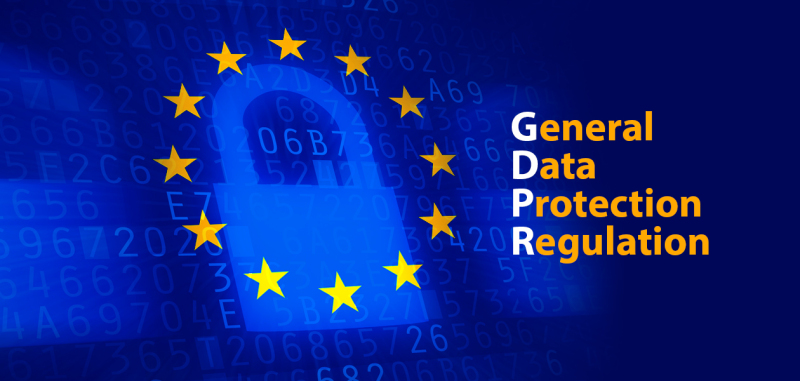
HOW AI CAN ENABLE ENTERPRISES TO IMPLEMENT GENERAL DATA PROTECTION REGULATION (GDPR) POLICIES
Add Your Heading Text Here
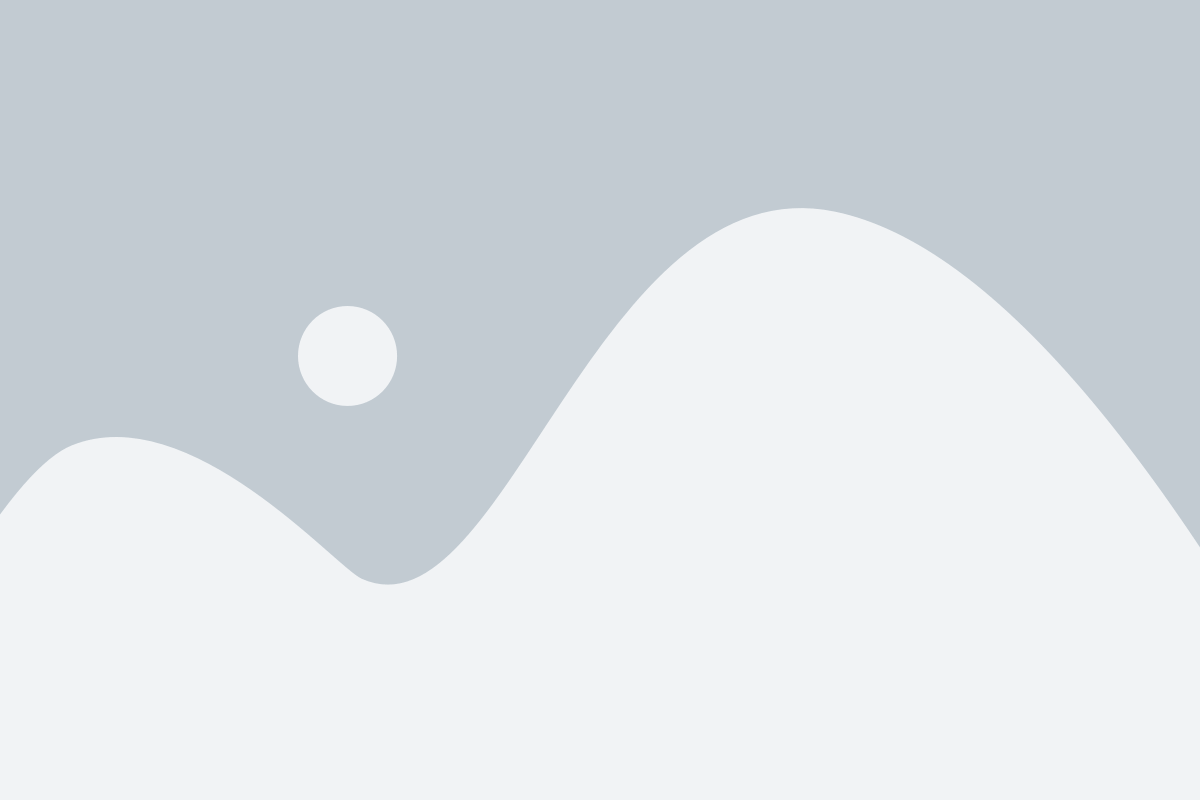
The GDPR General Data Protection Regulation (GDPR), which goes into effect May 25, 2018, requires all companies that collect data on citizens in EU countries to provide a “reasonable” level of protection for personal data. The ramifications for non-compliance are significant, with fines of up to 4% of a firm’s global revenues.
This European Union’s sweeping new data privacy law, is triggering a lot of sleepless nights for CIOs grappling with how to effectively comply with the new regulations and help their organizations avoid potentially hefty penalties.
Will AI be the only answer to the highly regulated GDPR to come?
The bar for GDPR compliance is set high. The regulation broadly interprets what constitutes personal data, covering everything from basic identity information to web data such as IP addresses and cookies, along with more personal artifacts including biometric data, sexual orientation, and even political opinions. The new regulation mandates, among other things, that personal data be erased if deemed unnecessary. Maintaining compliance over such a broad data set is all the more challenging when it is distributed among on-premises data centers, cloud offerings, and business partner systems.
The complexity of the problem has made GDPR a top data protection priority. A PwC survey found that 77% of U.S. organizations plan to spend $1 million or more to meet GDPR requirements. An Ovum report found that two-thirds of U.S. companies believe they will have to modify their global business strategies to accommodate new data privacy laws, and over half are expecting to face fines for non-compliance with the pending GDPR legislation.
This begs the question: Can AI help organizations meet the GDPR’s compliance deadline and avoid penalties? After all, AI is all about handling and deriving insights from vast amounts of data, and GDPR demands that organizations comb through their databases for rafts of personal information that falls under GDPR’s purview. The answer is not only in the affirmative, but there are several significant instances where AI solutions to regulation compliance and governance are already on the high.
For example, Informatica is utilizing advances in artificial intelligence (AI) to help their organizations improve visibility and control over geographically dispersed data. It will provide companies with a holistic, intelligent, and automated approach to governance, for the challenges posed by GDPR.
AI interventions in Data Regulation Compliance and Governance
Data location Discovery and PII Management
It’s essential to learn the location of all customer data in all systems. The first action a company need to do is creating a risk assessment with a guess about what kind of data is likely to be requested how many requests might be expected. Locating all customer data and ensuring GDPR compliant management can be a daunting task, but there are options for automating those processes.
With AI, one can quite easily recognize concepts like ‘person names,’ which is important in this context. To find out how many documents you have that refer to persons (as opposed to companies), or to find out how many documents, social security numbers, phone numbers you have in any one repository, one can combine those analytics, and then begin to understand that the odds are that they have a lot of personal data in this repository, which provides a way to prioritize in the context of GDPR.
For example, M-Files uses Artificial Intelligence to streamline the process of locating and managing PII (personally identifiable information), which often resides in a host of different systems, network folders and other information silos, making it even more challenging for companies to control and protect it.
AI based data cataloguing
A solution that utilizes AI-based machine learning techniques to improve tracking and cataloging data across hybrid deployments can help companies do more accurate reporting while boosting overall efforts to achieve GDPR compliance. By automating the process of discovering and properly recording all types of data and data relationships, organizations can develop a comprehensive view of compliance-related data tucked away in non-traditional sources such as email, social media, and financial transactions – a near-impossible task using traditional solutions and manual processes.
Contextual Engines for Diversely Changing Data Environments
The GDPR changes how companies should look at storage of data. The risk of data getting compromised is increased based on how is stored, in how many different systems it’s stored, how many people are involved in that process, and how long it’s kept. Now that PII on job applications is regulated under GDPR, a company may want to routinely get rid of that data fairly quickly to avoid risk of data breach or audit. There are those kinds of procedural things that organizations will have to really think about.
There are instances where completely removing all data is impossible. You have to retain some data like billing records and there might be conflicting regulations, such as records retention laws. Now, if the citizen asks you to remove that, it’s going to add a lot of complexity to the process, in terms of understanding what data can be removed from the system and what cannot be removed. There will be conflicting situations where this regulation says something, and then you might have an Accounting Act or something in a local or state regulation that says something else.
This requires contextual engines built using AI that can be highly context aware based on the changing circumstances around the data and create a plan of how each data should be stored, managed and purged. This can also provide accurate insights on the levels of encryption and complex data storage techniques that need to be implemented for different data, thereby conserving hardware resources and increasing protection against malignant attacks and data breaches while minimizing risk of GDPR violations.
Working out the Kinks in AI led GDPR
GDPR aims to give EU citizens greater control over their personal data and to hold companies accountable on matters such as data use consent, data anonymization, breach notification, cross-border data transfer, and appointment of data protection officers. For example, organizations will have to honor individuals’ “right to be forgotten,” where applicable — fulfilling requests to delete information and providing proof that it was done. They must also obtain explicit, rather than implied, permission to gather data. And they are required to allow people to see their own data in a commonly readable format.
The system will undoubtedly work those issues out, but, in the meantime, companies should roll up their sleeves and take a thorough, systematic multi-step approach. The multi-step strategy should include:
Data. A comprehensive plan to document and categorize the personal data an organization has, where it came from, and who it is shared with.
Privacy notices. A review of privacy notices to align with new GDPR requirements.
Individuals’ rights. People have enhanced rights, such as the right to be forgotten, and new rights, such as data portability. This demands a check of procedures, processes, and data formats to ensure the new terms can be met.
Legal basis for processing personal data. Companies will need to document the legal basis for processing personal data, in privacy notices and other places.
Consent. Companies should review how they obtain and record consent, as they will be required to document it. Consent must be a positive indication; it cannot be inferred. An audit trail is necessary.
Children. There will be new safeguards for children’s data. Companies will need to establish systems to verify individuals’ ages and gather parental or guardian consent for data-processing activity.
Data breaches. New breach notification rules and new fines will affect many organizations, making it essential to understand how to detect, report, and investigate personal data breaches.
Privacy by design. A privacy by design and data minimization approach will become an express legal requirement. It’s important for organizations to plan how to meet the new terms.
Data protection officers. Organizations may need to designate a data protection officer and figure out who will take responsibility for compliance and how they will position the role.
Will GDPR Aligning Measures Be Necessarily Disruptive?
Many companies are going through significant changes as a result of the new regulations, and the efficiency and speed the AI-powered regulation compliance platform offer can significantly help streamline the entire process if companies want to ensure compliance.
Hence, there are plenty of challenges keeping CIOs up at night. By taking a more intelligence-driven approach to data discovery, preparation, management, and governance, the impending GDPR mandate doesn’t have to be one of them.
Related Posts
AIQRATIONS
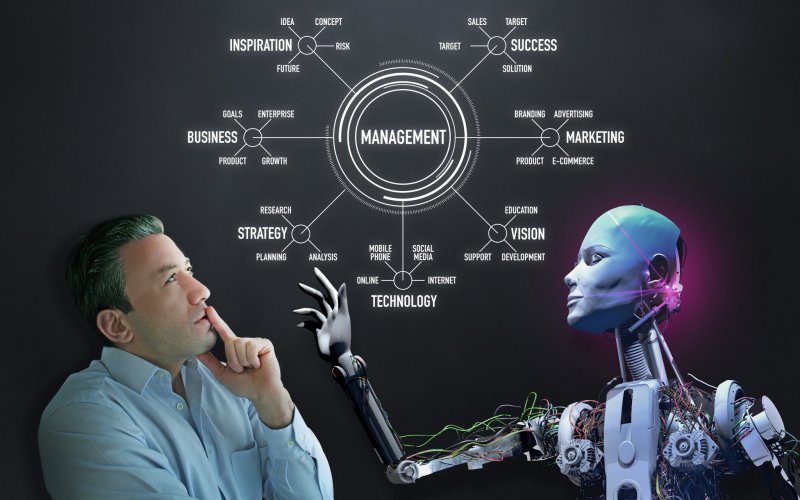
How AI is Challenging Management Theories and Disrupting Conventional Strategic Planning Processes
Add Your Heading Text Here
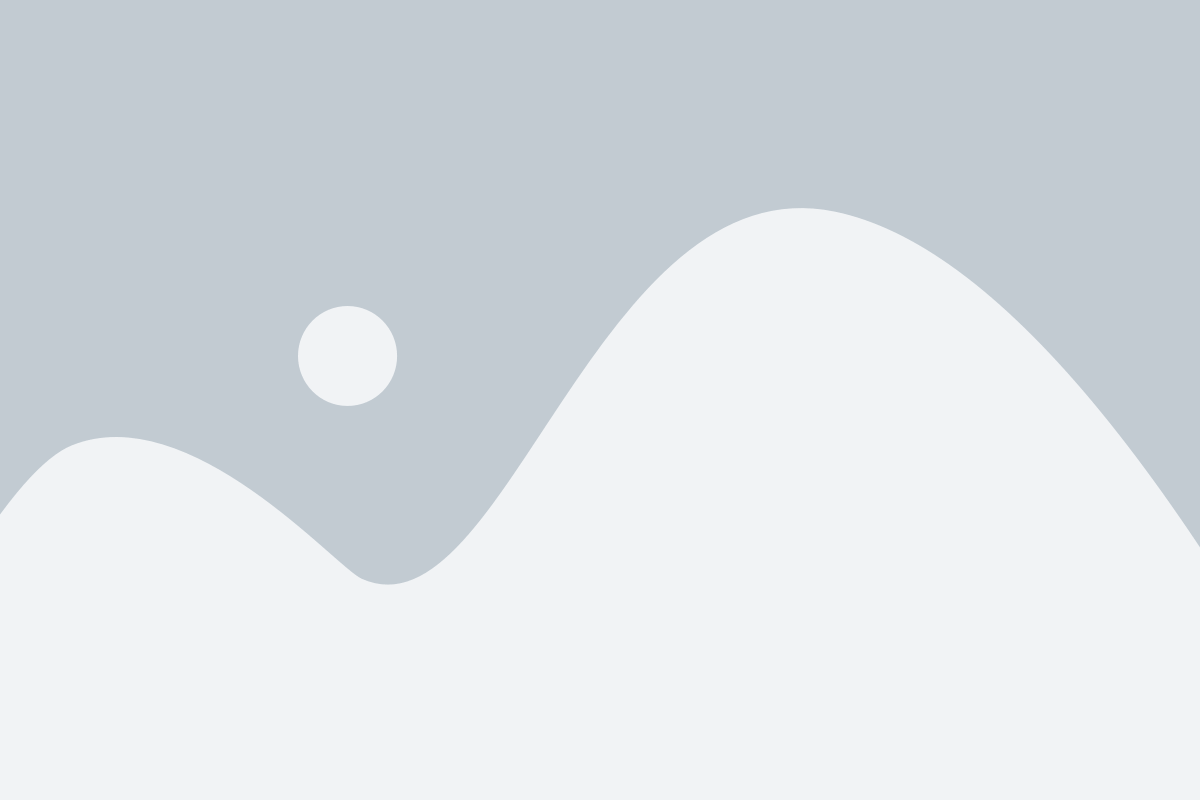
When it comes to AI, businesses think ambitiously. Nearly 85% of executives believe AI will allow their company to obtain or sustain a competitive advantage in the marketplace. Contrastingly, just one in five companies have incorporated AI into their organization and less than 39% of companies have an AI strategy.
