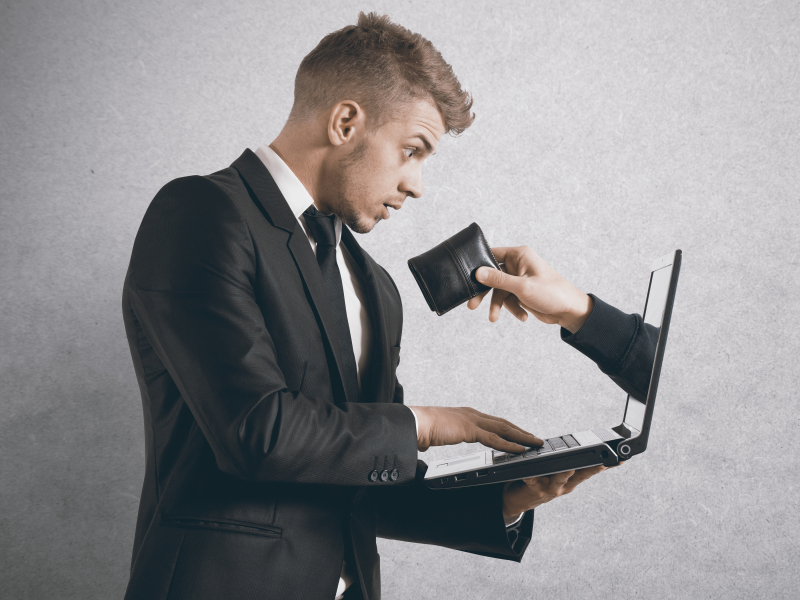
How AI is Enabling Mitigation of Fraud in the Banking, Insurance Enterprises
Add Your Heading Text Here
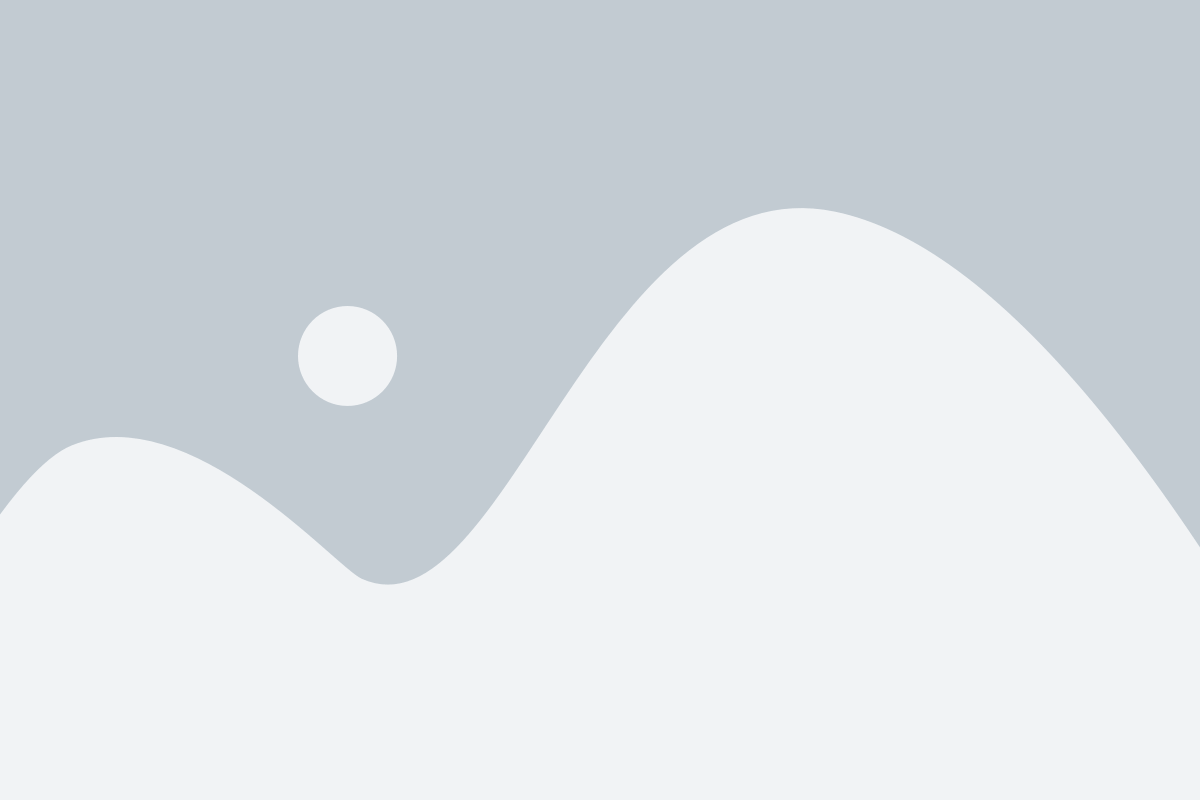
The Banking and Finance sector (BFSI) is witnessing one of its most interesting and enriching phases. Apart from the evident shift from traditional methods of banking and payments, technology has started playing a vital role in defining this change.
Mobile apps, plastic money, e-wallets and bots have aided the phenomenal swing from offline payments to online payments over the last two decades. Now, the use of Artificial Intelligence (AI) in BFSI is expediting the evolution of this industry.
But as the proliferation of digital continues, the number of ways one can commit fraud has also increased. Issuers, merchants, and acquirers of credit, debit, and prepaid general purpose and private label payment cards worldwide experienced gross fraud losses of US$11.27 billion in 2012, up 14.6% over the previous year1. Fraud losses on all general purpose and private label, signature and PIN payment cards reached US$5.33 billion in United States in the same period, up 14.5%1. These are truly big numbers, and present the single-biggest challenge to the trust reposed in banks by customers. Besides the risk of losing customers, direct financial impact for banks is also a significant factor.
Upon reporting of a fraudulent transaction by a customer, the bank is liable for the transaction cost, it has to refund merchant chargeback fee, as well as additional fee. Fraud also invites fines from regulatory authorities. The recently-passed Durbin Amendment caps processing fee that can be charged per transaction, and this increases the damage caused by unexpected fraud losses. The rapidly rising use of electronic payment modes has also increased the need for effective, efficient, and real-time methods to detect, deter, and prevent fraud.
Nuances of Banking Fraud Prevention Using AI
AI enables a computer to behave and take decisions like a human being. Coined in 1956 by John McCarthy at MIT, the term AI was little known to the layman and merely a subject of interest to academicians, researchers and technologists. However, over the past few years, it is more commonly seen in our everyday lives; in our smartphones, shopping experiences, hospitals, travel, etc.
Machine Learning, Deep Learning, NLP Platforms, Predictive APIs and Image and Speech Recognition are some core AI technologies used in BFSI today. Machine Learning recognises data patterns and highlights deviations in data observed. Data is analysed and then compared with existing data to look for patterns. This can help in fraud detection, prediction of spending patterns and subsequently, the development of new products.
Key Stroke Dynamics
Key Stroke Dynamics can be used for analysing transactions made by customers. They capture strokes when the key is pressed (dwell time) and released on a keyboard, along with vibration information.
As second factor authentication is mandatory for electronic payments, this can help detect fraud, especially if the user’s credentials are compromised. Deep Learning is a new area in Machine Learning research and consists of multiple linear and non-linear transformations. It is based on learning and improving representations of data. A common application of this can be found in the crypto-currency, Bitcoin.
Adaptive Learning
Adaptive Learning is another form of AI currently used by banks for fraud detection and mitigation. A model is created using existing rules or data in the bank’s system. Incremental learning algorithms are then used to update the models based on changes observed in the data patterns.
AI instances in Insurance for Fraud Prevention
Applying for Insurance
When a customer submits their application for insurance, there is an expectation that the potential policyholder provides honest and truthful information. However, some applicants choose to falsify information to manipulate the quote they receive.
To prevent this, insurers could use AI to analyse an applicant’s social media profiles and activity for confirmation that the information provided is not fraudulent. For example, in life insurance policies, social media pictures and posts may confirm whether an applicant is a smoker, is highly active, drinks a lot or is prone to taking risks. Similarly, social media may be able to indicate whether “fronting” (high-risk driver added as a named driver to a policy when they are in fact the main driver) is present in car insurance applications. This could be achieved by analysing posts to see if the named driver indicates that the car is solely used by them, or by assessing whether the various drivers on the policy live in a situation that would permit the declared sharing of the car.
Claims Management & Fraud Prevention
Insurance carriers can greatly benefit from the recent advances in artificial intelligence and machine learning. A lot of approaches have proven to be successful in solving problems of claims management and fraud detection. Claims management can be augmented using machine learning techniques in different stages of the claim handling process. By leveraging AI and handling massive amounts of data in a short time, insurers can automate much of the handling process, and for example fast-track certain claims, to reduce the overall processing time and in turn the handling costs while enhancing customer experience.
The algorithms can also reliably identify patterns in the data and thus help to recognize fraudulent claims in the process. With their self-learning abilities, AI systems can then adapt to new unseen cases and further improve the detection over time. Furthermore, machine learning models can automatically assess the severity of damages and predict the repair costs from historical data, sensors, and images.
Two companies tackling the management of claims are Shift Technology who offer a solution for claims management and fraud detection and RightIndem with the vision to eliminate friction on claims. Motionscloud offer a mobile solution for the claims handling process, including evidence collection and storage in various data formats, customer interaction and automatic cost estimation. ControlExpert handle claims for the auto insurance, with AI replacing specialized experts in the long-run. Cognotekt optimize business processes using artificial intelligence. Therefore the current business processes are analyzed to find the automation potentials. Applications include claims management, where processes are automated to speed up the circle time and for detecting patterns that would be otherwise invisible to the human eye, underwriting, and fraud detection, among others. AI techniques are potential game changers in the area of fraud. Fraudulent cases may be detected easier, sooner, more reliable and even in cases invisible to the human eye.
Conclusion
Those who wish to defraud insurance companies currently do so by finding ways to “beat” the system. For some uses of AI, fraudsters can simply modify their techniques to “beat” the AI system. In these circumstances, whilst AI creates an extra barrier to prevent and deter fraud, it does not eradicate the ability to commit insurance fraud. However, with other uses of AI, the software is able to create larger blockades through its use of “big data”. It can therefore provide more preventative assistance. As AI continues to develop, this assistance will become of greater use to the insurance industry in their fight against fraud.
