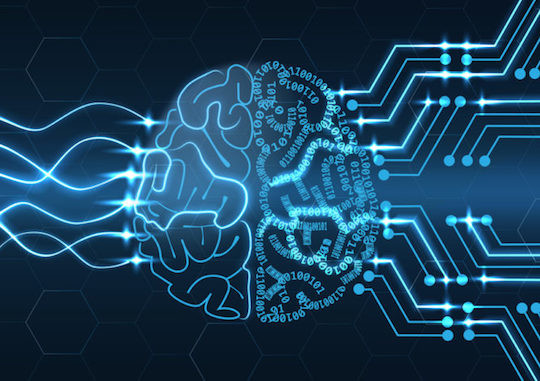
Redesigning exponential technologies landscape with AI & Blockchain fusion
Add Your Heading Text Here
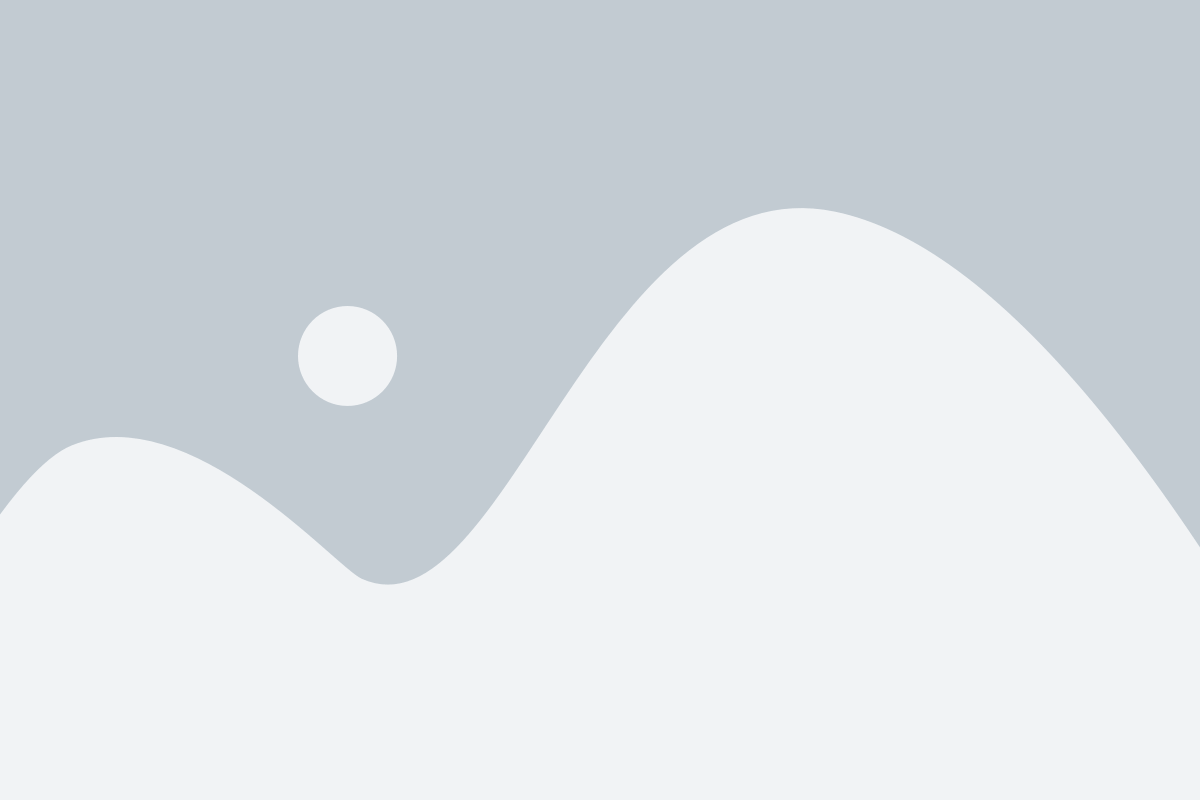
AI and blockchain are two of the prime drivers in the technology space that catalyze the pace of innovation and demonstrating radical shifts across every industry. Each of this technical venture comes with a degree of technical complexity and business implications. Fusion of the two will be able to redesign the entire technical landscape along with a human effect from scratch.
Blockchain has its own limitations, it is a mix of technology-related and culture influence from the financial services sector, but most of them can be conceited by AI in a way or another.
The illustrated points below will be able to give a gist of the potentials that can be realized at the intersection of AI and Blockchain:
Energy consumption in mining: Mining has already proven that it requires tons of energy and is heavy in the economic perspective. AI has mastered in optimizing energy consumption across multiple sectors, similar results can be expected for the blockchain as well. AI can dramatically reduce the costs of maintaining servers and validate potential savings to lower investments in mining hardware.
Federated Learning: Blockchain is growing at a steady pace of 1MB every 10 minutes. Blockchain pruning is a possible solution through AI. A new decentralized learning system such as federated learning, for example, or new data sharing techniques to make the system more efficient.
Security: Concerns still exist on the security system of built-in layers and applications for Blockchain (e.g., the DAO, Bitfinex, etc.). The mileage created by machine learning in the last two years makes AI a solid candidate for the blockchain to guarantee secure applications deployment, especially given the fixed structure of the system.
Blockchain-AI Data gates: Blockchain has proven its ability for record keeping, authentication, and execution while AI drives decisions by assessing/understanding patterns and datasets, ultimately engendering autonomous interaction. The combo (AI and blockchain) will be become a data gate with these several characteristics that will ensure a seamless interaction in the nearest future.
Auditing of AI through blockchain: AI is seen as a black box ( complex set of calculations and algorithms) to distinguish patterns or trends. This makes it a difficult task for the humans to govern the choices taken by the artificial intelligence in yielding results. Accountability of the AI black box is seen as biggest challenge, considering concerns across the community for tampering or the altering happening to the calculations for the given input which eventually reflects in the output generated. This challenge can be easily comprehended by the blockchain innovation. Implementing robust auditing of these calculations utilizing the blockchain is seen as the biggest driver for enhancing the credibility of the business organizations and reinstating trust in the reliability of the information.
Leverage on Artificial Trust: Future roadmap of this fusion can successfully lead into creation of virtual agents that will create new ledger by themselves. Machine to machine interaction will be the new norm reinstating trust in a secure way to share data and coordinate decisions, as well as a robust mechanism to reach a quorum.
Machine performance monitoring and changes: Blockchain miners (companies and individuals) pour an incredible amount of money into specialized hardware components. AI can complement such as machine/equipment monitoring to deploy more efficient systems and do away with the unproductive heavy ones.
Blockchain for better information management: AI has a proven mechanism that runs of an incorporated or centralized database. In such a case, there are always chances for information occurrence of a mishap, i.e. gets lost, altered, or undermined.
Blockchain and artificial intelligence fusion can eliminate the above concern. Under the umbrella of blockchain the data is decentralized and stored within different nodes or systems. This reinstates trust on that your information is safe and unaltered. Most importantly the information is time-stamped and is in the sequence making recuperation less demanding and exact.
Some key challenges on the block: The fusion throws open technical and ethical implications arising from the interaction between these two technologies, such as the need to edit data on a blockchain and most importantly the duo pushing to become data hoarder. Experimentations alone will be able to provide a detailed answer on these lines.
In conclusion blockchain and AI are the two sides of the technology spectrum. One efficiently fosters centralized intelligence while the other promotes decentralized applications in an open-data environment. The fusion of the two will be an intelligent way to amplify positive externalities and advance mankind, most importantly reap the maximum potential for business needs.
Related Posts
AIQRATIONS

The New Age Enterprise – Enabled by AI
Add Your Heading Text Here
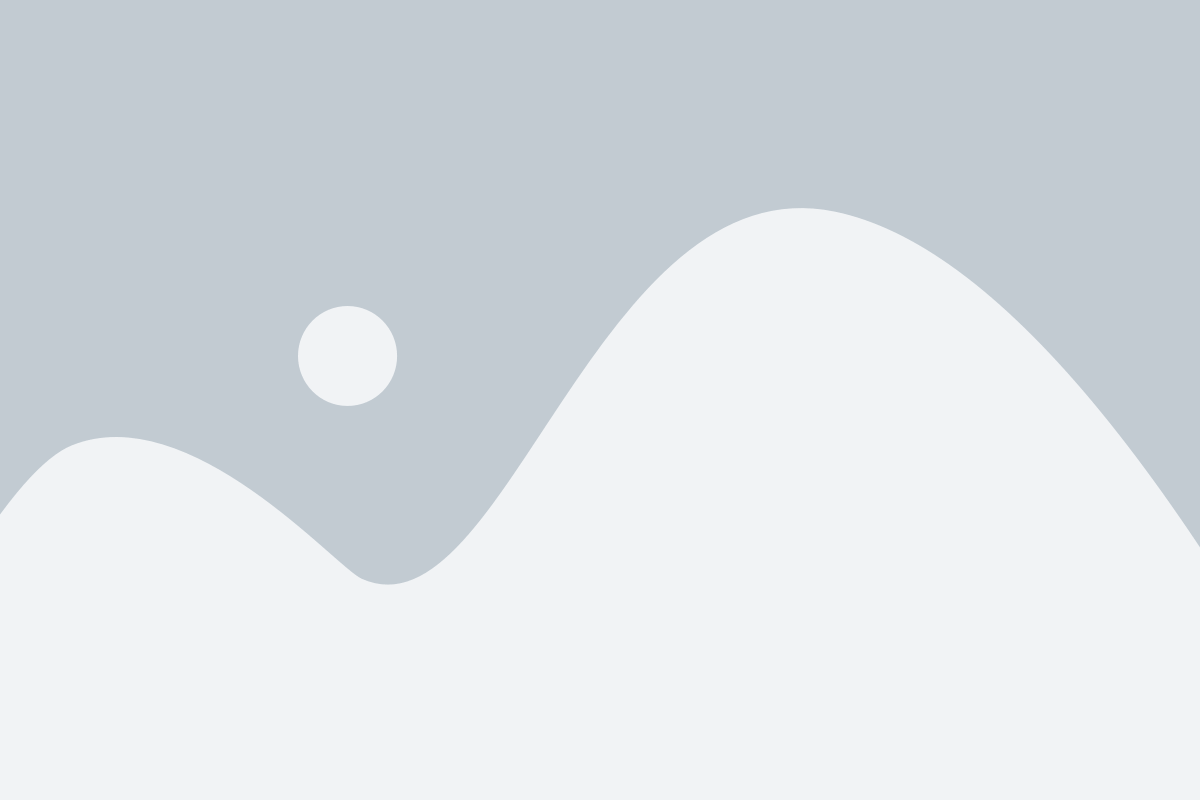
The excitement around artificial intelligence is palpable. It seems that not a day goes by without one of the giants in the industry coming out with a breakthrough application of this technology, or a new nuance is added to the overall body of knowledge. Horizontal and industry-specific use cases of AI abound and there is always something exciting around the corner every single day.
However, with the keen interest from global leaders of multinational corporations, the conversation is shifting towards having a strategic agenda for AI in the enterprise. Business heads are less interested in topical experiments and minuscule productivity gains made in the short term. They are more keen to understand the impact of AI in their areas of work from a long-term standpoint. Perhaps the most important question that they want to see answered is – what will my new AI-enabled enterprise look like?
The question is as strategic as it is pertinent. For business leaders, the most important issues are – improving shareholder returns and ensuring a productive workforce – as part of running a sustainable, future-ready business. Artificial intelligence may be the breakout technology of our time, but business leaders are more occupied with trying to understand just how this technology can usher in a new era of their business, how it is expected to upend existing business value chains, unlock new revenue streams, and deliver improved efficiencies in cost outlays. In this article, let us try to answer these questions.
AI is Disrupting Existing Value Chains
Ever since Michael Porter first expounded on the concept in his best-selling book, Competitive Advantage: Creating and Sustaining Superior Performance, the concept of the value chain has gained great currency in the minds of business leaders globally. The idea behind the value chain was to map out the interlinkages between the primary activities that work together to conceptualize and bring a product / service to market (R&D, manufacturing, supply chain, marketing, etc.), as well as the role played by support activities performed by other internal functions (finance, HR, IT etc.). Strategy leaders globally leverage the concept of value chains to improve business planning, identify new possibilities for improving business efficiency and exploit potential areas for new growth.
Now with AI entering the fray, we might see new vistas in the existing value chains of multinational corporations. For instance:
- Manufacturing is becoming heavily augmented by artificial intelligence and robotics. We are seeing these technologies getting a stronger foothold across processes requiring increasing sophistication. Business leaders need to now seriously consider workforce planning for a labor force that consists both human and artificial workers at their manufacturing units. Due attention should also be paid in ensuring that both coexist in a symbiotic and complementary manner.
- Logistics and Delivery are two other areas where we are seeing a steady growth in the use of artificial intelligence. Demand planning and fulfilment through AI has already reached a high level of sophistication at most retailers. Now Amazon – which handles some of the largest and most complex logistics networks in the world – is in advanced stages of bringing in unmanned aerial vehicles (drones) for deliveries through their Amazon Prime Air program. Business leaders expect outcomes to range from increased customer satisfaction (through faster deliveries) and reduction in costs for the delivery process.
