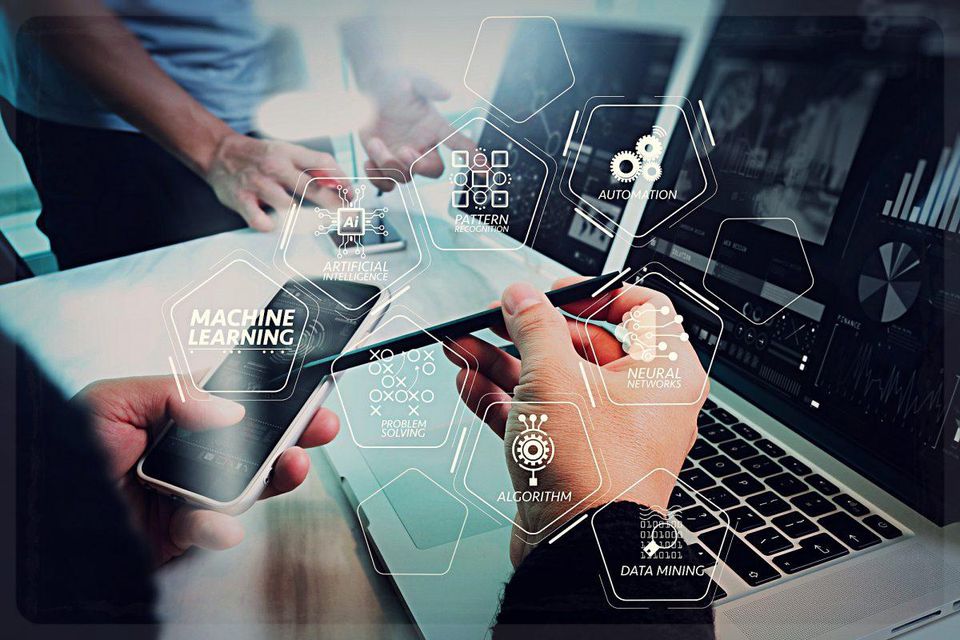
Reimagining Enterprise Decision-Making With Artificial Intelligence
Add Your Heading Text Here
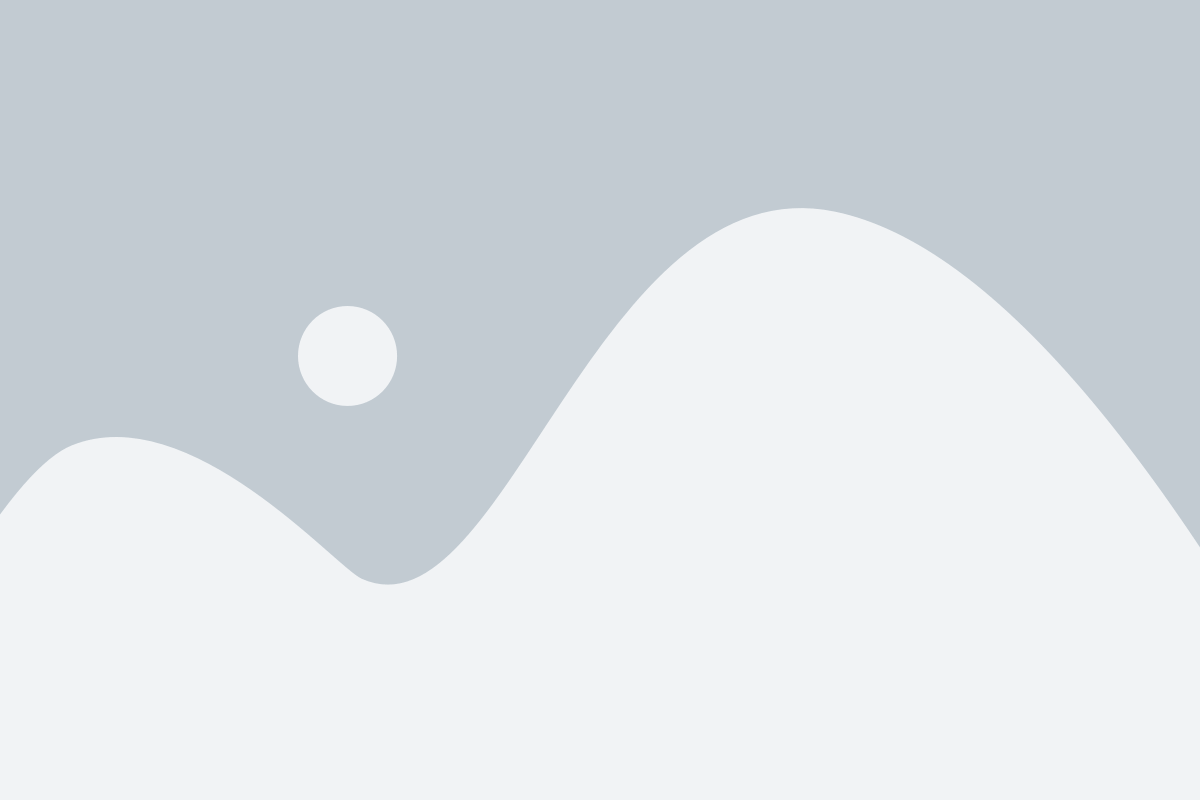
Artificial Intelligence will deliver revolutionary impact on how enterprises make decisions today. In the last few years alone, we have rapidly moved beyond heuristics-based decision-making to analytics-driven decision-support. In the VUCA phase, businesses globally are now pivoting to an AI-led, algorithm-augmented style of decision-making. With huge computing power and ever-increasing data storage and analytics prowess, we are entering a new paradigm, a probable and interesting scenario wherein, Artificial Intelligence will play a huge role in augmenting human intelligence and enabling decision-making with complete autonomy. The big hope is that this new paradigm will not only reduce human biases and errors that are common with heuristic decisions, but also reduce the time involved in making these critical decisions.
Here, I’ll attempt to focus on how we moved from simpler data driven decision-support to AI-powered decisions. The evolution of this technology has been breathtaking to behold and just might provide clues as to what we can expect in the future. Further, I’ll cover a few critical aspects that need to be inculcated by organizations on the AI transformation journey, and provide a few insightful cues that will make this journey exciting and fruitful.
Transformation of Decision-Making: From Analytics to AI
First, let us look at how we got here. Some truly pathbreaking events happened along the way while we were trying to make more accurate business decisions, leading us to reimagine how decisions will be made in the enterprise.
Organizations are Becoming Math Houses
With data deluge and digital detonation, combined with the appreciation of the fact that robust analytical capabilities lead to more informed decisions, we are witnessing AI savvy organizations rapidly maturing into ‘math houses.’ Data science – the ability to extract meaningful insights out of data has become de rigueur. Why? Because we now know that data, when seen in isolation, is inherently dumb. It is the ability to process this data and identify patterns and anomalies – using sophisticated algorithms and ensemble techniques – that makes all the difference. These self-intuitive algorithms are where real value resides – as they define the intelligence required to uncover insights and make smart recommendations. Organizations today are evolving into algorithm factories. There is a real understanding today that by enabling continuous advancement in mathematical algorithms, we can deliver consistent decisions based on prescribed as well as evolving business rules.
It is now an established reality that companies with robust mathematical capabilities possess a huge advantage over those that don’t. Indeed, it’s this math-house orientation that separates companies like Amazon and Google from the ones they leave in their wake, with their ability to understand their customers better, identify anomalies and recognize key patterns.
AI: From Predictive to Prescriptive
We saw a similar evolution in the age of analytics – wherein the science and value veered from descriptive analytics, providing diagnostics of past events to prescriptive analytics, helping see and shape the future. We are seeing a similar evolution in how AI gets leveraged in the enterprise and where its maximum value lies.