Exactly why is AI so disruptive to traditional business models and traditional notions of industry competition? A useful way to analyse the situation is by looking at Porter’s model of the five forces of industry competition and exploring how artificial intelligence is impacting each of the various forces.
According to Michael E. Porter, in one of his landmark books, titled Competitive Strategy, “In any industry, whether it is domestic or international or produces a product or a service, the rules of competition are embodied in five competitive forces: the entry of new competitors, the threat of substitutes, the bargaining power of buyers, the bargaining power of suppliers, and the rivalry among the existing competitors.”
Figure 1: Porter’s Five Forces
Let’s look at each of these five forces and examine the role and impact of AI:
The entry of new competitors
There’s no doubt that AI is changing the nature of competition. Today, it’s not just traditional industry competitors you need to worry about, but new entrants from outside your industry, equipped with new AI based business models and value propositions.
This is often tech giants and startups that have envisioned and built a new business model from the ground up, powered by a new platform ecosystem for AI. They’re leveraging the familiar social, mobile, analytics and cloud technologies, but are often adding in personas and context, intelligent automation, chatbots and the Internet of Things, to further enhance the value proposition of their platform.
Why can new entrants move in so easily? Digital business changes the rules by lowering the traditional barriers to entry. A digitally based business model requires far less capital and can bring large economies of scale for example. Read more about how AI Startups are creating disruptive competition here.
The threat of substitutes
The threat of substitutes is high in many industries since switching costs are low and buyer propensity to substitute is high. For example, In the taxi services, customers can easily switch from traditional models to the new digital app based taxi services, employing AI routines to create differential pricing and intelligent route mapping to increase margin as well as decrease price for the customers. Propensity to switch from the traditional model is high due to consumer wait times for taxis, lack of visibility into taxi location and so on.
In case of BPO industry, the advent of AI has been extremely disruptive, with their clients completely substituting their services with building in-house automation offerings and circumventing their need, sometimes completely. Read more in detail about the disruption of BPO/BPM by AI here.
The bargaining power of buyers
Perhaps the strongest of the five forces impacting industry competition is the bargaining power of buyers since the biggest driver of AI and digital business comes from the needs and expectations of consumers and customers themselves.
This bargaining power lays out a new set of expectations for the AI and digital customer experience and necessitates continual corporate innovation across business models, processes, operations, products and services.
For example, the most used instances of chatbots are through customer support, and now they are heading in the direction of changing the retail sector altogether. The expectations of the Millennials are directing the course of this new technology. This is why chatbots have the burden to exceed the expectations in the retail sector.
Also, in another example, in the customer facing marketing aspect, AI is causing circular rise in customer expectations as rise of expectations, mostly from millennials, has forced the companies to adopt an AI solution to the problem, which further has emboldened their expectations. Amazon, the company that wants to eat everyone’s lunch, is already driving a third of its business from a AI-powered function: its recommended purchases. Read more about how AI is accentuating customer experience to address rising expectations Here.
The bargaining power of suppliers
Suppliers can accelerate or slow down the adoption of a AI based business model based upon how it impacts their own situation. Those pursuing AI models themselves, such as the use of APIs to streamline their ability to form new partnerships and manage existing ones, may help accelerate your own model.
Those who are suppliers to the traditional models, and who question or are still determining their new role in the digital equivalent, may use their bargaining power to slow down or dispute the validity or legality of the new model.
Good examples are the legal and business issues surfacing around the digital-sharing economy (i.e. ride-sharing, room-sharing etc.) where suppliers and other constituents work to ensure the AI based business model and process innovations (like route optimization, or deep customer behaviour analysis using private data) still adhere to established rules, regulations, privacy, security and safety. This is a positive and needed development since, coupled with bargaining power of buyers, it can help to keep new models “honest” in terms of how they operate.
The rivalry among the existing competitors
A lot of organisations are in exploratory stages as they realise that their strategy and customer engagement needs to get smarter. The combination of optimism and fear that clients today have shows that for them it is a competitive necessity to adopt AI and digital technologies.
In 20 years, probably every job will be touched by AI. The technology is growing universally. WhatsApp and Facebook — everything is driven by AI. And what this means is that on the job front, there may be blood. Once AI, ML, and virtual and augmented reality go mainstream, these technologies will prove to be a huge job creator.
But currently, the most competitive space in AI adoption is in the implementation of chatbots across industries and functions. While we might see chatbots starting to appear through the likes of Facebook Messenger and WhatsApp platforms in the coming 12 months, and will be dedicating teams of engineers to train the platforms, rather than relying on the general public. Read more about the competitive atmosphere and underlying need to better customer experience using chatbot here.
How AI will transform Strategic Planning Process
How can managers — from the front lines to the C-suite — thrive in the age of AI? In many ways, the lack of understanding when it comes to AI is due to the variety of ways AI can be implemented as a part of strategic planning for a business. Different industries, or even different companies within the same industry, may use AI in different ways. Ping An, which employs 110 data scientists, has launched about 30 CEO-sponsored AI initiatives that support, in part, its vision – that technology will be the key driver to deliver top-line growth for the company in the years to come. Yet in sharp contrast, elsewhere in the insurance industry, other large companies’ AI initiatives are limited to experimenting with chatbots. Obviously, integrating AI is not going to be simple. There will be a massive learning curve for organizations before they’re able to start implementing AI effectively. But the core shift in strategic planning will happen in the following ways:
AI will take over almost all Administrative Tasks
According to an HBR report, managers across all levels spend more than half of their time on administrative coordination and control tasks. (For instance, a typical store manager or a lead nurse at a nursing home must constantly juggle shift schedules because of staff members’ illnesses, vacations, or sudden departures.) These are the very responsibilities that the same managers expect to see AI affecting the most. And they are correct: AI will automate many of these tasks.
Figure 2: Source – HBR (How Artificial Intelligence Will Redefine Management)
For example, in case of report writing The Associated Press expanded its quarterly earnings reporting from approximately 300 stories to 4,400 with the help of AI-powered software robots. In doing so, technology freed up journalists to conduct more investigative and interpretive reporting.
Strategy Managers will focus more on Judgement-oriented Creative Thinking Work
The human factor, which AI still cannot permeate – the application of experience, expertise and a capacity to improvise, to critical business decisions and practices – need to be focused on by strategy managers. Many decisions require insight beyond what artificial intelligence can squeeze from data alone. Managers use their knowledge of organizational history and culture, as well as empathy and ethical reflection. Managers we surveyed have a sense of a shift in this direction and identify the creative thinking skills and experimentation, data analysis and interpretation, and strategy development as three of the four top new skills that will be required to succeed in the future. And since the potential of machine learning is the ability to help make decisions, the AI technology would be better placed as an assisting hand than administrative mind.
Think of AI not as Machines, but Colleagues
Managers who view AI as a kind of colleague will recognize that there’s no need to “race against a machine.” While human judgment is unlikely to be automated, intelligent machines can add enormously to this type of work, assisting in decision support and data-driven simulations as well as search and discovery activities. In fact, 78% of the surveyed managers believe that they will trust the advice of intelligent systems in making business decisions in the future.
Not only will AI augment managers’ work, but it will also enable managers to interact with intelligent machines in collegial ways, through conversation or other intuitive interfaces.
For example, Kensho Technologies, a provider of next-generation investment analytics, allows investment managers to ask investment-related questions in plain English, such as, “What sectors and industries perform best three months before and after a rate hike?” and get answers within minutes.
Design Thinking needs to be adopted both ways – Managers & AI
While managers’ own creative abilities are vital, perhaps even more important is their ability to harness others’ creativity. Manager-designers bring together diverse ideas into integrated, workable, and appealing solutions. Creative thinking and experimentation is a key skill area that managers need to learn to stay successful as AI increasingly takes over administrative work. ‘Collaborative Creativity’ is the operating word here.
But this doesn’t mean that design thinking necessarily need to become a forte exclusive to managers. Even though AI engines may not have reached radical thinking and improvisation as humans, AI algorithms should be viewed as cognitive tools capable of augmenting human capabilities and integrated into systems designed to go with the grain of human—and organizational—psychology. This calls for Divergence from More Powerful Intelligence To More Creative Intelligence in AI.
To make design thinking meaningful for consumers, companies can benefit from carefully selecting use cases and the information they feed into AI technologies. In determining which available data is likely to generate desired results, enterprises can start by focusing on their individual problems and business cases, create cognitive centres of excellence, adopt common platforms to digest and analyze data, enforce strong data governance practices, and crowdsource ideas from employees and customers alike. Read more about Design Thinking in AI here.
Create New Business Processes manifested from Augmented Working Strategy
Simply put, my recommendation is to adopt AI in order to automate administration and to augment but not replace human judgment. If the current shortage of analytical talent is any indication, organizations can ill afford to wait and see whether their managers are equipped to work alongside AI. This calls for change in business processes, and the way they are implemented itself. To navigate in an uncertain future, managers must explore early, and experiment with AI and apply their insights to the next cycle of experiments.
AI augmentation will drive the adoption of new key performance indicators. AI will bring new criteria for success: collaboration capabilities, information sharing, experimentation, learning and decision-making effectiveness, and the ability to reach beyond the organization for insights.
Accordingly, organizations need to develop training and recruitment strategies for creativity, collaboration, empathy, and judgment skills. Leaders should develop a diverse workforce and team of managers that balance experience with creative and social intelligence — each side complementing the other to support sound collective judgment.
Final Word
While oncoming AI disruptions in Management Principles and Strategic Planning space won’t arrive all at once, the pace of development is faster and the implications more far-reaching than most executives and managers realize. Those managers capable of assessing what the workforce of the future will look like can prepare themselves for the arrival of AI.