Related Posts
AIQRATIONS
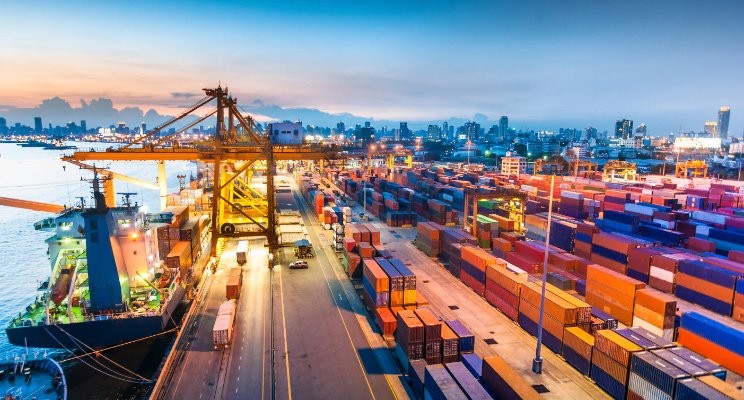
Fluid Supply Chain Transformation = AI + Automation
Add Your Heading Text Here
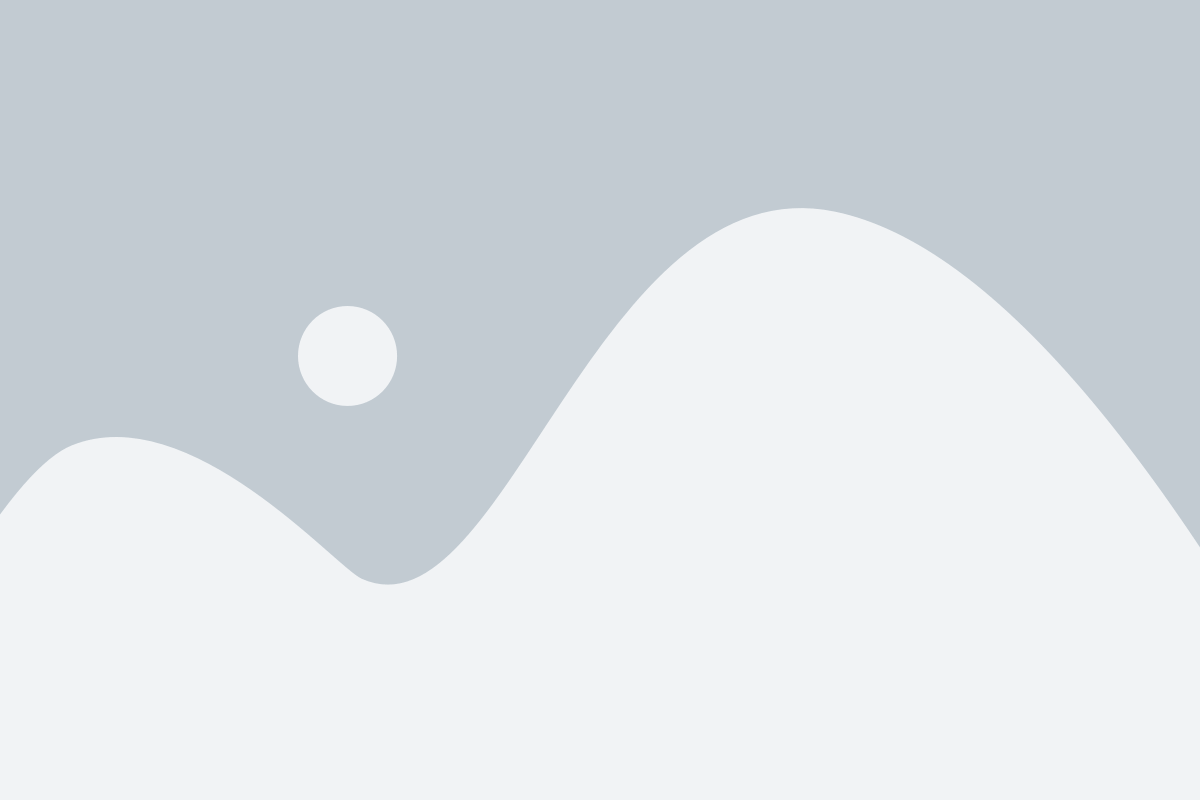
Rapidly evolving technology and a digitally focused world have opened the door for a new wave of automation to enter the workforce. Robots already stand side-by-side with their human counterparts on many manufacturing floors, adding efficiency, capacity (robots don’t need to sleep!) and dependability. Add in drones and self-driving vehicles and it’s no wonder many are questioning the role of humans going forward.
Supply chains, although automated to a degree, still face challenges brought about by the amount of slow, manual tasks required, and the daily management of a complex web of interdependent parts. The next generation of process efficiency gains and visibility could be on your doorstep with artificial intelligence in supply chain management, if only you’d let the robots automatically open it for you.
Robotic Process Automation
RPA works by automating the end-to-end supply chain, enabling the management of all tasks and sections in tandem. It allows you to spend less time on low value, high frequency activities like managing day-to-day processes, and provides more time to work on high value, exception-based requirements, which ultimately drives value for the entire business.
PwC estimates businesses could automate up to 45% of current work, saving $2 trillion in annual wages. “In addition to the cost and efficiency advantages, RPA can take a business to the next level of productivity optimization,” the firm says. Those ‘lights out’ factories and warehouses are becoming closer to a reality.
Four key elements need to be in place for you to take full advantage of robotic process automation in your supply chain:
- robots for picking orders and moving them through the facility;
- sensors to ensure product quality and stock;
- cognitive learning systems;
- and, artificial intelligence to turn processes into algorithms to guide the entire operation.
In addition, you’ll need strong collaboration internally and among suppliers and customers to tie all management systems back to order management and enterprise resource planning platforms.
Artificial Intelligence In Supply Chain Automation
AI is changing the traditional way in which companies are operating. Siemens in its “lights out” manufacturing plant, has automated some of its production lines to a point where they are run unsupervised for several weeks.
Siemens is also taking a step towards a larger goal of creating Industrie 4.0 or a fully self-organizing factory which will automate the entire supply chain. Here, the demand and order information would automatically get converted into work orders and be incorporated into the production process.
This would streamline manufacturing of highly customized products.
Artificial Intelligence In Supplier Management And Customer Service
Organizations are also increasingly leveraging AI for supplier management and customer management. IPsoft’s AI platform, Amelia automates work knowledge and is able to speak to the customers in more than 20 languages. A global oil and gas company has trained Amelia to help provide prompt and more efficient ways of answering invoicing queries from its suppliers. A large US-based media services organization taught Amelia how to support first line agents in order to raise the bar for customer service.
Artificial Intelligence In Logistics & Warehousing
Logistics function will undergo a fundamental change as artificial intelligence gets deployed to handle domestic and international movement of goods. DHL has stated that its use of autonomous fork lifts is “reaching a level of maturity” in warehouse operations. The next step would be driver less autonomous vehicles undertaking goods delivery operations.
Artificial Intelligence In Procurement
AI is helping drive cost reduction and compliance agenda through procurement by generating real time visibility of the spend data. The spend data is automatically classified by AI software and is checked for compliance and any exceptions in real time. Singapore government is carrying out trials of using artificial intelligence to identify and prevent cases of procurement fraud.
The AI algorithm analyzes HR and finance data, procurement requests, tender approvals, workflows, non-financial data like government employee’s family details and vendor employee to identify potentially corrupt or negligent practices. AI will also take up basic procurement activities in the near future thereby helping improve the procurement productivity.
Artificial Intelligence in new product development
AI has totally overhauled the new product development process.by reducing the time to market for new products. Instead of developing physical prototypes and testing the same, innovators are now creating 3D digital models of the product. AI facilitates interaction of the product developers in the digital space by recognizing the gestures and position of hand. For example, the act of switching on a button of a digital prototype can be accomplished by a gesture.
AI In Demand Planning And Forecasting
Getting the demand planning right is a pain point for many companies. A leading health food company leveraged analytics with machine learning capabilities to analyze their demand variations and trends during promotions.
The outcome of this exercise was a reliable, detailed model highlighting expected results of the trade promotion for the sales and marketing department. Gains included a rapid 20 percent reduction in forecast error and a 30 percent reduction in lost sales.
AI in Smart Logistics
The impact of data-driven and autonomous supply chains provides an opportunity for previously unimaginable levels of optimization in manufacturing, logistics, warehousing and last mile delivery that could become a reality in less than half a decade despite high set-up costs deterring early adoption in logistics.
Changing consumer behavior and the desire for personalization are behind two other top trends Batch Size One and On-demand Delivery: Set to have a big impact on logistics, on-demand delivery will enable consumers to have their purchases delivered where and when they need them by using flexible courier services.
A study by MHI and Deloitte found more than half (51%) of supply chain and logistics professionals believe robotics and automation will provide a competitive advantage. That’s up from 39% last year. While only 35% of the respondents said they’ve already adopted robotics, 74% plan to do so within the next 10 years. And that’s likely in part to keep up with key players like Amazon, who have been leading the robotics charge for the past few years.
What is the mantra ?
These examples showcase that in today’s dynamic world, AI embedded supply chains offer a competitive advantage. AI armed with predictive analytics can analyze massive amounts of data generated by the supply chains and help organizations move to a more proactive form of supply chain management.
Thus, in this digital age where the mantra is “evolve or be disrupted”, companies are leveraging AI to reinvent themselves and scale their businesses quickly. AI is becoming a key enabler of the changes that businesses need to make and is helping them manage complexity of the constant digital change.
Related Posts
AIQRATIONS
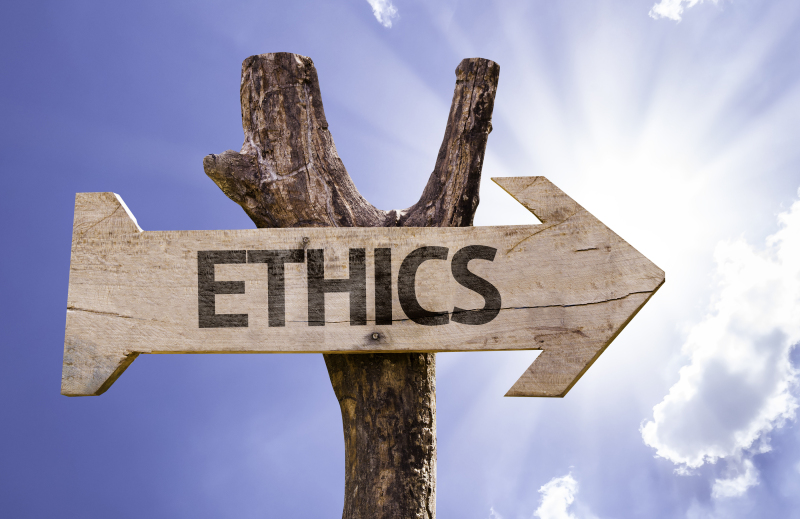
Ethics and Ethos in Analytics – Why is it Imperative for Enterprises to Keep Winning the Trust from Customers?
Add Your Heading Text Here
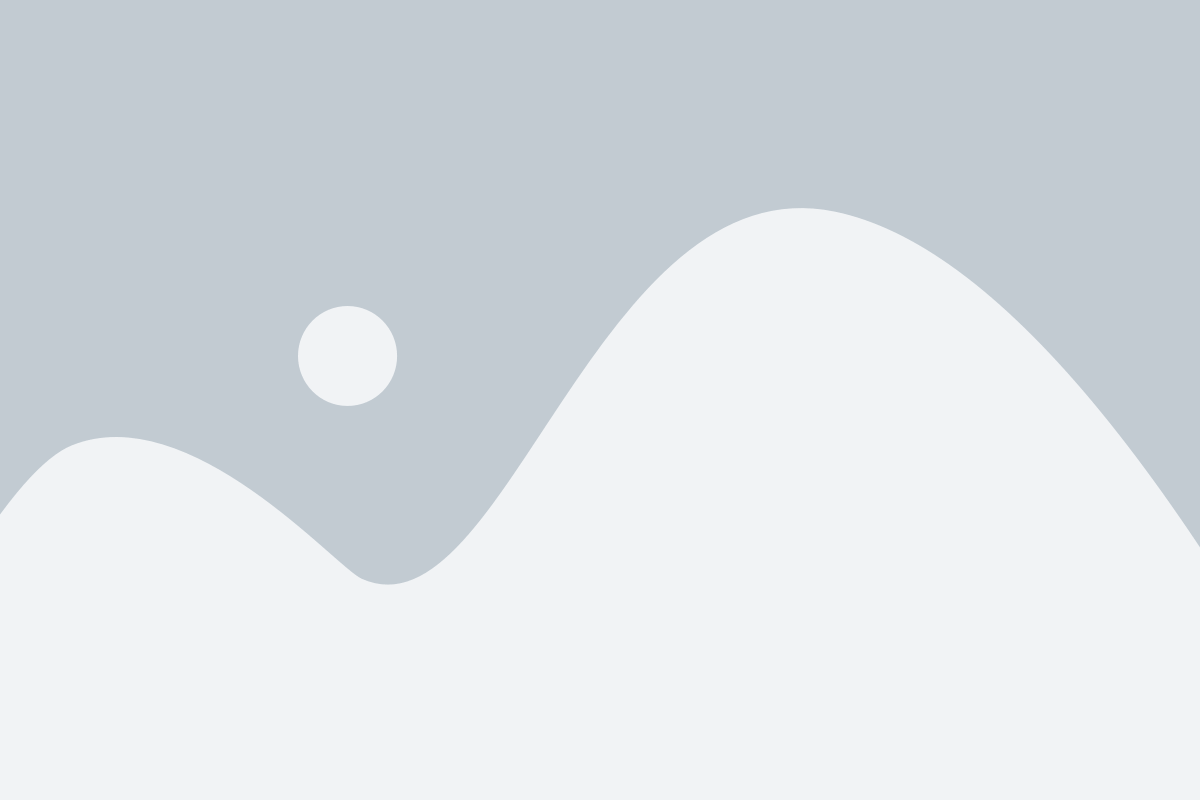
Data Sciences and analytics technology can reap huge benefits to both individuals and organizations – bringing personalized service, detection of fraud and abuse, efficient use of resources and prevention of failure or accident. So why are there questions being raised about the ethics of analytics, and its superset, Data Sciences?