In early implementations, it was common to see AI as just a tool to predict and forecast future conditions, while accounting for the dynamism seen in the external environment. Today, AI-enabled decision-making is more prescriptive, with AI providing enterprises not just a look into the future, but also key diagnostics and suggestions on potential decision options and their payoffs. Such evolved applications of AI can help businesses make decisions that can potentially exploit more business opportunities, while averting potential threats much earlier.
Mr. Algorithm to Drive Decision Making
The culmination of this AI-era advancement would be the introduction of smart algorithms in every walk of life and business. Algorithms will become further mainstream leading to what will be the most sweeping business change since the industrial revolution. Organizations – those that already aren’t – will start developing a suite of algorithmic IP’s that will de-bias most enterprise decisions.
If Mr. Algorithm is going to drive most enterprise decisions of tomorrow, we need to create some checks and balances to ensure that it does not go awry. It is more critical today than ever before that the algorithmic suite developed by enterprises has a strong grounding in ethics and can handle situations appropriately for which explicit training may not have been provided.
How to Enable this AI Era of Change
Ushering into an AI-centric era of decision-making will require organizational transformation from business, cultural and technical standpoints. The following facets will be the enablers of this change:
Developing an Engineering Mindset
Instrumenting AI in the enterprise requires a combination of data scientists and computer scientists. As AI matures in the enterprise, the users, use cases and data will increase exponentially. To deliver impactful AI applications, scale and extensibility is critically important. This is where having an engineering mindset comes in. Imbibing an engineering mindset will help standardize the use of these applications while ensuring that they are scalable and extensible.
Learning, Unlearning, Relearning
The other critical aspect to a culture where AI can thrive is creating an environment supporting continuous unlearning and relearning. AI can succeed if the people developing and operating it are rewarded for continuous experimentation and exploration. And just like AI, people should be encouraged to incorporate feedback loops and learn continuously. As technology matures it’s important that the existing workforce keeps up. For one, it’s critical that the knowledge of algorithm theory, applied math alongside training on AI library and developer tools, is imparted into the workforce – and is continuously updated to reflect new breakthroughs in this space.
Embedding Design-Thinking and Behavioral Science at the Center of this Transformation
Finally, given the nature of AI applications, it’s critical that they are consumed voraciously. User input very often activates the learning cycles of artificial intelligence applications. To ensure high usage of these applications, it’s very important that we put the user at the center while designing these applications. This is where the application of behavioral sciences and human-centered design will deliver impact. By imparting empathy in these applications for the user, we will be able to design better and more useful AI applications.
As we augment decision-making with algorithmic, AI-centered systems and platforms – the big expectation is that they will bring untold efficiencies in terms of cost, alongside improvement in the speed and quality with which decisions get made. It’s time to reimagine and deliver on enterprise decision-making that is increasingly shaped through artificial intelligence. These aspects – how the AI is progressing and how to exploit its potential are of paramount importance to keep in mind for an AI transformation.
Related Posts
AIQRATIONS
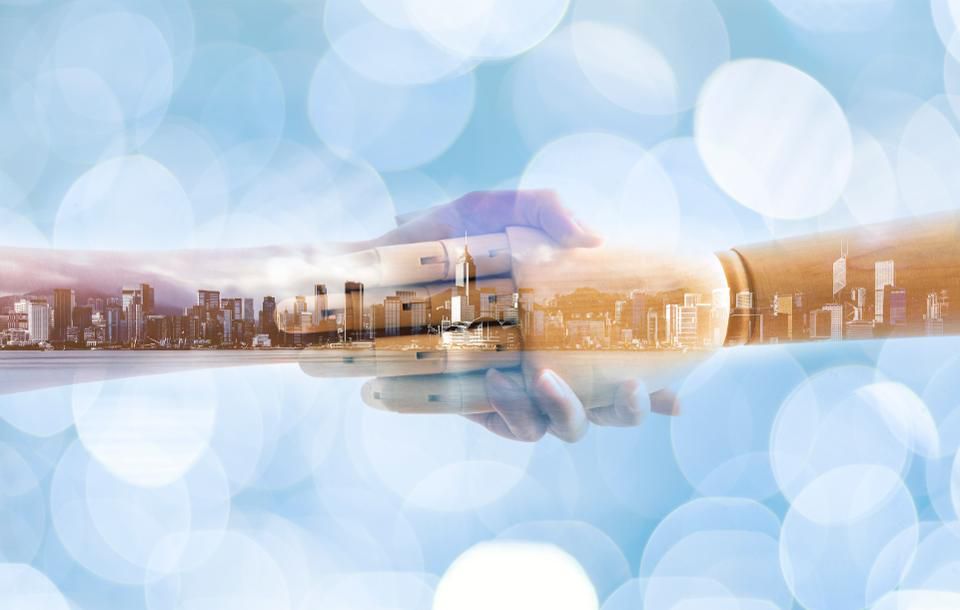
Mapping the AI Transformation Journey In Your Organization
Add Your Heading Text Here
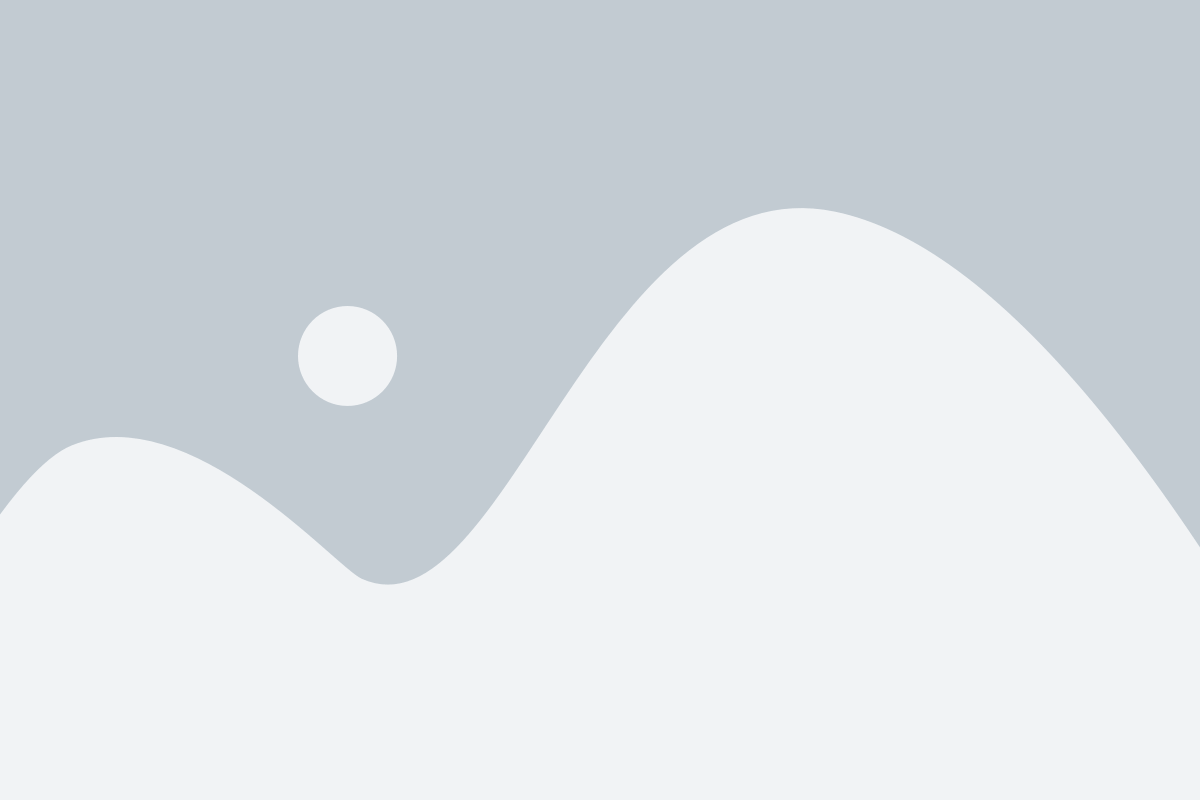
We are well and truly in the midst of the AI revolution. Research houses, academicians, think-tanks, business and technology leaders all agree upon the significant value waiting to be unlocked through the positive and progressive use of Artificial Intelligence – by re-engineering the old and envisioning the new. According to a research by Gartner, organizations using cognitive ergonomics and system design in new artificial intelligence projects will achieve long term success four times more often than others. Citing research by the MIT Center for Digital Business, from a competitive standpoint, companies that embrace digital transformation are 26 percent more profitable than their average industry competitors and enjoy a 12 percent higher market valuation.
