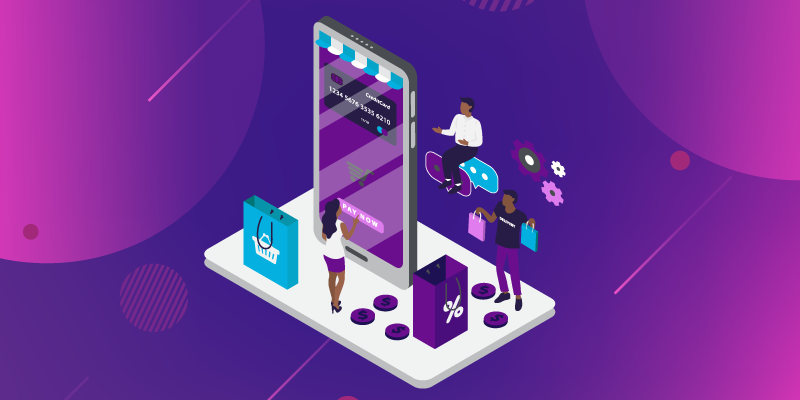
How AI is powering the transformation of the retail industry
Add Your Heading Text Here
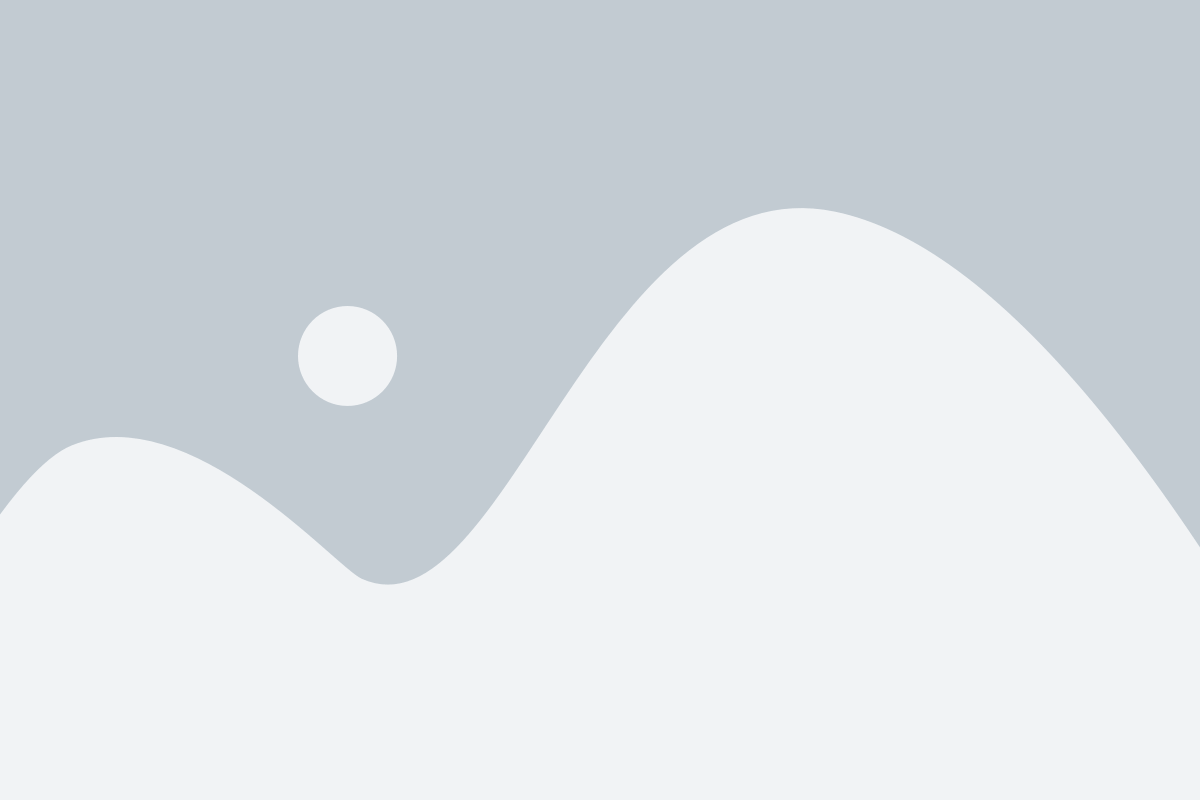
The brick-and-mortar retail industry is not in a good moment right now. Much of the turmoil in this industry comes from the fact that consumers are seeking a richer and indulging retail experience that reduces friction – much like what they have now become used to as they shop online. Consumers also expect traditional retailers to capture the essence of their individuality – who they are, what they like, and how they prefer to consume information. Retailers need to understand and align themselves with the expectations of the consumers in order to increase profitability and customer loyalty. They need to not only be aware, but also go full throttle for adopting technologies such as AI for influencing and revolutionising customer behaviour.
