Facebook are looking to implement AI into their Messenger and WhatsApp platforms, which are currently used by more than 1.8 billion people worldwide. However, does this bode well for the relationship between humans and Artificial Intelligence programs? Would you rather speak to an intelligent algorithm rather than a fellow human being?
The Sales and Customer Support Bot-ler ?
Chatbots, done right, are the cutting-edge form of interactive communications that captivate and engage users. But what kind of potential do they have for sales & customer support ?
To answer this, I should emphasize that customer service can be a delicate field. A lot of consumer engagement with a company happens when something goes wrong — such as a recently-purchased broken product or an incorrect bill or invoice.
By nature, these situations can be highly emotional. And as a business, you want to be responsive to potentially problematic customer inquiries like these. So relying on a chatbot to resolve issues that require a human touch might not be the best idea.
This is especially true if you let your bot “learn” from interactions it sees (say, in user forums) with no or minimal supervision. Things can easily go wrong, as the disaster around Microsoft’s Twitter bot “Tay” showed.
On the other hand, with the right supervision and enough training data, machine learning as an A.I. technique can help build very responsive and accurate informational chatbots — for example those that are meant to help surface data from large text collections, such as manuals.
I’d say that machine learning as a technique has been shown to work best on image processing. The advancements that Google, Facebook, and innovative startups such as Moodstocks (just acquired by Google) are showing in that space are truly amazing. Part of the amazement however, comes from the fact that we now see software take on another cognitive task that we thought could only be managed by humans.
What can bots do for the bottom line?
In my opinion, a bot’s primary application lies in customer service since most companies unfortunately continue to rely on an ancient methodology to manage customer interaction. And this is to be expected as most consumers themselves are still “hard-wired” to pick up a phone and dial a number when they want to engage with a company.
Companies haven’t necessarily made it easy for consumers to transition to digital-first interaction. Consumers are forced to either download a mobile app, browse websites, or use voice, the “dumbest” channel the smartphone has to offer, to retrieve information or perform transactions.
This is truly unfortunate because when it comes to paying a bill, checking on an order status, or reviewing account transactions, nothing is easier than sending a simple message. And with 900 million users now on Facebook Messenger, 1 billion on WhatsApp, and hundreds of millions more on basic SMS, companies have a consumer-preferred new medium for engaging with customers.
With messaging, a simple question can be posed in a simple message such as “Where is my order?”
Contrast this to the conventional options of being forced to shepherding that question through a maze of web or mobile app menus, or with IVR systems over the phone. Now imagine how a consumer-adopted, digital and automated interaction for simple questions vs. agent interaction over the phone could impact customer service and its cost. When chatbots handle the most commonly-asked questions, agent labor is reduced or redeployed to manage more complex and time-consuming interactions. Simple and moderate issues are resolved faster, leading to greater customer satisfaction and long-term loyalty. Bots can help deflect calls from the contact center and your IVR, which further reduces speech recognition license and telephony cost.
Could there be Bot-tle-necks?
There is also the question of whether these chatbots will take jobs from humans; a subject of fierce debate for all industries and levels in the last few months. Facebook itself has been quick to clarify that these chatbots are not going to replace the people in their organisation, but instead to work alongside them. For example, Facebook have said that the customer service executives will be required to train the AI bots, and to step in when the AI comes unstuck, which is likely to be fairly frequently in the early stages! Chinese messenger service WeChat has taken the chatbot idea on, with companies having official accounts through which they are able to communicate with their customers. However, the platform is still in its early stages, and is reported to be incredibly frustrating to use, so those in the customer service sector needn’t worry that their jobs are under threat quite yet!
While we might see chatbots starting to appear through the likes of Facebook Messenger and WhatsApp platforms in the coming 12 months, and will be dedicating teams of engineers to train the platforms, rather than relying on the general public. There are three main factors on which their success depends.
The first is with how much freedom AI in general is allowed to be developed, especially given the hesitation that the likes of Elon Musk and Bill Gates have about a potential ‘Singularity’, with Musk recently being quoted as saying that ‘Artificial Intelligence is our biggest existential threat’.
The second is arguably more important; how willing the general public are to help develop the chatbots, by having conversations with them, in the knowledge that they are talking to an autonomous entity.
More important still, are these chatbots going to be safe from cyberattacks? How will you know if your financial information will be secure if you disclose it to a chatbot, especially if there are unlikely to be the same multi-stage security checks that are the hallmark of P2P customer service interactions?
The Road Ahead?
Many companies are already launching bots for customer acquisition or customer service. We will see failures, and in parts, have already seen some. Bots are not trivial to build: you need people with experience in man-machine interface design. But to quote Amara’s Law: “We tend to overestimate the effect of a technology in the short run and underestimate the effect in the long run.”
Bots are here to stay, and will be a great new platform and make things easier for all of us. But bots that try to do too much or set unreasonable expectations will slow consumer confidence and acceptance of them. What might help us now is maybe to calm down a bit with the hype, and focus on building good bots that have value — then share our experiences, and show the world where the true value lies.