Related Posts
AIQRATIONS
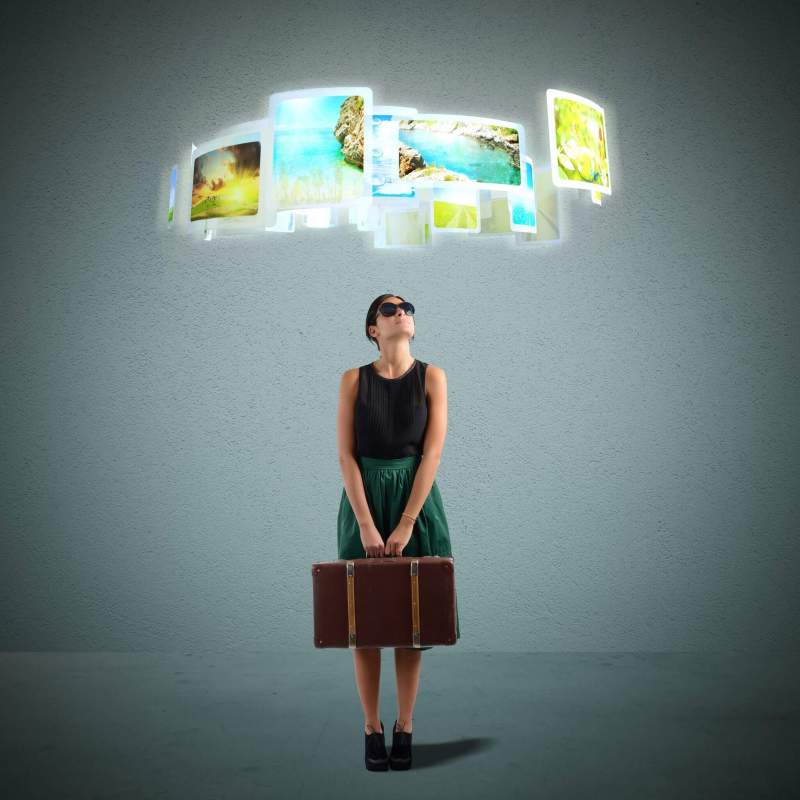
Travel & Hospitality Industry Transformation: Served Fast with AI
Add Your Heading Text Here
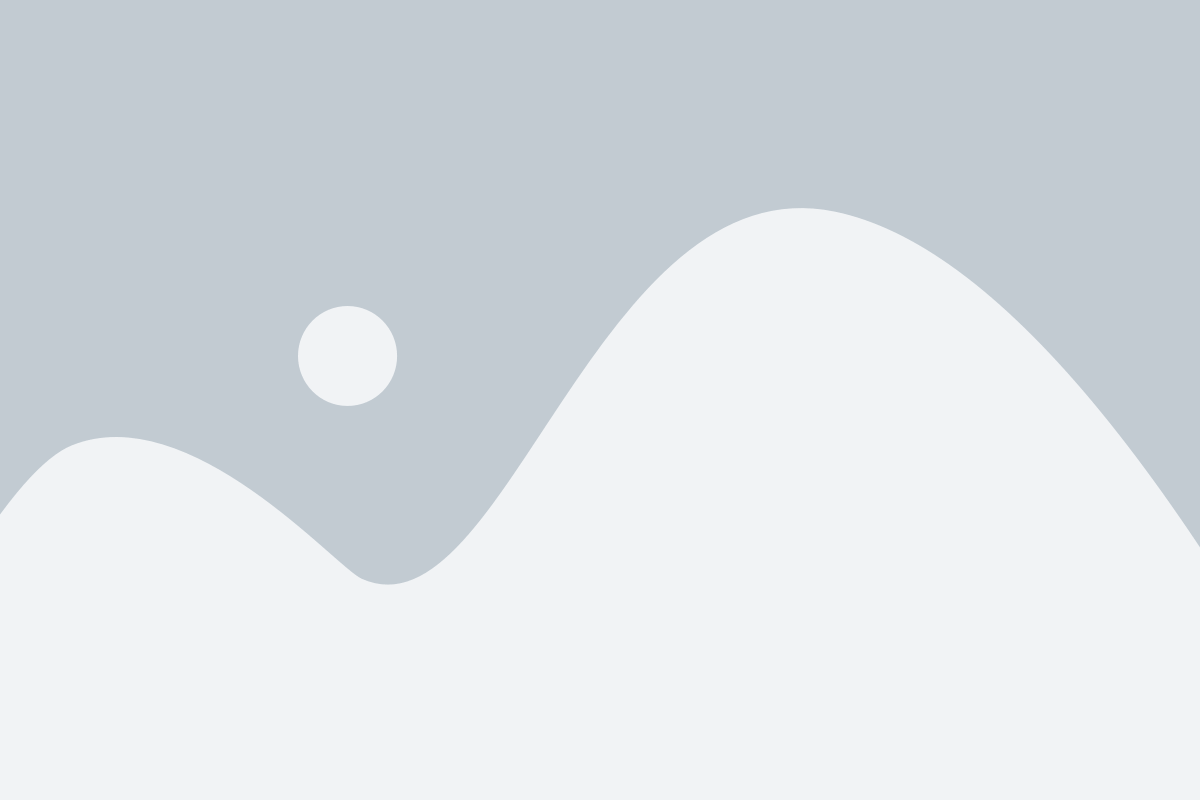
Over the years, the influence of AI has spread to almost every aspect of the travel and the hospitality industry. 30% of hospitality businesses use artificial intelligence to augment at least one of their primary sales processes and most customer personalization is done using AI.
30% of hospitality businesses use artificial intelligence to augment at least one of their primary sales processes.
The sudden popularity of Artificial Intelligence in the Travel industry can be credited to the humongous amount of data being generated today. Artificial Intelligence helps analyse unstructured data, brings value in partnership with Big Data and turns it into meaningful and actionable insights. Trends, outliers and patterns are figured out using this smart data which helps in guiding a Travel company to make informed decisions. The discounts, schemes, tour packages, seasons to target and people to target are formulated using this data. Usually, surveys and social media sensing are done to know customer’s insights and behaviour.
Let’s look at how AI has influenced each aspect of the business
Bleisure – Personalized Experience
There are always a few who are up for a new challenge and adopt to new technology. Many hotels have started using an AI concierge. One great example of an AI concierge is Hilton World wide’s Connie, who is the first true AI-powered concierge bot.
Connie stands at 2 feet high and guests can interact with it during their check-in. Connie is powered by IBM’s Watson AI and uses WayBlazer travel database. It can provide information to guests on local attractions, places to visit, etc. Being an AI, it can learn and adapt and respond to each guest.
In the Travel business, Mezi, using Artificial Intelligence and Natural Language Processing, provides a personalized experience to Business travellers who usually are strapped for time. It talks about bringing on a concept of bleisure (business+leisure) to address the needs of the workforce. A research done by them states that 84% of business travellers return feeling frustrated, burnt out and unmotivated. The kind of tedious and monotonous planning that goes into the travel booking could be the reason for it. With AI and NLP, Mezi collects preferences and generates suggestions so that a customized and streamlined experience is given and the issues faced by them are addressed properly.
Increased Productivity – Instant Connectivity
Increased productivity now begins with the search for the hotel, and technology has paved its way for the customer to access more data than ever before. Booking sites like Lola (www.lola.com) who provide on-demand travel services have developed technologies that can not only instantly connects people to their team of travel agents who find and book flights, hotels, and cars but have been able to empower their agents with tremendous technology to make research and decisions an easy process.
Intelligent Travel Assistants – Chatbots
Chatbot technology is another big strand of AI, and unsurprisingly, many travel brands have already launched their own versions in the past year or so. Skyscanner is just one example, creating a bot to help consumers find flights in Facebook Messenger. Users can also use it to request travel recommendations and random suggestions. Unlike ecommerce or retail brands using chatbots, which can appear gimmicky, there is an argument that examples like Skyscanner are much more relevant and useful for everyday consumers.
After all, with the arrival of many more travel search websites, consumers are being overwhelmed by choice – not necessarily helped by it. Consequently, a bot like Skyscanner is able to cut through the noise, connecting with consumers in their own time and in the social media spaces they most frequently visit.
Recently, Aeromexico started using Facebook Messenger chatbot to answer the very generic questions by the customers. The main idea was to cater to 80% of questions which are usually the repeated ones and about common topics. Thus, to avoid a repetitive process, artificial intelligence is of great application. Airlines hugely benefit from this. KLM Royal Dutch Airlines uses artificial intelligence to respond to the queries of customers on twitter and facebook. It uses an algorithm from a company called Digital Genius which is trained on 60,000 questions and answers. Not only this, Deutsche Lufthansa’s bot Mildred can help in searching the cheapest fares.
Discovery & Data Analysis – Intelligent Recommendations
International hotel search engine Trivago acquired Hamburg, Germany machine learning startup, Tripl, as it ramps up its product with recommendation and personalization technology, giving them a customer-centric approach.
The AI algorithm gives tailored travel recommendations by identifying trends in users’ social media activities and comparing it with in-app data of like-minded users. With its launch in July 2015, users could sign up only through Facebook, potentially sharing oodles of profile information such as friends, relationship status, hometown, and birthday.
Persona based travel recommendations, use of customised pictures and text are now gaining ground to entice travellers to book your hotels. KePSLA’s travel recommendation platform is one of the first in the world to do this by using deep learning and NLP solutions.
With 81% of people believing that robots would be better at handling data than humans, there is also a certain level of confidence in this area from consumers.
Knowing your Travellers – Deep Customer Behaviour
Dorchester Collection is another hotel chain to make use of AI. However, instead of using it to provide a front-of-house service, it has adopted it to interpret and analyse customer behaviour in the form of raw data.
Partnering with technology company, RicheyTX, Dorchester Collection has helped to develop an AI platform called Metis.
Delving into swathes of customer feedback such as surveys and reviews (which would take an inordinate amount of time to manually find and analyse) it is able to measure performance and instantly discover what really matters to guests.
For example, Metis helped Dorchester to discover that breakfast it not merely an expectation – but something guests place huge importance on. As a result, the hotels began to think about how they could enhance and personalise the breakfast experience.
Flight Fare and Hotel Price Forecasting
Flight fares and hotel prices are ever-changing and vary greatly depending on the provider. No one has time to track all those changes manually. Thus, smart tools which monitor and send out timely alerts with hot deals are currently in high demand in the travel industry.
The AltexSoft data science team has built such an innovative fare predictor tool for one of their clients, a global online travel agency, Fareboom.com. Working on its core product, a digital travel booking website, they could access and collect historical data about millions of fare searches going back several years. Armed with such information, they created a self-learning algorithm, capable of predicting the future price movements based on a number of factors, such as seasonal trends, demand growth, airlines special offers, and deals.
With the average confidence rate at 75 percent, the tool can make short-term (several days) as well as long-term (a couple of months) forecasts.
Optimized Disruption Management
While the previous case is focused mostly on planning trips and helping users navigate most common issues while traveling, automated disruption management is somewhat different. It aims at resolving actual problems a traveler might face on his/her way to a destination point.
Mostly applied to business and corporate travel, disruption management is always a time-sensitive task, requiring instant response. While the chances to get impacted by a storm or a volcano eruption are very small, the risk of a travel disruption is still quite high: there are thousands of delays and several hundreds of canceled flights every day.
With the recent advances in technology, it became possible to predict such disruptions and efficiently mitigate the loss for both the traveler and the carrier. The 4site tool, built by Cornerstone Information Systems, aims at enhancing the efficiency of enterprise travel. The product caters to travelers, travel management companies, and enterprise clients, providing a unique set of features for real-time travel disruption management.
For example, if there is a heavy snowfall at your destination point and all flights are redirected to another airport, a smart assistant can check for available hotels there or book a transfer from your actual place of arrival to your initial destination.
Not only passengers are affected by travel disruptions; airlines bear significant losses every time a flight is canceled or delayed. Thus, Amadeus, one of the leading global distribution systems (GDS), has introduced Schedule Recovery system, aiming to help airlines mitigate the risks of travel disruption. The tool helps airlines instantly address and efficiently handle any threats and disruptions in their operations.
Future potential
So, we’ve already seen the travel industry capitalise on AI to a certain extent. But how will it evolve in the coming year?
Business travel
Undoubtedly, we’ll see many more brands using AI for data analysis as well as launching their own chatbots. There’s already been a suggestion that Expedia is next in line, but it is reportedly set to focus on business travel rather than holidaymakers. Due to the greater need for structure and less of a desire for discovery, it certainly makes sense that artificial intelligence would be more suited to business travellers.
Specifically, it could help to simplify the booking process for companies, as well as help eliminate discrepancies around employee expenses. With reducing costs and improving efficiency two of the biggest benefits, AI could start to infiltrate business travel even more so than leisure in the next 12 months.
Voice technology
Lastly, we can expect to see greater development in voice-activated technology.
With voice-activated search, the experience of researching and booking travel has the potential to become quicker and easier than ever before. Similarly, as Amazon Echo and Google Home start to become commonplace, more hotels could start to experiment with speech recognition to ramp up customer service.
This means devices and bots could become the norm for brands in the travel industry.