Ethical Business Processes in Analytics Industry
At its core, an organization is “just people” and so are its customers and stakeholders. It will be individuals who choose what to organization does or does not do and individuals who will judge its appropriateness. As an individual, our perspective is formed from our experience and the opinions of those we respect. Not surprisingly, different people will have different opinions on what is appropriate use of Data Sciences and analytics technology particularly – so who decides which is “right”? Customers and stakeholders may have different opinions on to the organization about what is ethical.
This suggests that organizations should be thoughtful in their use of this Analytics; consulting widely and forming policies that record the decisions and conclusions they have come to. They will consider the wider implications of their activities including:
Context – For what purpose was the data originally surrendered? For what purpose is the data now being used? How far removed from the original context is its new use? Is this appropriate?
Consent & Choice – What are the choices given to an affected party? Do they know they are making a choice? Do they really understand what they are agreeing to? Do they really have an opportunity to decline? What alternatives are offered?
Reasonable – Is the depth and breadth of the data used and the relationships derived reasonable for the application it is used for?
Substantiated – Are the sources of data used appropriate, authoritative, complete and timely for the application?
Owned – Who owns the resulting insight? What are their responsibilities towards it in terms of its protection and the obligation to act?
Fair – How equitable are the results of the application to all parties? Is everyone properly compensated? Considered – What are the consequences of the data collection and analysis?
Access – What access to data is given to the data subject?
Accountable – How are mistakes and unintended consequences detected and repaired? Can the interested parties check the results that affect them?
Together these facets are called the ethical awareness framework. This framework was developed by the UK and Ireland Technical Consultancy Group (TCG) to help people to develop ethical policies for their use of analytics and Data Sciences. Examples of good and bad practices are emerging in the industry and in time they will guide regulation and legislation. The choices we make, as practitioners will ultimately determine the level of legislation imposed around the technology and our subsequent freedom to pioneer in this exciting emerging technical area.
Designing Digital Business for Transparency and Trust
With the explosion of digital technologies, companies are sweeping up vast quantities of data about consumers’ activities, both online and off. Feeding this trend are new smart, connected products—from fitness trackers to home systems—that gather and transmit detailed information.
Though some companies are open about their data practices, most prefer to keep consumers in the dark, choose control over sharing, and ask for forgiveness rather than permission. It’s also not unusual for companies to quietly collect personal data they have no immediate use for, reasoning that it might be valuable someday.
In a future in which customer data will be a growing source of competitive advantage, gaining consumers’ confidence will be key. Companies that are transparent about the information they gather, give customers control of their personal data, and offer fair value in return for it will be trusted and will earn ongoing and even expanded access. Those that conceal how they use personal data and fail to provide value for it stand to lose customers’ goodwill—and their business.
At the same time, consumers appreciate that data sharing can lead to products and services that make their lives easier and more entertaining, educate them, and save them money. Neither companies nor their customers want to turn back the clock on these technologies—and indeed the development and adoption of products that leverage personal data continue to soar. The consultancy Gartner estimates that nearly 5 billion connected “things” will be in use in 2015—up 30% from 2014—and that the number will quintuple by 2020.
Resolving this tension will require companies and policy makers to move the data privacy discussion beyond advertising use and the simplistic notion that aggressive data collection is bad. We believe the answer is more nuanced guidance—specifically, guidelines that align the interests of companies and their customers, and ensure that both parties benefit from personal data collection
Understanding the “Privacy Sensitiveness” of Customer Data
Keeping track of the “privacy sensitiveness” of customer data is also crucial as data and its privacy are not perfectly black and white. Some forms of data tend to be more crucial for customers to protect and maintained private. To see how much consumers valued their data , a conjoint analysis was performed to determine what amount survey participants would be willing to pay to protect different types of information. Though the value assigned varied widely among individuals, we are able to determine, in effect, a median, by country, for each data type.
The responses revealed significant differences from country to country and from one type of data to another. Germans, for instance, place the most value on their personal data, and Chinese and Indians the least, with British and American respondents falling in the middle. Government identification, health, and credit card information tended to be the most highly valued across countries, and location and demographic information among the least.
This spectrum doesn’t represents a “maturity model,” in which attitudes in a country predictably shift in a given direction over time (say, from less privacy conscious to more). Rather, our findings reflect fundamental dissimilarities among cultures. The cultures of India and China, for example, are considered more hierarchical and collectivist, while Germany, the United States, and the United Kingdom are more individualistic, which may account for their citizens’ stronger feelings about personal information.
Adopting Data Aggregation Paradigm for Protecting Privacy
If companies want to protect their users and data they need to be sure to only collect what’s truly necessary. An abundance of data doesn’t necessarily mean that there is an abundance of useable data. Keeping data collection concise and deliberate is key. Relevant data must be held in high regard in order to protect privacy.
It’s also important to keep data aggregated in order to protect privacy and instill transparency. Algorithms are currently being used for everything from machine thinking and autonomous cars, to data science and predictive analytics. The algorithms used for data collection allow companies to see very specific patterns and behavior in consumers all while keeping their identities safe.
One way companies can harness this power while heeding privacy worries is to aggregate their data…if the data shows 50 people following a particular shopping pattern, stop there and act on that data rather than mining further and potentially exposing individual behavior.
Things are getting very interesting…Google, Facebook, Amazon, and Microsoft take the most private information and also have the most responsibility. Because they understand data so well, companies like Google typically have the strongest parameters in place for analyzing and protecting the data they collect.
Finally, Analyze the Analysts
Analytics will increasingly play a significant role in the integrated and global industries today, where individual decisions of analytics professionals may impact the decision making at the highest levels unimagined years ago. There’s a substantial risk at hand in case of a wrong, misjudged model / analysis / statistics that can jeopardize the proper functioning of an organization.
Instruction, rules and supervisions are essential but that alone cannot prevent lapses. Given all this, it is imperative that Ethics should be deeply ingrained in the analytics curriculum today. I believe, that some of the tenets of this code of ethics and standards in analytics and data science should be:
- These ethical benchmarks should be regardless of job title, cultural differences, or local laws.
- Places integrity of analytics profession above own interests
- Maintains governance & standards mechanism that data scientists adhere to
- Maintain and develop professional competence
- Top managers create a strong culture of analytics ethics at their firms, which must filter throughout their entire analytics organization
Related Posts
AIQRATIONS
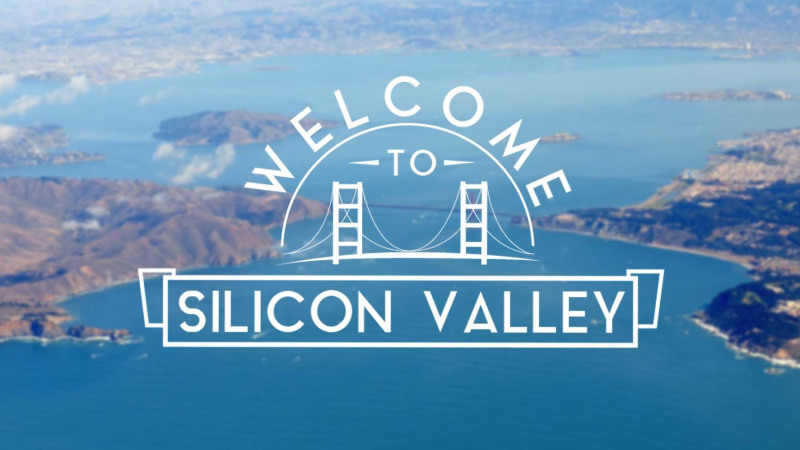
The Rush for Artificial Intelligence in Silicon Valley…Is This Here to Stay?
Add Your Heading Text Here
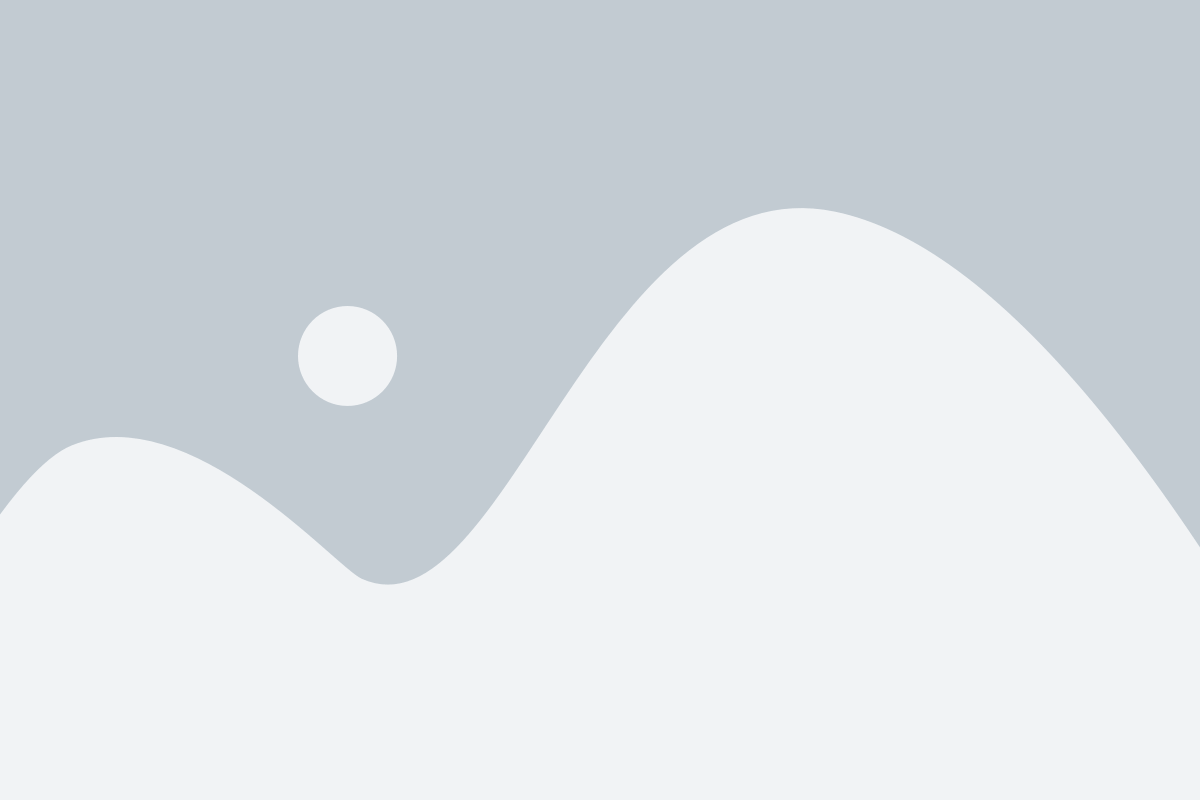
For more than a decade, Silicon Valley’s technology investors and entrepreneurs obsessed over social media and mobile apps that helped people do things like find new friends, fetch a ride home or crowdsource a review of a product or a movie.
Robots after the “Like” Button
Now Silicon Valley has found its next shiny new thing. And it does not have a “Like” button.
The new era in Silicon Valley centers on artificial intelligence and robots, a transformation that many believe will have a payoff on the scale of the personal computing industry or the commercial internet, two previous generations that spread computing globally. Computers have begun to speak, listen and see, as well as sprout legs, wings and wheels to move unfettered in the world.
The shift was evident in a Lowe’s home improvement store here this month, when a prototype inventory checker developed by Bossa Nova Robotics silently glided through the aisles using computer vision to automatically perform a task that humans have done manually for centuries.
The robot, which was skilled enough to autonomously move out of the way of shoppers and avoid unexpected obstacles in the aisles, alerted people to its presence with soft birdsong chirps. Gliding down the middle of an aisle at a leisurely pace, it can recognize bar codes on shelves, and it uses a laser to detect which items are out of stock.
Silicon Valley’s financiers and entrepreneurs are digging into artificial intelligence with remarkable exuberance. The region now has at least 19 companies designing self-driving cars and trucks, up from a handful five years ago. There are also more than a half-dozen types of mobile robots, including robotic bellhops and aerial drones, being commercialized.
The Surge after the Static – The Social Way
There has been a slow trickle in investments in robotics all this while, and suddenly, there seem to be a dozen companies securing large investment rounds focusing on specific robotic niches. Funding in A.I. start-ups has increased more than fourfold to $681 million in 2015, from $145 million in 2011, according to the market research firm CB Insights. The firm estimates that new investments will reach $1.2 billion this year, up 76 percent from last year.