- Marketing and Sales are constantly on the forefront for some of the most exciting inventions in AI. One of the most recent and evolved applications of AI is Reactful. A tool developed for eCommerce properties, Reactful helps drive better customer conversions by analyzing the clickstream and digital footprints of people who are on web properties and persuades them into making a purchase. Business leaders need to explore new ideas such as this that can help drive meaningful engagement and top line growth through these new AI-powered tools.
AI is Enabling New Revenue Streams
The second way business leaders are thinking strategically around AI is for its potential to unlock new sources of revenue. Earlier, functions such as internal IT were seen as a cost center. In today’s world, due to the cost and competitive pressure, areas of the business which were traditionally considered to be cost centers are require to reinvent themselves into revenue and profit centers. The expectation from AI is no different. There is a need to justify the investments made in this technology – and find a way for it to unlock new streams of revenue in traditional organizations. Here are two key ways in which business leaders can monetize AI:
- Indirect Monetization is one of the forms of leveraging AI to unlock new revenue streams. It involves embedding AI into traditional business processes with a focus on driving increased revenue. We hear of multiple companies from Amazon to Google that use AI-powered recommendation engines to drive incremental revenue through intelligent recommendations and smarter bundling. The action item for business leaders is to engage stakeholders across the enterprise to identify areas where AI can be deeply ingrained within tech properties to drive incremental revenue.
- Direct Monetization involves directly adding AI as a feature to existing offerings. Examples abound in this area – from Salesforce bringing in Einstein into their platform as an AI-centric service to cloud infrastructure providers such as Amazon and Microsoft adding AI capabilities into their cloud offerings. Business leaders should brainstorm about how AI augments their core value proposition and how it can be added into their existing product stack.
AI is Bringing Improved Efficiencies
The third critical intervention for a new AI-enabled enterprise is bringing to the fore a more cost-effective business. Numerous topical and early-stage experiments with AI have brought interesting success for reducing the total cost of doing business. Now is the time to create a strategic roadmap for these efficiency-led interventions and quantitatively measure their impact to business. Some food for thought for business leaders include:
- Supply Chain Optimization is an area that is ripe for AI-led disruption. With increasing varieties of products and categories and new virtual retailers arriving on the scene, there is a need for companies to reduce their outlay on the network that procures and delivers goods to consumers. One example of AI augmenting the supply chain function comes from Evertracker – a Hamburg-based startup. By leveraging IOT sensors and AI, they help their customers identify weaknesses such as delays and possible shortages early, basing their analysis on internal and external data. Business leaders should scout for solutions such as these that rely on data to identify possible tweaks in the supply chain network that can unlock savings for their enterprises.
- Human Resources is another area where AI-centric solutions can be extremely valuable to drive down the turnaround time for talent acquisition. One such solution is developed by Recualizer – which reduces the need for HR staff to scan through each job application individually. With this tool, talent acquisition teams need to first determine the framework conditions for a job on offer, while leaving the creation of assessment tasks to the artificial intelligence system. The system then communicates the evaluation results and recommends the most suitable candidates for further interview rounds. Business leaders should identify such game-changing solutions that can make their recruitment much more streamlined – especially if they receive a high number of applications.
- The Customer Experience arena also throws up very exciting AI use cases. We have now gone well beyond just bots answering frequently asked questions. Today, AI-enabled systems can also provide personalized guidance to customers that can help organizations level-up on their customer experience, while maintaining a lower cost of delivering that experience. Booking.com is a case in point. Their chatbot helps customers identify interesting activities and events that they can avail of at their travel destinations. Business leaders should explore such applications that provide the double advantage of improving customer experience, while maintaining strong bottom-line performance.
The possibilities for the new AI-enabled enterprises are as exciting as they are varied. The ideas shared in this article are by no means exhaustive, but hopefully seed in interesting ideas for powering improved business performance. Strategy leaders and business heads need to consider how their AI-led businesses can help disrupt their existing value chains for the better, and unlock new ideas for improving bottom-line and top-line performance. This will usher in a new era of the enterprise, enabled by AI.
Related Posts
AIQRATIONS
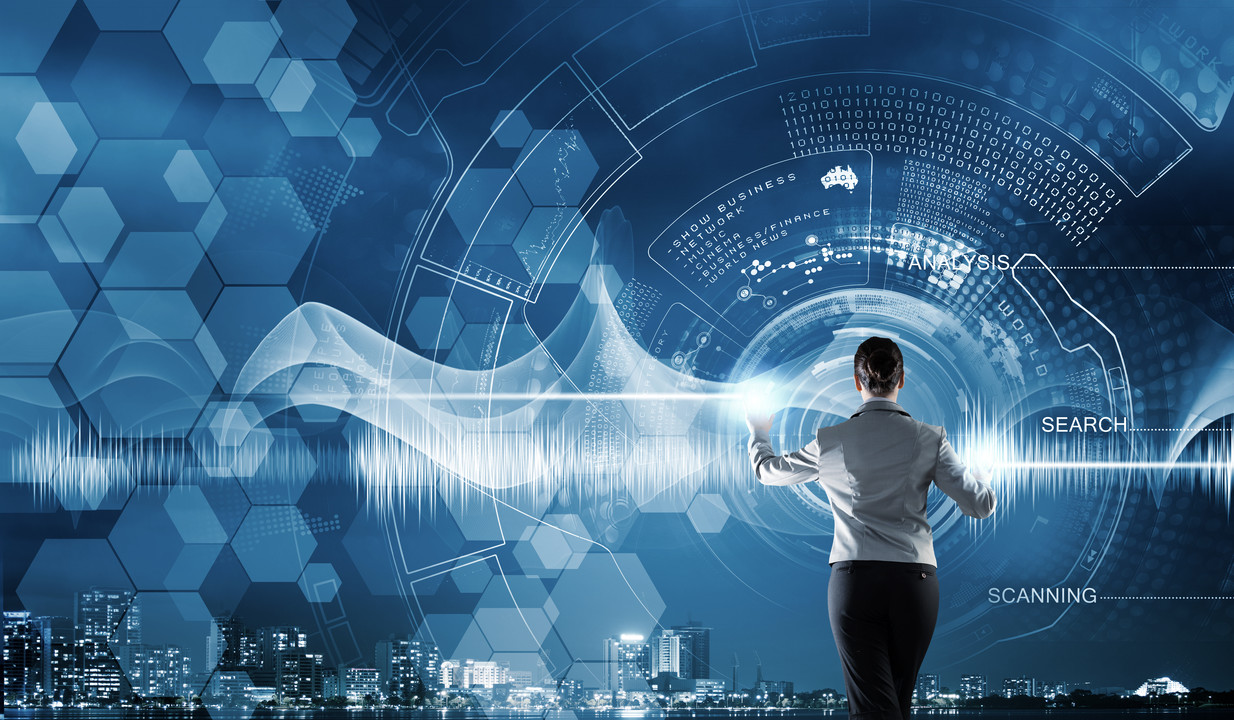
Top 10 Exponential Technologies Trends – 2019
Add Your Heading Text Here
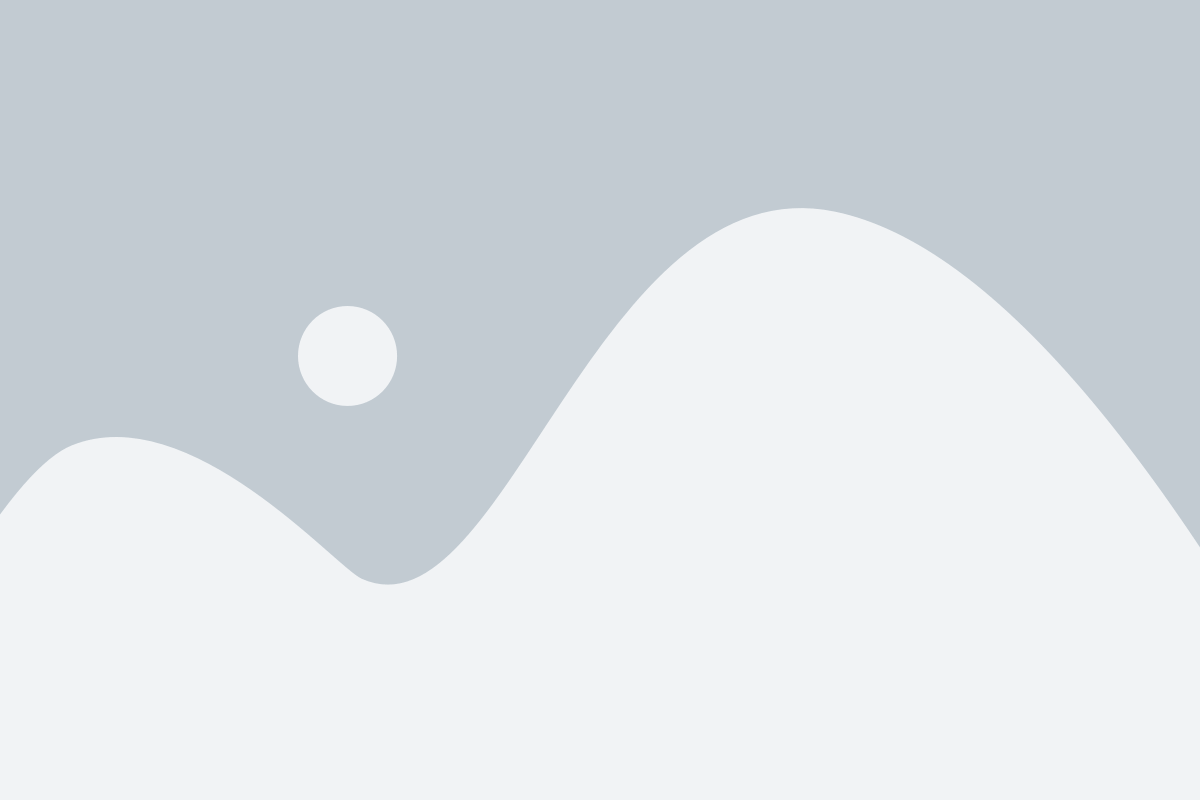
Across the world of technology, we are seeing the proliferation of new age developments across software and hardware – titled “Exponential Technologies”. The term refers to a wide range of recent technology breakthroughs – Artificial Intelligence, Internet of Things, Cloud Computing, Augmented and Virtual Reality, Blockchain and the allied. They are collectively referred to as ‘exponential’ considering the humungous potential value that they could possibly add to business. As these technologies continue to mature in their development and adoption, the world is gaining a more concrete insight into the worth of these technologies and their use cases. 2019 will most certainly be the year where these technologies will go mainstream – and deliver exponential value to their proponents. With high investor interest (and money) riding on these new age technologies, I am confident that in 2019, there will be a high uptake in their commercialization. Here are the top10 trends that I foresee in 2019 in exponential technologies :
1. Blockchain Beyond the Hype
In 2018, there was no doubt a lot of excitement and buzz as technology vendors and investors started investigating blockchain and cryptocurrency. In 2019, expect blockchain to move beyond the hype and enter the mainstream. Gartner estimates that blockchain applications will create $3.1 trillion in business value by 2030. Over 2018, several tech-savvy businesses started their own experiments with blockchain in areas such as supply chain, which is ripe for a blockchain-powered disruption. Within blockchain, I foresee:
Increased collaboration between businesses and tech vendors to unlock the power of blockchain across multiple use cases. Given its immutable and decentralized nature, blockchain will be invaluable in sectors such as manufacturing, defense and financial services – and we will see innovative use cases coming out of these domains
Within blockchain, smart contracts specifically will gain immense traction. The business value of smart contracts is remarkably clear – they drastically reduce the time and effort for routine but lengthy paperwork processes, while maintaining the sanctity through a blockchain network
Due to the numerous crypto frauds seen uncovered in the last year, more and more sovereign governments will push legislation to regulate and establish clear rules around blockchain and cryptocurrency. I have no doubts that this will have a net positive impact – as it will demonstrably improve the consumer confidence and enterprise adoption for these technologies by laying down a clear legal framework for their use
2. 3rd Platform Technology to Accelerate Digital Transformation
A combination of social, mobile, data-driven decision-making and cloud infrastructure and processing is commonly referred to today as 3rd platform technology. In 2019, there will be no stopping the juggernaut of internal IT departments moving ever faster towards digital technology.
According to a research by IDC, it is expected that by 2023, 75% of all IT spending will be on such 3rd platform technology, with over 90% of all enterprises building “digital native” IT environments
Further advanced technologies such as distributed cloud, hyperagile app technologies and architectures, AI at the edge and AI-powered voice UIs will be central to how enterprises enable digital transformation using 3rd platform technologies.
This expansion in demand for 3rd platform technologies will be the outcome on increasing pressures on internal IT to become profit centers and unlocking new sources of revenue for the parent enterprise. Using easily scalable and replicable digital frameworks, early adopter IT departments would be able to commercialize this technologies to their competitors while giving their businesses critical competitive advantage
3. Quantum Computing to Come of Age
Quantum computing is a non-traditional form of computing operating on the quantum state of subatomic particles and representing information as elements denoted through quantum bits. The unmitigated rise in the development and permeation of quantum computing is the third key trend that I see for 2019. It is estimated that by 2023, 20% of organizations will carve out budgets for quantum computing projects, as opposed to less than 1% today.