Related Posts
AIQRATIONS
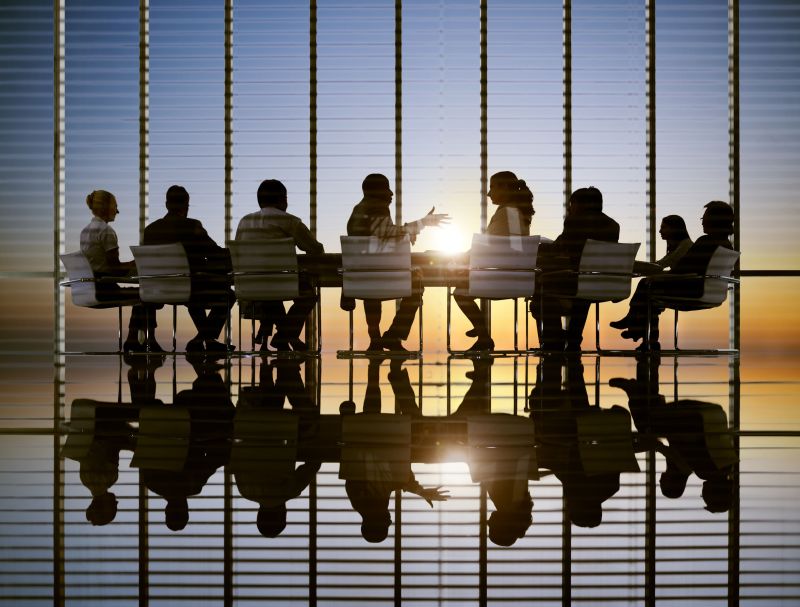
How CXOs are Leveraging AI to Pivot Business Strategy and Operational Models
Add Your Heading Text Here
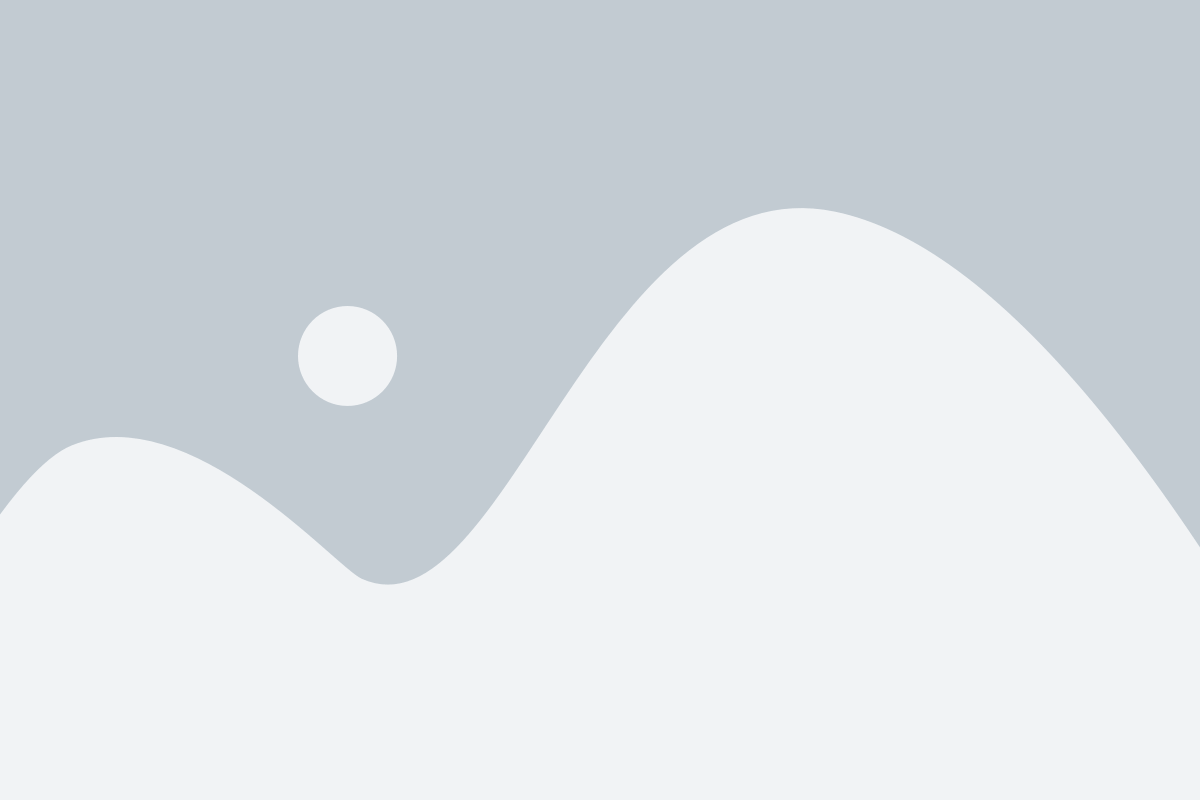
AlphaGo caused a stir by defeating 18-time world champion Lee Sedol in Go, a game thought to be impenetrable by AI for another 10 years. AlphaGo’s success is emblematic of a broader trend: An explosion of data and advances in algorithms have made technology smarter than ever before. Machines can now carry out tasks ranging from recommending movies to diagnosing cancer — independently of, and in many cases better than, humans. In addition to executing well-defined tasks, technology is starting to address broader, more ambiguous problems. It’s not implausible to imagine that one day a “strategist in a box” could autonomously develop and execute a business strategy. We’ve spoken to CXOs and leaders who express such a vision — and companies such as Amazon and Alibaba are already beginning to make it a reality.
Business Processes – Increasing productivity by reducing disruptions
AI algorithms are not natively “intelligent.” They learn inductively by analyzing data. While most leaders are investing in AI talent and have built robust information infrastructures,
As Airbus started to ramp up production of its new A350 aircraft, the company faced a multibillion-euro challenge. The plan was to increase the production rate of that aircraft faster than ever before. To do that, they needed to address issues like responding quickly to disruptions in the factory. Because they will happen. Airbus turned to artificial intelligence. It combined data from past production programs, continuing input from the A350 program, fuzzy matching, and a self-learning algorithm to identify patterns in production problems.
AI led to rectification of about 70% of the production disruptions for Airbus, by matching to solutions used previously — in near real time.
Just as it is enabling speed and efficiency at Airbus, AI capabilities are leading directly to new, better processes and results at other pioneering organizations. Other large companies, such as BP, Infosys, Wells Fargo, and Ping An Insurance, are already solving important business problems with AI. Many others, however, have yet to get started.
Integrated Strategy Machine – The Implementation Scope Augmented AI
The integrated strategy machine is the AI analog of what new factory designs were for electricity. In other words, the increasing intelligence of machines could be wasted unless businesses reshape the way they develop and execute their strategies. No matter how advanced technology is, it needs human partners to enhance competitive advantage. It must be embedded in what we call the integrated strategy machine. An integrated strategy machine is the collection of resources, both technological and human, that act in concert to develop and execute business strategies. It comprises a range of conceptual and analytical operations, including problem definition, signal processing, pattern recognition, abstraction and conceptualization, analysis, and prediction. One of its critical functions is reframing, which is repeatedly redefining the problem to enable deeper insights.
Amazon represents the state-of-the-art in deploying an integrated strategy machine. It has at least 21 data science systems, which include several supply chain optimization systems, an inventory forecasting system, a sales forecasting system, a profit optimization system, a recommendation engine, and many others. These systems are closely intertwined with each other and with human strategists to create an integrated, well-oiled machine. If the sales forecasting system detects that the popularity of an item is increasing, it starts a cascade of changes throughout the system: The inventory forecast is updated, causing the supply chain system to optimize inventory across its warehouses; the recommendation engine pushes the item more, causing sales forecasts to increase; the profit optimization system adjusts pricing, again updating the sales forecast.
Manufacturing Operations – An AI assistant on the floor
CXOs at industrial companies expect the largest effect in operations and manufacturing. BP plc, for example, augments human skills with AI in order to improve operations in the field. They have something called the BP well advisor that takes all of the data that’s coming off of the drilling systems and creates advice for the engineers to adjust their drilling parameters to remain in the optimum zone and alerts them to potential operational upsets and risks down the road. They are also trying to automate root-cause failure analysis to where the system trains itself over time and it has the intelligence to rapidly assess and move from description to prediction to prescription.
Customer-facing activities – near real time scoring
Ping An Insurance Co. of China Ltd., the second-largest insurer in China, with a market capitalization of $120 billion, is improving customer service across its insurance and financial services portfolio with AI. For example, it now offers an online loan in three minutes, thanks in part to a customer scoring tool that uses an internally developed AI-based face-recognition capability that is more accurate than humans. The tool has verified more than 300 million faces in various uses and now complements Ping An’s cognitive AI capabilities including voice and imaging recognition.
AI Strategy for Different Operational Models
To make the most of this technology implementation in various business operations in your enterprise, consider the three main ways that businesses can or will use AI:
Assisted intelligence
Now widely available, improves what people and organizations are already doing. For example, Google’s Gmail sorts incoming email into “Primary,” “Social,” and “Promotion” default tabs. The algorithm, trained with data from millions of other users’ emails, makes people more efficient without changing the way they use email or altering the value it provides. Assisted intelligence tends to involve clearly defined, rules-based, repeatable tasks.
Assisted intelligence apps often involve computer models of complex realities that allow businesses to test decisions with less risk. For example, one auto manufacturer has developed a simulation of consumer behavior, incorporating data about the types of trips people make, the ways those affect supply and demand for motor vehicles, and the variations in those patterns for different city topologies, marketing approaches, and vehicle price ranges. The model spells out more than 200,000 variations for the automaker to consider and simulates the potential success of any tested variation, thus assisting in the design of car launches. As the automaker introduces new cars and the simulator incorporates the data on outcomes from each launch, the model’s predictions will become ever more accurate.
Augmented intelligence
Augmented Intelligence, emerging today, enables organizations and people to do things they couldn’t otherwise do. Unlike assisted intelligence, it fundamentally alters the nature of the task, and business models change accordingly.
For example, Netflix uses machine learning algorithms to do something media has never done before: suggest choices customers would probably not have found themselves, based not just on the customer’s patterns of behavior, but on those of the audience at large. A Netflix user, unlike a cable TV pay-per-view customer, can easily switch from one premium video to another without penalty, after just a few minutes. This gives consumers more control over their time. They use it to choose videos more tailored to the way they feel at any given moment. Every time that happens, the system records that observation and adjusts its recommendation list — and it enables Netflix to tailor its next round of videos to user preferences more accurately. This leads to reduced costs and higher profits per movie, and a more enthusiastic audience, which then enables more investments in personalization (and AI).
Autonomous intelligence
Being developed for the future, Autonomous Intelligence creates and deploys machines that act on their own. Very few autonomous intelligence systems — systems that make decisions without direct human involvement or oversight — are in widespread use today. Early examples include automated trading in the stock market (about 75 percent of Nasdaq trading is conducted autonomously) and facial recognition. In some circumstances, algorithms are better than people at identifying other people. Other early examples include robots that dispose of bombs, gather deep-sea data, maintain space stations, and perform other tasks inherently unsafe for people.
As you contemplate the introduction of artificial intelligence, articulate what mix of the three approaches works best for you.
- Are you primarily interested in upgrading your existing processes, reducing costs, and improving productivity? If so, then start with assisted intelligence, probably with a small group of services from a cloud-based provider.
- Do you seek to build your business around something new — responsive and self-driven products, or services and experiences that incorporate AI? Then pursue an augmented intelligence approach, probably with more complex AI applications resident on the cloud.
- Are you developing a genuinely new technology? Most companies will be better off primarily using someone else’s AI platforms, but if you can justify building your own, you may become one of the leaders in your market.
The transition among these forms of AI is not clean-cut; they sit on a continuum. In developing their own AI strategy, many companies begin somewhere between assisted and augmented, while expecting to move toward autonomous eventually.
Related Posts
AIQRATIONS

The most strategic agenda in CEO’s mind – Is the enterprise AI ready ?
Add Your Heading Text Here
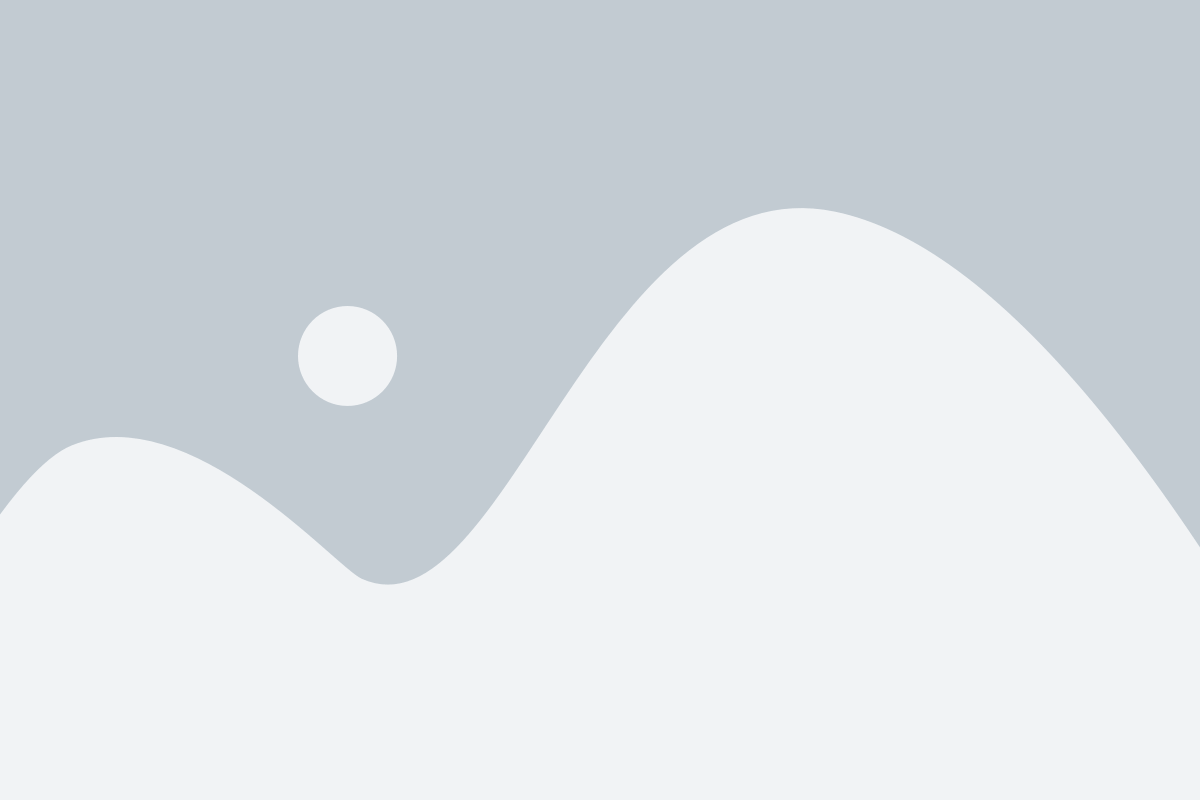
For the larger mass of professionals, the words “artificial intelligence,” or AI, often conjure up images of robots, the sorts of robots that might someday take their jobs. But at the enterprise level, AI means something different. It has enormous power and potential: it can disrupt, innovate, enhance, and in many cases totally transform a business. Forrester Research predicts a 300% increase in AI investment in 2017 from last year, and IDC estimates that the AI market will surge from about $8 billion in 2016 to more than $47 billion in 2020. There’s solid proof that the investment can pay off—if CEO’s can adopt the right strategy. Organizations that deploy AI strategically enjoy advantages ranging from cost reductions and higher productivity to top-line benefits such as increasing revenue and profits, richer customer experiences, and working-capital optimization. The survey shows that the companies winning at AI are also more likely to enjoy broader business success.