By contrast, funding for social media start-ups peaked in 2011 before plunging. That year, venture capital firms made 66 social media deals and pumped in $2.4 billion. So far this year, there have been just 10 social media investments, totaling $6.9 million, according to CB Insights. Last month, the professional social networking site LinkedIn was sold to Microsoft for $26.2 billion, underscoring that social media has become a mature market sector.
Even Silicon Valley’s biggest social media companies are now getting into artificial intelligence, as are other tech behemoths. Facebook is using A.I. to improve its products. Google will soon compete with Amazon’s Echo and Apple’s Siri, which are based on A.I., with a device that listens in the home, answers questions and places e-commerce orders. Satya Nadella, Microsoft’s chief executive, recently appeared at the Aspen Ideas Conference and called for a partnership between humans and artificial intelligence systems in which machines are designed to augment humans.
The auto industry has also set up camp in the valley to learn how to make cars that can do the driving for you. Both technology and car companies are making claims that increasingly powerful sensors and A.I. software will enable cars to drive themselves with the push of a button as soon as the end of this decade — despite recent Tesla crashes that have raised the question of how quickly human drivers will be completely replaced by the technology.
AI Outdoes the Silicon Valley Reset Trend
Silicon Valley’s new A.I. era underscores the region’s ability to opportunistically reinvent itself and quickly follow the latest tech trend. This is at the heart of the region’s culture that goes all the way back to the Gold Rush. The valley is built on the idea that there is always a way to start over and find a new beginning.
The change spurred a rush for talent in A.I. that has become intense. It is unusual that the number of people trying to get the students to drop out of the class halfway through because now they know a little bit of this stuff is crazy. The valley’s tendency toward reinvention dates back to the region’s initial emergence from the ashes of a deep aerospace industry recession as a consumer-electronics manufacturing center producing memory chips, video games and digital watches in the mid-1970s. A malaise in the personal computing market in the early 1990s was followed by the World Wide Web and the global expansion of the consumer internet.
A decade later, in 2007, just as innovation in mobile phones seemed to be on the verge of moving away from Silicon Valley to Europe and Asia, Apple introduced the first iPhone, resetting the mobile communications marketplace and ensuring that the valley would — for at least another generation — remain the world’s innovation center.
In the most recent shift, the A.I. idea emerged first in Canada in the work of cognitive scientists and computer scientists like Geoffrey Hinton, Yoshua Bengio and Yann LeCun during the previous decade. The three helped pioneer a new approach to deep learning, a machine learning method that is highly effective for pattern recognition challenges like vision and speech. Modeled on a general understanding of how the human brain works, it has helped technologists make rapid progress in a wide range of A.I. fields.
The Road Ahead
How far the A.I. boom will go is hotly debated. For some technologists, today’s technical advances are laying the groundwork for truly brilliant machines that will soon have human-level intelligence. Yet Silicon Valley has faced false starts with A.I. before. During the 1980s, an earlier generation of entrepreneurs also believed that artificial intelligence was the wave of the future, leading to a flurry of start-ups. Their products offered little business value at the time, and so the commercial commercial enthusiasm ended in disappointment, leading to a period now referred to as the “A.I. Winter.” The current resurgence will not fall short this time, and the economic potential in terms of new efficiency and new applications is strong.
Related Posts
AIQRATIONS
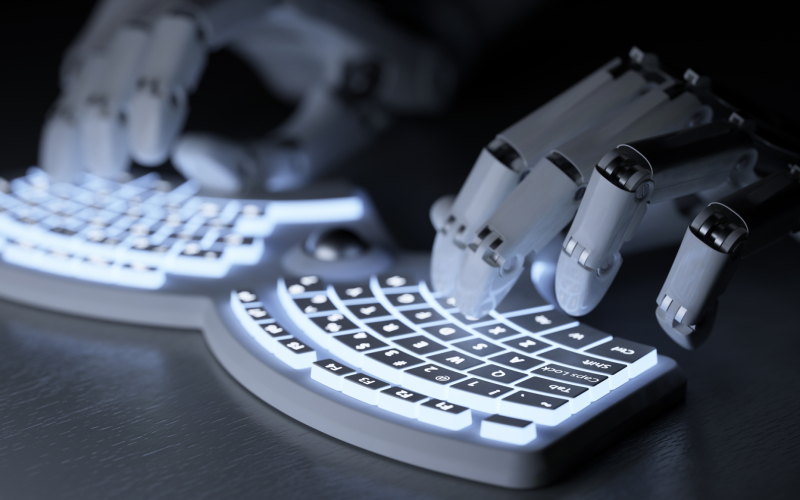
Chatbots – The Protege of AI & Data Sciences
Add Your Heading Text Here
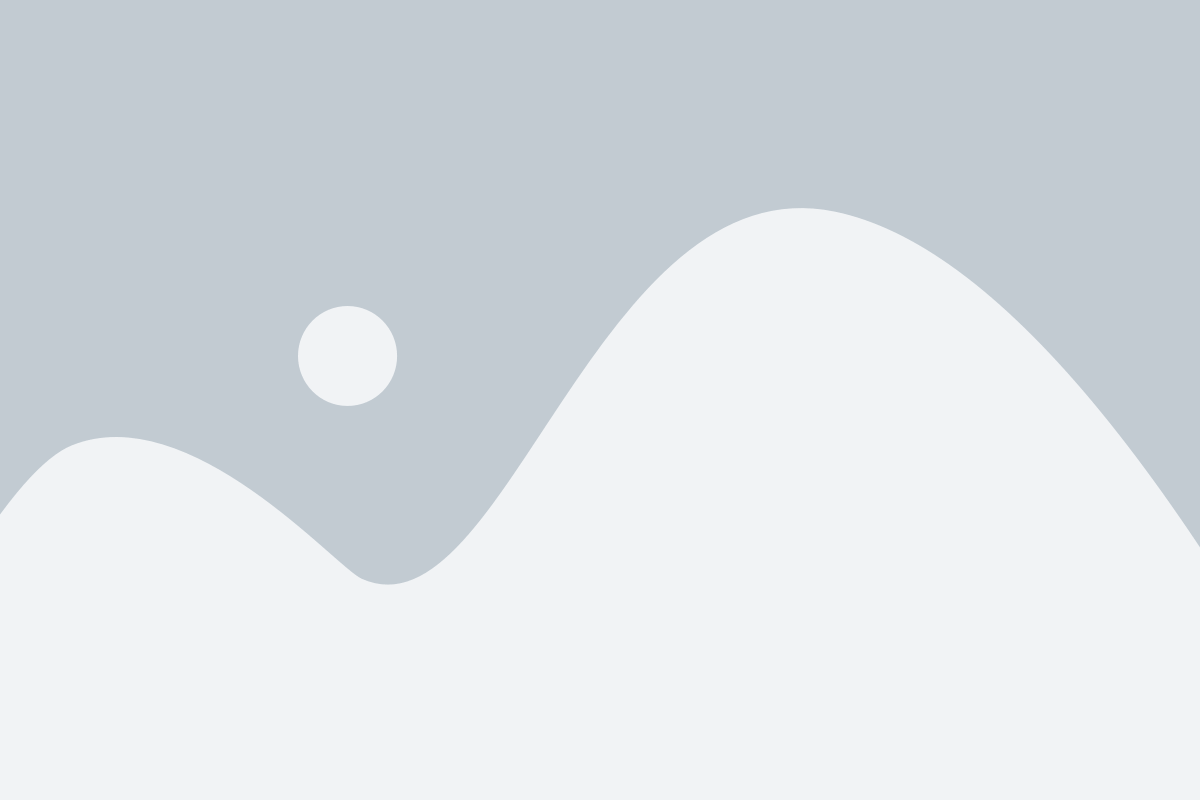
There has been a great deal of talk about the use of Artificial Intelligence chatbots in the last few weeks, especially given the news that Facebook are looking to implement AI into their Messenger and WhatsApp platforms, which are currently used by more than 1.8 billion people worldwide. However, does this bode well for the relationship between humans and Artificial Intelligence programs? Would you rather speak to an intelligent algorithm rather than a fellow human being?
The Sales and Customer Support Bot-ler ?
Chatbots, done right, are the cutting-edge form of interactive communications that captivate and engage users. But what kind of potential do they have for sales & customer support ?
To answer this, I should emphasize that customer service can be a delicate field. A lot of consumer engagement with a company happens when something goes wrong — such as a recently-purchased broken product or an incorrect bill or invoice.
By nature, these situations can be highly emotional. And as a business, you want to be responsive to potentially problematic customer inquiries like these. So relying on a chatbot to resolve issues that require a human touch might not be the best idea.
This is especially true if you let your bot “learn” from interactions it sees (say, in user forums) with no or minimal supervision. Things can easily go wrong, as the disaster around Microsoft’s Twitter bot “Tay” showed.
On the other hand, with the right supervision and enough training data, machine learning as an A.I. technique can help build very responsive and accurate informational chatbots — for example those that are meant to help surface data from large text collections, such as manuals.
I’d say that machine learning as a technique has been shown to work best on image processing. The advancements that Google, Facebook, and innovative startups such as Moodstocks (just acquired by Google) are showing in that space are truly amazing. Part of the amazement however, comes from the fact that we now see software take on another cognitive task that we thought could only be managed by humans.
What can bots do for the bottom line?
In my opinion, a bot’s primary application lies in customer service since most companies unfortunately continue to rely on an ancient methodology to manage customer interaction. And this is to be expected as most consumers themselves are still “hard-wired” to pick up a phone and dial a number when they want to engage with a company.
Companies haven’t necessarily made it easy for consumers to transition to digital-first interaction. Consumers are forced to either download a mobile app, browse websites, or use voice, the “dumbest” channel the smartphone has to offer, to retrieve information or perform transactions.
This is truly unfortunate because when it comes to paying a bill, checking on an order status, or reviewing account transactions, nothing is easier than sending a simple message. And with 900 million users now on Facebook Messenger, 1 billion on WhatsApp, and hundreds of millions more on basic SMS, companies have a consumer-preferred new medium for engaging with customers.
With messaging, a simple question can be posed in a simple message such as “Where is my order?”
Contrast this to the conventional options of being forced to shepherding that question through a maze of web or mobile app menus, or with IVR systems over the phone. Now imagine how a consumer-adopted, digital and automated interaction for simple questions vs. agent interaction over the phone could impact customer service and its cost. When chatbots handle the most commonly-asked questions, agent labor is reduced or redeployed to manage more complex and time-consuming interactions. Simple and moderate issues are resolved faster, leading to greater customer satisfaction and long-term loyalty. Bots can help deflect calls from the contact center and your IVR, which further reduces speech recognition license and telephony cost.
Could there be Bot-tle-necks?
There is also the question of whether these chatbots will take jobs from humans; a subject of fierce debate for all industries and levels in the last few months. Facebook itself has been quick to clarify that these chatbots are not going to replace the people in their organisation, but instead to work alongside them. For example, Facebook have said that the customer service executives will be required to train the AI bots, and to step in when the AI comes unstuck, which is likely to be fairly frequently in the early stages! Chinese messenger service WeChat has taken the chatbot idea on, with companies having official accounts through which they are able to communicate with their customers. However, the platform is still in its early stages, and is reported to be incredibly frustrating to use, so those in the customer service sector needn’t worry that their jobs are under threat quite yet!
While we might see chatbots starting to appear through the likes of Facebook Messenger and WhatsApp platforms in the coming 12 months, and will be dedicating teams of engineers to train the platforms, rather than relying on the general public. There are three main factors on which their success depends.
The first is with how much freedom AI in general is allowed to be developed, especially given the hesitation that the likes of Elon Musk and Bill Gates have about a potential ‘Singularity’, with Musk recently being quoted as saying that ‘Artificial Intelligence is our biggest existential threat’.
The second is arguably more important; how willing the general public are to help develop the chatbots, by having conversations with them, in the knowledge that they are talking to an autonomous entity.
More important still, are these chatbots going to be safe from cyberattacks? How will you know if your financial information will be secure if you disclose it to a chatbot, especially if there are unlikely to be the same multi-stage security checks that are the hallmark of P2P customer service interactions?
The Road Ahead?
Many companies are already launching bots for customer acquisition or customer service. We will see failures, and in parts, have already seen some. Bots are not trivial to build: you need people with experience in man-machine interface design. But to quote Amara’s Law: “We tend to overestimate the effect of a technology in the short run and underestimate the effect in the long run.”