The writing is on the wall. Intelligent business interventions made through AI will, to a large extent, define if your business will be an industry leader or a laggard tomorrow. And with that end in mind, businesses are rapidly changing their mindset and approach to AI – from topical experiments performed by forward-thinking business units, to more of a strategic mandate for enabling competitive differentiation. Businesses realize that for truly unlocking business value, they need to not only weave AI into the fabric of their enterprise, but also operationalize it – with the right personnel and change management initiatives. Given that AI can bring both cost efficiencies to business as well as potentially new revenue streams, businesses today are exploring an ‘AI Transformation’ – moving the dial on what is truly possible through a business model, engineered around AI. To enable your organization to do so, here are three powerful ideas to map the AI Transformation journey of your business.
Ensure Enterprise Readiness to Build and Adopt AI
The first step in the journey to AI Transformation for your enterprise is to understand and address if there are any disparities between your vision for AI and the ability of your organization to follow through with it. To that end, it is important to assess just how ready your enterprise is, in its current state, to build, deploy, adopt and benefit from AI-centric solutions. Ideas for AI Transformation need to be communicated clearly and grounded in the realities of organizational capabilities. When they are not, even the best intentions can go awry.
To do so, it is critical that business leaders measure their current AI maturity and assess the availability of internal skills. This will enable you to baseline just how empowered your current workforce is to develop industry-leading AI solutions. Once such a baseline is established on workforce readiness for building and adopting AI-led solutions, organizations need to start improving on these metrics – through internal trainings and external capability augmentation.
By developing this baseline score for AI readiness – organizations can have an objective view of where they are, how far they need to go and what the potential milestones to be achieved are in the journey to AI Transformation. This sort of pre-survey, combined with relevant training and assessment can help organizations craft a relevant roadmap with realistic timelines, as well as concrete actionables.
Build an AI ‘Win Team’
An AI Transformation is not unlike an extremely complex business re-engineering exercise. It entails massive changes – from the way you do business to how you run internal processes and staff multiple business units. Not only is it important to reskill a huge section of the workforce, there is also an important aspect of enabling change management to reinforce the importance of an AI-centric mindset.
To overcome this challenge, enterprises need to foster the consensus and engagement of a ‘win-team.’ This win-team would typically comprise functional and technical leaders who would be responsible for enabling the AI Transformation within their business units – from orienting the employees to the new mindset and ensuring capability readiness for the tasks at hand. On one hand, functional leaders can help their teams identify the processes that can be re-imagined using AI and manage resistance to change. On the other hand, technical leaders would lead the solutioning of technical components, while setting the training priorities and calendars for the workforce.
On change management, enterprises need employees to clearly appreciate the topline and bottomline benefits of an AI Transformation and focus towards enabling it. Employees stand to benefit themselves – as the professional benefits of making this transformation will accrue for their future. To further explore how companies can reduce the defensiveness in implementing AI-led processes further, they could also set innovation objectives for stakeholders as part of their performance metrics. Doing this will help create a strong alignment between individual, team and organizational objectives. A key aspect of AI transformation is ensuring large-scale adoption and usage of AI-powered solutions. AI applications typically fare better with every incremental user feedback and enriched data sources. Adoption and continuous use is a key parameter for the success of this transformation.
Integrated Business Processes over Siloed Business Functions
For years, the view of technology transformation and procurement has been of one that happens at a department / functional level – HR teams buy talent management software, finance teams sanction the purchase of accounting software, and CRMs get implemented to aid the efforts of sales teams. While this serves small technology initiatives, a sea-change is required for progressing an AI Transformation. To foster this, enterprises need to make a shift from a siloed, function-centric mindset to an integrated, process-centric mindset.
This is because AI use cases can often span multiple business units and functions, while tapping into multiple data sources for providing cross-team value, seamlessly. The very nature of AI deployments thus requires a process-centric view, with a strong consensus and buy-in from multiple stakeholders. Furthermore, the budget for purchasing AI services / applications is likely to come from the allocations of multiple beneficiaries across functions. This makes it all the more imperative that enterprises deprioritize functions in favor of processes.
An AI Transformation is doubtless the most strategic subject to be tackled by organizations today. Successful transformations will ensure enterprises go beyond mere automation and cost-cutting strategies and unveil previously unseen business and revenue opportunities. It is also extremely important to consider the role of digitization in building a new technology infrastructure that is AI-ready – possibly decentralized, cloud-based and highly available. There is now an urgent need for business leaders to have more than just a superficial understanding of AI and its successes. They will now be tasked with building and delivering a concrete, value-oriented roadmap for enabling a key transformation in the history of their organizations.