So How to make your Enterprise AI Ready?
just one quarter of organizations say they are getting significant impact from it. But these leading businesses have taken clear, practical steps to get the results they want. Here are five of their key strategies:
- Core AI Resource Assimilation using Funding or Acquisition
- Gain senior management support
- Focus on process, not function
- Reskill your teams and foster a learning culture
- Shift from system-of-record to system-of-intelligence apps, platforms
- Encourage innovation
Core AI Resource Assimilation using Funding or Acquisition
As per insights from Forbes and Cowen & Company, 81% of IT leaders are currently investing in or planning to invest in Artificial Intelligence (AI). Based on the study, CIOs have a new mandate to integrate AI into IT technology stacks. The study found that 43% are evaluating and doing a Proof of Concept (POC) and 38% are already live and planning to invest more. The following graphic provides an overview of company readiness for machine learning and AI projects.
Through 2020, organization using cognitive ergonomics and system design in new AI projects will achieve long term success four times more often than others
– Gartner
With $1.7 billion invested in AI startups in Q1 2017 alone, and the exponential efficiencies created by this sort of technology, this evolution will happen quicker than many business leaders are prepared for. If you aren’t sure where to start, don’t worry – you’re not alone. The good news is that you still have options:
- You can acquire, or invest in, an innovative technology company applying AI/ML in your market, and gain access to new product and AI/ML talent.
- You can seek to invest as a limited partner in a few early stage AI focused VC firms, gaining immediate access and exposure to vetted early stage innovation, a community of experts and market trends.
- You can set out to build an AI-focused division to optimize your internal processes using AI, and map out how AI can be integrated into your future products. But recruiting in the space is painful and you will need a strong vision and sense of purpose to attract and retain the best.
- You can use outside development-for-hire shops like new entrant Element.ai, who raised over $100M last June, or more traditional consulting firms, to fill the gaps or get the ball rolling.
Process Based Focus Rather than Function Based
One critical element differentiates AI success from AI failure: strategy. AI cannot be implemented piecemeal. It must be part of the organization’s overall business plan, along with aligned resources, structures, and processes. How a company prepares its corporate culture for this transformation is vital to its long-term success. That includes preparing people by having senior management that understands the benefits of AI; fostering the right skills, talent, and training; managing change; and creating an environment with processes that welcome innovation before, during, and after the transition.
The challenge of AI isn’t just the automation of processes—it’s about the up-front process design and governance you put in to manage the automated enterprise. The ability to trace the reasoning path AI technologies use to make decisions is important. This visibility is crucial in financial services, where auditors and regulators require firms to understand the source of a machine’s decision.
Taking down Resistance to change of Upper Management
One of the biggest challenges to digital transformation is resistance to change. The survey found that upper management is the group most strongly opposed to AI implementation. C-suite executives may not have warmed up to it either. There is such a lack of understanding about the benefits which the technology can bring that the C-suite or board members simply don’t want to invest in it, nor do they understand that failing to do so will adversely affect their bottom line and even cause them to go out of business. Regulatory uncertainty about AI, rough experiences with previous technological innovation, and a defensive posture to better protect shareholders, not stakeholders, may be contributing factors.
Pursuing AI without senior management support is difficult. Here the numbers again speak for themselves. The majority of leading AI companies (68%) strongly agree that their senior management understands the benefits AI offers. By contrast, only 7% of laggard firms agree with this view. Curiously, though, the leading group still cites the lack of senior management vision as one of the top two barriers to the adoption of AI.
Reskilling Teams and HR Redeployment
HR and corporate management will need to figure out new jobs for people to do. Redeployment is going to be a huge factor that the better companies will learn how to handle. The question of job losses is a sensitive one, most often played up in news headlines. But AI also creates numerous job opportunities in new and different areas, often enabling employees to learn higher-level skills. In healthcare for example, physicians are learning to work with AI-powered diagnostic tools to avoid mistakes and make better decisions. The question is who owns the data. If HR retains ownership of people data, it continues to have a role. If it loses that, all bets are off.
HR’s other role in an AI future will be to help make decisions about if and when to automate, whether to reskill or redeploy the human workforce, and the moral and ethical aspects of such decisions. Companies which are experimenting with bots and AI with no thought for the implications need to realize that HR should be central to the governance of AI automation.
Given the potential of AI to complement human intelligence, it is vital for top-level executives to be educated about reskilling possibilities. It is in the best interest of companies to train workers who are being moved from jobs that are automated by AI to jobs in which their work is augmented by AI.
The Dawn of System-of-Intelligence Apps & Platforms
Cowen predicts that an Intelligent App Stack will gain rapid adoption in enterprises as IT departments shift from system-of-record to system-of-intelligence apps, platforms, and priorities. The future of enterprise software is being defined by increasingly intelligent applications today, and this will accelerate in the future.
By 2019, AI platform services will cannibalize revenues for 30% of market leading companies -Gartner
Cowen predicts it will be commonplace for enterprise apps to have machine learning algorithms that can provide predictive insights across a broad base of scenarios encompassing a company’s entire value chain. The potential exists for enterprise apps to change selling and buying behaviour, tailoring specific responses based on real-time data to optimize discounting, pricing, proposal and quoting decisions.
The Process of Supporting Innovation
Besides developing capabilities among employees, an organization’s culture and processes must also support new approaches and technologies. Innovation waves take a lot longer because of the human element. You can’t just put posters on the walls and say, ‘Hey, we have become an AI-enabled company, so let’s change the culture.’ The way it works is to identify and drive visible examples of adoption.
Algorithmic trading, image recognition/tagging, and patient data processing are predicted to the top AI uses cases by 2025. Tractica forecasts predictive maintenance and content distribution on social media will be the fourth and fifth highest revenue producing AI uses cases over the next eight years.
In the End, it’s about Transforming Enterprise
AI is part of a much bigger process of re-engineering enterprises. That is the major difference between the sci-fi robots of yesteryear and today’s AI: the technologies of the latter are completely integrated into the fabric of business, allowing private and public-sector organizations to transform themselves and society in profound ways. You don’t have to turn to sci-fi. The story of human/machine collaboration is already playing at an enterprise near
Related Posts
AIQRATIONS
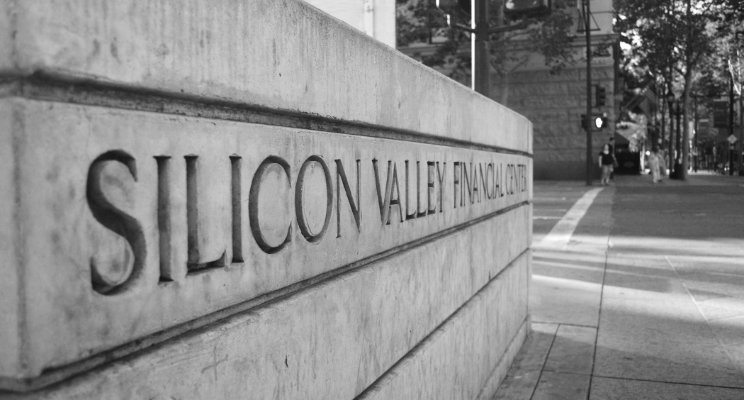
The gold rush for AI – silicon valley vs. China – a perspective hard to ignore
Add Your Heading Text Here
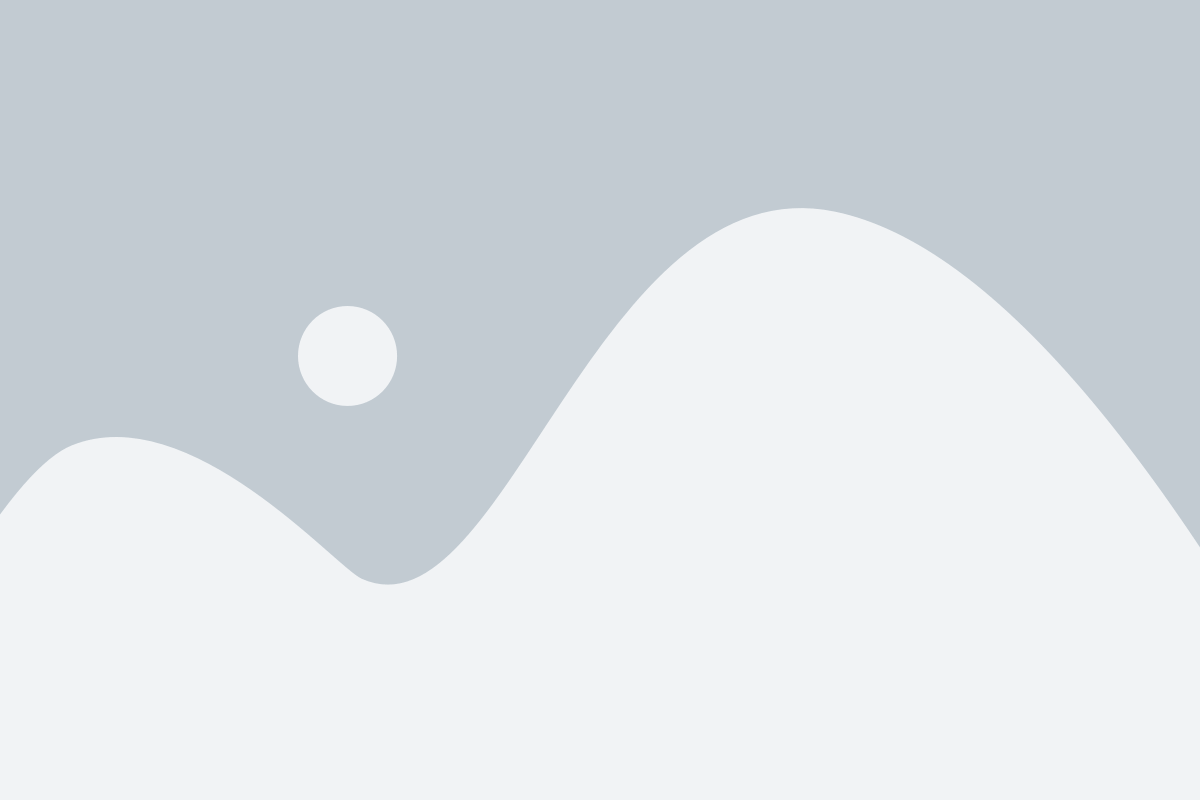
The buzzword among the business and tech communities in China for the past year has been ‘AI’, or artificial intelligence. Artificial intelligence, which allows software to “learn” human ways of thinking, is being incorporated into the largest e-commerce platforms, including Baidu, Alibaba, and Tencent, as well as into data-intensive traditional sectors. With strong government backing and concentrated research in this area, AI is poised to drive China’s economy forward toward higher levels of growth.
China is developing artificial intelligence in improving the capabilities of robotics, developing driverless cars, divining consumer preferences, inventory forecasting, selling enhanced products, and marketing goods and services. According to Liu Lihua, Vice Minister of Industry and Information Technology, China has thus far applied for 15,745 AI patents.
China plans to launch a national AI plan, which will strengthen AI development and application, introduce policies to contain risks associated with AI, and work toward international cooperation. The plan will also provide funds to back these endeavors. Some municipalities also support AI research programs. Beijing, for example, is home to the CAS Institute of Automation, a consortium of universities and firms that provides venture capital funding of 1 billion RMB ($150 million) to AI development. Zhejiang province has also embraced AI programs. Already, Geely Automobile in Zhejiang is using intelligent manufacturing and internet marketing services based on AI to boost sales.
BAT – Chinese AI Frontier Giants
China’s BAT, or Baidu, Alibaba and Tencent, is leading the way for AI in China. Baidu was the first Chinese company to embark upon research in AI, using a system known as Duer to be used in home devices and driverless cars. Driverless auto software provided by Baidu will be made available to car manufacturers under the Apollo Project. Alibaba is using AI to forecast regional order quantities and to improve logistics efficiency, while Tencent has released a platform for deep learning using social data.
Baidu, Alibaba and Tencent have been vying for top talent in AI in order to become leaders in this area. Making headlines several days ago, Alibaba lured Ren Xiaofeng from Amazon.com to lead its own technology lab, which aims to make headway in artificial intelligence. Tencent brought Baidu’s AI expert Zhang Tong on board in March. In 2014, Baidu poached Andrew Ng from the Google Brain project to lead the Baidu Research Institute (though he recently stepped down).
Bay Area dominates this year’s AI funding
Venture investment in startups that are applying artificial intelligence or machine learning has more than tripled in the U.S. since 2013, according to PitchBook Data, with about 60 percent of that coming to founders in the Silicon Valley Bay Area.
The Seattle investment research firm put together a ranking of the top 20 AI deals done around the world this year for me while I was researching this week’s Silicon Valley Business Journal cover story. Almost half of the startups that were funded and nearly three-quarters of the investors involved were from San Francisco and the Silicon Valley region.
The new era in Silicon Valley centers on artificial intelligence and robots, a transformation that many believe will have a payoff on the scale of the personal computing industry or the commercial internet, two previous generations that spread computing globally. Computers have begun to speak, listen and see, as well as sprout legs, wings and wheels to move unfettered in the world.
Silicon Valley’s financiers and entrepreneurs are digging into artificial intelligence with remarkable exuberance. The region now has at least 19 companies designing self-driving cars and trucks, up from a handful five years ago. There are also more than a half-dozen types of mobile robots, including robotic bellhops and aerial drones, being commercialized.