With heavier software paradigms such as Internet of Things, Artificial Intelligence and blockchain achieving mainstream status, there will be large scale demand for quantum computing to come out of the shadows of academia and into business. Quantum computing will move well beyond a buzzword and will be part of multiple projects at an experimental scale at corporations.
Quantum Computing will succeed where traditional computing has failed, providing parallel execution and exponential scalability. Such systems will take on problems too complex for a traditional approach or where the latency for traditional algorithms would be untenable
Business leaders across multiple industries – automotive, financial, insurance, pharmaceuticals, military and research organizations – will see massive gains through the advancements in Quantum Computing .
4.Acceleration in the Pervasiveness of the Internet of Things
While Internet of Things has demonstrably hit mainstream status across industries such as consumer goods and retail, and use cases such as supply chain and logistics, we will see further acceleration in its adoption in 2019
IOT-enabled hardware devices will proliferate nearly all walks of human life. Devices from sensors, wearables, smart assistants and wearables will be a feature in everyday life for most individuals in the developed world and will be a key focus for powering digital transformation
With increasing demand for IOT-powered devices across use cases will definitively bring endpoint security into focus for enterprises. As IOT devices become the first frontier for communication with consumers through highly sensorized environments, we will see a rapid escalation in the adoption of endpoint security practices and software
To support this deep network of the Internet of Things will require an immediate focus on rapidly enabling 5G connectivity in 2019. Not having a robust underlying infrastructure to support IOT will be disastrous for businesses and individuals who will be highly reliant on it for their day-to-day activity.
5. Convergence of AI, Blockchain, Cloud and IO
Could a future software stack comprise AI, Blockchain and IOT running on the cloud? It is not too hard to imagine how these exponential technologies can come together to create great value. In 2019, I expect that we will see a strong spread of use cases that effectively combine these technologies.
Internet of Things devices will largely be the interface with which consumers and other societal stakeholder will interact. Voice-enabled and always connected devices – such as Google Home and Amazon’s Alexa will augment the customer experience and eventually become the primary point of contact with businesses
Artificial Intelligence frameworks such as Speech Recognition and Natural Language Processing are making huge advances. These will be the translation layer between the sensor on one end and the deciphering technology on the other end
Blockchain-like decentralized databases will act as the immutable core for managing contracts, consumer requests and transactions between various parties in the supply chain
Cloud will be the mainstay for running these applications requiring huge computational resources and very high availability. I expect more cloud vendors to come forward (Amazon and Google for instance already have) with specialized cloud frameworks to handle the torrent of requests that these type of applications would require.
6.New UI/UX Interfaces to Emerge on the Scene
To unlock and harness the true value of exponential technology it is incumbent that we do not rely only on existing paradigms of end-user interfaces such as web and mobile. We need to reinvent new paradigms and explore game changing new interfaces that will help usher better customer and user experiences.
Conversational platforms – ones which are primarily activated through voice and voice-recognition AI will conduct numerous exchanges on behalf of customers. Already we are seeing rapid adoption of conversational interfaces such as Google Home, Amazon Alexa and Apple’s Siri. These will only grow and prominence and entire CX use cases will be centered around these platforms
Virtual Reality (VR), Augmented Reality (AR) and Mixed Reality (MR) will be increasingly leveraged across a vast selection of topical use cases. Incorporating these alongside traditional interfaces will be crucial to delivering the future of an immersive user experience. According to Gartner, we will shift from thinking about individual devices and fragmented user interface (UI) technologies to a multichannel and multimodal experience.
These immersive experience-led interfaces such as VR and AR will become increasingly popular, with 70% of enterprises experimenting with such technology for consumer and enterprise use and 25% of organizations deploying it into production.
7.Edge Computing to become an Enterprise Mandate
Simply put, edge computing is a computing topology in which information processing, and content collection and delivery, are placed closer to these endpoints. For reducing the latency running AI algorithms and eventual response times, edge computing will become an enterprise mandate for use cases involving a convergence of IOT and AI.
In 2019, adoption of edge computing will be driven by the need to keep the processing power close to endpoints as opposed to a centralized cloud server. Having said that, edge computing will not necessitate the creation of a new architecture. Cloud and edge computing will complement each other. Cloud services will be charged with centralized service execution, not only on centralized servers, but also across distributed servers on-premises and on-the-edge devices themselves.
Five years down the line expect to see specialized AI chips, supporting greater processing power, storage and other advanced capabilities. They will be incorporated into a wider array of edge devices. Not too far into the future, we will see 40% of organizations’ cloud deployments include an element of edge computing and 25% of endpoint devices and systems will execute AI algorithms.
We will see more intelligent and empowered edge computing devices as well. According to Gartner, storage, computing and advanced AI and analytics capabilities will expand the capabilities of edge devices through 2028.
8. DevOps Augmented by AI
Despite almost universal acceptance of the DevOps framework across global enterprises, adoption has been patchy and slow. This is due to numerous reasons, ranging from a distributed toolset and a paucity of expert practitioners. However with the emergence of AI, we will see an increased process automation between software development and deployment, accelerating the enablement of DevOps
AI-powered QA suites will increase the automation quotient in the DevOps process. Given the advancements seen in automation, AI will rapidly intervene in the QA process across unit testing, regression testing, functional testing and user acceptance testing.
DevSecOps will combine the power of DevOps and AI in the field of information security. A centralized logging architecture recording suspicious activity and threats combined with ML-based anomaly detection techniques will empower developers to accurately pinpoint potential threats to their system and secure it for the future.
AI will also break the cultural barriers that typically exist between developer and operations teams. . AI-powered systems will enable DevOps teams to have a single, unified view into system issues across a complex toolchain while improving the collective knowledge of anomalies detected and the pathways for redressal.
9.Autonomous Things on the Rise:
At present, we are seeing experiments at an advanced level in the field of autonomous things. Autonomous things comprise whole gamut of unmanned objects – from drones, cars and robots. In 2019, I expect there to be a steady rise in the adoption and appreciation of this area of technology
Autonomous things of today are largely centered around the current paradigm of basic automation and rigid if-else programming rules. The next revolution in the field of autonomous things will be by exploiting the power of AI to exhibit more advanced, proactive and multi-threaded behaviors
Demand for autonomous things will continue to grow, specifically for autonomous vehicles. According to a Gartner survey, by 2021, 10% of new vehicles will have autonomous driving capability, compared to less than 1% in 2017.
Robotics and drones powered by AI will be able to address more complex use cases bringing in further efficiencies to incumbent businesses in the field of logistics delivery, warehouse management and manufacturing
10. AI to Disrupt Cybersecurity
Finally, the last key trend in the exponential technologies space for 2019 pertains to cybersecurity. While this is a remarkably advanced field, we will see continued growth and evolution of cybersecurity in combination with artificial intelligence
Using anomaly detection and machine learning, AI will hugely disrupt the field of cyber security. Security practitioners will be empowered to identify intrusions and malafide behavior faster using automated, always-on algorithms to constantly survey the secured network for wrongful activity and address concerns before they break-ins occur
AI can be quickly training over a massive data set of cyber security, network, and even physical information. Cyber security vendors will soon roll out AI-enabled solutions that will learn at an abstract level to detect and block abnormal behavior, even when this behavior does not fit within a known pattern. I expect that in 2019 companies will incorporate ML into every category of cybersecurity products.
By extension, we will see a fight between good AI and bad AI in the domain of cybersecurity. There are genuine fears that the next generation of attacks will not be carried out by human hackers but pieces of code designed to rapidly infiltrate a secure environment. Countering that with so-called ‘good AI’ will be crucial in undermining the impact these fast-paced attacks can have
Related Posts
AIQRATIONS
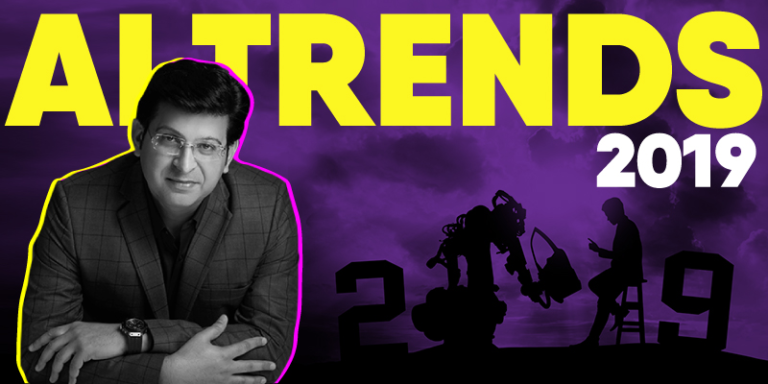
Here are the top 10 AI trends to watch out for in 2019
Add Your Heading Text Here
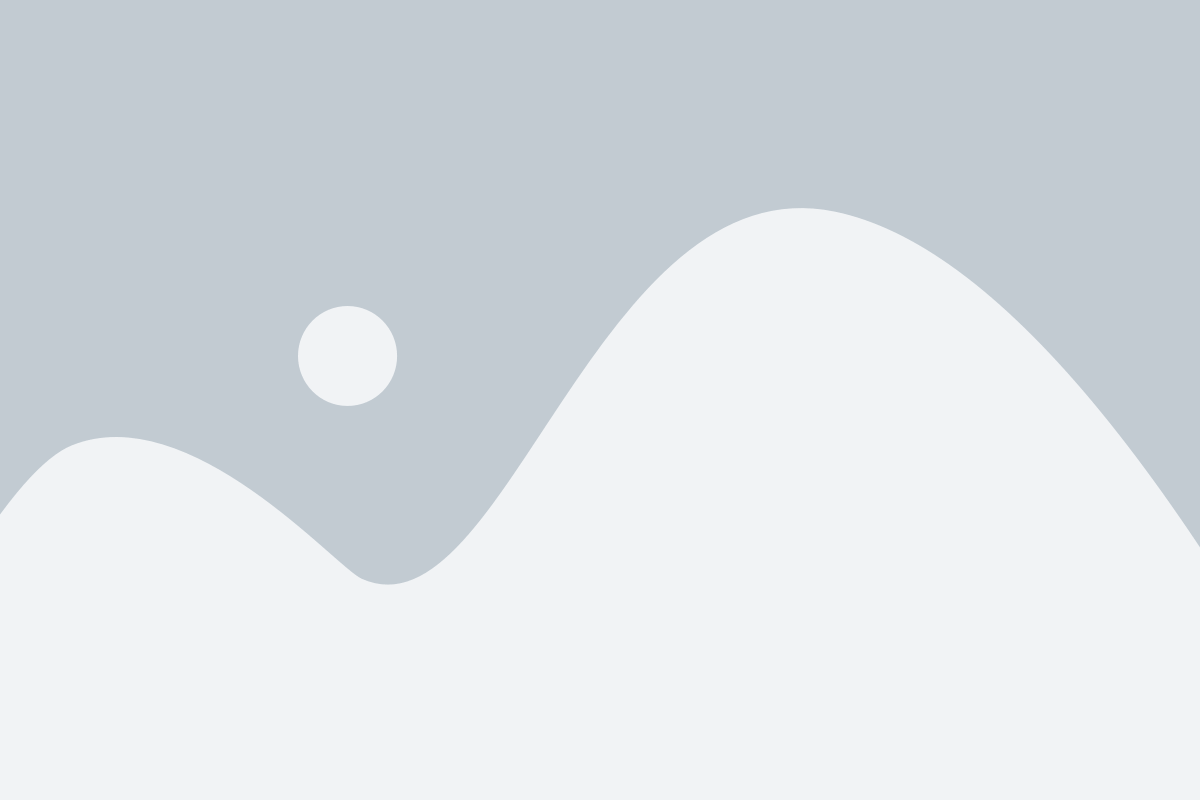
The year 2018 will be remembered as the year that artificial intelligence stopped being on the periphery of business and entered the mainstream realm. With increasing awareness and capability of AI among the numerous stakeholders, including tech buyers, vendors, investors, governments, and academia, I expect AI will go beyond just tinkering and experiments and will become the mainstay in the business arena.
With an increasing percentage of these stakeholders professing their commitment to leveraging this technology within their organisations, AI has arrived on the world scene. We are sure to see transformative business value being derived through AI in the coming years. As we come to the close of 2018, let us gaze into the crystal ball to see what 2019 will hold for this game-changing technology:
The rise of topical business applications
Currently, we have a lot of general purposes AI frameworks such as Machine Learning and Deep Learning that are being used by corporations for a plethora of use cases. We will see a further evolution of such technology into niche, topical business applications as the demand for pre-packaged software with lower time-to-value increases. We will see a migration from the traditional AI services paradigm to very specific out-of-the-box applications geared to serve particular use cases. Topical AI applications in this space that serve such use cases will be monumentally useful for furthering the growth of AI, rather than bespoke services that require longer development cycles and may cause bottlenecks that enterprises cannot afford.