Related Posts
AIQRATIONS
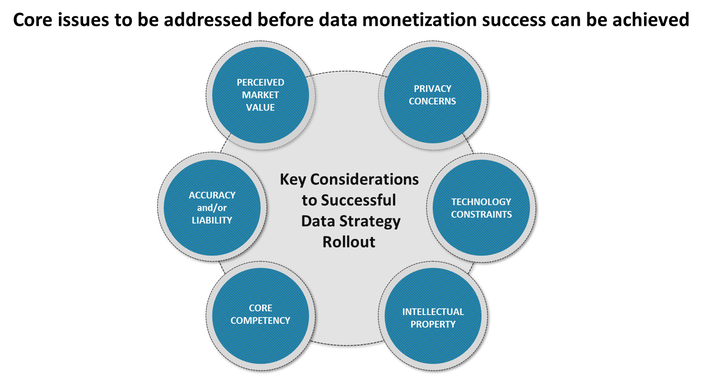
Building a Robust Data Strategy Roadmap – Part III
Add Your Heading Text Here
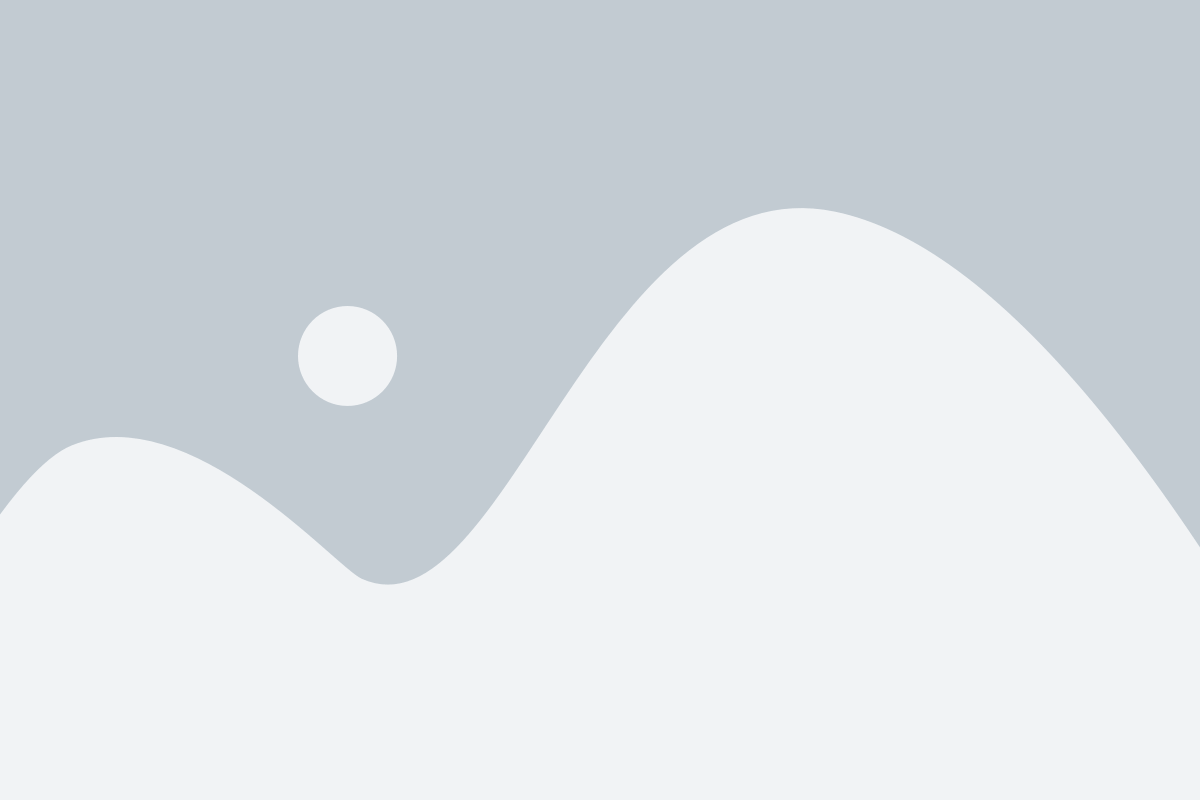
In continuation to my last article on Building a Robust Data Strategy, let me meaningfully conclude it by highlighting some of the core issues which need to be addressed before data monetization could really be called our as a success and ROI is achieved.
Privacy Concerns
Company needs to have the implicit and/or explicit statutory or legal right, or the ethical right, to divulge private consumer data – either personalized or depersonalized, individualized or at an aggregated level. Especially in industries where regulatory bodies have a heavy clout over what data is being used to cull out actionable insights or even the data flow within or beyond the walls of the organizations. Numerous articles, reports & surveys have highlighted how crucial is for businesses to operate within the ethical boundaries of data gathering or dissemination. Leave no stone unturned to see what policies/restrictions/guidelines are in place for the industry you operate in, how easy/difficult is to access data, and what are customer or end user reactions. You definitely do not intend to burn bridges with your existing customer base or repel away new prospects. Legal actions can be fatal to business at times. Be doubly sure what you are up for!
Technology Constraints
Do have a thorough understanding of the technological or hardware-related considerations to implement the strategy chosen to monetize the data. At times, organizations don’t have the requisite resources to execute on their strategy, may be because that’s not their core area of operation or it’s happening in silo’es across the organization which the business unit in question is not privy to. A complete landscaping exercise to understand the current state of business, what’s new in the market & what the competition is up to, what’s the future state & a step-by-step roadmap to mature technological prowess. In many cases, businesses hire external consultants or seek handholding by analytics service providers who have the requisite experience in recommending about the gaps & even executing on filling those. A thorough detailed analysis (but not analysis-paralysis) is crucial to the overall success.
Intellectual Property
At times, organizations sitting on huge pile of valuable data choose to make it available in the market (as another viable revenue model to monetize data). How much data to sell and how to determine costs vs. benefits in putting valuable data on the open market should be thought through. Be privy to the pros & cons of each approach & choose your business model accordingly.
Core Competency
Depending on its core competency, organization needs to identify at which level it wants to monetize the data in the data value chain. Data at each & every touchpoint in the value chain may have its own peculiar problems (missing data, incorrect data etc) and not all of it may be relevant. If your differentiator is “speedy delivery” of goods to your customers, focus on picking the right data sets across the value chain which helps streamlining operations, optimize inventory & transit time. Know what you are best at or what you are known for in the market and harness data capabilities to strengthen your business on that front.
Data Accuracy and/or Liability
Potential problems with inaccurate or directly or indirectly regulated data insights or products hitting the market place. Make sure that data assimilation, aggregation & cleansing exercise is robust enough to ensure the analysis/insights being generated out of it have a high probability of giving the right sense of direction to the business. At times, over-ambitious expectations or poor data quality can directly impact the quality of the outcomes. Garbage-in Garbage-out is the mantra & business managers should perfectly understand the gaps in the data & be cautious before making any solid commitments.
Perceived Market Value
For larger market opportunities, it is likely that an organization would want to play at the higher level in the data value chain. Umpteen times that completely derails the whole Analytics ROI & data monetization exercise. Focus should be specifically on business model(s) used to monetize the data than otherwise.
All the aforementioned considerations should set a good pretext to the data monetization exercise and may be the key to unlocking true value from data strategy initiative. In my subsequent edition, I shall bring to light the “Analytics Centre of Excellence” concept & how can organizations setup a full-fledged Analytics unit to deliver insights to departmants/LOB’s/functions across the business and also serve as a backbone to building a data-driven organization of the future. Stay tuned !