Funding in A.I. start-ups has increased more than fourfold to $681 million in 2015, from $145 million in 2011, according to the market research firm CB Insights. The firm estimates that new investments will reach $1.2 billion this year, up 76 percent from last year.
Even Silicon Valley’s biggest social media companies are now getting into artificial intelligence, as are other tech behemoths. Facebook is using A.I. to improve its products. Google will soon compete with Amazon’s Echo and Apple’s Siri, which are based on A.I., with a device that listens in the home, answers questions and places e-commerce orders. Satya Nadella, Microsoft’s chief executive, recently appeared at the Aspen Ideas Conference and called for a partnership between humans and artificial intelligence systems in which machines are designed to augment humans.
The auto industry has also set up camp in the valley to learn how to make cars that can do the driving for you. Both technology and car companies are making claims that increasingly powerful sensors and A.I. software will enable cars to drive themselves with the push of a button as soon as the end of this decade — despite recent Tesla crashes that have raised the question of how quickly human drivers will be completely replaced by the technology.
AI is in it for the long-haul
Whenever there is a new idea, the valley swarms it. But you have to wait for a good idea, and good ideas don’t happen every day. Silicon Valley’s new A.I. era underscores the region’s ability to opportunistically reinvent itself and quickly follow the latest tech trend. This is at the heart of the region’s culture that goes all the way back to the Gold Rush. The valley is built on the idea that there is always a way to start over and find a new beginning.
Related Posts
AIQRATIONS
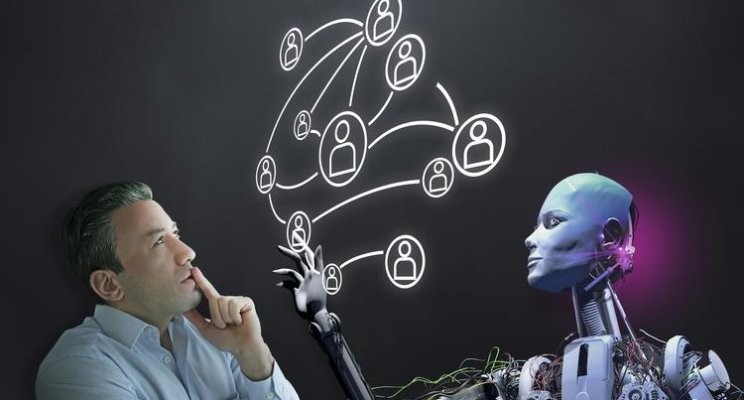
Future of HR :Redefined by AI – perspectives for chief people officer
Add Your Heading Text Here
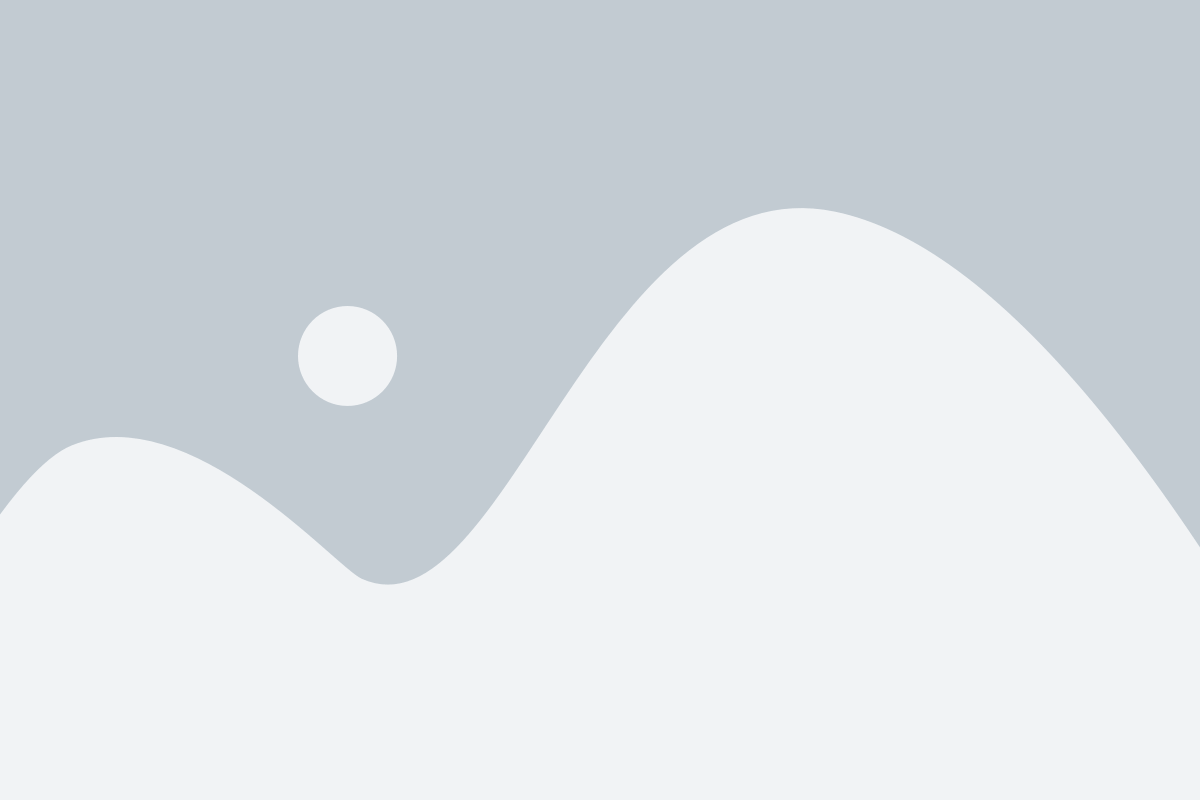
Artificial intelligence is transforming our lives at home and at work. At home, you may be one of the 1.8 million people who use Amazon’s Alexa to control the lights, unlock your car, and receive the latest stock quotes for the companies in your portfolio. In total, Alexa is touted as having more than 3,000 skills and growing daily. In the workplace, artificial intelligence is evolving into an intelligent assistant to help us work smarter. Artificial intelligence is not the future of the workplace, it is the present and happening today.
Investment in AI has accelerated from $282 million in 2011 to $2.4 billion in 2015, a 746% increase in five years. In 2016, this continued to increase with roughly another $1.5 billion being invested in more than 200 AI-focused companies in 2016. AI is becoming indispensable in the healthcare industry. Will we be saying the same thing about CHROs using artificial intelligence in the workplace? Will we consider it unthinkable not to use intelligent assistants to transform recruiting, HR service centers, and learning and development? I believe the answer is yes. HR leaders will need to begin experimenting with all facets of AI to deliver value to their organizations. As intelligent assistants become more widely used in our personal lives, we will expect to see similar usage in the workplace.
For employees, chatbots deliver an unmatched level of employee experience, from real time answers for HR questions to personalized learning and development. In addition, they are critically important to the 3.7 million workers, or 2.8% of the workforce, who work remotely at least half time and do not have easy access to an HR department.
For HR leaders, chatbots are well suited to improving talent acquisition and on-boarding processes by increasing speed and providing greater consistency in answering frequently asked HR questions, improving the talent acquisition process, and enhancing the online learning experience.
AI Chatbots To Answer Frequently Asked Employee Questions
Let’s consider Jane, a chatbot created by Loka, in 2014. Jane provides real time answers to a range of HR questions, including, “Are we off on President’s Day?” or “What are my dental benefits?” Jane is capable of answering any question and answer set that can be stored in a database. In addition to answering frequently asked questions, CEO Bobby Mukherjee says Jane is designed to proactively promote benefits to employees they may not yet know about. Says Mukherjee, “Companies are coming up with lots of new benefits, but they do not have an effective way to promote usage.” Imagine Jane can reach out to employees with, “Hey John, have you tried our Yoga class that we are offering in your building today at 3:00 pm? Click here to automatically book yourself. You’ve been working hard and you deserve it!”
Another value of Jane is the opportunity to track employee issues using real time analytics and then apply sentiment analysis to address these issues. Let’s say that a majority of employees are asking questions about late payments for travel reimbursements. This data can indicate something in the system isn’t working correctly. Before things become a full blown issue, HR leaders can uncover the issue and communicate a solution.
Granted there will be questions Jane cannot answer yet, but the opportunity is here to provide AI for all types of HR related questions that might be coming into your HR Service Center.
AI To Improve Talent Acquisition
Talent acquisition and new hire on-boarding are ripe areas where intelligent assistants can tap multiple data sources to develop candidate profiles, schedule interviews, and make decisions about prospective job candidates.
Talla is a chatbot designed to augment the HR processes that source job candidates. Talla can provide a set of interview questions based upon the role, and can even conduct a Net Promoter Score survey following the recruiting process. Rob May, CEO of Talla, sees, “an intelligent assistant as being able to augment a mid-level HR professionals’ job so she can focus on more strategic HR issues.” The vision behind launching Talla is to ultimately become a real time advisor to HR professionals in how they source and on-board new hires.
May estimates that Talla will save many hours in recruiting and on-boarding new hires and will greatly enhance the employee experience. Improving talent acquisition and new hire on-boarding is a priority for CHROs. According to Eric Lesser, Research Director of IBM Institute for Business Value, “More than half of the CHROs surveyed believe cognitive computing will affect a wide range of roles in the HR organization, ranging from senior executives to individuals working in service centers.”
AI for Talent Management Inside Workplace
AI can also be used for intricate data collection of employees in their workplace in an automated fashion. One option would be to track the movements of the employees inside office space to assess their activities on ground. Companies can track employees’ whereabouts in the office. Bluvision makes radio badges that track movement of people or objects in a building, and display it in an app and send an alert if a badge wearer violates a policy set by the customer—say, when a person without proper credentials enters a sensitive area. The system can also be used to track time employees spend, say, at their desks, in the cafeteria or in a restroom.
Bluvision’s AI compensates for the margin of error in determining location of radio transmitters, allowing the system to locate badges with one-meter accuracy, according to COO John Sailer. Without it, people near one another would be indistinguishable, and the positions of doors, desks, walls and the like—useful information for security and optimizing use of space—would be blurred.
The system is also useful in situations where contractors are paid hourly or piecemeal, such as on a construction site, where subcontractors must complete work in order and on schedule to avoid cost overruns. Although Bluvision tracks individuals, it can also be set to present only aggregate trends. That allows customers to take advantage of location tracking without breaking privacy laws or agreements protecting personally identifying information about employees.
The limits of AI Currently
For all their promise, these systems raise a number of issues. Some are evident today, in the early stages of adoption, while others may take time to become clear.
Privacy is an obvious concern when tracking employees, particularly personal behavior. Systems that sort job candidates also raise questions. Entelo’s may emphasize people with a large online footprint; SAP’s might prefer those who best match characteristics of people who were hired in the past.
Also, the limitations of current approaches boil down to the difficulty of drawing valid conclusions from incomplete data. For instance, measurements of employee performance at any given company are based on the set of people hired and lack information about candidates who were passed over—or weren’t even interviewed—who may have, say, produced more in less time. Aggregating data from many customers, as some larger vendors including SAP and Workday do, can reduce bias, but the problem remains that different companies may not track the same variables in the same way, and subtle but important ones are likely to be missing.
Related Posts
AIQRATIONS
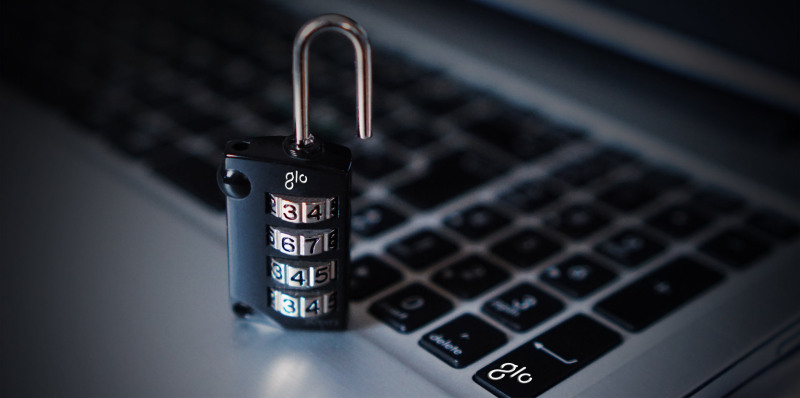
Beating Back Cyber Attacks with Analytics – A Topical Perspective
Add Your Heading Text Here
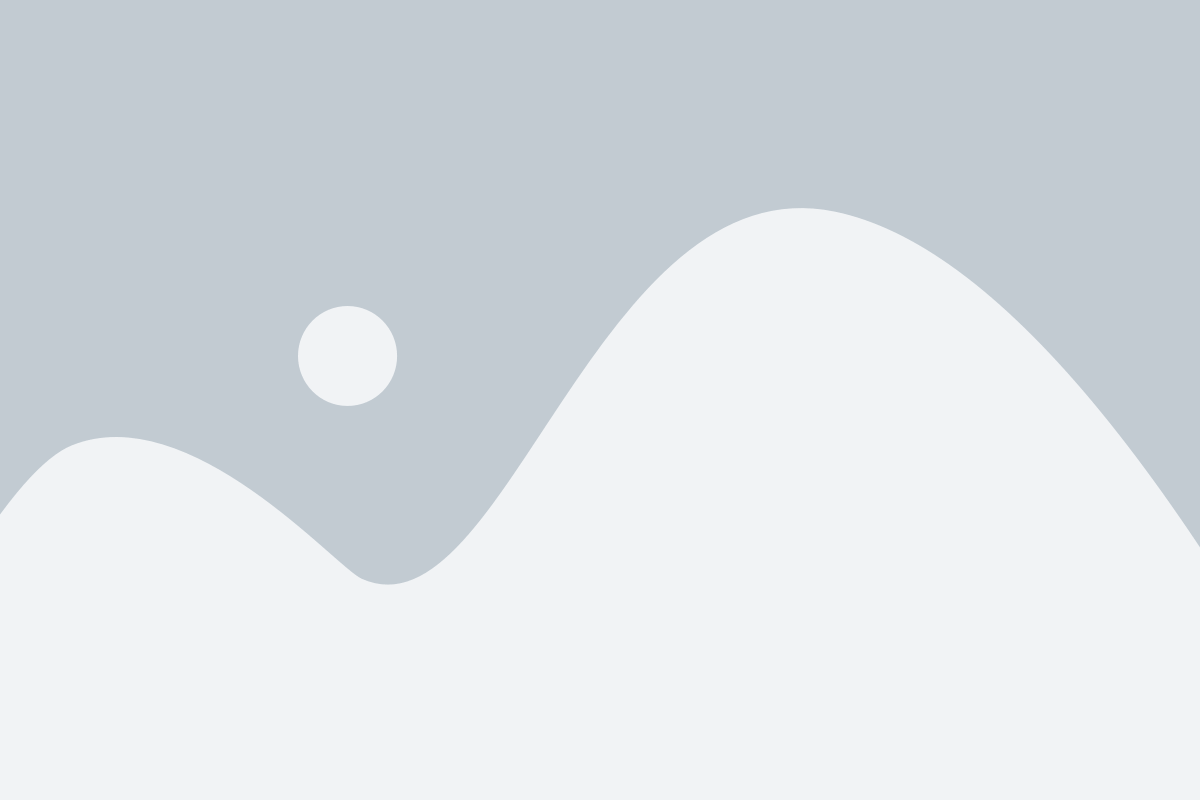
The worldwide cyber attack that began last Friday and goes by the name of “WannaCry” has highlighted the need for governments and businesses to strengthen their security infrastructure, in addition to calling attention to the need to mandate security updates and educate lawmakers about the intricacies of cyber security.