Related Posts
AIQRATIONS
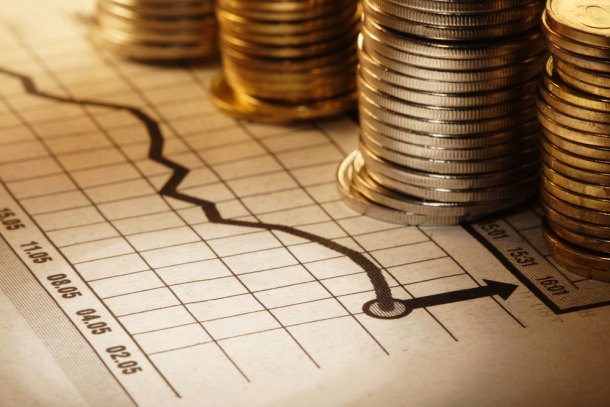
Data Sciences @ Fintech Companies for Competitive Disruption & Advantage
Add Your Heading Text Here
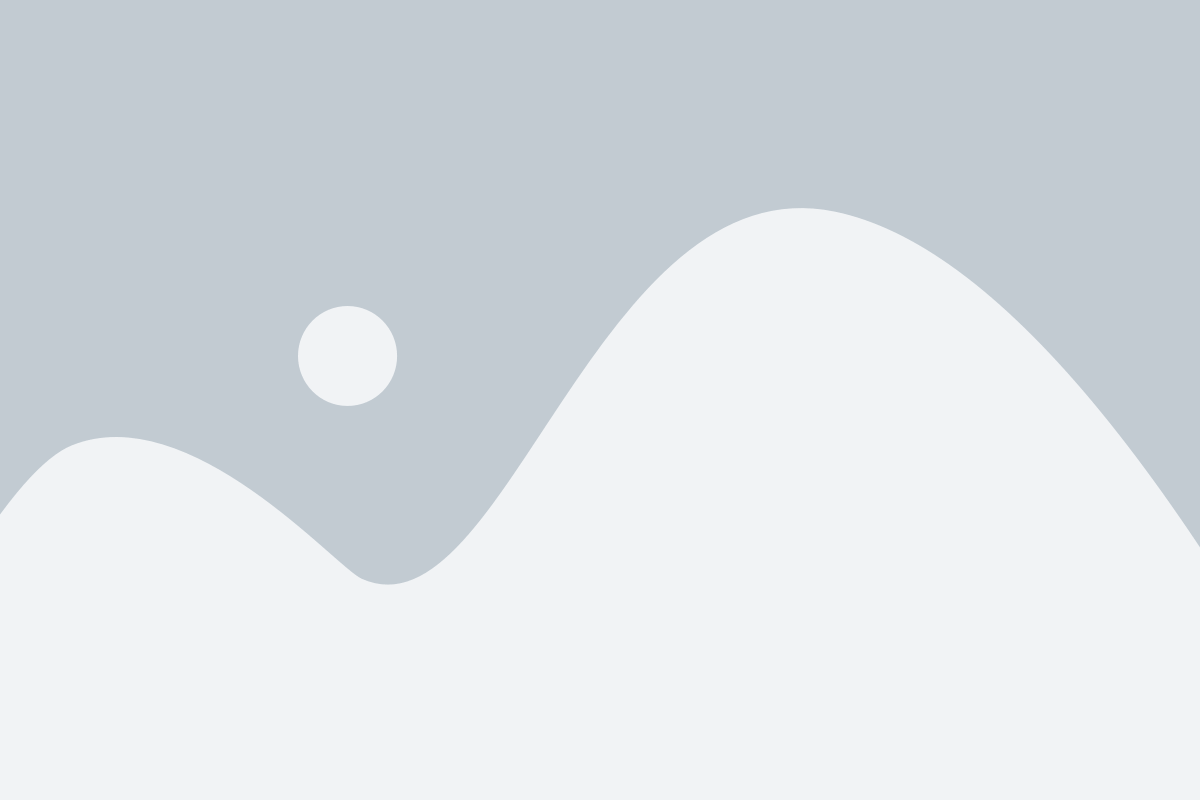
Long considered an impenetrable fortress dominated by a few well-known names, the banking and financial services industry is currently riding a giant wave of entrepreneurial disruption, disinter-mediation, and digital innovation. Everywhere, things are in flux. New, venture-backed arrivals are challenging the old powerhouses. Banks and financial services companies are caught between increasingly strict and costly regulations, and the need to compete through continuous innovation.
How does an entire industry remain relevant, authoritative, and trustworthy while struggling to surmount inflexible legacy systems, outdated business models, and a tired culture? Is there a way for banks and other traditional financial services companies to stay on budget while managing the competitive threat of agile newcomers and startups that do business at lower costs and with better margins? The threat is real. Can established institutions evolve in time to avoid being replaced? What other strategies can protect their extensive infrastructures and win the battle for the customer’s mind, heart, and wallet?
Financial technology, or fintech, is on fire with innovation and investment. The movement is reshaping entrepreneurial businesses and shaking the financial industry, reimagining the methods and tools consumers use to manage, save, and spend money. Agile fintech companies and their technology-intensive offerings do not shy away from big data, analytics, cloud computing, and machine learning, and they insist on a data-driven culture.