Bots are here to stay, and will be a great new platform and make things easier for all of us. But bots that try to do too much or set unreasonable expectations will slow consumer confidence and acceptance of them. What might help us now is maybe to calm down a bit with the hype, and focus on building good bots that have value — then share our experiences, and show the world where the true value lies.
Related Posts
AIQRATIONS
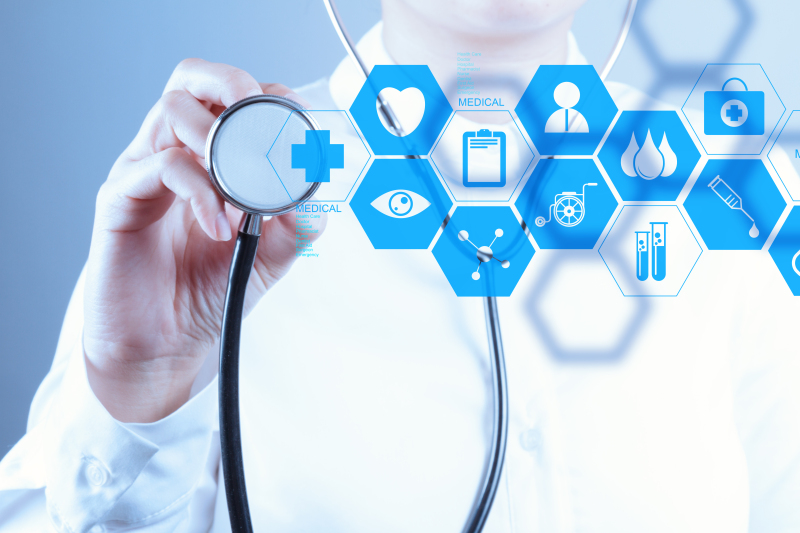
How Healthcare Industry will Benefit by Embracing Data Sciences
Add Your Heading Text Here
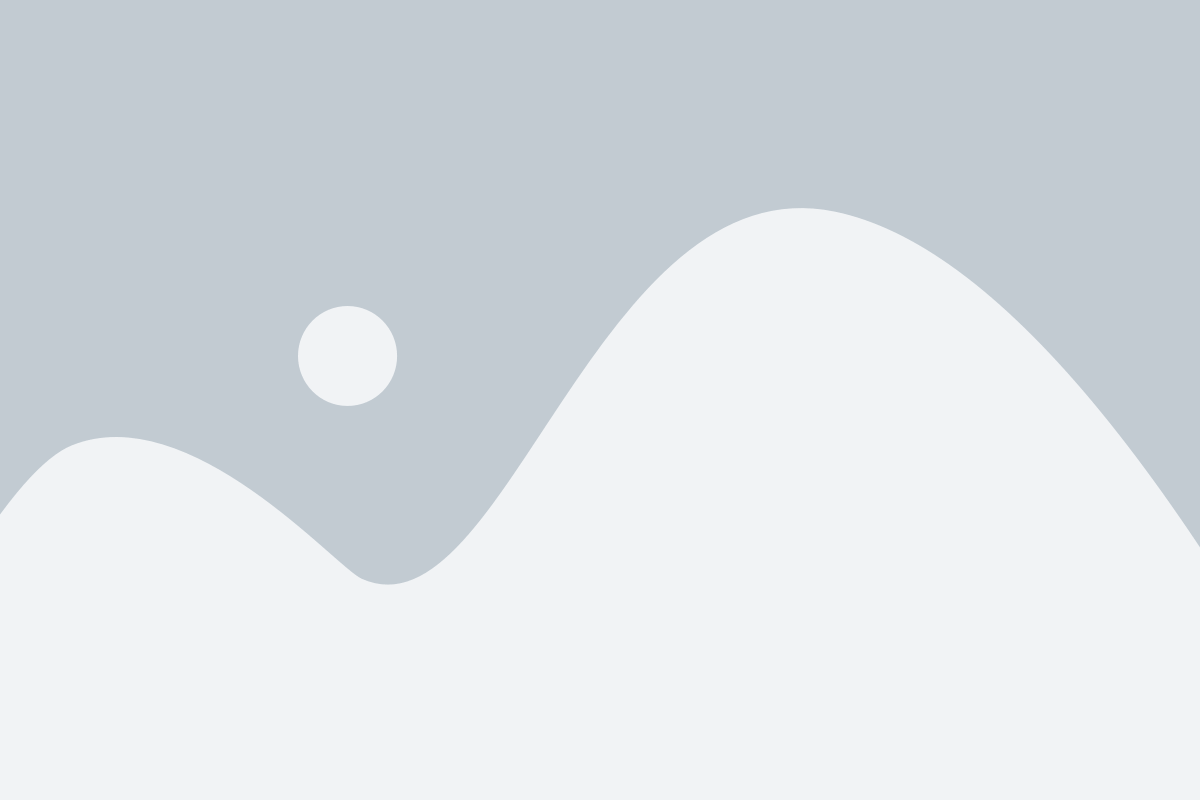
In the healthcare industry, what could be more important than having better healthcare outcomes? Each and every day healthcare workers around the globe are striving hard to find more ways of improving our lives. However, the world is changing, and frankly, at a faster rate than most of us can keep up. Intuition alone will no longer be enough for quality patient outcomes. The amount of healthcare data continues to mound every second, making it harder and harder to find any form of helpful information. Big Data is not to be romanticized; it can be a blessing and a curse. It can contribute to both the insight and the fog of visibility.
In truth, data science is proving invaluable to improving outcomes due to its ability to automate so much of the heavy lifting – in fast, scalable, and precise ways. All one has to do is look at our ability to predict epidemics, advance cures, and make patient stays in hospitals safer and more pleasant. In healthcare, data science should be seen as a beneficial intelligence rather than only artificial intelligence, providing an augmentation of services to the healthcare experts already in play.
Hospital Claims Data
In 2010, there were 35.1 million discharges with an average length of stay of 4.8 days according to the National Hospital Discharge Survey. That same survey went on to note that there were 51.4 million procedures performed. The National Hospital Ambulatory Medical Care Survey in 2011 stated the number of outpatient department visits were 125.7 million with 136.3 million emergency department visits. These are some of the basic figures showing the amount of care the U.S. health care system has provided. Using Data Science to annualize this sort of data allows healthcare providers to start building a new intuition built on a data narrative that could possibly help avoid the spread of diseases or address specific health threats. Using a combination of descriptive statistics, exploratory data analysis, and predictive analytics, it becomes relatively easy to identify the most cost-effective treatments for specific ailments and allows for a process to help reduce the number of duplicate or unnecessary treatments. The power in predicting a future state is in using that knowledge to change the behavior patterns of today.
Electronic Health Record (EHR)
Interoperable electronic health records (EHRs) for patient care hold tremendous potential to reduce the growth in costs. EHRs can help healthcare organizations improve chronic disease management, increase operating efficiencies, transform their finances, and improve patient outcomes. However, EHR implementations are in various stages of maturity across the country, and their benefits have not been fully realized. One of the primary challenges healthcare decision-makers face is how to make meaningful use of the data collected, available, and accessible in EHRs.
By optimizing the use of data accessible in EHRs, we can uncover hidden relationships and identify patterns and trends in this diverse and complex information to improve chronic disease management, increase operating efficiencies, and transform healthcare organizations’ finances.
Patient Behavior and Sentiment Data
A study by AMI Research suggests that “wearables” are expected to reach $52 million by 2019. Wearables monitor heart rates, sleep patterns, walking, and much more while providing new dimensions of context, geolocation, behavioral pattern, and biometrics. Combine this with the unstructured “lifestyle” data that comes across social media and you have a potent combination that is more than just numbers and tweets.
It is obvious that we will experience huge benefits from analyzing the in’s and out’s of healthcare data. In my judgment, we will continue to see a push for prevention over cure which puts predicting outcomes front and center. After all, catching things in the earlier stages is easier to treat and outbreaks can be more easily contained.
It may not resonate as widely today, but in the future we will look back on data science as something significant for healthcare. It is reasonable to expect that we will likely recover more quickly from illness and injury, live longer because of newly discovered drugs, and benefit from more efficient hospital surgeries – and in large part this will be because of how we analyze Big Data.
What makes living in the era of Big Data such a delight is that the healthcare industry is being pressed to find better tools, skills, and techniques to deal competently with the deluge of patient data and its inherent insights? When healthcare makes the choice to fully embrace data science, it will change the future for everyone.
Genomics
Inexpensive DNA sequencing and next-generation genomic technologies are changing the way healthcare providers do business. We now have the ability to map entire DNA sequences and measure tens of thousands of blood components to assess health.
Next-generation genomic technologies allow data scientists to drastically increase the amount of genomic data collected on large study populations. When combined with new informatics approaches that integrate many kinds of data with genomic data in Healthcare applications such as disease research, Prescription Effectiveness etc, we will better understand the genetic bases of drug response and disease. Researchers aim to achieve ultra-personalized healthcare. As a beginning, the FDA has already begun to issue medicine labels that specify different dosages for patients with particular genetic variants.
Predictive Analytics and Preventive Measures
Prevention is always better than cure. For the health-care industry, it also happens to save a lot of money. (The Centers for Medicaid and Medicare Services, for instance, can penalize hospitals that exceed average rates of readmission – indicating that they could be doing more to prevent medical problems.)
Take, for example, the partnership between Mount Sinai Medical Center and former Facebook guru Jeff Hammerbach. Mount Sinai’s problem was how to reduce readmission rates. Hammerbach’s solution was predictive analytics:
- In a pilot study, Hammerbach and his team combined data on disease, past hospital visits and other factors to determine a patient’s risk of readmission.
- These high-risk patients would then receive regular communication from hospital staff to help them avoid getting sick again.
Sinai isn’t alone. In 2008, Texas Health partnered with Healthways to merge and analyze clinical and insurance claims information. Their goal was the same – identify high-risk patients and offer them customized interventions.
Meanwhile, in 2013, data scientists at Methodist Health System are looking at accountable-care organization claims from 14,000 Medicare beneficiaries and 6,000 employees. Their aim? You guessed it. Predict which patients will need high-cost care in the future.
Patient Monitoring and Home Devices
Doctors can do a lot, but they can’t follow a patient around every minute of the day. Wearable body sensors – sensors tracking everything from heart rate to testosterone to body water – can.
Sensors are just one way in which medical technology is moving beyond the hospital bed. Home-use, medical monitoring devices and mobile applications are cropping up daily. A scanner to diagnose melanomas? A personal EEG heart monitor? No problem.
These gadgets are designed to help the patient, naturally, but they’re also busy harvesting data.
For example:
- Asthmapolis’s GPS-enabled tracker, already available by 2011, records inhaler usage by asthmatics. This information is collated, analyzed and merged with data on asthma catalysts from the CDC (e.g., high pollen counts in New England) to help doctors learn how best to prevent attacks.
- With Ginger.io’s mobile application, out in 2012, patients consent to have data about their calls, texts, location and movements monitored. These are combined with data on behavioral health from the NIH and other sources to pinpoint potential problems. Too many late-night phone calls, for instance, might signal a higher risk of anxiety attack.
- To improve patient drug compliance, Eliza, a Boston-based company, monitors which types of reminders work on which types of people. Smarter targeting means more compliance.
The Challenges Ahead
There are plenty of hurdles to creating a data-driven health care industry. Some are technical, some emotional. Health care providers have had decades to accumulate paper records, inefficiencies and entrenched routines. A remedy will not be quick.
And some say it shouldn’t. At least, not without a hard look at patient privacy, data ownership and the overall direction of U.S. health care.