The merger of AI, Blockchain, cloud, and IoT
Could a future software stack comprise AI, Blockchain, and IoT running on the cloud? It is not too hard to imagine how these exponential technologies can come together to create great value. IoT devices will largely be the interface with which consumers and other societal stakeholders will interact. Voice-enabled and always connected devices – such as Google Home and Amazon’s Alexa – will augment the customer experience and eventually become the primary point of contact with businesses. AI frameworks such as Speech Recognition and Natural Language Processing will be the translation layer between the sensor on one end and the deciphering technology on the other end. Blockchain-like decentralised databases will act as the immutable core for managing contracts, consumer requests, and transactions between various parties in the supply chain. The cloud will be the mainstay for running these applications, requiring huge computational resources and very high availability.
Focus on business value rather than cost efficiency
2019 will finally be the year that majority of the executive and boardroom conversations around AI will move from reducing headcount and cost efficiency to concrete business value. In 2019, more and more businesses will realise that focusing on AI solutions that reduce cost is a criminal waste of wonderful technology. Ai can be used to identify revenues lost, plug leakages in customer experience, and entirely reinvent business models. I am certain that businesses that focus only on the cost aspect will stand to lose ground to competitors that have a more cogent strategy to take the full advantage of the range of benefits that AI offers.
Development of AI-optimised hardware and software
Ubiquitous and all-pervasive availability of AI will require paradigm shifts in the design of the hardware and software that runs it. In 2019, we will see an explosion of hardware and software designed and optimised to run artificial intelligence. With the increasing size and scale of data fueling AI applications and even more complex algorithms, we will see a huge demand for specialised chipsets that can effectively run AI applications with minimal latency. Investors are showing heavy interest in companies developing GPUs, NPUs, and the like – as demonstrated by Chinese startup Cambricon, which stands valued at a whopping $2.5 billion since its last round of funding this year. End-user hardware such as smart assistants and wearables will also see a massive increase in demand. Traditional software paradigms will also continue to be challenged. Today’s novel frameworks such as TensorFlow will become de rigueur. Architectural components such as edge computing will ensure that higher processing power is more locally available to AI-powered applications.
‘Citizen AI’ to be the new normal
One of the reasons we saw widespread adoption of analytics and data-driven decision-making is because we built applications that democratised the power of data. No longer was data stuck in a remote silo, accessible only to the most sophisticated techies. With tools and technology frameworks we brought data into the mainstream and made it the cornerstone of how enterprises plan and execute strategy. According to Gartner, the number of citizen data scientists will grow five times faster than the number of expert data scientists. In 2019, I expect Citizen AI to gain traction as the new normal. Highly advanced AI-powered development environments that automate functional and non-functional aspects of applications will bring forward to a new class of “citizen application developers”, allowing executives to use AI-driven tools to automatically generate new solutions.
Policies to foster and govern AI
Following China’s blockbuster announcement of a National AI Policy in 2017, other countries have rushed to share their take on policy level interventions around AI. I expect to see more countries come forward with their versions of a policy framework for AI – from overarching vision to allaying concerns around ethical breaches. At the same time, countries will also be asked to temper their enthusiasm of widespread data proliferation by releasing their own versions of GDPR-like regulations. For enabling an ecosystem where data can be used to enrich AI algorithms, the public will need to be convinced that this is for the overall good, and they have nothing to fear from potential data misuse and theft.
Speech Recognition will revolutionise NLP
In the last few years, frameworks for Natural Language Understanding (NLU) and Natural Language Generation (NLG) have made huge strides. NLP algorithms are now able to decipher emotions, sarcasm, and figures of speech. Going forward, voice assistants will use data from voice and combine that with deep learning to associate the words spoken with emotions, enriching the overall library that processes speech and text. This will be a revolutionary step forward for fields such as customer service and customer experience where many bots have typically struggled with the customer’s tone of voice and intonation.
The growth of explainable AI
And finally, with numerous decisions powered by AI – and specifically unsupervised learning models – we will see enterprises demand “explainable” AI. In simplified terms, explainable AI helps executives “look under the hood” to understand the “what” and “why” of the decisions and recommendations made by artificial intelligence. Development of explainable AI will be predicated on the need for increased transparency and trust. Explainable AI will be essential to ensure that there is some level of transparency (and potentially, learning) that is gleaned from unsupervised systems.
Convergence of AI and analytics
This is a trend that is a logical consequence of the decisive power of data in business today. In 2019, we will see a merger of analytics and AI – as the one-stop for uncovering and understanding insights from data. With advancements in AI seen so far, the algorithms are more than capable of taking up tasks that involve complex insight generation from multi-source, voluminous data. This convergence of AI and analytics will lead to automation that will improve the speed and accuracy of the decisions that power business planning and strategy. AI-powered forecasting will help deliver faster decisions, with minimal human interventions and create higher cost savings for the business.
Focus on physical and cybersecurity paradigms
Two of the domains ripe for an AI transformation are the fields of physical and cybersecurity. As intrusions into physical and virtual environments become commonplace and threats become hugely pervasive, AI will be a massive boost to how we secure these environments. Advances in fields such as ML-powered anomaly detection will drastically reduce the time required to surface potential intrusions into secure environments. This will enable organisations to better protect user data. When combined with Blockchain, AI will give cybersecurity a huge boost through decentralised, traceable databases containing valuable client and strategic information. On the physical security side, Computer Vision is rapidly gaining currency in the fields of physical intruder detection. Surveillance cameras, originally manned by security guards, will soon be replaced by AI-powered systems that will be able to react faster and more proactively to intruders that pose a threat to physical premises. When you combine that with face recognition, working with a database of known offenders, we will see a quantum drop in the time required to adjudicate and address cases of theft and unauthorised entry by law enforcement agencies.
In summary, the broad directions that I predict AI will take include interventions to make it more embedded, responsible, and explainable; convergence with other exponential technologies such as cloud, Blockchain, and IoT; cybersecurity; a greater proliferation and development of use cases; and great strides in the technology and its supporting infrastructure. Enterprises would do well to adopt this revolutionary technology and ensure a strong availability of talent to conceptualise, develop, and unleash value from AI applications.
Related Posts
AIQRATIONS
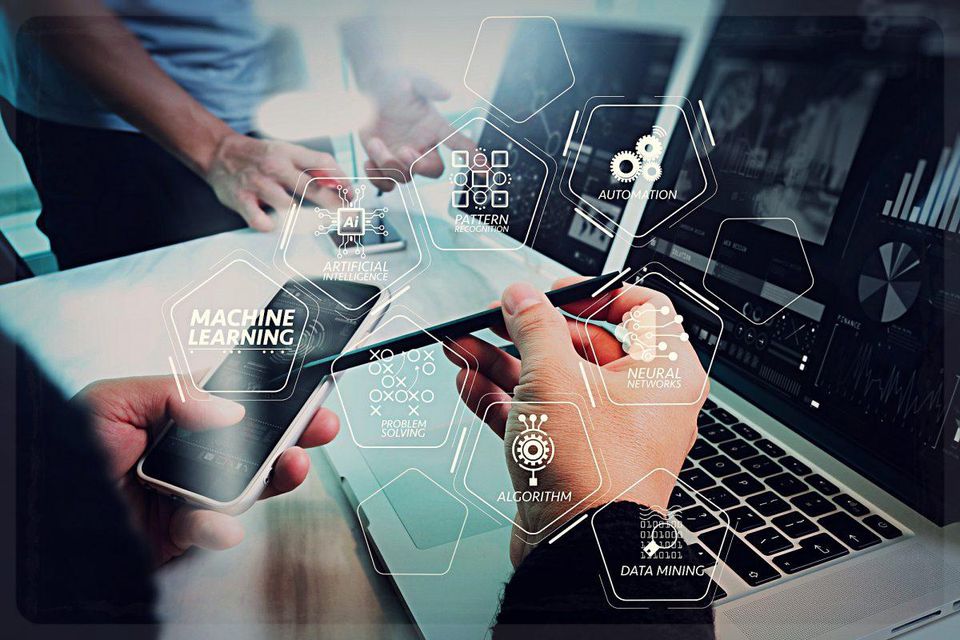
Reimagining Enterprise Decision-Making With Artificial Intelligence
Add Your Heading Text Here
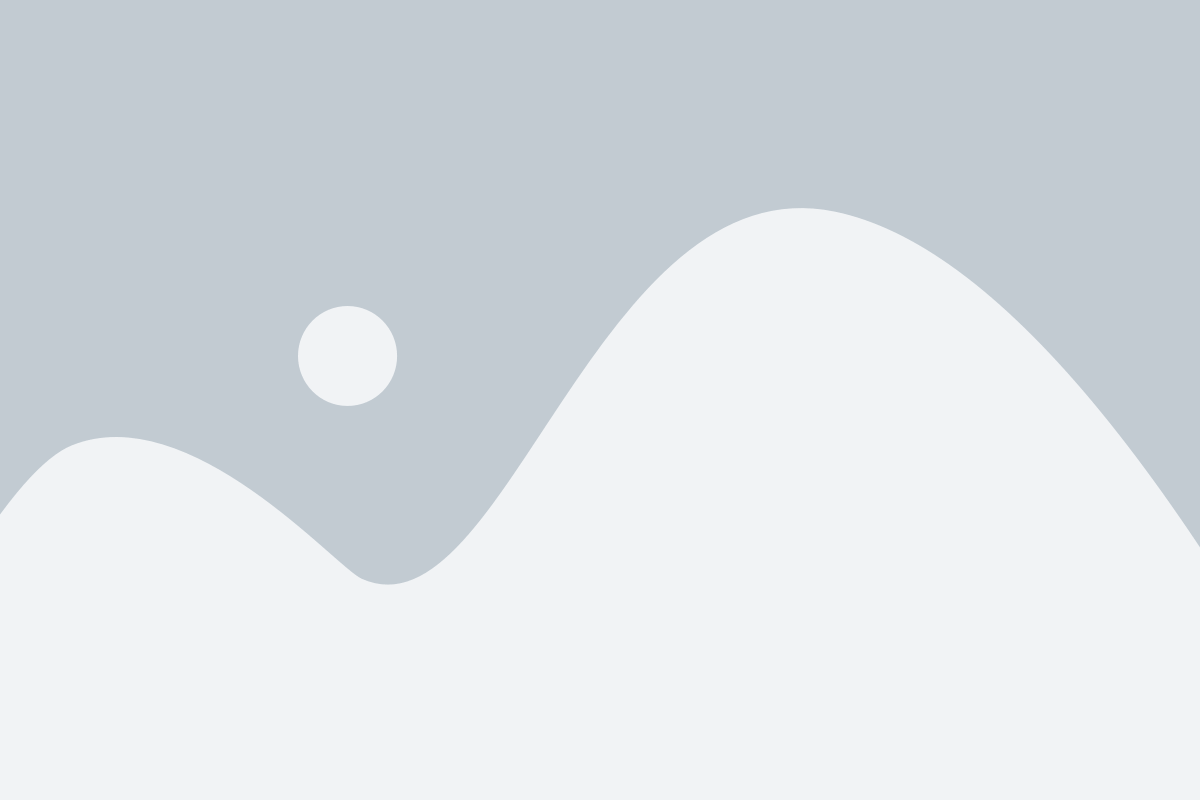
Artificial Intelligence will deliver revolutionary impact on how enterprises make decisions today. In the last few years alone, we have rapidly moved beyond heuristics-based decision-making to analytics-driven decision-support. In the VUCA phase, businesses globally are now pivoting to an AI-led, algorithm-augmented style of decision-making. With huge computing power and ever-increasing data storage and analytics prowess, we are entering a new paradigm, a probable and interesting scenario wherein, Artificial Intelligence will play a huge role in augmenting human intelligence and enabling decision-making with complete autonomy. The big hope is that this new paradigm will not only reduce human biases and errors that are common with heuristic decisions, but also reduce the time involved in making these critical decisions.
Here, I’ll attempt to focus on how we moved from simpler data driven decision-support to AI-powered decisions. The evolution of this technology has been breathtaking to behold and just might provide clues as to what we can expect in the future. Further, I’ll cover a few critical aspects that need to be inculcated by organizations on the AI transformation journey, and provide a few insightful cues that will make this journey exciting and fruitful.
Transformation of Decision-Making: From Analytics to AI
First, let us look at how we got here. Some truly pathbreaking events happened along the way while we were trying to make more accurate business decisions, leading us to reimagine how decisions will be made in the enterprise.
Organizations are Becoming Math Houses
With data deluge and digital detonation, combined with the appreciation of the fact that robust analytical capabilities lead to more informed decisions, we are witnessing AI savvy organizations rapidly maturing into ‘math houses.’ Data science – the ability to extract meaningful insights out of data has become de rigueur. Why? Because we now know that data, when seen in isolation, is inherently dumb. It is the ability to process this data and identify patterns and anomalies – using sophisticated algorithms and ensemble techniques – that makes all the difference. These self-intuitive algorithms are where real value resides – as they define the intelligence required to uncover insights and make smart recommendations. Organizations today are evolving into algorithm factories. There is a real understanding today that by enabling continuous advancement in mathematical algorithms, we can deliver consistent decisions based on prescribed as well as evolving business rules.
It is now an established reality that companies with robust mathematical capabilities possess a huge advantage over those that don’t. Indeed, it’s this math-house orientation that separates companies like Amazon and Google from the ones they leave in their wake, with their ability to understand their customers better, identify anomalies and recognize key patterns.
AI: From Predictive to Prescriptive
We saw a similar evolution in the age of analytics – wherein the science and value veered from descriptive analytics, providing diagnostics of past events to prescriptive analytics, helping see and shape the future. We are seeing a similar evolution in how AI gets leveraged in the enterprise and where its maximum value lies.