During the WannaCry attacks, hospitals had to turn away patients, and their ability to provide care was altered significantly. Even though the threat is widely acknowledged to be real by the information security community and anyone not living under a rock, and the stakes are higher than ever, most organizations and almost all healthcare providers are still using old-school cybersecurity technologies and retain their reactive security postures.
The WannaCry ransomware attack moved too quickly for security teams to respond, but a few organizations were able to spot the early indicators of the ransomware and contain it before the infection spread across their networks. While it wreaked havoc across the globe, there was nothing subtle about it. All of the signs of highly abnormal behavior on the networks were there, but the pace of the attack was far beyond the capacity of human teams contain it. The latest generation of AI technology enabled those few organizations to defend their networks at the first sign of threat.
Meanwhile, threats of similar – or perhaps worse – attacks have continued to surface. This was not the big one. This was a precursor of a far worse attack that will inevitably strike — and it is likely, unfortunately, that [the next] attack will not have a kill switch. This is an urgent call for action for all of us to get the fundamentals finally in place to enable us to withstand robustly this type of a crisis situation when the next one hits.
Modern malware is now almost exclusively polymorphic and designed in such a way as to spread immediately upon intrusion into a network, infecting every sub-net and system it encounters in near real-time speed. Effective defense systems have to be able to respond to these threats in real time and take on an active reconnaissance posture to seek out these attacks during the infiltration phase. We now have defense systems that have applied artificial intelligence and advanced machine learning techniques and are able to detect and eradicate these new forms of malware before they become fully capable of executing a breach, but their adoption has not matched the early expectations.
As of today, the vast majority of businesses and institutions have not adopted nor installed these systems and they remain at high risk. The risk is exacerbated further by targets that are increasingly involved with life or death outcomes like hospitals and medical centers. All of the new forms of ransomware and extortionware will increasingly be aimed at high-leverage opportunities like insulin pumps, defibrillators, drug delivery systems and operating room robotics.
Network behavioral analytics that leverage artificial intelligence can stop malware like WannaCry and all of its strains before it can form into a breach. And new strains are coming. In fact, by the time this is published, it would not surprise me to see a similar attack in the headlines.
Aanlytics is Turning the Table on Security Threats
The more comprehensive, sensitive and greater volume of end user and customer data you store, the more tempting you are to someone wanting to do harm. That said, the same data attracting the threat can be used to thwart an attack. Analytics includes all events, activities, actions, and occurrences associated with a threat or attack:
- User: authentication and access location, access date and time, user profiles, privileges, roles, travel and business itineraries, activity behaviors, normal working hours, typical data accessed, application usage
- Device: type, software revision, security certificates, protocols
- Network: locations, destinations, date and time, new and non-standard ports, code installation, log data, activity and bandwidth
- Customer: customer database, credit/debit card numbers, purchase histories, authentication, addresses, personal data
- Content: documents, files, email, application availability, intellectual property
The more log data you amass, the greater the opportunity to detect, diagnose and protect an organization from cyber-attacks by identifying anomalies within the data and correlating them to other events falling outside of expected behaviors, indicating a potential security breach. The challenge lies in analyzing large amounts of data to uncover unexpected patterns in a timely manner. That’s where analytics comes into play.
Leveraging Data Science & Analytics to Catch a Thief
Using data science, organizations can exercise real-time monitoring of network and user behaviors, identifying suspicious activity as it occurs. Organizations can model various network, user, application and service profiles to create intelligence-driven security measures capable of quickly identifying anomalies and correlating events indicating a threat or attack:
- Traffic anomalies to, from or between data warehouses
- Suspicious activity in high value or sensitive resources of your data network
- Suspicious user behaviors such as varied access times, levels, location, information queries and destinations
- Newly installed software or different protocols used to access sensitive information
- Identify ports used to aggregate traffic for external offload of data
- Unauthorized or dated devices accessing a network
- Suspicious customer transactions
Analytics can be highly effective in identifying an attack not quite underway or recommending an action to counter an attack, thus minimizing or eliminating losses. Analytics makes use of large sets of data with timely analysis of disparate events to thwart both the smallest and largest scale attacks.
The Analytics Solution to Security Monitoring
If security monitoring is a data storage problem, then it requires a analytics solution capable of analyzing large amounts of data in real time. The natural place to look for that solution is within Apache Hadoop, and the ecosystem of dependent technologies. But although Hadoop does a good job performing analytics on large amounts of data, it was developed to provide batch analysis, not real-time streaming analytics required to detect security threats.
In contrast, the solution for real-time streaming analytics is Apache Storm, a free and open source real-time computation system. Storm functions similar to Hadoop, but was developed for real-time analytics. Storm is fast and scalable, supporting not only real-time analytics but machine learning as well, necessary to reduce the number of false positives found in security monitoring. Storm is commonly found in cloud solutions supporting antivirus programs, where large amounts of data is analyzed to identify threats, supporting quick data processing and anomaly detection.
The key is real-time analysis. Big data contains the activities and events signaling a potential threat, but it takes real-time analytics to make it an effective security tool, and the statistical analysis of data science tools to prevent security breaches.
When do you need to start? – Yesterday
Yesterday would have been a good time for companies and institutions to arm themselves against this pandemic. Tomorrow will be too late.
Related Posts
AIQRATIONS
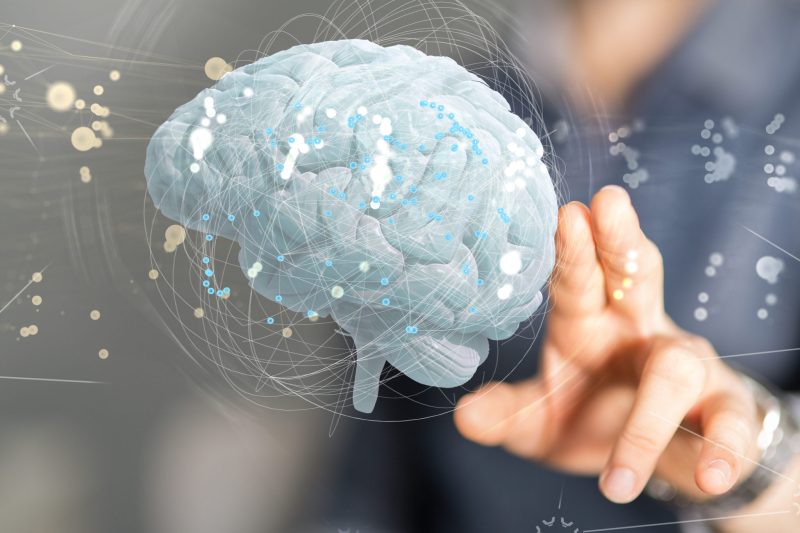
Thick Data – How Science of Human Behavior will Augment Analytics Outcomes
Add Your Heading Text Here
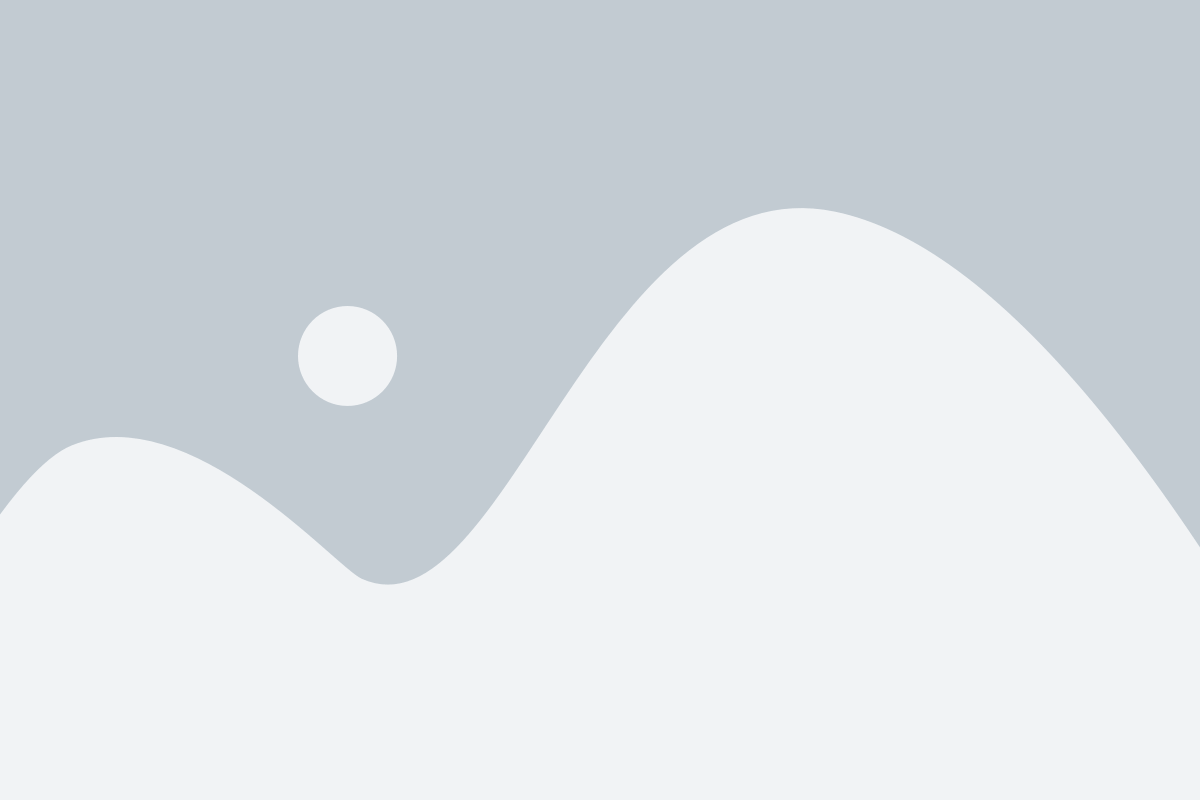
In recent years, there has been a lot of hype around “big” data in the marketing world. Big data is extremely helpful with gathering quantitative information about new trends, behaviors and preferences, so it’s no wonder companies invest a lot of time and money sifting through and analyzing massive sets of data. However, what big data fails to do is explain why we do what we do.
“Thick” data fills the gap. Thick data is qualitative information that provides insights into the everyday emotional lives of consumers. It goes beyond big data to explain why consumers have certain preferences, the reasons they behave the way they do, why certain trends stick and so on. Companies gather this data by conducting primary and secondary research in the form of surveys, focus groups, interviews, questionnaires, videos and other various methods. Ultimately, to understand people’s actions and what drives them to your business (or not), you need to understand the humanistic context in which they pursue these actions.
Human Behavior vs Human Data
It’s crucial for successful companies to analyze the emotional way in which people use their products or services to develop a better understanding of their customers. By using thick data, companies can develop a positive relationship with their customers and it becomes easier for those companies to maintain happy customers and attract new ones.
Take for example Lego, a successful company that was near collapse in the early 2000’s because they lost touch with their customers. After failed attempts to reposition the company with action figures and other concepts, Jørgen Vig Knudstorp, CEO of the Danish Lego firm, decided to engage in a major qualitative research project. Children in five major global cities were studied to help Lego better understand the emotional needs of children in relation to legos. After evaluating hours of video recordings of children playing with legos, a pattern emerged. Children were passionate about the “play experience” and the process of playing. Rather than the instant gratification of toys like action figures, children valued the experience of imagining and creating. The results were clear; Lego needed to go back to marketing its traditional building blocks and focus less on action figures and toys. Today, Lego is once again a successful company, and thick data proved to be its savior.