Related Posts
AIQRATIONS
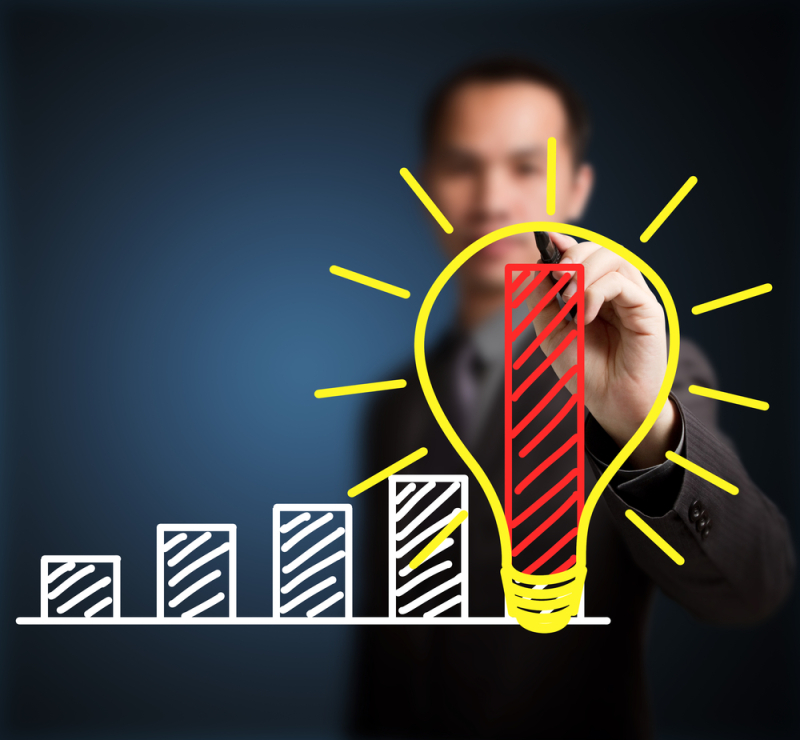
How Startups can Improve Visibility in the Market Using Analytics
Add Your Heading Text Here
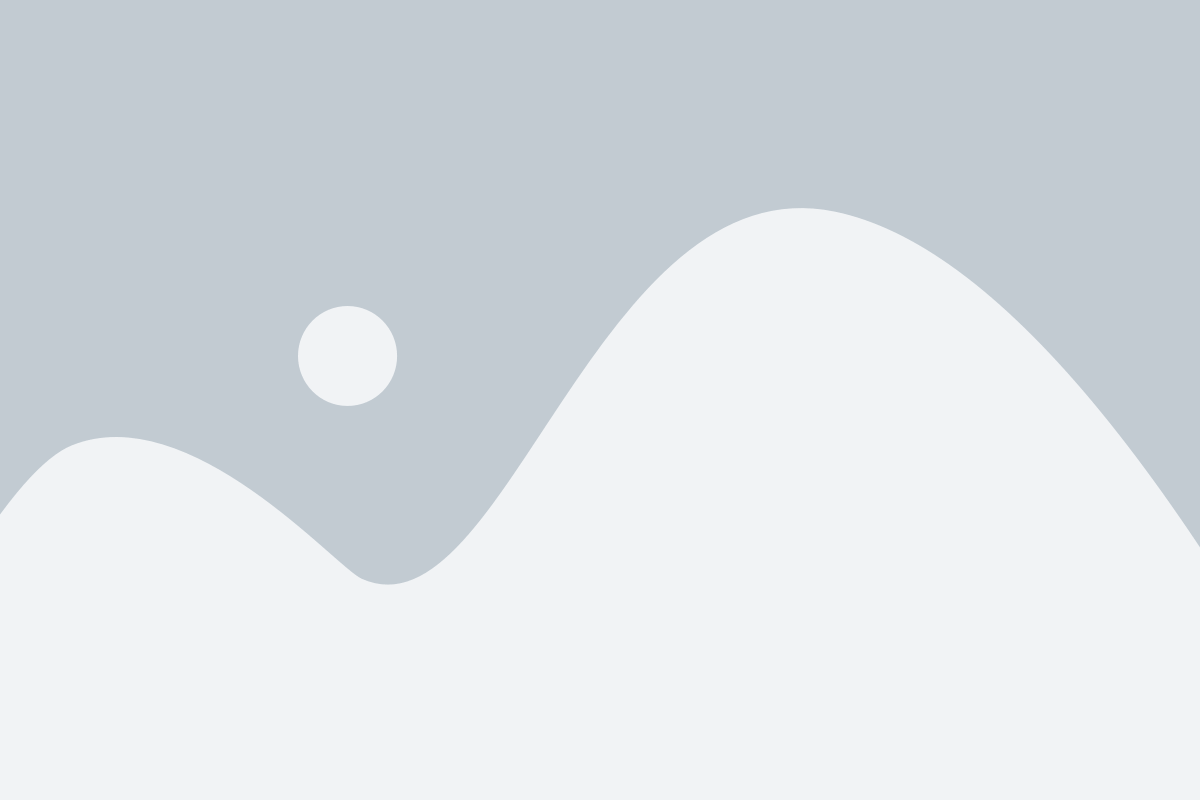
One might be tempted to think we are living in a startup bubble, with investors being largely optimistic about startups and investing millions of dollars in them, with many startups crossing the billion dollar valuation on a regular basis. But managing a startup is tough, with almost unreal targets set in between funding rounds. The founders need to, at all times, be focused on the direction in which they need to head, and be sure of the selective performance indicators that they need to keep watch of. Creating data has become easy at current times. Though acquiring data from multiple sources has its potential benefits, but for a company at its seminal stage, dealing with multiple KPIs is a huge risk. Startups can easily get side-tracked by following the wrong KPI. In an ideal scenario, startups should keep only one performance indicator and keep scaling up in that direction before achieving a milestone and involving others in the development plan. With a large number of startups around, existence of a red ocean, and ample amount of data giving number of insights and scope for strategies, implementing analytics can be a sure shot way to keep startups focused on the optimal way to scale up, and in extension create organic buzz and visibility to scale further. Analytics has the capacity to point out which should be the root nerve of a startup and how to scale further in that direction. The seminal stages of creating a high visibility in the market and expansion of a startup involves a repeated cycle of – Building and improving of the Core Competency, and measuring the effect in KPIs and increase in adoption by customers. Let’s see where and how analytics can be optimally implemented for these scenarios.
Building Core Competency
The starting point for understanding core competences is understanding that businesses need to have something that customers uniquely value if they’re to make good profits. “Me too” businesses (with nothing unique to distinguish them from their competition) are doomed to compete on price: the only thing they can do to make themselves the customer’s top choice is drop price. And as other “me too” businesses do the same, profit margins become thinner and thinner.
This is why there’s such an emphasis on building and selling USPs (Unique Selling Points) in business. If you’re able to offer something uniquely good, customers will want to choose your products and will be willing to pay more for them. Here are three ways to turn analytics insights into actions that make your company more competitive:
Gain control along with visibility of patterns
People often use analytics to understand what’s already happened, but don’t look beyond “what”, to ask “why.” By understanding why certain patterns emerge in your data, you gain greater visibility and control over what’s happening right now.
For example, when you understand why certain factors affect your margins, your sales team is better able to address underperforming products and customers, identify potential revenue opportunities and design more optimal coverage models for your reps.
Put analytics in the right functional areas to drive change
To get results, you need a way to deliver analytical information to sales reps at the product and account level. This empowers reps to negotiate from an informed position and use data to have strategic conversations with customers.
Also, when reps have good access to customer analytics, they’re better able to invest coverage resources in high-quality leads. It helps them to identify opportunities with large value and position sales offers in the context of a dynamic market. For example, if there’s a lot of variability in a commodity and price wars break out, you want to quickly reposition your offer in relation to that dynamic market.
Build an ecosystem
To get the best results from your analytics, you need the ability to monitor what’s happening and use that data to adapt. As you build this process into your company’s DNA, constantly evaluate the criteria you’re using to ensure they stay relevant: Are you looking at the right variables and assessing the marketplace effectively? By maintaining the quality of this information, you’re developing a competitive advantage through pricing and sales sophistication.
Measuring Business Traction
Traction Analytics
Analytics helps your business determine what is working well, and what needs to be improved. We can always go off of a hunch, but the real power comes when we know the hard data behind our marketing or business management efforts, and can make informed decisions that improve our business over and over. Seasoned entrepreneurs know just how important analytics are in growing your business. Without a serious analytics strategy, you are simply relying on hope and luck to grow your company.
In a startup you are constantly under pressure and have way too many distractions. Having a set of metrics that you watch & that you feel are the key drivers of your success helps keep clarity. And the more public you can make your goals for these key metrics the better. Make them widely available inside the company and share your most important goals with your board. Transparency of goals drives performance because it creates both a commitment and a sense of urgency.
If you don’t have a stability goal stated for the company and if you don’t regularly measure how you’re doing against this goal you won’t have your resources focused on the right priorities in the company.
Most companies have some measurements, but I would argue that people often measure the wrong stuff, measure with the wrong precision. The best way is to start by asking yourself at management team level: what are our company objectives and how do we best measure them? Because it can be hard to define or agree company objectives at an early stage I believe most people avoid them.
Customer Acquisition
At the highest level you’ll obviously want to track how many customers you’re adding every month (and for some businesses that have hit scale this is measured on a daily basis). If you can break this down by channel that you’ve acquired them from this is obviously better.
How many additions came through organic SEO? How many through affiliate deals? How many through SEM? Do you have a customer referral program? If so, make sure you can track which leads come from this. Measuring viral adoption is obviously important.
Usually you have a catch-all bucket for “direct” or similar that often came through PR or word-of-mouth.
If you have multiple versions of your product, how many are web vs. mobile? How do the mobile customers break down by device type?
The next step after measuring the customers you’re adding is to add the “cost to acquire” by channel. This is important because it will later tell you whether you have a scalable business or not. In the early phases if you can’t acquire customers cost effectively enough you’ll need to diagnose why and how to fix it.
The Final Question of Scalability
The repeated cycles of Building and re-engineering and Core Competency and Measuring the Market environment effects takes the startups further and further into the final stages of having a scalable model. Like I mentioned at the beginning, there could be n number of directions a startup can head towards, as many as there are number of significant KPIs that need to be improved. But finding the right nerve and chasing the wrong performance indicator is the difference between ending up with a scalable business model and ending up with a marginally incremental model. In this current age of every changing topography of the market with disruptive ideas entering and washing off many hopeful businesses, only having an optimal analytics solution to track their locus can make sure startups sustain and succeed.
Related Posts
AIQRATIONS
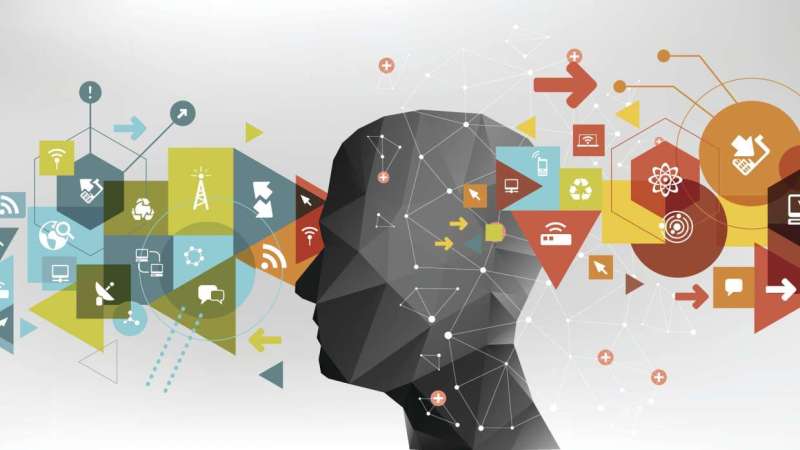
Analytics is all About Talent, not Pedigree
Add Your Heading Text Here
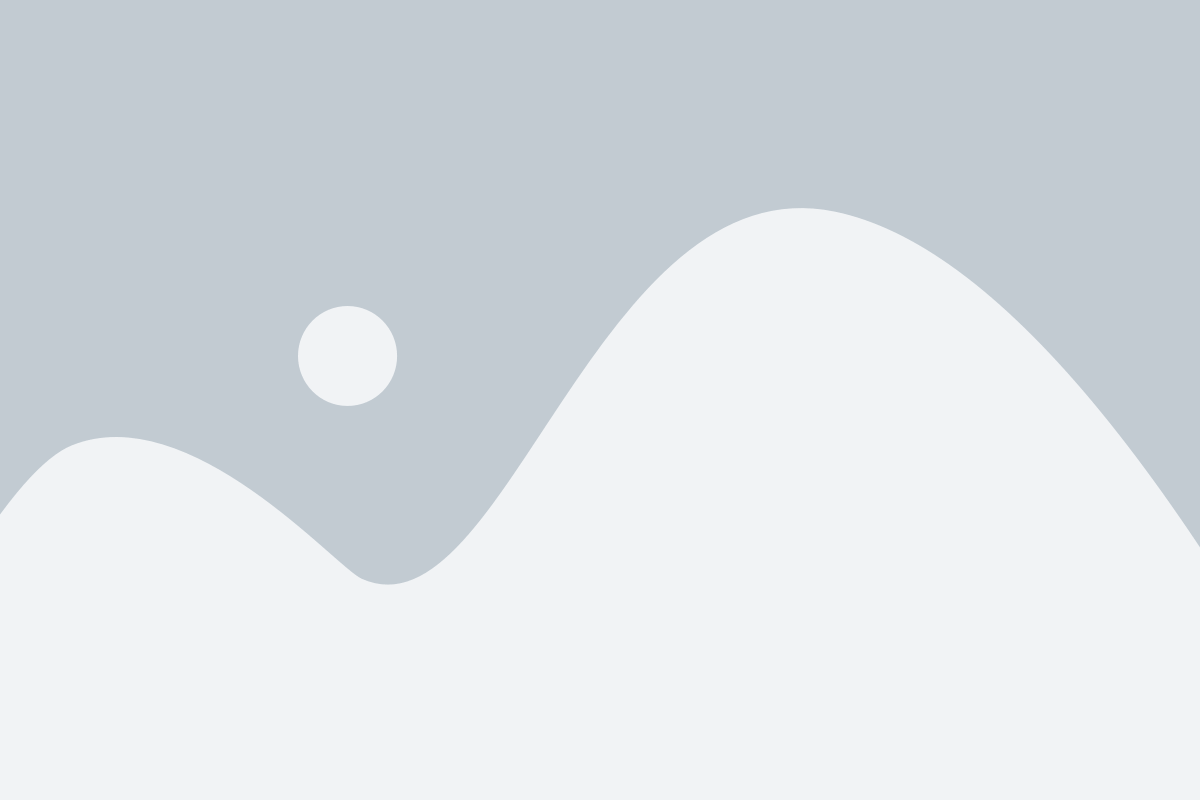
Organizations across the globe today are grappling with a data deluge and with the increasing reliance on mining data to carve out actionable insights and drive strategic imperatives, the relevance of building the right ecosystem of Analytics professionals is becoming commonplace. Qualified analytics professionals are scarce, though in great demand and generally command higher salaries than the industry normal because of their specialized skills.