Related Posts
AIQRATIONS
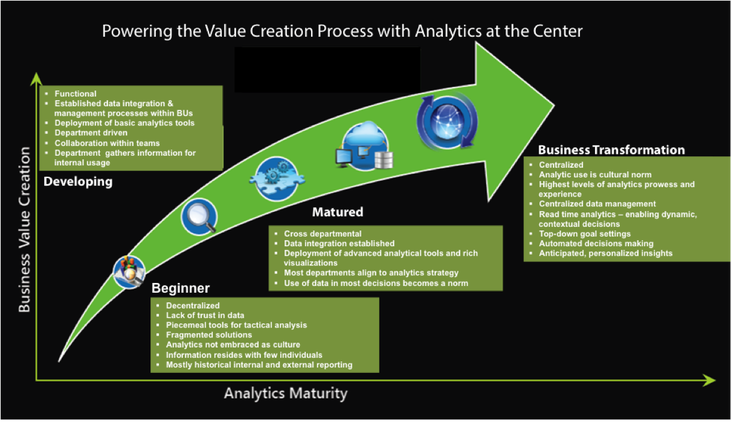
Building a Robust Data Strategy Roadmap – Part II
Add Your Heading Text Here
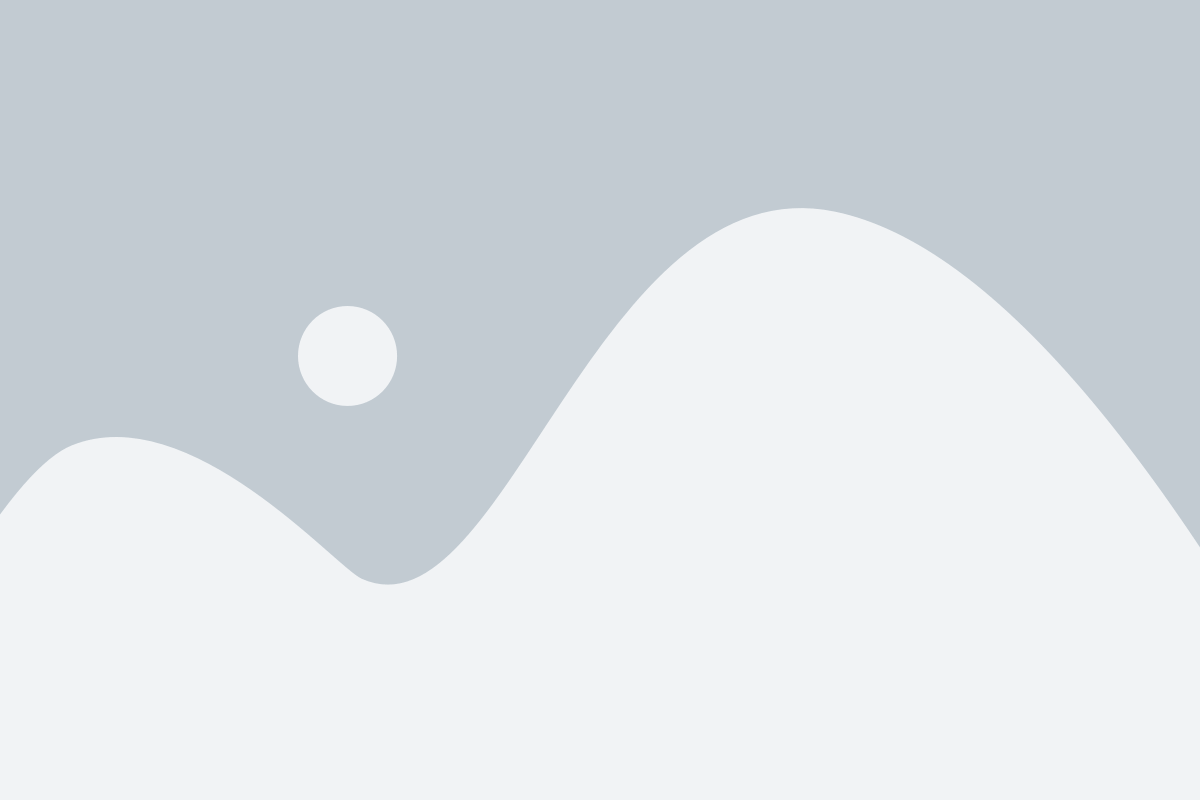
Unarguably, data and technology is truly redefining & rehashing the way companies do business. Organizations have always had data, which they have utilized to run their businesses more efficiently but recent developments have transformed the way data is utilized by such organizations.
In today’s disruptive economic environment, all leaders are vying for identifying new revenue streams and identifying existing value streams inside the organization especially data. This is where the concept of crafting a Robust Data Strategy comes in, how do we make most of the Dark Data ? Data is now being looked as an asset and business models are now being build around this vast value pool which is hidden inside the data being stored. Enterprises are now anticipating future needs based on preference insights culled out from past & present data. They are creating new products and services in tune with what their customers exactly seek. They are lending an ear to all suggestions/recommendations/feedback shared and also responding to queries/concerns in real time. They are doing it all with data and analytics.
While many companies are becoming aware of the opportunities embedded in their enterprise data, only a few have developed active strategies to monetize it successfully. Data Strategy requires companies to not only understand their data, but also to uncover gaps and evaluate suitable business model(s) for appropriately monetizing the enterprise data. To evaluate their respective monetization opportunities in a more informed and results-driven manner, companies need to assess the value of enterprise data, determine how best to maximize its potential and figure out how to get the data to the market efficiently.
Four Stages to Analytics Sophistication
Based on the current state of data affairs, any organization can be categorized as a beginner, developing, matured or leader. In the initial stages of transformation, organization typically
lacks synergies due to silo’ed efforts, is less agile and more prone to errors, with perennial data quality concerns. As they mature to be leaders in the Analytics space, data sits at the heart of business, with increasingly automated, instant, accurate and seamless data driven decision-making.
- Beginner: Basic infrastructure and tools, proliferation of dashboards and reports
- Developing: Building tools and processes for historical as well as deep diving analysis to gain some insights for future actions
- Matured: Organization adoption of advanced analytical capabilities to predict future outcomes
- Business Transformation or Leader: Centralized analytics focus with capabilities to anticipate future and act in a data driven manner
Time’s ripe to ride on the Data & Analytics wave
Enterprises capture a lot of data, most of which is often overlooked. With reducing costs of capturing and storing data, increasing data analysis capabilities and superior analytical technologies available, enterprises have started to recognize data as one of their most valuable assets. In the few years, enterprises who lead the way in reorienting their approach, initiating enterprise wide data-led transformations and effectively monetizing their data are expected to be in the forefront. Typical market forces driving widespread adoption of Analytics are:
Technological Advancement
- Technology advancement has facilitated real time data analysis and personalized communication
- Big data technologies, cloud computing, machine intelligence and other advancements etc. have made analysis simpler & efficient
Rise of Consumerism
- Influx of more demanding consumers will force a wave of change
- Consumer engagement and experience management are key levers to success
Data Explosion
- Daily volume of data being captured increasing rapidly
- Cost of storing data decreasing massively
- Recognition of amount of under-utilized data that can be used to derive additional value
Increasing importance of Analytics & BI
- Business Intelligence and Analytics becoming an integral part of organization’s decision making
Economic Pressures
- Pressure on profit margins are forcing increased focus on efficiency and cost reduction
- Increasing competitive pressure
Is your Data truly worth it?
How much business value can be created via data on which organizations are sitting on depends primarily on the following factors & to an extent determines the success of any Analytics initiative.
Predict Behaviour (Patterns)
Enterprise data should be detailed enough to build a successful data monetization strategy. E.g. Customer data should be detailed enough to be able to predict customer behavior, patterns etc.
Size of the Ecosystem
Businesses with high volume, large breadth of data have the ability to generate highest value from the data. Companies with national or global scale can easily establish market view, which makes it more meaningful and valuable
Accessibility and Actionable
Data becomes valuable only if its rich, actionable and accessible. Structured, & readily scalable data makes the process of monetization simpler and efficient, providing higher potential for data monetization
Customer Identification (Granularity)
Data becomes valuable only if it is granular enough to be able to identify the end user/ customer. Ability to identify/ profile customers helps in expanding the range of products and servives that can be offered
Uniqueness
Uniqueness of the enterprise data is extremely valuable. It makes the products/services offered by the enterprise exclusive to the enterprise, sustainable differentiation which most organization yearn for
Stages to Data Maturity
Based on maturity of organization’s data, it can take a call what kind of a player it wants to be in the market – a “data seller” or a “full services provider”.
Raw Data
- Selling raw unprocessed data to outside stakeholders
- Companies with rich pool of high quality raw data can onsell such data with little investment required
E.g. – Pharma related data or even NASDAQ’s “Data on Demand” service to its ecosystem of partners in the capital markets
Processed Data
- Companies collect and integrate data from multiple sources
- Data is processed, stored and leveraged in summary form
- Secure capture and transport of data
- Proper storage and management of data using a data platform
E.g. Card Advisory companies provide processed data to merchants and/ or use it for improving its operational efficiency
Business Intelligence/ Predictive Insights
- Tools and technologies such as data mining, predictive modeling and analytics convert data into insights
- Insights are made available to the stakeholders (both internal and external) to drive business decisions
E.g. Wal-Mart segments its customers into three primary groups based on purchasing patterns to spur growth
Products & Solutions Implementation
- Data-driven interactions with end users
- APIs and ability for companies to access platform and data to build comprehensive products and solutions
- Companies use the intelligence to improve product and solutions offering portfolio
E.g. Tesco bank uses Clubcard customer data to identify customer needs and creates new personalized offers
Key Elements to Designing a Robust Data Strategy
Unravel Customer Needs
- Continually understand the customer needs to unearth customer requirements and preferences
- Understanding the delivery and integration models that clients require in order to benefit from enhancements
- Create a business model which fits into the core competency and create offerings which fir into client platforms and applications
- Invest in continuous learning and management of customers’ unmet needs ranging from enhancements to new products/ solutions
Decrypting the Enterprise Data
- Understand the enterprise data captured across all business lines and develop an enterprise wide nomenclature for the same
- Identify and map data and analytics services across business units to understand what types of capabilities can be leveraged to build new products and services using the appropriate business model
Gauging the Market Potential
- Calculate the market potential for the various opportunities identified
- Estimate the revenue potential, internal rate of return, investment required, cost reduction, efficiency etc. for the process
- Understand the key competition, factor in macro and micro factor which can affect the marketplace demand
- Seek out opportunities to enhance the core business or develop new products and services.