In early implementations, it was common to see AI as just a tool to predict and forecast future conditions, while accounting for the dynamism seen in the external environment. Today, AI-enabled decision-making is more prescriptive, with AI providing enterprises not just a look into the future, but also key diagnostics and suggestions on potential decision options and their payoffs. Such evolved applications of AI can help businesses make decisions that can potentially exploit more business opportunities, while averting potential threats much earlier.
Mr. Algorithm to Drive Decision Making
The culmination of this AI-era advancement would be the introduction of smart algorithms in every walk of life and business. Algorithms will become further mainstream leading to what will be the most sweeping business change since the industrial revolution. Organizations – those that already aren’t – will start developing a suite of algorithmic IP’s that will de-bias most enterprise decisions.
If Mr. Algorithm is going to drive most enterprise decisions of tomorrow, we need to create some checks and balances to ensure that it does not go awry. It is more critical today than ever before that the algorithmic suite developed by enterprises has a strong grounding in ethics and can handle situations appropriately for which explicit training may not have been provided.
How to Enable this AI Era of Change
Ushering into an AI-centric era of decision-making will require organizational transformation from business, cultural and technical standpoints. The following facets will be the enablers of this change:
Developing an Engineering Mindset
Instrumenting AI in the enterprise requires a combination of data scientists and computer scientists. As AI matures in the enterprise, the users, use cases and data will increase exponentially. To deliver impactful AI applications, scale and extensibility is critically important. This is where having an engineering mindset comes in. Imbibing an engineering mindset will help standardize the use of these applications while ensuring that they are scalable and extensible.
Learning, Unlearning, Relearning
The other critical aspect to a culture where AI can thrive is creating an environment supporting continuous unlearning and relearning. AI can succeed if the people developing and operating it are rewarded for continuous experimentation and exploration. And just like AI, people should be encouraged to incorporate feedback loops and learn continuously. As technology matures it’s important that the existing workforce keeps up. For one, it’s critical that the knowledge of algorithm theory, applied math alongside training on AI library and developer tools, is imparted into the workforce – and is continuously updated to reflect new breakthroughs in this space.
Embedding Design-Thinking and Behavioral Science at the Center of this Transformation
Finally, given the nature of AI applications, it’s critical that they are consumed voraciously. User input very often activates the learning cycles of artificial intelligence applications. To ensure high usage of these applications, it’s very important that we put the user at the center while designing these applications. This is where the application of behavioral sciences and human-centered design will deliver impact. By imparting empathy in these applications for the user, we will be able to design better and more useful AI applications.
As we augment decision-making with algorithmic, AI-centered systems and platforms – the big expectation is that they will bring untold efficiencies in terms of cost, alongside improvement in the speed and quality with which decisions get made. It’s time to reimagine and deliver on enterprise decision-making that is increasingly shaped through artificial intelligence. These aspects – how the AI is progressing and how to exploit its potential are of paramount importance to keep in mind for an AI transformation.
Related Posts
AIQRATIONS
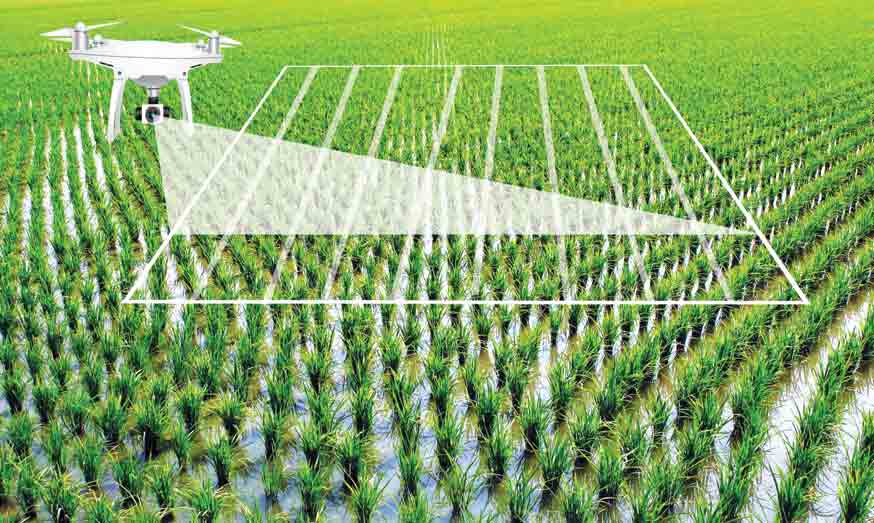
Delivering Smarter Agriculture
Add Your Heading Text Here
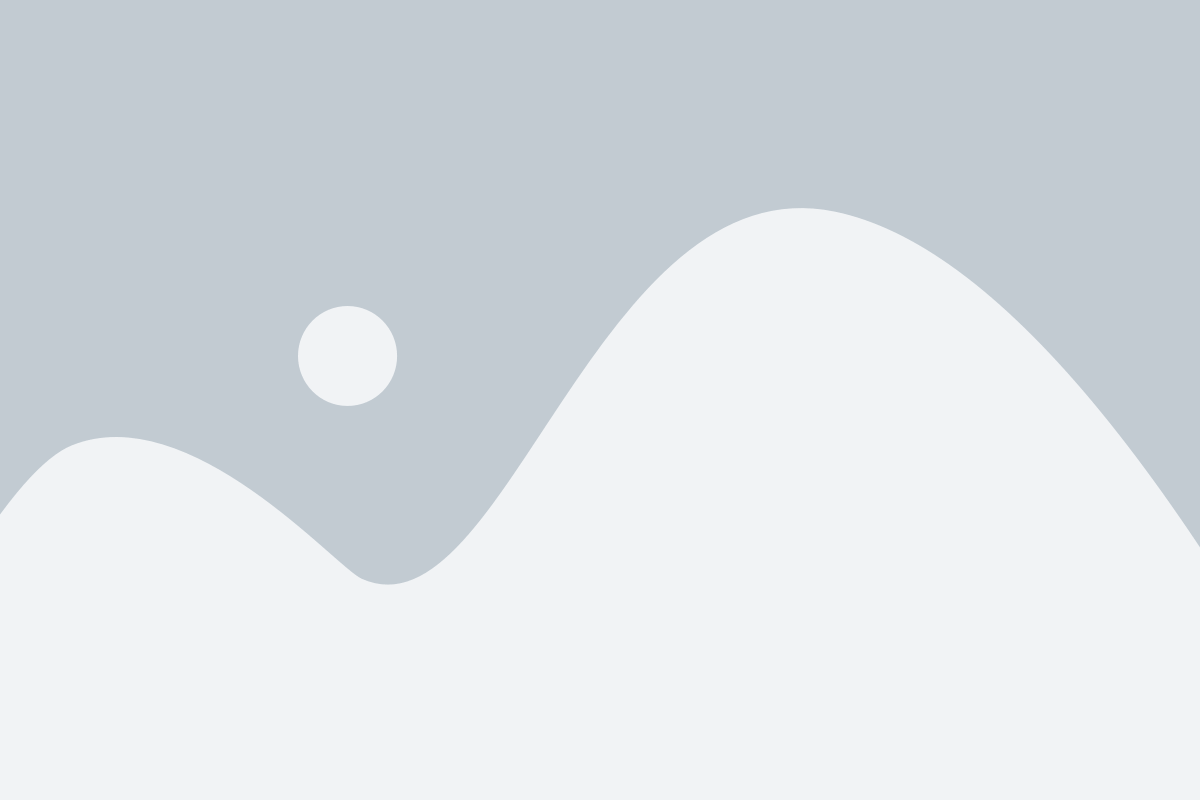
Artificial Intelligence can make a huge impact in the sector by improving operations and productivity
For the many potential application areas where artificial intelligence can deliver breakthrough transformation, unlock efficiencies and augment human life, one of the most impactful areas – at least in the Indian context – will be the value it can bring to the field of agriculture.
According to Indian Brand Equity Foundation, nearly 58% of India’s population relies on agriculture as their primary source of livelihood. The total export of agricultural commodities for India is expected to hit $38.1 billion in FY18, making the country one of the top 15 exporters globally.
However, agriculture in India is riddled with systemic problems, which AI is well placed to address. First, the traditionally unorganised agriculture sector continues to be difficult to organise due to our vast geography combined with our cultural and linguistic diversity. Second, farmers tend to be severely cash-strapped owing to smaller landholding, leading to lower farm productivity. Due to this poor cash position, capital expenditure associated with mechanisation and technology adoption continues to remain slow – thus precluding the possibility of improving farm productivity. Third, farmers are unable to tap a wider base of knowledge and tend to rely on community-based sources of knowledge. This handicap keeps them insulated from the progress of their peers.
Almost all the other large and small topical problems faced by farmers in India flow from one of these three macro-level problems – a lack of organisation, weaker capital muscle to introduce modernisation initiatives and poorer access to a larger, refined body of knowledge. On the bright side, with improved data collection techniques and easier access to cutting-edge research, enabled with better access to telecommunications infrastructure and improving technology literacy, these endemic problems might finally see an amenable solution; combined with the burgeoning interest of the private sector and thrust by the government for modernising farming.
So, it is time for artificial intelligence to step in and deliver a smarter, leaner and productive agrarian sector. Here are the three novel ideas for improving and augmenting farm productivity output using AI:
Communication in Local Languages
AI-powered technology provides a unique opportunity for agriculturists to break down traditional linguistic barriers. Through the infusion of natural language processing (NLP) and machine learning in agriculture, farmers would be able to communicate and learn from their peers and with civic bodies in their local language.
One such example is the development of ‘Kisan Helplines’. Farmers typically need to reach out to civic bodies for information and guidance but this may get hampered due to the poor availability and limited scalability offered by human-centric contact centres. Step in, chatbot. Chatbots can offer high availability, high scalability and reduce the wait time for farmers in need of advice or warnings. Through multi-lingual chatbots powered by NLP, farmers could get easier access to information that may be extremely critical for their activities.
Further, using an NLP-powered communication exchange, they would be able to connect with peers across States for information. Such a communication platform would help crowdsource information on weather progression and potential pest attacks. It would also help seek reviews and feedback on a multitude of farm procurement – seeds, fertilizers, pesticides and mechanical equipment – as well as trade information on successes and practices. By reducing this communication gap and enabling farmers to organise, they would be empowered to make better decisions from sowing to selling and everything in between.
Improving Farming Productivity
While increasing landholding might be challenging, AI can help make the most out of the land available by improving the yield through farm productivity interventions.
By using AI techniques such as machine vision and deep learning, farmers will be able to make many small tweaks to their farming activity that will ultimately result in sizeable gains. For instance, in the pre-sowing and sowing period, machine vision will help farmers monitor soil health and defects – helping them take corrective action early. During the sowing period, farmers can use AI to predict the near-term weather conditions, which can inform the optimal time to sow their seeds. Prior to the harvest, they could harness the power of machine vision to mitigate the impact of pest attacks. Finally, at the time of harvest, machine vision can help farmers grade their produce and demand the right market price.
One such brilliant example of this in action is Microsoft’s initiative with the International Crop Research Institute for the Semi-Arid Tropics (Icrisat), Hyderabad. Their AI sowing app uses machine learning and Microsoft’s PowerBI platform to predict and inform farmers the optimal date to sow. A feature phone capable of receiving text messages is all they need to receive this information. Early results from the use of this technology suggest a 30% improvement in yield per hectare achieved through 175 farmers, who were part of this programme.
Another example is the work being done by Bengaluru-based startup CropIn. Their suite of solutions captures data from multiple sources to provide real-time actionable insights – around weather information, scheduling and monitoring farm activity, monitoring crop health and estimating size of harvest. AI-enabled solutions such as these have great potential to reduce inefficiencies and uncertainties.
Pricing and Supply Chain
While pricing strategies and supply chain optimisation have reached a high level of maturity in the corporate sphere, there is a huge scope for them to impact the agricultural space in India. Using AI, farmers would be able to sense market demand for their goods and keep a pulse of customer choices and seasonality. Market sensing around consumer demand would help them aggressively price their goods and yield a better return.
AI-powered supply chains, on the other hand, can help improve their bottom line by reducing the costs incurred in managing distributed logistics and a multitude of middlemen. Through technology-powered communication networks and smarter routing, smaller farmers too will be able to organise their route-to-market more efficiently and gain benefits of scale. They would also be able to get their perishable goods to market quicker without the intervention of middlemen – thus reducing wastage and losses. An efficient, synchronous farm-to-table supply chain would help bring down their costs and improve their financial position.
At present, AI is only making its first inroads into agriculture. A multitude of use cases exists beyond these as well – from accurately forecasting commodities in the futures market to speeding up loan approval requests through faster credit scoring. AI for agriculture is not only a huge opportunity for startups and large organisations. It also offers the chance to make a huge impact in a sector crying for outside help to improve its operations and productivity.