For most, analytics is still in the realms of software tools and creating highly visual dashboards/reports/charts etc. But there’s definitely more to it than what meets the eye. Analytics has lot more to than just jazzing up data; it can enable fact-based business decisions based on that data. It primarily means working closely with the business stakeholders to uncover gaps in the business and using the knowledge to work with data appropriately, to come up with useful insights and recommendations the organization can focus on, to increase top-line or rationalize costs at a high level.
And many a times, the general perception about great talent directly correlates to the pedigree of an individual. Most organizations, especially in analytics space, are extra careful about their hiring channels when it comes to onboarding Analytics talent. And more often than not, we are generally biased to absorbing talent which has a strong pedigree credentials (academic excellence, b-school or t-school grade or tier et al) and fall prey to such generalized notions about building great teams. Unfortunately, Analytics is a different ball game altogether and successful career in Analytics has more to do with the underlying fundamental behavior of an individual. It’s an interplay of multidisciplinary skills ranging from mathematics, to statistics, computer science, communication and not to mention the business knowhow. Pedigree may be just a guiding beacon to highlight potential but definitely not a key ingredient to governing success. Let me shed some light on what it takes to build a successful career in analytics:
Intellectual Quotient
Successful people in the analytics industry today have that inquisitiveness and high curiosity attitude ingrained in their natural DNA. For any given situation they are presented with, they can think through and formulate the right set of questions, the “why’s” “what’s” & “how’s” which is key to succeeding in a professional setup. Even before jumping to the data analysis piece, it’s crucial to understand the business problem at hand, crafting out the specifics of the probable solution approaches and most importantly questioning the underlying assumptions being undertaken.
Especially ‘big data’ is more about the questions being put forward than the data itself. No data can speak for itself unless appropriately questioned. Success on dealing with ‘big data’ projects requires a thorough understanding of the problem, narrowing down the right questions, getting those answered by SME’s or business experts on right forums, making sure you harness the right amount of data to answer the questions at hand and then eventually communicating the solution to the target audience (which may be clients or the internal stakeholders).
Driven by Numbers
Being accustomed to using mathematical concepts and mathematical tools is commonplace in analytics space. Mathematics & statistics forms the basic foundation here and if for any reason this word strikes fear in your heart, think again! As you progress your career in Analytics and if you aspire to be truly a Data scientist, few additional skills shall be instrumental to your success: Machine learning, statistical modeling, experiment design, Bayesian inference, Supervised learning: decision trees, random forests, logistic regression Or Unsupervised learning: clustering, dimensionality reduction, Optimization: gradient descent and variants etc. The key aspect to note here is that most of these skills are picked up during the job or as special trainings and not directly linked to an individual’s pedigree. The number-crunching attitude forms the basis here and this is something inherent to an individual irrespective of which institute or academic background they hail from.
Ability to see the Holistic picture
Data here is just a means to an end and behind the scenes there’s a larger business problem at hand being dealt with. Unless there’s absolute clarity on what the client is actually intending to solve, you might end up looking at the wrong place or assimilate wrong pieces of information which may not be of any use. At times, the client isn’t quite sure about the problem they intend to seek answers to which may derail the whole exercise. Getting clarity on what’s the root cause driving actions is crucial.
There may be too many variables under consideration at the same time, but being able to see through clearly and importantly, being able to identify the next steps based on the larger intent is imperative. For instance, if the individual is assigned a problem pertaining to pricing analytics in an FMCG industry, it is very important for them to understand the dynamics between marketing, pricing, sales, promotions etc. work in this industry before. If it’s about evaluating the effectiveness of a marketing campaign for an FMCG product, domain knowledge shall help in narrowing down the key 10 or 100 variables that need thorough consideration from amongst the thousands available at disposition.
Again this ties back to our initial premise of inherent inquisitiveness of an individual to get the right set of questions framed and answered before any detailed analysis begins. Asking the “Why” questions at every juncture may help to uncover the latent objectives which client may not be articulate well in certain cases.
Orientation to Detail
Cognitive “attitude” and willingness to search for deeper knowledge about everything is a common strain running across all successful analytics professional. Though a bird’s eye view is good to have to better understand the larger business problem being tackled but at the same time balancing it against the specifics which need further drill-down is crucial. While dealing with voluminous stacks of structured or unstructured data, it’s easy to lose sight of specifics which be of immense value in crafting a solution to the original problem. Having that “hawk’s eye” to suddenly fish out significant patterns which may be of interest to business is a must have. Visualizing data through various plotting methods (box plots, histograms, correlation matrix et al) can help uncover those meaningful nuggets which the business is interested in.
Ability to Interpret within the Realms of Business Context
End of the day, it’s important to realize that numbers won’t speak for themselves unless the right set of tools/techniques/methodologies are employed to present the data in a consumable form. Numerous tools in the industry today have plethora of features to simplify data interpretation but the understanding of which visualization technique is most suited to give you the right picture, given the data in question and business problem at hand is the prowess of a well-acquainted analytics professional; one who knows his toolbox in & out. In some cases histograms may deem fit to understand the distribution of data and at the same time the box plot may get you a better idea of how the majority of data points are spread across the spectrum, or if there are any outliers. Domain expertise & business knowhow can help leapfrog your analysis to a different level altogether, help interpret the results in the business context, assess usefulness of results, bringing out insights which may not be that obvious to common folks.
Communication and Visualization
You may be a champion in your rarefied field, but you may not succeed as an analytics professional unless you can’t communicate the value of your analysis in simplistic terms, a language which the client or business user understands. Communicating the value to business people and asking the right set of questions on what’s important is table stake. Ability to convince that what you’ve done is viable and will deliver business value is something one should be excelling at.
Umpteen times there are disparate pieces of information which a good analytics professional should be able to connect and able to convey a compelling story which makes sense to the target audience. As an analogy, a leading insurer was observing overall dipping sales and post analysis it came to notice that customer service in certain pockets or geographies has dwindled because of inappropriate handling of customers over certain touchpoints. The analytics team was able mine the sales data for pain points, narrow down upon the areas with stagnant or negative sales growth and also uncover pattern between unsatisfactory customer comments over social channels (FB page, twitter handle etc). Survey results again hinted that certain geographies had observed lack of customer empathy as a major factor impeding lead conversion and high attrition. Sales data, social data and survey results in totality were able to narrow down upon those specific areas of concerns mapped to respective geographies, which now the business could pursue to chart out a customer experience roadmap for targeted geographies & remedial measures to mitigate potential bottlenecks identified.
To sum it all, a pedigree can convey so much so about an individual’s ability to succeed in building a thriving analytics career. It’s more about those innate capabilities, domain/analytics experience one garners on the job and regular trainings which forms the secret sauce to a differentiating career trajectory in analytics.
Related Posts
AIQRATIONS
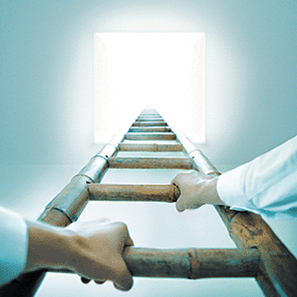
Keeping Your Analytics Talent Motivated & Productive
Add Your Heading Text Here
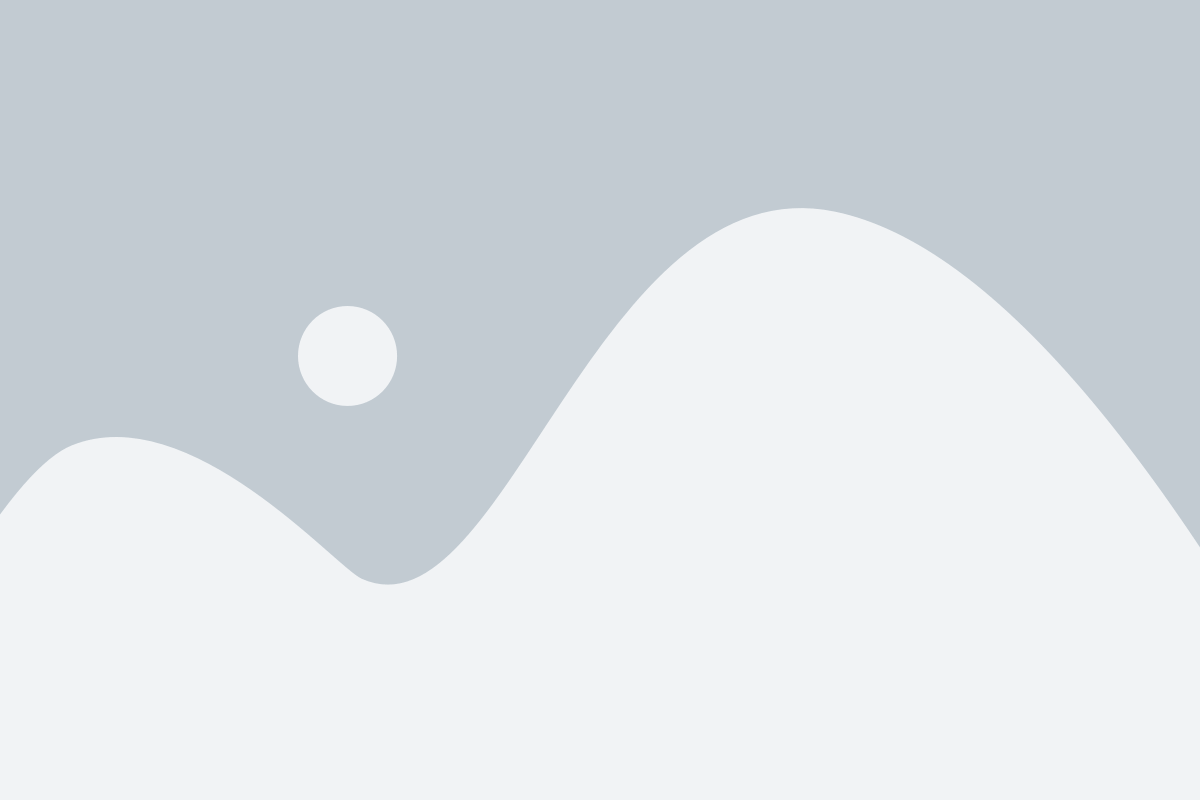
Numerous studies in the past have shown that an engaged workforce significantly out-produces an unmotivated one. And even a greater number of those studies reinforce the common notion that employee creativity is the key to innovation and eventual customer or client satisfaction. To a great degree, for most industries and organizations, talent is one of the most treasured asset and to an extent a key differentiator in the marketplace. Every company needs creative team, decision makers and visionaries, but on a similar note, it’s equally important to have a motivated workforce who can give their all for the cause day-by-day. As clichéd it may sound, Analytics truly is one of the industries where the average churn or attrition rate has typically been on the higher side (as compared to industry average in general) due to great demand for Analytics experts in the industry across the globe and dearth of skills required. I presume all my readers would concur on the perennial challenge of analytics talent crunch most businesses are grappling with today. And even if you find the so-called right analytics resource that align with your skills requirements, you’re bound to chart out engaging career prospects and develop that talent over what could amount to a decade before that person is achieving optimum results for your company.