Deciphering the Value Chain
- Develop insights into partners and competitors across the value chain including upstream suppliers, data partners etc.
- Identify the new opportunities that can be available across the value chain
- Create a comprehensive view of the data ecosystem
Enhance the existing infrastructure
- Develop a sophisticated yet flexible architecture, suitable technology and applications which can help unlock the value that the opportunities might presents
- Put in place a data infrastructure that can provide the necessary foundation to enable the organization to unlock the value of data assets
The crux of the matter is that with the huge amount of data available with the enterprise’s in today’s competitive and converging business environment, they should start looking for market opportunities leveraging the data available with them. Most of the enterprises still do not consider data as an asset which they can monetize if they choose the correct business strategy and build the required capabilities. Enterprises can not only make better use of their internal data to enhance the current product and services portfolio, it can also provide new insights into the value chain and could transform the enterprise, unleashing a whole new set of products and services for the customers.
By utilizing internal data with external data, powerful generation of high margin solutions can be developed which can transform an entire organization which possesses enormous revenue potential. Done properly, data ecosystems can fund the transformation, create value for customers, and build long lasting relationships with other partners firms, 3rd party vendors & suppliers. But to ensure the true value of data is being monetized by the enterprise, it is essential that it follows a streamlined process to identify the most suitable business model(s) taking into account all constraints which the process might need addressing.
Related Posts
AIQRATIONS

Journey to Analytics Transformation is a Marathon, not a Sprint
Add Your Heading Text Here
The environment today in which organizations across the globe operate in continues to grow more complex with every passing second. With innumerable choices to make, relentless pressure to deliver consistently in a time bound manner and rationalizing profit margins, the decision-making process becomes yet more daunting and convoluted. Unarguably, Analytics consistently delivers significant value – from strategic to tactical, managing top-line to bottom-line – to the organizations and business executives who use it. But senior leaders are still grappling with the question whether they are truly harnessing the fullest value from the massive amounts of data at their disposal; “dark data” sitting within their organizations in silos. Advent of newer technologies are making data collection faster than ever before, and it may seem like an overwhelming task to turn data into insights and answers that drive the strategic imperative. Storage & computational capacities have grown by leaps and bounds, opening up doors to intelligent decision-making for varied business stakeholders, yet many organizations are still looking for better ways to obtain value from their data and compete more effectively in the marketplace. The fundamental question about how best to achieve value still boggles most of the leaders.
Is competition equipped to obtain more incisive, timely and valuable insights? Are they catching the pulse of the global economy, the marketplace, the customers & the industry much better than what we do? Do they have better foresight to unravel what happened and why it happened in the past, and are they in a much better shape to decipher their current and future state to take actions closely aligned to market realities for optimal results? What do these analytically mature organizations do differently and what sets them apart from the crowd? Have we gotten our approach and data strategy right? Have we empowered our workforce enough to effectively leverage our Analytics insights? Has it seeped in appropriately to all the downstream decision makers? Plentiful questions abound and more often than not these perennial doubts do keep bothering the senior leadership, “are we doing it the right way”?
And not so astonishingly, most of the well thought through Analytics initiatives and robust Analytics transformation journeys go for a complete toss or fail to deliver value. From lack of senior leadership buy-in to Analytics value, advanced analytics applications not being put to best of their use, or a proliferation of analytics applications that fail to deliver a unified, solid strategic direction, many companies are falling short of the value analytics can provide. No wonder leaders end up losing patience and Analytics remains to be an elusive concept to most, putting up barriers to widespread Analytics adoption at the very beginning itself. Consequently, the shutters are pulled down much before the Analytics champions get a chance to showcase even marginal business impact. All the tall claims mutually agreed upon just end up being farfetched dreams !
When businesses venture into the Analytics space and think about transforming their organization into an Analytics think tank, it’s no easy ask. The way expectations are set initially, that analytics-driven insights to be consumed in a manner that triggers new actions across the organization, they must be closely linked to business strategy, easy for end-users to understand and embedded into organizational processes so that action can be taken at the right time. Now just mulling over what I just mentioned here, it’s a mammoth task in itself with too many ifs and buts. Just imagine the complexity we are dealing with. Let’s quickly take a high-level perspective of what kind of challenges do most organizations stumble upon, and understand the critical ingredients to a perfect Analytics recipe are:
- Right problem statement where analytics could have a strong play
- Right Data to begin with
- A strong team of Analytics professionals (Data Cleansers, Data Visualizers, Modelers etc) with a right blend of skill sets
- Senior leadership buy-in and requisite budgets
- Clearing other internal toll gates
- Program review framework to track progress & suggest realignment
- And the biggest of them all, a drastic shift in the mindsets of business users consuming these insights, how to make the transition process seamless
Aforementioned list is just a flavor of typical roadblocks your Analytics initiative could run up against and I haven’t even gotten into the finer details of spending wasteful time on Analytics tools/techniques which may not fit the bill. In a nutshell, the pitfalls are too many and as advocates of Analytics, it’s imperative for us to convey the same picture to the right set of business stakeholders in the organization that Analytics may take time to deliver value. And the wait could get even longer if a structured and methodical approach is not followed here. Undoubtedly, it requires painstaking focus on the way insights are infused into everything from manufacturing and new product development to credit approvals and call center interactions.
So what truly makes certain companies so successful with analytics initiatives while others fail to get the results they are looking for? Analytically mature organizations approach business operations very differently than their peers do. Disproportionately analytic leaders are having management support and mandate for analytics throughout the organization, including top-down diktat for analytics, sponsors and champions; they are open to breeding change and accept new ideas; having a focused approach to customer experience driven by analytics; and heavily use analytics to identify and address strategic threats to the organization. On a specific note, they deploy analytics across widest range of decisions, be it large or small. There’s a high correlation between organizational performance and analytics-driven management, and Analytics forms the key to all performance related aspects, be it seeking growth, efficiency or competitive differentiation.
The path to realizing value out your Analytics efforts and investments is a long-drawn process. But still, how can organizations bring down the time-to-value for analytics? How can you avoid common pitfalls which may derail the Analytics intent your organization set out with? Analytics value creation can be achieved during the initial budding phases on the path to analytics sophistication. Contrarian to common assumptions here, it doesn’t require the presence of perfect data or a full-scale organizational transformation and small pilots to convey value should suffice. After initial successes, snowballing effect shall come to rescue.
Think Big!
Does targeting the biggest challenge of all imply setting stage for big failures? Not always! Remember higher stakes command top management attention, appropriate investment, attracts best of the breed talent and incite action. Hence, focusing on the biggest and highest-value opportunities may not be that bad an idea. Don’t pick insurmountable problems though and ensure focus on achievable steps.