Retailers need to explore use cases pertaining to exponential technologies to address the disruption that their industry is going through. They need to catch up with how recommendation engines are redefining customer experience, how retail business value chain transformation is shaping up, and how AI can enhance the supply chain aspects of their business. And as I mentioned, awareness is simply not enough – they need to assess and adopt these technologies on a war footing to survive in the world we live in today.
The data-powered disruption of retail
Data in the retail industry is increasing exponentially in terms of volume, variety, velocity – and more importantly – value with every passing year. Smarter retailers are increasingly aware of how every interaction between the business and customers holds the potential to increase customer loyalty and drive additional customer revenue. Retailers that adopt AI today have the potential to raise their operating margins by as much as 60 percent.
But just having the data and building reports that summarise customer behaviour at an aggregate level are not enough. Insights are important, no doubt, but retailers desperately need systems that can make actionable decisions from the data. Insights into average user behaviour is simply too broad. Retailers need to now create a meaningful dialogue with each individual customer that honours their shopper’s preferred level and mode of engagement. This requires more than summarised reports. It requires technologies powered by AI – the ability to minutely understand every customer individually and take actions that each individual customer expects.
We now live in a time where data-driven decisions are extremely pervasive and useful. So much so that the worlds of ecommerce and traditional commerce are seamlessly merging. Every company is now omni-channel. Whether you think of Walmart buying Flipkart to boost their online presence or you take Amazon purchasing Whole Foods to bolster their brick-and-mortar presence. It is not about the web, the app, or the store – it is about having all of those. With the quantum of data available, we’ve seen an extraordinary few years in the retail industry – in the sense that data is actively deconstructing and rebuilding what retail will look like tomorrow. Traditional incumbents need to pay heed to the warning signs signalled by their defunct counterparts and aggressively embrace the data-driven disruption of retail.
AI transforming retail
Predictive analytics has been used in retail for several years now. However, in the last few years – with advances in technology and artificial intelligence – we are seeing the high speed, scale, and value that predictive analytics can deliver. The AI-enabled world of retail helps business transition into a world where consumers are always connected, more mobile, more social, and have choices about where they shop.
Deep learning in commerce
The retail industry is one with a lot of benefit to be gained from deep learning, in part because it’s such a data-rich industry and because there is some momentum around AI gathering already. Further a lot of the AI techniques enjoying success in other applications across industries powered by deep learning are well positioned to make serious impact on retail, streamlining processes, and transforming customer experience into something that largely resembles the experience customers get when they visit online portals.
Deep learning has been the fuel for much of the recent success in applied AI, so it is not surprising that some of the first attempts at augmenting the offline shopping experience have been making use of the power of deep learning in classifying images. If you look at something like Shelf analytics to seek out merchandising effectiveness, you can see the beginnings of how deep learning fits snugly in a retail context.
Automated AI
Now with minimal effort, retailers that can leverage automated AI capabilities will see a strong rise in customer engagement and sales. The best part is – this can be accomplished by data that is already available to them and captured in their enterprise systems. There’s more. The algorithms required for powering these systems, such as collaborative filtering, are relatively simple to deploy and efficient to run.
Intelligent product searches
Another great use case for retailers is leveraging AI for powering intelligent product searches. By using AI, customers can take pictures of things that they see in the real world, or even in an ad, and then locate a retailer who has that item in stock. This can easily serve as the start of a shopping experience. Typically, consumers do often see something that they like, but do not know the name of the item, brand or where they can source it from.
But taking photos is not the only modality for shopping, and there are other areas in the shopping experience where AI can play a part. In online commerce retail, for instance, customers often know what they are looking for but do not know the exact search terms or must scroll through multiple pages of inventory to find it. Deep learning can be of help here as well. Auto-encoding features of images in an inventory based on similarities and differences brings about a rich model of what is available in the inventory, and the model is surprisingly close to how we, as humans, perceive shoppable items. The model alone, of course, is not enough: We need a way to understand a shopper’s preferences as they interact with the inventory.