Related Posts
AIQRATIONS
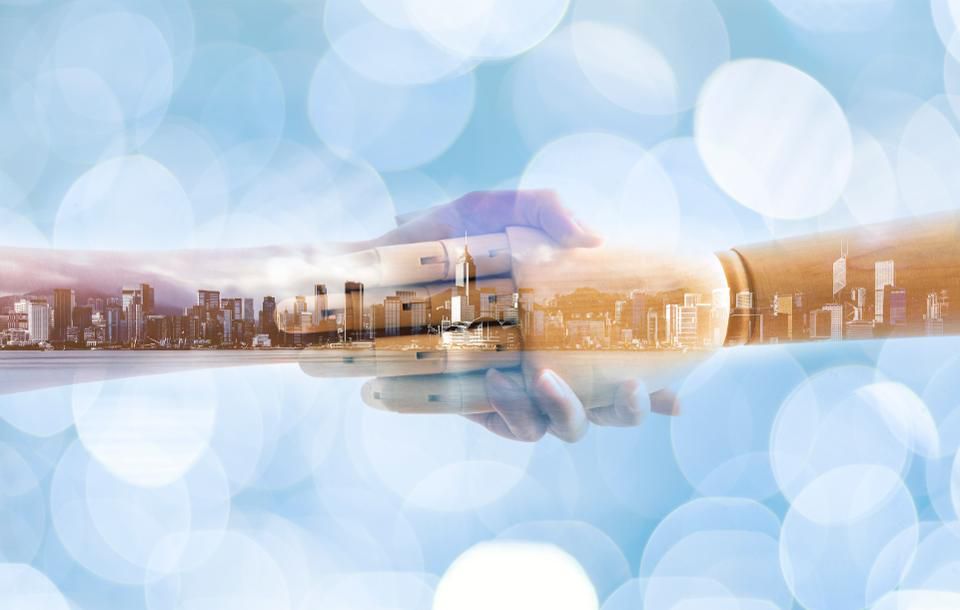
Mapping the AI Transformation Journey In Your Organization
Add Your Heading Text Here
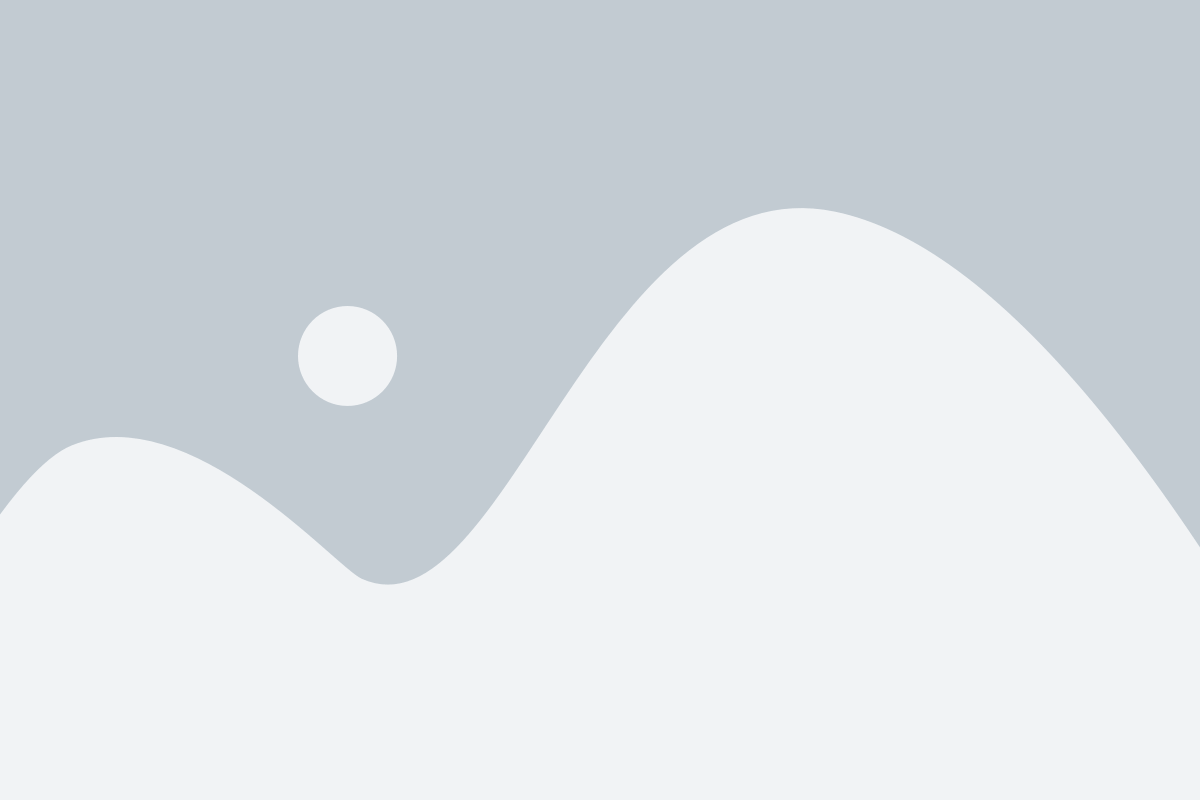
We are well and truly in the midst of the AI revolution. Research houses, academicians, think-tanks, business and technology leaders all agree upon the significant value waiting to be unlocked through the positive and progressive use of Artificial Intelligence – by re-engineering the old and envisioning the new. According to a research by Gartner, organizations using cognitive ergonomics and system design in new artificial intelligence projects will achieve long term success four times more often than others. Citing research by the MIT Center for Digital Business, from a competitive standpoint, companies that embrace digital transformation are 26 percent more profitable than their average industry competitors and enjoy a 12 percent higher market valuation.
The writing is on the wall. Intelligent business interventions made through AI will, to a large extent, define if your business will be an industry leader or a laggard tomorrow. And with that end in mind, businesses are rapidly changing their mindset and approach to AI – from topical experiments performed by forward-thinking business units, to more of a strategic mandate for enabling competitive differentiation. Businesses realize that for truly unlocking business value, they need to not only weave AI into the fabric of their enterprise, but also operationalize it – with the right personnel and change management initiatives. Given that AI can bring both cost efficiencies to business as well as potentially new revenue streams, businesses today are exploring an ‘AI Transformation’ – moving the dial on what is truly possible through a business model, engineered around AI. To enable your organization to do so, here are three powerful ideas to map the AI Transformation journey of your business.
Ensure Enterprise Readiness to Build and Adopt AI
The first step in the journey to AI Transformation for your enterprise is to understand and address if there are any disparities between your vision for AI and the ability of your organization to follow through with it. To that end, it is important to assess just how ready your enterprise is, in its current state, to build, deploy, adopt and benefit from AI-centric solutions. Ideas for AI Transformation need to be communicated clearly and grounded in the realities of organizational capabilities. When they are not, even the best intentions can go awry.
To do so, it is critical that business leaders measure their current AI maturity and assess the availability of internal skills. This will enable you to baseline just how empowered your current workforce is to develop industry-leading AI solutions. Once such a baseline is established on workforce readiness for building and adopting AI-led solutions, organizations need to start improving on these metrics – through internal trainings and external capability augmentation.
By developing this baseline score for AI readiness – organizations can have an objective view of where they are, how far they need to go and what the potential milestones to be achieved are in the journey to AI Transformation. This sort of pre-survey, combined with relevant training and assessment can help organizations craft a relevant roadmap with realistic timelines, as well as concrete actionables.
Build an AI ‘Win Team’
An AI Transformation is not unlike an extremely complex business re-engineering exercise. It entails massive changes – from the way you do business to how you run internal processes and staff multiple business units. Not only is it important to reskill a huge section of the workforce, there is also an important aspect of enabling change management to reinforce the importance of an AI-centric mindset.
To overcome this challenge, enterprises need to foster the consensus and engagement of a ‘win-team.’ This win-team would typically comprise functional and technical leaders who would be responsible for enabling the AI Transformation within their business units – from orienting the employees to the new mindset and ensuring capability readiness for the tasks at hand. On one hand, functional leaders can help their teams identify the processes that can be re-imagined using AI and manage resistance to change. On the other hand, technical leaders would lead the solutioning of technical components, while setting the training priorities and calendars for the workforce.
On change management, enterprises need employees to clearly appreciate the topline and bottomline benefits of an AI Transformation and focus towards enabling it. Employees stand to benefit themselves – as the professional benefits of making this transformation will accrue for their future. To further explore how companies can reduce the defensiveness in implementing AI-led processes further, they could also set innovation objectives for stakeholders as part of their performance metrics. Doing this will help create a strong alignment between individual, team and organizational objectives. A key aspect of AI transformation is ensuring large-scale adoption and usage of AI-powered solutions. AI applications typically fare better with every incremental user feedback and enriched data sources. Adoption and continuous use is a key parameter for the success of this transformation.
Integrated Business Processes over Siloed Business Functions
For years, the view of technology transformation and procurement has been of one that happens at a department / functional level – HR teams buy talent management software, finance teams sanction the purchase of accounting software, and CRMs get implemented to aid the efforts of sales teams. While this serves small technology initiatives, a sea-change is required for progressing an AI Transformation. To foster this, enterprises need to make a shift from a siloed, function-centric mindset to an integrated, process-centric mindset.
This is because AI use cases can often span multiple business units and functions, while tapping into multiple data sources for providing cross-team value, seamlessly. The very nature of AI deployments thus requires a process-centric view, with a strong consensus and buy-in from multiple stakeholders. Furthermore, the budget for purchasing AI services / applications is likely to come from the allocations of multiple beneficiaries across functions. This makes it all the more imperative that enterprises deprioritize functions in favor of processes.
An AI Transformation is doubtless the most strategic subject to be tackled by organizations today. Successful transformations will ensure enterprises go beyond mere automation and cost-cutting strategies and unveil previously unseen business and revenue opportunities. It is also extremely important to consider the role of digitization in building a new technology infrastructure that is AI-ready – possibly decentralized, cloud-based and highly available. There is now an urgent need for business leaders to have more than just a superficial understanding of AI and its successes. They will now be tasked with building and delivering a concrete, value-oriented roadmap for enabling a key transformation in the history of their organizations.
Related Posts
AIQRATIONS
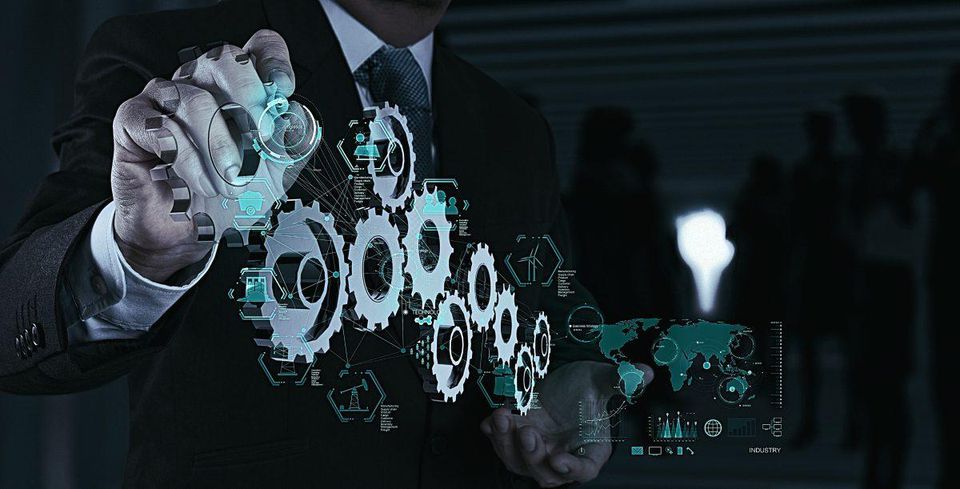
AI is the new MVP in sports
Add Your Heading Text Here
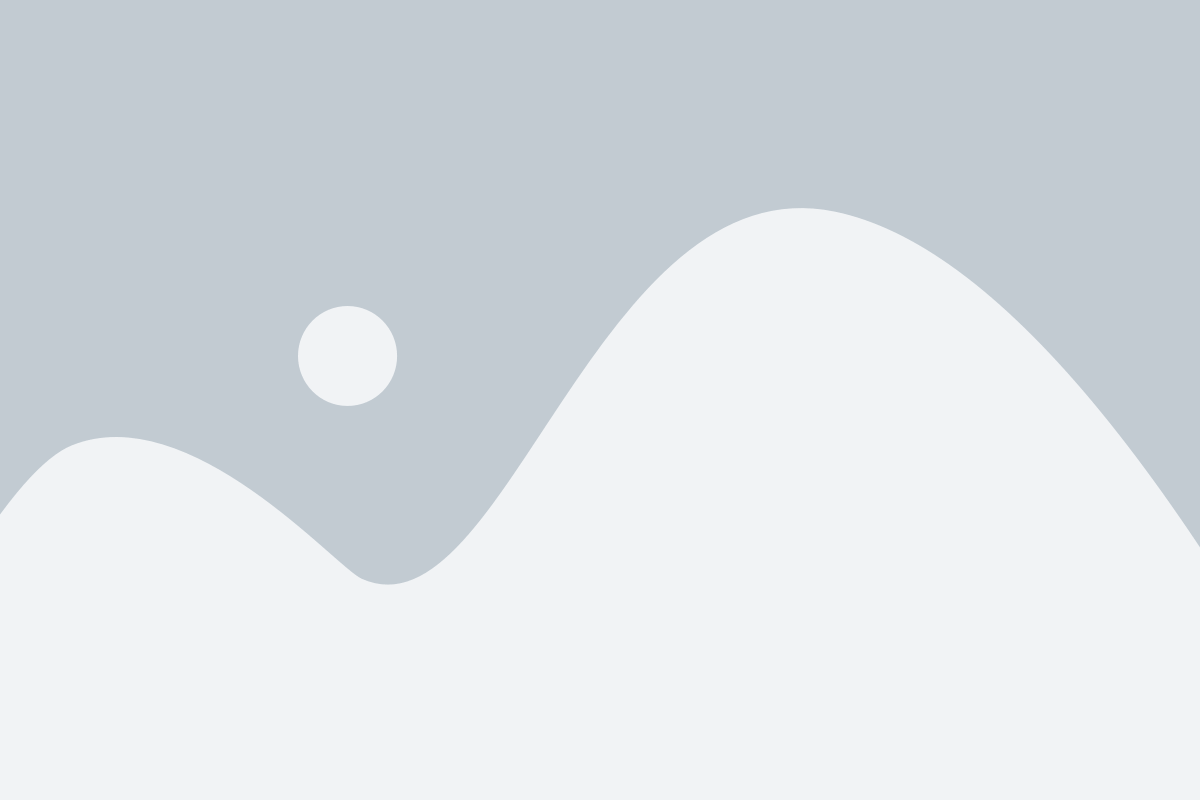
Over the last few years, technology has been rapidly permeating the sporting arena — from DRS in cricket to VAR (video assistant referee) and goal-line technology in football. Moneyball – the seminal book and movie on the use of analytics for smarter player acquisition – was the tipping point in how analytics and AI could be gainfully used in managing the business of sports better.