Framing the Right Questions
More often than not organizations are tempted to start the data assimilation process, way before they kick-start their analysis. A lot of valuable time & effort is spent in aggregating this data across various departmental silos, cleansing, harmonization, conversion etc. leaving little time for actually thinking through the intent of analyzing the data, and uncovering potential uses. To get optimal results, the idea should be start carving out the insights and questions which need to be answered to meet the bigger business objective rather than jumping on getting the data pieces together. Such an exercise at times can illuminate gaps in the existing data infrastructure and business-as-usual processes. Data-first strategy could mean lot of unintended rework, approaching a dead end towards the later stages and may be budget overruns in case additional resources are to be pooled in.
Easing out Information Consumption
In the end, the consumers of insights are the business users. In that case, the Analytics team may have to don the hat of a business stakeholder and be able to represent information in a meaningful way which sees direct applicability to their audience. Ability to convey the story in effective manner, figuring out better ways to communicate complex insights is crucial so that users can quickly absorb the meaning of the data and take appropriate actions. Leveraging numerous visualization and reporting tools can simplify insights, make results more comprehensible & easier to act upon. They can transform numbers into information and insights that can be readily put to use, versus having to rely on ambiguous interpretations or leaving them unused due to uncertainty about how to act.
Embedding insights at the right touch points & delivering value
With the proliferation of analytics applications and tools, embedding information into existing business processes, workflows etc is a lot more streamlined. For e.g. insights from your location analytics tools can easily be superimposed over existing maps-based applications deployed at the Salesforce level to help them plan out their routes optimally. Oil exploration companies can easily embed the production or pipeline information into their existing enterprise-level systems for informed decision-making around the next best drilling site. Such innovative ways have to be through to make consumption of complex Analytics insights a lot easier across the organization. Point to note here is that, putting together a new system for consuming Analytics insights could mean a drastic cultural shift for the business users, and high resistance to change in such cases could lead to failures. If somehow these insights could be seamlessly infused into existing apps/tools or processes would mean smoother transitioning & better outcomes due to increased adherence.
Slow & Steady Scale-up
As the business mature on the analytics front over time, data-driven decision making slowly starts spreading its wings across the organization. And as the Analytics experience and usage grows, the value analytics can deliver grows multi-folds, enabling business benefits to accrue much faster than originally imagined. Not all functions/LOB’s/departments are at an equipotential when it comes to Analytics maturity. Business functions like finance and supply chain are inherently data intensive and are often where analytics first makes its mark. Harping on the early successes, organizations can begin expanding analytics reach to other units. Crafting reusable assets which could be repurposed, with slight modifications by other units could speed up the transformation process.
Crafting a Data Agenda
Dealing with disparate sources of information, sitting in silos across the organization, in varying formats & structures, and churning out divergent insights can be a daunting task and also convey a highly convoluted, incomprehensible picture at times. The data agenda should provide a high-level roadmap that aligns business needs to growth in analytics sophistication as the organization matures along the way. It should be flexible enough to keep pace with the changing business priorities and must have clearly stated guidelines or frameworks to aid transforming data into a strategic asset; data which is integrated, consistent and dependable enough. Data quality & effective governance processes can be set up to ensure seamless assimilation and healthiness of data being put to greater use. Even though, you tread down the analytics path with the biggest organizational challenge, start putting the data pieces together which deliver insights & get you closer to the actual solution, but then how this data foundation crafted aligns with the overall data agenda is crucial. Comprehensiveness of the data agenda builds up the requisite momentum to deliver meaningful nuggets of information across disparate systems organization-wide. Eventually the data agenda is at the very core of any analytics initiative, ensuring the “right piece of information” reaches out to the “right stakeholders”, with the “right set of business priorities” at the “right time”.
To expedite the process to path to value, start by identifying big business issues which would garner the right management attention and resources for execution, carefully cherry-pick challenges for which you see Analytics as the key enabler, taking into account the foreseeable changes in the operating ecosystem as you go along. Riding on assets capabilities already inherent to the organization, the core strengths, is the key and creating reusable components can help scale up fast to increase reach. And the most important of all, keep embedding insights generated at every step into existing business processes to deliver continuous business impact and monitor change.
Related Posts
AIQRATIONS
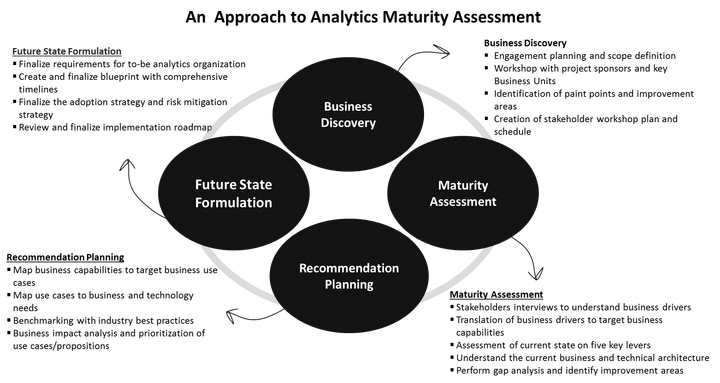
Building a Robust Data Strategy Roadmap – Part I
Add Your Heading Text Here
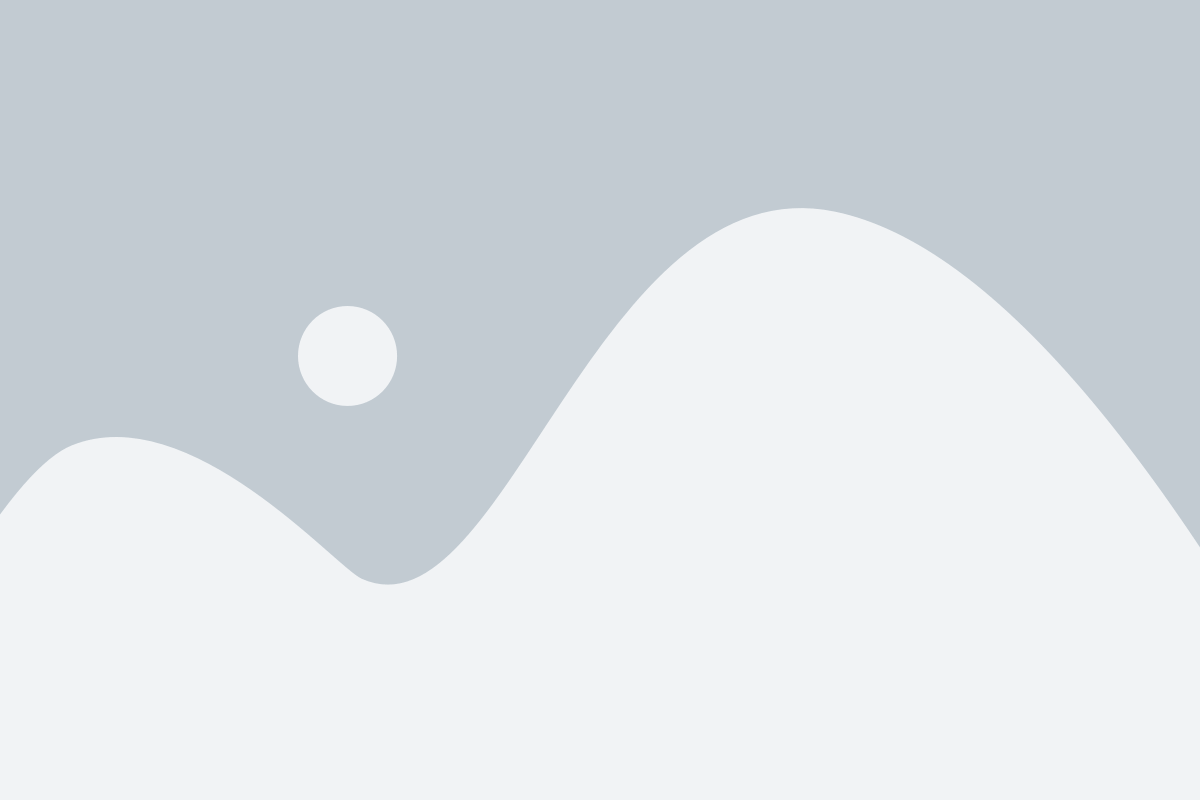
Imagine a Lamborghini or Ferrari or any car of your choice for that matter, with a fantastic engine parked in the garage, you’d love to get your hands on the wheel, wouldn’t you? After all, it makes no sense to invest so heavily in such a mean machine and yet leave it hidden under a wrap, right? Think of the Data sitting inside the organizations as the great, potential “engine”; an invaluable asset which remains elusive most of the times, well, pretty much in storage, as most CXO’s agree that they’re not doing nearly enough to maximize the use of effective analytics to unleash the potential of dark data they are sitting on. The future belongs to those organizations that effectively employ analytics to understand their markets, customers and operations. Forward-thinking organizations recognize that data is becoming the new source of competitive advantage, and organizations are re-thinking value creation and investing in new analytics infrastructure. In fact, data analytics is routinely cited by CXO’s as among their top one or two priorities year after year. Companies are already making use of data to advance a variety of business goals and to help consumers. Few of the leading organizations who are pioneering in this space of harnessing data for business value (like Facebook, Google, LinkedIn, and Amazon have) shown the world what is possible when data is used to its truest potential in cutting-edge ways, and the idea that enterprises must recommit themselves to become data-driven is now a widely held notion. While many companies have excelled in the use of data analytics and predictive modeling, data-driven decision-making is no slam-dunk. Several companies are struggling to make data-driven decision-making part of their DNA.