While it’s impossible to read the minds of customers, thick data allows us to be closer than ever to predicting the quirks of human behavior. The problem with big data is that companies can get too caught up in numbers and charts and forget the humanistic reality of their customers’ lives. By outsourcing our thinking to Big Data, our ability to make sense of the world by careful observation begins to wither, just as you miss the feel and texture of a new city by navigating it only with the help of a GPS.
The Perils of Big Data Exceptionalism
As the concept of “Big Data” has become mainstream, many practitioners and experts have cautioned organizations to adopt Big Data in a balanced way. Many qualitative researchers from Genevieve Bell to Kate Crawford and danah boyd have written essays on the limitations of Big Data from the perspective of Big Data as a person, algorithmic illusion, data fundamentalism, and privacy concerns respectively. Journalists have also added to the conversation. Inside organizations Big Data can be dangerous. People are getting caught up on the quantity side of the equation rather than the quality of the business insights that analytics can unearth. More numbers do not necessarily produce more insights.
Another problem is that Big Data tends to place a huge value on quantitative results, while devaluing the importance of qualitative results. This leads to the dangerous idea that statistically normalized and standardized data is more useful and objective than qualitative data, reinforcing the notion that qualitative data is small data.
These two problems, in combination, reinforce and empower decades of corporate management decision-making based on quantitative data alone. Corporate management consultants have long been working with quantitative data to create more efficient and profitable companies.
Without a counterbalance the risk in a Big Data world is that organizations and individuals start making decisions and optimizing performance for metrics — metrics that are derived from algorithms. And in this whole optimization process, people, stories, actual experiences, are all but forgotten. By taking human decision-making out of the equation, we’re slowly stripping away deliberation — moments where we reflect on the morality of our actions.
Where does Thick Data come from ?
Harvard Business Review (HBR) defines thick data as a tool for developing ‘hypotheses’ about ‘why people behave’ in certain ways. While big data can indicate trends in behavior that allow marketers to form hypotheses, thick data can fill in the gaps and allow marketers to understand why their customers are likely to take certain actions.
While ‘thick data’ is recently receiving a great deal of attention among big data thought leaders, it’s not a new concept. There’s little difference between ‘thick’ data and ‘prescriptive analytics,’ both of which represent advanced maturity in marketing big data. By shifting your focus from predictive big data to forming and testing hypotheses, marketers can better understand how their buyers will act in the future.
Historically, big data has been transactional, while thick data has been qualitative. For data-driven brands of years past, insights into consumer behavior were typically derived from behavioral observation, voice of the customer (VOC) or Net Promoter Score (NPS) surveying, focus groups, or other time-intensive research methods.
Today, insights into consumer behavior can come from a variety of sources. Thanks to social media, internet of things technologies and other drivers of big data, marketers can gain insight into why humans act the way they do with data sources such as:
- Online or Mobile Behavior
- User-generated social media content
- 3rd-party transactional data
Studies indicate that currently, 95% of brand research into consumer preferences is performed manually, using methods such as surveying or focus groups. However, in an era where consumers produce thousands of insights each day from mobile usage, online shopping and social media updates, the insights are easy to obtain.
Finally, will Thick Data take over Big Data ?
This is not to say big data is useless. It is a powerful and helpful tool companies should invest in. However, companies should also invest in gathering and analyzing thick data to uncover the deeper, more human meaning of big data. Together, thick data and big data give you an incredibly insightful advantage.
Related Posts
AIQRATIONS
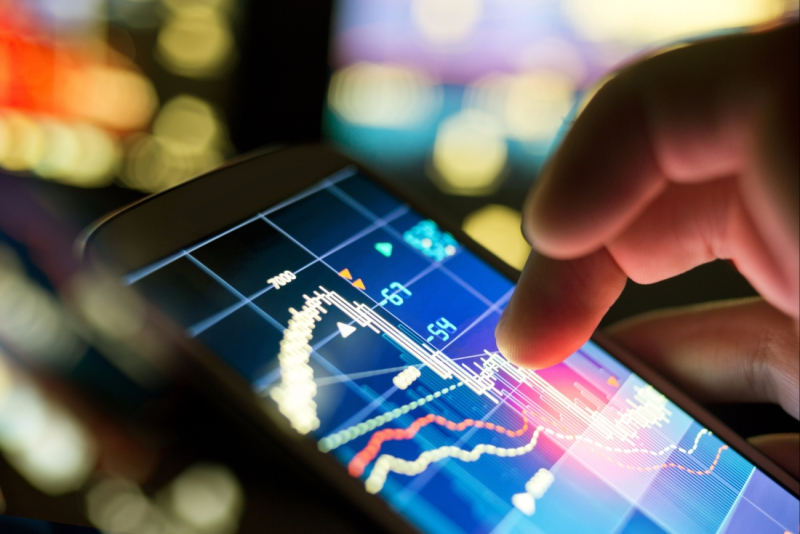
AI & FINTECH – TWO GAME CHANGING REVOLUTIONS IN THE DIGITAL ERA
Add Your Heading Text Here
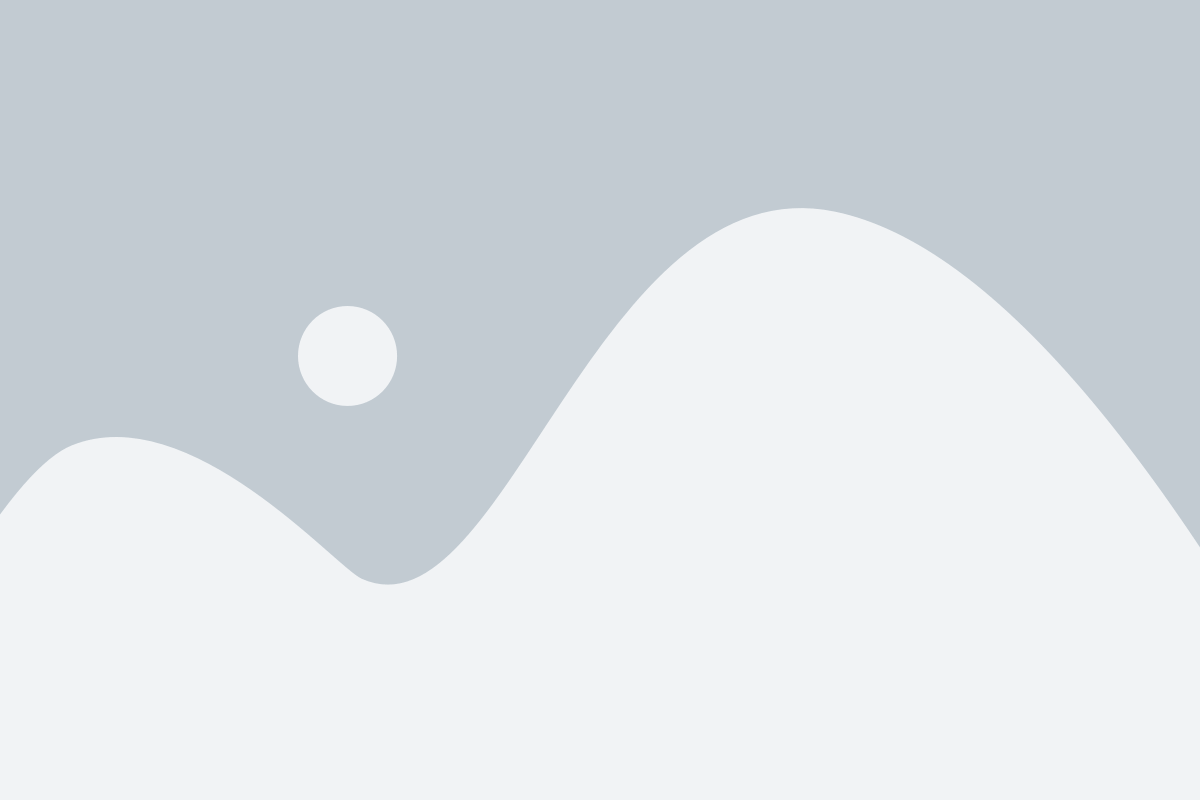
More investors are setting their sights on the financial technology (Fintech) arena. According to consulting firm Accenture, investment in Fintech firms rose by 10 percent worldwide to the tune of $23.2 billion in 2016.
China is leading the charge after securing $10 billion in investments in 55 deals which account for 90 percent of investments in Asia-Pacific. The US came second taking in $6.2 billion in funding. Europe, also saw an 11 percent increase in deals despite Britain seeing a decrease in funding due to the uncertainty from the Brexit vote.
The excitement stems from the disruption of traditional financial institutions (FIs) such as banks, insurance, and credit companies by technology. The next unicorn might be among the hundreds of tech startups that are giving Fintech a go.
What exactly is going to be the next big thing has yet to be determined, but artificial intelligence (AI) will play a huge part.
Stiffening competition
The growing reality is that, while opportunities are abound, competition is also heating up.
Take, for example, the number of Fintech startups that aim to digitize routine financial tasks like payments. In the US, the digital wallet and payments segment is fiercely competitive. Pioneers like PayPal see themselves being taken on by other tech giants like Google and Apple, by niche-oriented ventures like Venmo, and even by traditional FIs.
Most recently, the California-based robo-advisor, Wealthfront, has added artificial intelligence capabilities to track account activity on its own product and other integrated services such as Venmo, to analyze and understand how account holders are spending, investing and making their financial decisions, in an effort to provide more customized advice to their customers. Sentient Technologies, which has offices in both California and Hong Kong, is using artificial intelligence to continually analyze data and improve investment strategies. The company has several other AI initiatives in addition to its own equity fund. AI is even being used for banking customer service. RBS has developed Luvo, a technology which assists it service agents in finding answers to customer queries. The AI technology can search through a database, but also has a human personality and is built to learn continually and improve over time.
Some ventures are seeing bluer oceans by focusing on local and regional markets where conditions are somewhat favorable.
The growth of China’s Fintech was largely made possible by the relative age of its current banking system. It was easier for people to use mobile and web-based financial services such as Alibaba’s Ant Financial and Tencent since phones were more pervasive and more convenient to access than traditional financial instruments.
In Europe, the new Payment Services Directive (PSD2) set to take effect in 2018 has busted the game wide open. Banks are obligated to open up their application program interfaces (APIs) enabling Fintech apps and services to tap into users’ bank accounts. The line between banks and fintech companies are set to blur so just about everyone in finance is set to compete with old and new players alike.
Leveraging Digital
Convenience has become a fundamental selling point to many users that a number of Fintech ventures have zeroed in on delivering better user experiences for an assortment of financial tasks such as payments, budgeting, banking, and even loan applications.
There is a mad scramble among companies to leverage cutting-edge technologies for competitive advantage. Even established tech companies like e-commerce giant Amazon had to give due attention to mobile as users shift their computing habits towards phones and tablets. Enterprises are also working on transitioning to cloud computing for infrastructure.
But where do more advanced technologies such as AI come in?
The drive to eliminate human fallibility has also made artificial intelligence (AI) driven to the forefront of research and development. Its applications range from sorting what gets shown on your social media newsfeed to self-driving cars. It’s also expected to have a major impact in Fintech due to potential of game changing insights that can be derived from the sheer volume of data that humanity is generating. Enterprising ventures are banking on it to expose the gap in the market that has become increasingly small due to competition.
All about algorithms
AI and finance are no strangers to each other. Traditional banking and finance have relied heavily on algorithms for automation and analysis. However, these were exclusive only to large and established institutions. Fintech is being aimed at empowering smaller organizations and consumers, and AI is expected to make its benefits accessible to a wider audience.
AI has a wide variety of consumer-level applications for smarter and more error-free user experiences. Personal finance applications are now using AI to balance people’s budgets based specifically to a user’s behavior. AI now also serves as robo-advisors to casual traders to guide them in managing their stock portfolios.
For enterprises, AI is expected to continue serving functions such as business intelligence and predictive analytics. Merchant services such as payments and fraud detection are also relying on AI to seek out patterns in customer behavior in order to weed out bad transactions.
People may soon have very little excuse of not having a handle of their money because of these services
Concerns Going Forward
While artificial intelligence holds the promise of efficiency, better decision-making, stronger compliance and potentially even more profits for investors, the technology is young. Banks need to find ways to lower costs and technology is the most obvious answer. A logical response by banks is to automate as much decision-making as possible, hence the number of banks enthusiastically embracing AI and automation. But the unknown risks inherent in aspects of AI have not been eliminated. According to a Euromoney Survey and report commissioned by Baker & McKenzie, out of 424 financial professionals, 76% believe that financial regulators are not up to speed on AI and 47% are not confident that their own organizations understand the risks of using AI. Additionally an increasing reliance on artificial intelligence technologies comes with a reduction in jobs. Many argue that the human intuition plays a valuable role in risk assessment and that the black box nature of AI makes it difficult to understand certain unexpected outcomes or decisions produced by the technology.
Towards the future
With the stiff competition in Fintech, ventures have to deliver a truly valuable products and services in order to stand out. The venture that provides the best user experience often wins but finding this X factor has become increasingly challenging.
The developments in AI may provide that something extra especially if it could promise to eliminate the guess work and human error out of finance. It’s for these reasons that AI might just hold the key to what further Fintech innovations can be made.