Consider a recent Forbes article by Chance Barnett, which quantifies fintech startup investments at $12 billion in 2014, quadrupling the $3 billion level achieved a year earlier. Adding to the wonderment, crowdfunding is likely to surpass venture capital in 2016 as a primary funding source. And people are joining the conversation. Barnett writes, “According to iQ Media, the number of mentions for ‘fintech’ on social media grew four times between 2013 and 2014, and will probably double again in 2015.” All of this activity underscores how technology is rattling the financial status quo and changing the very nature of money.
Yesterday’s Bank: A Rigid Culture, Strapped for Funds
Established banking institutions are strapped. The financial meltdown in 2008 questioned their operations, eroded trust, and invited punitive regulation designed to command, control, and correct the infractions of the past. Regulatory requirements have drained budgets, time, and attention, locking the major firms into constant compliance reporting. To the chagrin of some, these same regulations have also opened the door for new market entrants, technologies, platforms, and modalities—all of which are transforming the industry.
For traditional banking institutions, focus and energy for innovation are simply not there, nor are the necessary IT budgets. Gartner’s Q3 2015 forecast for worldwide IT spending growth (including all devices, data center systems, enterprise software, IT and Telecom services) hints at the challenge banks face: global IT spending is now down to -4.9%, even further from the -1.3% originally forecast, evidence of the spending and investment restraint we see across the financial landscape.
With IT budgets limited, it is hard to imagine banking firms easily reinventing themselves. Yet some are doing just that. Efficient spending is a top strategic priority for banking institutions. Many banks are moving away from a heavy concentration on compliance spending to instead focus on digital transformation, innovation, or collaboration with fintech firms. There is a huge amount of activity on all fronts. To begin, let’s review the competitive landscape of prominent fintech startups.
Data Sciences Intervention
Digital data has snowballed, with the proliferation of the internet, smartphones and other devices. Companies and governments alike recognize the massive potential in using this information – also known as Big Data – to drive real value for customers, and improve efficiency.
Big Data could transform businesses and economies, but the real game changer is data science.
Data science goes beyond traditional statistics to extract actionable insights from information – not just the sort of information you might find in a spreadsheet, but everything from emails and phone calls to text, images, video, social media data streaming, internet searches, GPS locations and computer logs.
“Data sciences enables us to process data better, faster and cheaper than ever
With powerful new techniques, including complex machine-learning algorithms, data science enables us to process data better, faster and cheaper than ever before.
We’re already seeing significant benefits of this – in areas such as national security, business intelligence, law enforcement, financial analysis, health care and disaster preparedness. From location analytics to predictive marketing to cognitive computing, the array of possibilities is overwhelming, sometimes even life-saving. The New York City Fire Department, for example, was one of the earlier success stories of using data science to proactively identify buildings most at risk from fire.
Unleashing the power of Advanced Data Mining using Data Sciences
For banks – in an era when banking is becoming commoditized – the data mining provides a massive opportunity to stand out from the competition. Every banking transaction is a nugget of data, so the industry sits on vast stores of information.
By using data science to collect and analyses Data, banks can improve, or reinvent, nearly every aspect of banking. Data science can enable hyper-targeted marketing, optimized transaction processing, personalized wealth management advice and more – the potential is endless.
A large proportion of the current Data Mining projects in banking revolve around customers – driving sales, boosting retention, improving service, and identifying needs, so the right offers can be served up at the right time.
“Data sciences can help strengthen risk management such as cards fraud detection
Banks can model their clients’ financial performance on multiple data sources and scenarios. Data science can also help strengthen risk management in areas such as cards fraud detection, financial crime compliance, credit scoring, stress-testing and cyber analytics.
The promise of Big Data is even greater than this, however, potentially opening up whole new frontiers in financial services.
Seamless experience for customers
Over 1.7 billion people with mobile phones are currently excluded from the formal financial system. This makes them invisible to credit bureaus, but they are increasingly becoming discoverable through their mobile footprint. Several innovative Fintech firms have already started building predictive models using this type of unconventional data to assess credit risk and provide new types of financing.
While banks have historically been good at running analytics at a product level, such as credit cards, or mortgages, very few have done so holistically, looking across inter-connected customer relationships that could offer a business opportunity – say when an individual customer works for, supplies or purchases from a company that is also a client of the bank. The evolving field of data science facilitates this seamless view.
Blockchain as the new database
Much more is yet to come. Blockchain, the underlying disruptive technology behind cryptocurrency Bitcoin, could spell huge change for financial services in the future. Saving information as ‘hash’, rather than in its original format, the blockchain ensures each data element is unique, time-stamped and tamper-resistant.
The semi-public nature of some types of blockchain paves the way for an enhanced level of security and privacy for sensitive data – a new kind of database where the information ‘header’ is public but the data inside is ‘private’.
As such, the blockchain has several potential applications in financial markets – think of trade finance, stock exchanges, central securities depositories, trade repositories or settlements systems.
Data analytics using blockchain, distributed ledger transactions and smart contracts will become critical in future, creating new challenges and opportunities in the world of data science.