Across industries, “Big Data” and Analytics are helping businesses to become smarter, more productive, and better at making predictions. Organizations today are collecting increasing amounts of disparate data. In fact, they are collecting more data than they can manage or analyze; which means most of the data being collected is underutilized. Yet, organizations understand and know that data and data analysis can provide an important strategic competitive advantage. Businesses today are under extreme pressure and face significant challenges to reduce overall costs, improve outcomes, adapt to new technologies, comply with strict regulatory restrictions and face the ever increasing power of the consumer. Organizations agree that building analytics competency can and will drive improved delivery outcomes, quality and cost leveraging the “power” of data. Best in class organizations are adopting analytics to drive decision making, improve outcomes, increase member loyalty/ retention, reduce unnecessary costs, and increase accountability.
Organizations that know where they stand on the analytics maturity continuum are better prepared to turn challenges into opportunities. By performing their current state assessment and building an enterprise value roadmap for analytics adoption, organizations can define the “best way forward” to completely engage a data-driven culture. Tapping this potential for your organization begins with shaping a plan. You have to set a strategy; draw a detailed road map for investing in assets such as technology, tools, and data sets; and tackle the intrinsic challenges of securing leadership buy-in, reinventing processes, and changing organizational behavior. Analytics is not just about generating insights, but getting those insights to the right people. To sustain the long-term success of data-driven innovation, it is necessary to continually revise one’s analytical approach in order to generate insights that lead to new innovation and competitive advantage.
The first stepping stone in the direction or crafting a robust data strategy starts with doing a comprehensive Analytics Maturity Assessment exercise. Inherent question which crosses our minds is, “Why Analytics Maturity Assessment”?
Need for Analytics Maturity Assessment
- The most critical aspect to any organization is to leverage true benefit of data, decipher where they are today, where they’ve been in the past, the progression curve and a direction where they intend to go in the future based on data/information available at their disposal
- By leveraging maturity assessment framework, organizations can measure the current maturity of the data (how good is it to perform analysis) and the overall analytics program in an objective way across various dimensions that are key to deriving accelerated value from data
- Uncover how their data efforts stand in comparison to those of their peers in order to ensure best-in-class insight and support, and ensure we are in tune with the contemporary market trends
- The assessment shall also render guidance to companies at the cusp of starting their data journey, by helping them understand industry best practices used by companies across geos, of different sizes & even from industries that are more mature in their deployments
- After performing the benchmark study, organizations will be able to quantify the maturity of their deployment in an objective way, understand the progress, and identify what it takes to graduate to the next level of maturity
Key Challenges impeding Analytics Proliferation
Organizations want to leverage data analytics but face challenges while trying to formulate a strategy around it due to:
- Lack of Vision
- Business leadership doesn’t have a defined corporate strategy to drive data driven culture
- No vision on how to embed analytics into the decision making process
- Disparate Data Sources
- Data stored in silos across departments
- Many different types of data sources
- Large amount of data generated
- Talent Crunch
- Lack of people with Domain knowledge as well as business analytics expertise
- Lack of people with knowledge of varied data types and tools to integrate, process and develop insights
- Resource Availability
- Lack of resources to quickly turn around on-demand analytics
- Low bandwidth with IT resources to provide near real time information
Maturity Assessment Guiding Principles
In order to ensure your Analytics Maturity Assessment exercise in worth the time & effort, a few guiding principles would come in handy.
- Data aggregation across multiple data sources: Analytics needs to gather the information from multiple sources across business/ functional areas
- Blending existing and new data: Analytics should be capable to use the existing data that is available inside the organization and utilize with the new data outside the organization (social, market research, surveys, competition etc)
- Business user friendly: Analytics should be understandable to all relevant stakeholders intended to be consuming the insights
- Predictive Analytics: Analytics must provide the anticipative/predictive model and should also support “what-if” analysis for different scenarios
- Scalability and Flexibility: Analytics should be able to get customized but at the same time should be scalable and extensible for future needs
- Real-time Analytics Tools/Services: Analytics should be using tools to quickly process the data and translate that into actionable insight
Key Levers impacting Analytics Usage & Adoption
For any organization’s expectations and aspirations, and the current state of analytics takeoff primarily banks on the following five key levers. Key stakeholders who shall be impacted (including CXO’s & Senior Managers) need to be included as part of the assessment workshop where appropriate brainstorming needs to happen on existing challenges being face by business, current maturity of Analytics usage across departments/functions, thorough deep-dive into use cases where analytics consumers share their experiences of how they see analytics as a key ingredient to value creation in LOB’s or departments under their ambit.
- People
- Identifying key stakeholders
- Carving out roles/responsibilities
- Talent needs
- Change management
- Training requirements
- Analytics skills & competencies
- Data
- Data sources & management
- Data integration & accessibility
- Data Infrastructure
- Aligning data sources to use cases
- Tools/tech/platforms requirement
- Data maturity
- Vision
- Analytics vision and goals
- Assessment of key BU’s vision
- Analysing business drivers & needs
- Executive Sponsorship
- Business readiness
- Top down buy-in for analytics uptake
- Accountability and ownership
- Process
- Streamline existing analytics, reporting and operational processes
- Identification of modelling approaches required
- Benchmarking to best practices
- Mapping information needs
- Monitoring and refresh
- Ongoing Improvement
- Governance, Risk and compliance
- Improvement Process & Analysis Methodology
- Data and systems governance
- Documenting and reporting distribution needs
- Identification of future investment areas
In the next edition to this data strategy blog, I shall be touching upon the key stages an organization goes through as it matures along the analytics adoption curve, core design principles to execute a successful data monetization strategy, and key data and analytics transformation dimensions to choose the ideal business model(s). Stay tuned !