For years, avid sports fans around the world have had the topline analytics for their favourite players and teams at their fingertips. From there, today carrying analytics on player performance is seeing a massive data detonation. Sports analytics is no longer a mere water-cooler conversation. It is increasingly a specialised science that is rapidly informing the way professional sports team pick, monitor and coach players – in the process, even transforming the way a game is played.
As sports become more competitive, the margin for error is becoming smaller than ever. Teams need to incorporate every possible scientific element that helps improve player performance for a sustained improvement in their rankings. One such science being co-opted in sports is Artificial Intelligence – which is now a key component in the way teams identify players with high potential, monitor in-game progress to provide feedback and provide coaching for long-term success to players. Let us unpack these three crucial areas and see how AI is playing a key role in managing sports teams.
1. Identifying Top Stars
With a wealth of data available right from lower rungs to youth team records, AI can be hugely decisive in scouting for players likely to be mainstays of the senior levels of sport for years to come. By ingesting performance data for each player from their very first game, AI can uncover youth players who have a strong potential or can complement existing players in the team.
Union Minister of State for Youth Affairs and Sports Rajyavardhan Rathore believes that AI will help sports pick future stars. His plan is for the Indian government to create a database of nearly 3 crore young children in the age group of 5 to 18 years, who will be further refined and trained based on their abilities in different sports.
2. Performance and Insights
The major chunk of identified AI use cases falls in the arena of monitoring key performance indicators of sportspersons and providing insights for improvement. A lot of investment has already gone into the development of various sensors and devices that can track speed, accuracy, motion during practise sessions and games.
These monitoring devices – coupled with computer vision and machine learning – will be crucial interventions in the way coaches and players refine their approaches to their sport. Whether it be identifying potential areas to work on during training, or an analysis of situations within the game, AI can combine well with coaches to uncover how to extract the best performance from their teams and players.
An example of this in action is Dutch company SciSports. The company has developed a product called BallJames. Leveraging computer vision and machine learning, the product uses 3D images to provide insights on movements and tactics for football players.
3. Coaching for Success
Augmenting coaches and long-term training programme is the third and rapidly emerging use case of AI in sports. Depending on the sport being played, elite athletes spend between 10 years and 20 years at the very senior level, and possibly 10 years before at junior levels before becoming elite pros. All of which means that these athletes require continuous mental and physical conditioning to stay at the top of their game for nearly 30 years – depending on the sport they are playing.
AI can be an important intervention in building customised training routines for sportspersons – helping them take a long-term view of their health and diet. For instance, using AI, players could identify areas of improvement on the physical side and strengthen muscles, which may be adjudged as below-par for their sport. On similar lines, AI-led apps could also help suggest dietary options based on the conditioning required and provide personalised pathways for these athletes depending on their unique physical characteristics.
Mental Health
The other equally, if not more important aspect of managing the long-term well-being of athletes, is mental health. Sports players often live away from their families, go through swings in form alongside enormous highs and lows of success and failure. Through all of this, it is critical that we also manage the long-term psychological health of athletes to ensure that they stay at the top of their game. Apps such as jolt.ai – chatbots for motivation and tracking workout adherence – could be instrumental if created specifically to manage the mentality of sports players.
While the above mostly dealt with the ‘game’ aspects of sports management, there is also a plethora of topical applications that can be utilised in the management of sports franchisees (fan loyalty and engagement, player acquisition and abilities matching and uplift from sponsorship deals) and sports administrators (spend and procurement management, asset management and tracking legal compliance and fraud). When you combine these two areas, it is evident that Artificial Intelligence could very well be the next MVP in sports!
Related Posts
AIQRATIONS
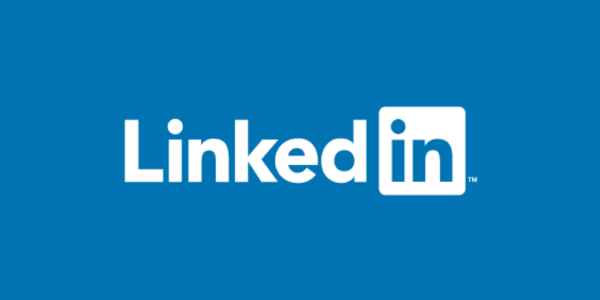
How India is competing for global AI supremacy – critical focus areas to get there
Add Your Heading Text Here
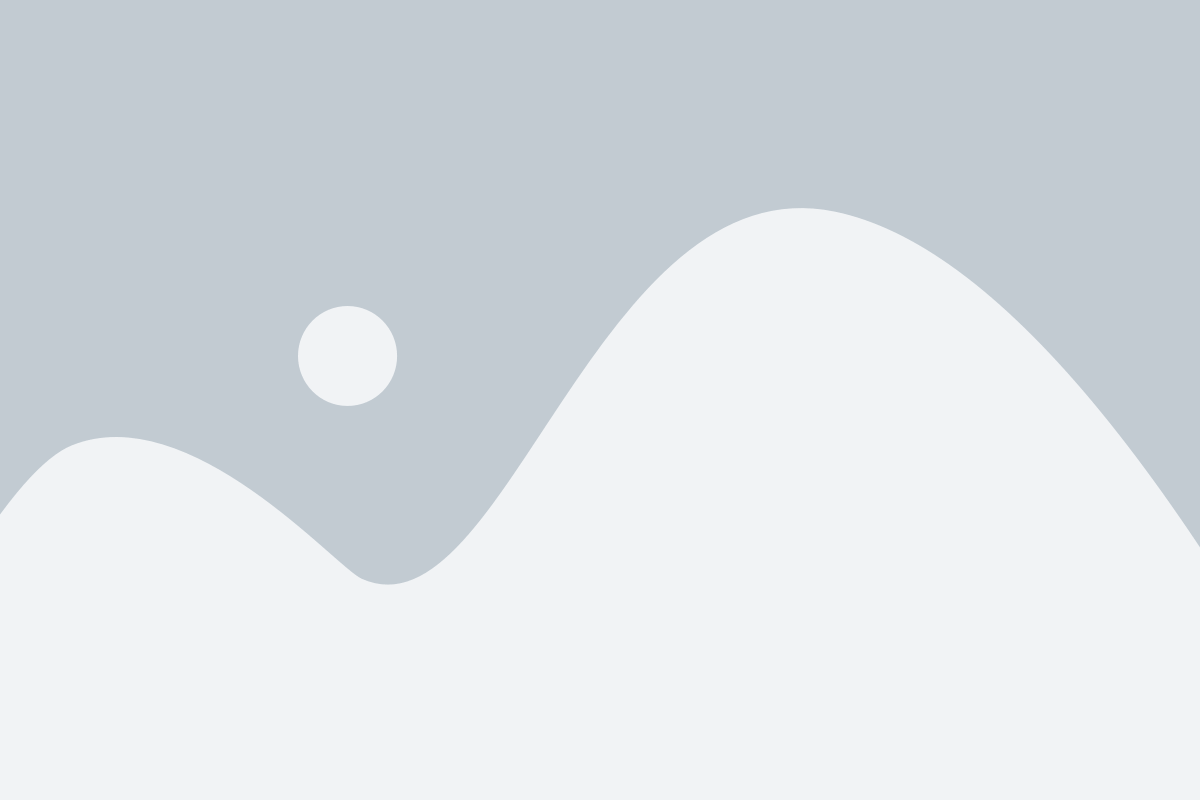
The AI Race is fast heating up. While private enterprises tend to view this through a lens of achieving competitive advantage through breakthrough business and process innovation, there is a much larger play between nations competing to achieve supremacy in the domain of Artificial Intelligence. Across the globe – from Japan in the east to United States in the west – every major industrialized nation is ramping up their efforts (and rhetoric) to build indigenous AI capability. These economies have shown great interest, from the federal to the local levels, to achieving the much-vaunted status as the world leader in Artificial Intelligence. While the approaches by each country may differ – the end goal is some variation of achieving a preeminent position as the single distinguished player in the field of AI.
At this point, it is natural to ask – why? Why are entire economies and governments frantically organizing themselves to win in this race? The answer lies mainly in the size of what is at stake. According to a recent report by global consulting company PwC, AI’s contribution to the global economy is expected to be $15.7 trillion by 2030. The nation that serves the largest portion of this need will not only have the highest revenue, but also the highest number of in-demand professionals, the lowest dependency on other nations in this massive field of work, alongside being the singular force to reckon with in the future of the world.
This might explain why, today, the US and China are at the forefront of this technology. According to the same report, China and North America will see the largest part of the global value-pie ($7trillion and $3.7 trillion respectively). When the stakes are this high, you probably do not want to depend on the benevolence of others. You ought to ensure that every capability you require is available within your own shores. In China, the government stands strongly behind AI adoption, announcing their intention to become “a principal world center of artificial intelligence innovation” by 2030. On the other hand, the US has the highest number of AI startups and one of the deepest wells of venture capital to fund the startups’ endeavors. Not to mention, they are also home to larger tech corporations – Google, Amazon, Facebook, Microsoft, IBM etc. – which are also pioneering AI research in their own way.
While the US and China have taken a quantum leap ahead over their other competitors, the field of AI is not exactly a duopoly. While these two are clearly the leaders across any measurement criteria that you would employ, there are several others in the fray – Japan, South Korea, Germany, France, the UK, Canada, Israel, Russia and India – who are all in various stages of launching their visionary plans and developing on-ground leadership through either private enterprise, public support – or both.
With the size of the prize outlined, the next logical question would be – how is India doing in this space? What steps is India taking to ensure that we do not fall by the wayside as the world runs to win this monumentally important race?
There’s some good news and some not so good news on that front. For one, India is not yet considered among the absolute top rung of AI superpowers today. While we do have significant numbers of STEM graduates passing through academia each year, most of them are currently involved in the so-called lower end of the IT value chain – infrastructure services and maintenance etc. On the bright side, India is uniquely positioned to deliver strong AI leadership, assuming we take steps in the right direction on the policy side, as well as in industry-academic collaboration.
Why do I feel India is uniquely positioned? Consider the following:
- India continues to have a strong continuing focus on STEM education. As AI enters the mainstream curricula of our universities, we will realize the benefits of having a robust intellectual capital in this arena.
- Typically, it is data that powers an AI application. India, with the second largest population in the world (and increasingly connected to smart devices) has the potential to not only generate massive data sets, but also one of the most diverse set of data due to the inherent diversity across class, language and other cultural aspects – which can power the most enriched applications of AI
- There is a strong impetus on the policy front in India for AI – with Digital India, Skill India programs started by this government, in addition to constituting NITI Aayog – a national-level think-tank to execute on a vision rich with emerging technology
So how can we combine India’s inherent advantages, with some strong moves already made in the AI space, to possibly achieve AI supremacy in the near future? Here are three clear areas that require a high degree of attention and action to fulfil that vision.
- Lead with Policy
With a strong, forward-looking government, India is already making the right noises on the development of AI. NITI Aayog – the think-tank I had mentioned earlier – has constituted a committee to study and deliver a National AI Strategy for India. In their June 2018 discussion paper, they identified 5 areas where India is uniquely poised to deliver AI leadership due to our intrinsic advantages – healthcare, agriculture, education, smart cities and smart mobility and transportation. While the Aadhar program has had its critics, it is likely to be instrumental in building a massive training set of citizen data, enabling India to build some thought-leading application in AI. The government has also pledged to put their money where their mouth is – with $480mn projected to be spent on the Digital India program in 2018. While this spending pales in comparison to the spending of other countries (China has committed $150bn up to 2030), it will be instrumental for founding a strong test-bed for incubating our AI vision. The government is also planning a national data and analytics platform in collaboration with private players to utilize the huge amount of data with the help of AI.