Speed and communication in real time
Just a few years ago, retailers used to take weeks to analyse customer data and make product offers. Machine learning and AI are changing the game by streaming live data and curating products in real time – based on their understanding of each customer. This significant drop in the amount of time taken between receiving data and powering an intelligent decision is made possible by AI and helps improve the uptake of products from retailers. For instance, by using mobile geo-location capabilities retailers can now offer deals or promotions when customers walk into or near the store (not after they’ve paid at the checkout and departed). Mobile push notifications on company apps allow retailers to track engagement the second it happens.
Given this rapid evolution, retailers have many choices on how to use AI in their marketing and sales strategies. Consumers are seeking a richer retail experience that reduces friction while also capturing the essence of who they are, what they like, and how they prefer to consume information. The sooner a retailer understands this and creates the best consumer experience they can, the sooner they will increase profitability and retention rates. I predict that this retail revolution will continue to unfold and expand over the next several years. But as the industry expands one thing is certain: in retail, personalisation and the customer journey are key, regardless of how you get there.
The ‘segment of one’ approach
A generic, aggregative understanding of customer behaviour is no longer enough. Individual segmentation is the next step for retailers looking to create a super-personalised experience for their users.
The worlds of traditional commerce retail and ecommerce retail are rapidly merging. I think ecommerce retail for many years was an interesting trend, but it was on the side, largely, of what was happening in retail. Today ecommerce retail is less an ancillary part of retail and more about the way business is now done. Online and offline experiences are fast coming together and without an omni-channel experience, it will be extremely difficult for a retailer to survive. That said, I do not doubt there is a future for brick-and-mortar retail, but there will need to be a transformation of retail real estate. Stores are going to become as much distribution and fulfilment centres as they are full-fledged shopping experiences. And they will need to be highly technology enabled.
Related Posts
AIQRATIONS
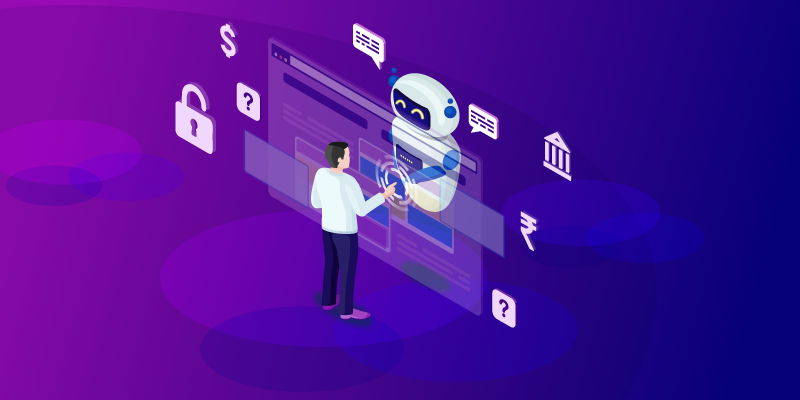
How artificial intelligence is changing the face of banking in India
Add Your Heading Text Here
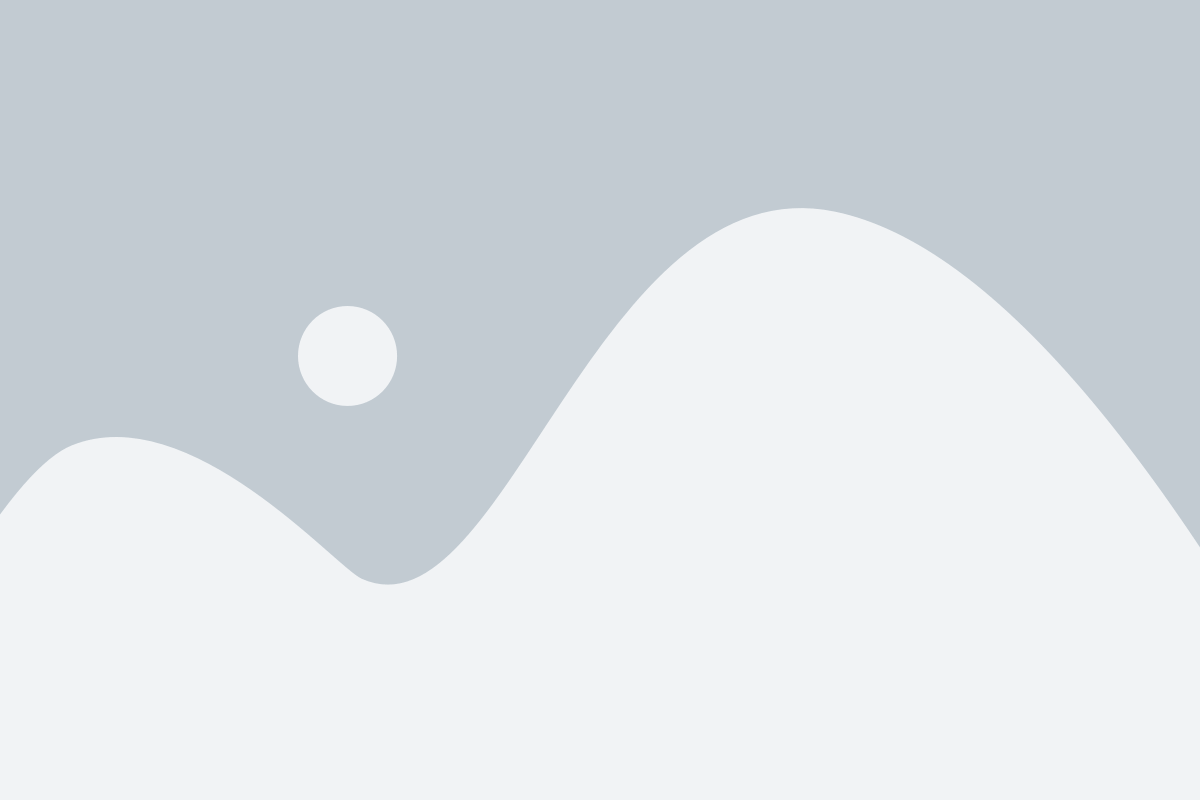