Analytics is all about working with an extremely talented & creative set of people, who need constant care and attention from their leaders & mentors. And undoubtedly, most businesses yearn to maximize output and increase topline, they need to have their team working as effectively as possible. But how should they go about this? A generally accepted phenomenon that happy workers tend to be productive ones – meaning there are clear benefits to keeping people engaged and motivated.
When it comes to job satisfaction, financial rewards may be lower on the list than most people think and as I reflected in my previous posts. Being happy with the job seems to depend more on the intangibles: feeling part of a team and being valued and appreciated consistently outrank money when employees are polled about job satisfaction. It’s all about keeping employees highly engaged & give them the due respect they deserve. Engaged analytics talent learns, grows, displays high leadership quotient, deliver heightened ROI and significantly decrease your organization’s turnover rate. However, keeping a team that approaches work with vigor and passion intact is easier discussed than executed.
Every other company which employs analytics services or is outsourcing it wants to hire and keep the best of the breed talent – how do you stay a step ahead? Clarity on company’s mission that they are driven to lead themselves is all commonplace and is a sanity in Analytics as with every other industry. And if an employee isn’t engaged creativity is not present! So what truly keeps this evolved species engaged enough?
- Where in the Organization your Analytics Talent sits
First & foremost, as leaders, we must acknowledge that Analytics talent is different from rest of the organizations and placing them rightly within the organization is crucial to ensure they are truly able to create the real impact. Bright minds cannot be chained or siloed or put in a bureaucratic hierarchy, lest they tend to lose their sheen and may end up attriting or be sitting ducks stuck up in the usual business stream where their skills are definitely not put to the best of use. Analytics talent should be led by analytic leaders who know this industry in & out; because they are the ones who understand how this talent needs to be groomed & nurtured, and shielded from the political bureaucracies and the analytics leaders should effectively communicate those differences throughout the organization constantly. Separate HR policies, working culture and operating rhythm is required to give these prodigies a conducive environment to deliver their best. They should acknowledge different processes for them, they should have different technical ladders, different job expectations. It must be thoroughly acknowledged that they have different motivations and is the organization in the capacity to carve out that special niche for thriving Analytics talent. Whether you keep them together in their own close knit group so they can keep their skills sharp with constant interaction with each other, or should they be spread across company’s business units because that is where they must have an impact?
It’s important for your analytics talent to garner complementary skill sets. Obviously, you intend to build a team with eclectic mix of skills instead of having all people who are good at data massaging or modelling or all people who do optimizations or visualizers. Analytics is a broad space and there are umpteen specialties, and piecing together these different puzzle elements is the key to generating impactful business insights. As an analytics leader because you understand the space, you understand these specialties. So to be a good leader of such a talented team, you really have to focus on the individuals on your team and help them succeed.
- A Robust Career Path
Talent always needs a clear future vision on their career trajectory & growth within the organizations with distinctive career paths through career ladders and lattices. A clarity/transparency on roles, career tracks and skill expectations has to be in place to affirm that your analytics professionals have a well thought through career roadmap charted out. Structured capability building, systematic learning and development frameworks could be crucial to ensuring that career architecture plan is laid out appropriately.
- A Rock-solid Training Regime
An effective training and development plan is one of the best ways to convey to your valued employees the commitment and faith you have by investing in them. And, when you consider this investment in the long run with a way higher ROI, the expense of a solid training program suddenly seems measly. With the pace the Analytics industry is maturing, it’s crucial that your talent is in tune with the current needs in the industry, has hands-on experience with the topical tools & technologies and is abreast with the latest and greatest techniques being used today to deliver business impact. Just to substantiate with an example here, R being open-source has tons of pre-built libraries & many more keep getting added to the repository, avoiding duplicity of effort and ensures optimal procedures/techniques are being employed which have proven to be effective & accredited by experts in the wider public analytics community. Smart talent is always hungry for more and it’s imperative for the analytics leaders to keep feeding their talent with all the trending skills/tools and keep their talent’s arsenal up-to-date.
- Empower them
Give your talent the wings of freedom, to innovate, to be creative, basically get out of their way and empower them, give them the requisite tools to deliver. Don’t keep them shielded from the real-world all the time & get them the exposure to be deeply embedded in the C-suite. The senior executives want to make decisions based on data and they trust this talent tremendously. Get them in front of the clients or the C-suite and give them the platform to talk to them directly as to what they are doing. That’s what keeping them there, as they feel that they are in the driver’s seat helping businesses navigate in a highly competitive, relentless environment. Highlight in appropriate forums about their achievements & the impact they have made.
- Business Exposure
Analytics professionals need to be spending a sizable time(between 6-10 years) to industry-specific challenges before they can have the right context to understand the problem well and know exactly know what’s needed & what not to go about finding a solution. This is among the scariest challenges which majority Analytics leaders across the globe are trying to figure out and still poses a big question mark for most on how to get their analytics talent a business bent of mind. Continuous exposure to the business, ongoing domain-specific trainings are hence an important ingredient to their success & keeping them relevant
- Challenging Work Environment
Throw all sorts of challenges and varied projects at your talent. Be it as far away from their comfort zones & let them drown in it. This is what they truly enjoy doing and they love finding their way out from the middle of nowhere. Organize week long hackathons, competitions & give them stringent timelines to deliver. Give them the opportunity to make the impossible possible. The sense of achievement & gratification after working their way out from challenges unimagined in their wildest dreams is what keeps them going. Let them mingle up with the wider Analytics talent beyond the walls of the organization; let them participate in global competitions organized by Kaggle, 5th Elephant, VCCircle, Analytics Vidya etc. Motivate them to join interesting discussions pertaining to Analytics online (twitter, blogs, linkedin communities) and make a contribution, be an expert advisor in the area they specialize in.
- Be the “Employer of Choice”
Value your talent, get them the requisite support and developmental environment from mentors & other experienced professional in your Analytics team, pay them well, recognize their contributions, and give them the opportunity to rise up the career ladder. Start collaborating with academic institutions from where you hire, increase interventions, do guest lectures, seminars, workshops, mentoring sessions, case competitions, “Shadow a Leader” program etc to be constantly engaged with your pipeline talent.
Related Posts
AIQRATIONS
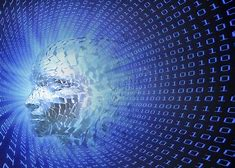
Managing High Performance in Analytics Teams
Add Your Heading Text Here
With the recent massive explosion of data availability, significant leap in computing capabilities, substantial reduction in data storage costs and greater belief of businesses in analytical models has fueled the growth of businesses across the globe and demand of skilled professionals across all levels. However, businesses are demanding high level of performance from their Analytics Service Providers (ASP’s) and are increasingly insisting on translating spend into real tangible, quantifiable outcomes. In the given scheme of things, it is quintessential to measure and improve the performance level of analytics team while simultaneously juggling with the talent crunch of analytics professionals.
As per a recent study a recent survey of 300 IT professionals, conducted by a company called InfoChimps, a mind boggling fifty-five percent of big data analytics projects are abandoned.
And not so surprisingly, the biggest impediment topping the charts was the talent crunch. Almost 80% of the survey participants highlighted that the top two reasons why analytics projects fail are
- The inability of managers to connect the dots around data to come up with relevant actionable insights
- Lack of appropriate business and domain context encircling the data
The Common Analytics “Fingerprint”
Typical analytics skill set is predominantly different from the usual IT ones (more technical or programming-oriented. Primarily there are 3 key roles in analytics: Data Management (includes data assimilation, cleansing, harmonization etc.), Data Modeling or the Data Scientists roles (one who build models) and Data Visualization (the reporting piece). Following skills at an aggregate level are crucial to high-performance of any analytics team but in a nutshell CREATIVITY and CURIOSITY is the most crucial element cutting across all:
- Good with Numbers & Statistics
- Simple linear regression, basic statistics, hypothesis testing, Z- and T-test analysis can get you so much so that you can take those baby steps in Analytics. But to tame the real BIG beast at other times, you definitely need advanced statistics skills when the data becomes voluminous, unstructured or even when you are headed for predictive analysis
- Ability to triangulate numbers & doing back of the envelope calculations is imperative and is being commonly used to evaluate potential candidates looking for venturing into the world of Analytics
- Data management capability
- You shall be headed to nowhere unless your data isn’t clean and enough to perform further analysis,
- Ability to take calculated, educated risks; especially when it comes to taking assumptions, supported by valid arguments and a strong business sense
- Business/Domain Know-how
- Deeper understanding of the data and business problem at hand
- It’s equally important to contextualize outcomes for relevant insights which the business can pursue
- Visualization Capability
- Represent complex data in a simple and easy-to-understand way
- Ability to effectively present findings; intuitive to the decision maker especially when the consumer is a business user
- Psychological Skills
- Being pragmatic, overcoming cognitive dissonance, bias, over-confidence, conflicting thoughts or situations
- Maintaining extremely high sense of quality, standards, and detail orientation
- Storytelling Ability
- Ability to connect the dots, from data to insights in a compelling way, understandable by the business user
- Structured thinking process (especially when the job requires you to deal with unstructured data and complex business situations which may need a well-structured approach)
- Innovation Quotient
- Can the individual see beyond the ordinary! Cognitive “attitude” and willingness to search for deeper knowledge about everything
- Ability to productize ideas e.g. packaging a predictive model as a point solution (targeting specific business challenge with a specific approach to deliver tangible business outcome) OR creating reusable assets out of usual business deliverables which could be easily cross-pollinated and applied to other business problems or even industries
In addition, an analytics professional should have at least some of the following capabilities:
- Strong interpersonal skills, effective oral and written communication and ppt skills
- Agility, take a detour based on inferences being reflected in the data
- Passionate about stumbling upon interesting business problems and inclination to solve them
- Proactively seek clarifications and ask appropriate questions based on what’s shared
How to Evaluate High-performing Analytics Teams
Evaluation is primarily based on which track in Analytics an associate is aligned to (Business, Technology, Delivery, Domain/Industry, Modeling/Data Scientist). Due to the inherent nature of how the Analytics industry works or what clients expect out of us, it eventually boils down to quantifiable business impact, either it’s increased top-line or decreased bottom-line. Eventually it boils to Following are the key pillars of evaluation:
Analytics Pillar
- Data Management Capabilities
- Use of Data treatment techniques
- Quality of assumptions taken
- Quality of Analytics Deliverables
- Output Accuracy & Feasibility
- Visualization ability, intuitiveness
- Analytics SME Quotient
- Domain Knowledge
- Analytics Acumen
- Certifications, Trainings & other Up-skilling/Re-skilling initiatives undergone
- Going the extra mile !
- Identify, conceptualize & execute new solutions, Analytical concepts, techniques and / or prototype tools for a market or cross-pollinating ideas
- Going beyond the call of duty
- Mentorship, Internal trainings etc.
Business Pillar
- Business Knowhow
- Domain understanding and knowing how the industry operates
- Understanding of client’s ecosystem
- Quality of Insights/Recommendations
- Client-centricity, Business acumen
- Quantifiable top-line or bottom-line impact, value creation
- Tangible business outcomes & how it impacted client’s business
- Curiosity to work on most important business problems, ones which add value to the client
That data also shows the No. 1 reason analytics professionals leave their jobs is because they’re bored.
http://www.allanalytics.com/author.asp?section_id=1411&doc_id=266183
Soft Skills
- Effective Presentation & Business Communication
- Collaboration/Team Player
- Coaching & mentoring
- “Winning @ Workplace” attitude, Self-motivated
- Adaptability
- Decision-making skills
- Negotiation skills
- Leadership traits
- Cultural Fit