2. Facilitate through Academia
Close to 2.6mn students graduated out of STEM fields from India in 2016. While I mentioned that these graduates have anywhere between no to a rudimentary understanding of AI today – it does represent the huge footfall seen in these fields, who would be well-served through a healthy training in AI-centric technologies.
The more pressing problem can be seen in core AI research. While India is ranked 5th in the world today terms of number of papers published (14,864 between 2010-16), we are still a fair way behind the US (63,344) and China (39,820) on this metric. Worse still, India ranks a distant 19th on the metric of H-Index (measured between 1996 and 2016), which leads to a concern on whether our current research is citation-worthy or rooted in business applicability. So, while the appetite for research exists, the contribution to the overall body of knowledge still needs some upgrading.
To address this, the aforementioned NITI Aayog discussion paper, recommends the set-up of a 2-tier integrated approach for boosting research in both core AI and applied AI. The first – COREs (Centers of Research Excellence in Artificial Intelligence) will be focused on developing a better understanding of existing core research and pushing technology frontiers through creation of new knowledge. The second – ICTAI (International Centre for Transformational Artificial Intelligence) will have a mandate of developing and deploying application-based research through Private sector collaboration. This framework would also consist an umbrella organization addressing issues relating to access to finance, social sustainability and the global competitiveness of the technologies developed. This body would be similar to the Campus for Research Excellence and Technological Enterprise (CREATE), Singapore program or Innovate UK.
3. Implement through Private Industry
While the first two points deal with strengthening the backbone of AI research and education, this final aspect deals with building high-class industry-grade IP with wide applicability. Due to a huge democratization in information, both large tech corporations and startups are aware of the challenges that can be solved through AI and are building solutions to address these challenges. Behemoths IT and consulting players are already investing in academic partnerships to set up a base for IP development and workforce training. Startups too, while not similarly endowed, are looking to build visionary products that will transform the industry through collaboration with academia. Through such an industry-academia collaboration, Indian technology companies would be able to foster synergy by developing bleeding edge research in India which can be gainfully employed to solve global challenges. Extending the Make in India initiative would be crucial to ensure that the intellectual property of the work done by Indians stays in the home country, boosting our credibility in this space.
In conclusion, while India is already among some of the top nations in the world today in the field of Artificial Intelligence, there still is a long way to go to hit the absolute pinnacle in this space. However, given that AI is still is in a nascent stage, there is significant scope for India to still emerge as the leading light in this space. With this sustained and rapid pace of progress, I am certain that India will soon emerge as the preeminent leader in the field of AI.
Related Posts
AIQRATIONS
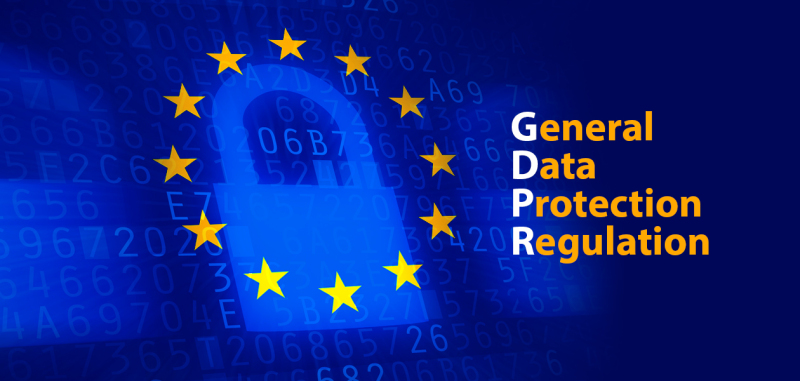
HOW AI CAN ENABLE ENTERPRISES TO IMPLEMENT GENERAL DATA PROTECTION REGULATION (GDPR) POLICIES
Add Your Heading Text Here
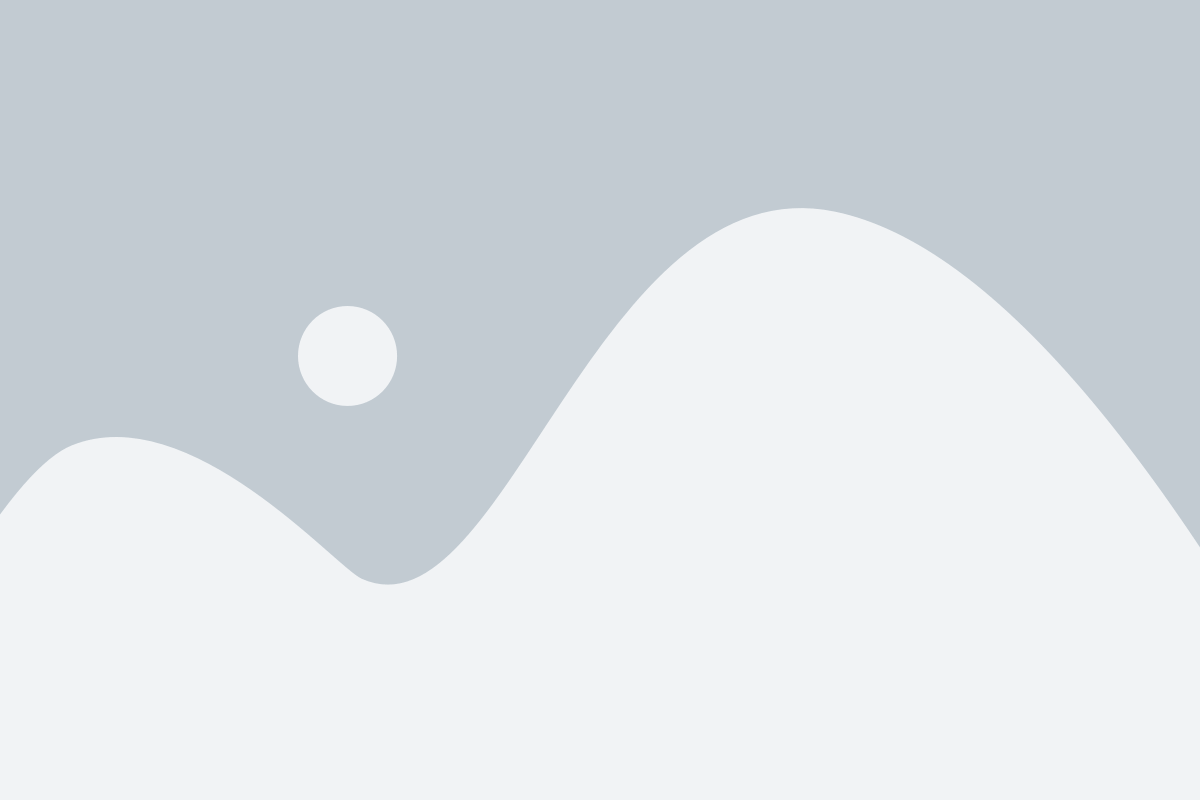
The GDPR General Data Protection Regulation (GDPR), which goes into effect May 25, 2018, requires all companies that collect data on citizens in EU countries to provide a “reasonable” level of protection for personal data. The ramifications for non-compliance are significant, with fines of up to 4% of a firm’s global revenues.
This European Union’s sweeping new data privacy law, is triggering a lot of sleepless nights for CIOs grappling with how to effectively comply with the new regulations and help their organizations avoid potentially hefty penalties.
Will AI be the only answer to the highly regulated GDPR to come?
The bar for GDPR compliance is set high. The regulation broadly interprets what constitutes personal data, covering everything from basic identity information to web data such as IP addresses and cookies, along with more personal artifacts including biometric data, sexual orientation, and even political opinions. The new regulation mandates, among other things, that personal data be erased if deemed unnecessary. Maintaining compliance over such a broad data set is all the more challenging when it is distributed among on-premises data centers, cloud offerings, and business partner systems.
The complexity of the problem has made GDPR a top data protection priority. A PwC survey found that 77% of U.S. organizations plan to spend $1 million or more to meet GDPR requirements. An Ovum report found that two-thirds of U.S. companies believe they will have to modify their global business strategies to accommodate new data privacy laws, and over half are expecting to face fines for non-compliance with the pending GDPR legislation.
This begs the question: Can AI help organizations meet the GDPR’s compliance deadline and avoid penalties? After all, AI is all about handling and deriving insights from vast amounts of data, and GDPR demands that organizations comb through their databases for rafts of personal information that falls under GDPR’s purview. The answer is not only in the affirmative, but there are several significant instances where AI solutions to regulation compliance and governance are already on the high.
For example, Informatica is utilizing advances in artificial intelligence (AI) to help their organizations improve visibility and control over geographically dispersed data. It will provide companies with a holistic, intelligent, and automated approach to governance, for the challenges posed by GDPR.
AI interventions in Data Regulation Compliance and Governance
Data location Discovery and PII Management
It’s essential to learn the location of all customer data in all systems. The first action a company need to do is creating a risk assessment with a guess about what kind of data is likely to be requested how many requests might be expected. Locating all customer data and ensuring GDPR compliant management can be a daunting task, but there are options for automating those processes.
With AI, one can quite easily recognize concepts like ‘person names,’ which is important in this context. To find out how many documents you have that refer to persons (as opposed to companies), or to find out how many documents, social security numbers, phone numbers you have in any one repository, one can combine those analytics, and then begin to understand that the odds are that they have a lot of personal data in this repository, which provides a way to prioritize in the context of GDPR.
For example, M-Files uses Artificial Intelligence to streamline the process of locating and managing PII (personally identifiable information), which often resides in a host of different systems, network folders and other information silos, making it even more challenging for companies to control and protect it.
AI based data cataloguing
A solution that utilizes AI-based machine learning techniques to improve tracking and cataloging data across hybrid deployments can help companies do more accurate reporting while boosting overall efforts to achieve GDPR compliance. By automating the process of discovering and properly recording all types of data and data relationships, organizations can develop a comprehensive view of compliance-related data tucked away in non-traditional sources such as email, social media, and financial transactions – a near-impossible task using traditional solutions and manual processes.
Contextual Engines for Diversely Changing Data Environments
The GDPR changes how companies should look at storage of data. The risk of data getting compromised is increased based on how is stored, in how many different systems it’s stored, how many people are involved in that process, and how long it’s kept. Now that PII on job applications is regulated under GDPR, a company may want to routinely get rid of that data fairly quickly to avoid risk of data breach or audit. There are those kinds of procedural things that organizations will have to really think about.
There are instances where completely removing all data is impossible. You have to retain some data like billing records and there might be conflicting regulations, such as records retention laws. Now, if the citizen asks you to remove that, it’s going to add a lot of complexity to the process, in terms of understanding what data can be removed from the system and what cannot be removed. There will be conflicting situations where this regulation says something, and then you might have an Accounting Act or something in a local or state regulation that says something else.
This requires contextual engines built using AI that can be highly context aware based on the changing circumstances around the data and create a plan of how each data should be stored, managed and purged. This can also provide accurate insights on the levels of encryption and complex data storage techniques that need to be implemented for different data, thereby conserving hardware resources and increasing protection against malignant attacks and data breaches while minimizing risk of GDPR violations.
Working out the Kinks in AI led GDPR
GDPR aims to give EU citizens greater control over their personal data and to hold companies accountable on matters such as data use consent, data anonymization, breach notification, cross-border data transfer, and appointment of data protection officers. For example, organizations will have to honor individuals’ “right to be forgotten,” where applicable — fulfilling requests to delete information and providing proof that it was done. They must also obtain explicit, rather than implied, permission to gather data. And they are required to allow people to see their own data in a commonly readable format.
The system will undoubtedly work those issues out, but, in the meantime, companies should roll up their sleeves and take a thorough, systematic multi-step approach. The multi-step strategy should include:
Data. A comprehensive plan to document and categorize the personal data an organization has, where it came from, and who it is shared with.
Privacy notices. A review of privacy notices to align with new GDPR requirements.
Individuals’ rights. People have enhanced rights, such as the right to be forgotten, and new rights, such as data portability. This demands a check of procedures, processes, and data formats to ensure the new terms can be met.
Legal basis for processing personal data. Companies will need to document the legal basis for processing personal data, in privacy notices and other places.
Consent. Companies should review how they obtain and record consent, as they will be required to document it. Consent must be a positive indication; it cannot be inferred. An audit trail is necessary.
Children. There will be new safeguards for children’s data. Companies will need to establish systems to verify individuals’ ages and gather parental or guardian consent for data-processing activity.
Data breaches. New breach notification rules and new fines will affect many organizations, making it essential to understand how to detect, report, and investigate personal data breaches.
Privacy by design. A privacy by design and data minimization approach will become an express legal requirement. It’s important for organizations to plan how to meet the new terms.
Data protection officers. Organizations may need to designate a data protection officer and figure out who will take responsibility for compliance and how they will position the role.
Will GDPR Aligning Measures Be Necessarily Disruptive?
Many companies are going through significant changes as a result of the new regulations, and the efficiency and speed the AI-powered regulation compliance platform offer can significantly help streamline the entire process if companies want to ensure compliance.
Hence, there are plenty of challenges keeping CIOs up at night. By taking a more intelligence-driven approach to data discovery, preparation, management, and governance, the impending GDPR mandate doesn’t have to be one of them.