Artificial intelligence (AI) will empower banking organisations to completely redefine how they operate, establish innovative products and services, and most importantly impact customer experience interventions. In this second machine age, banks will find themselves competing with upstart fintech firms leveraging advanced technologies that augment or even replace human workers with sophisticated algorithms. To maintain a sharp competitive edge, banking corporations will need to embrace AI and weave it into their business strategy.
In this post, I will examine the dynamics of AI ecosystems in the banking industry and how it is fast becoming a major disrupter by looking at some of the critical unsolved problems in this area of business. AI’s potential can be looked at through multiple lenses in this sector, particularly its implications and applications across the operating landscape of banking. Let us focus on some of the key artifiicial intelligence technology systems: robotics, computer vision, language, virtual agents, and machine learning (including deep learning) that underlines many recent advances made in this sector.
Industry Changes
Banks entering the intelligence age are under intense pressure on multiple fronts. Rapid advances in AI are coming at a time of widespread technological and digital disruption. To manage this impact, many changes are being triggered.
- Leading banks are aggressively hiring Chief AI Officers while investing in AI labs and incubators
- AI-powered banking bots are being used on the customer experience front.
- Intelligent personal investment products are available at scale
- Multiple banks are moving towards custom in-house solutions that leverage sophisticated ontologies, natural language processing, machine learning, pattern recognition, and probabilistic reasoning algorithms to aid skilled employees and robots with complex decisions
Some of the key characteristics shaping this industry include:
- Decision support and advanced algorithms allow the automation of processes that are more cognitive in nature
- Solutions incorporate advanced self-learning capabilities
- Sophisticated cognitive hypothesis generation/advanced predictive analytics
Surge of AI in Banking
Banks today are struggling to reduce costs, meet margins, and exceed customer expectations through personal experience. To enable this, implementing AI is particularly important. And banks have started embracing AI and related technologies worldwide. According to a survey by the National Business Research Institute, over 32 percent of financial institutions use AI through voice recognition and predictive analysis. The dawn of mobile technology, data availability and the explosion of open-source software provides artificial intelligence huge playing field in the banking sector. The changing dynamics of an app-driven world is enabling the banking sector to leverage AI and integrate it tightly with the business imperatives.
AI in Banking Customer Services
Automated AI-powered customer service is gaining strong traction. Using data gathered from users’ devices, AI-based relay information using machine learning by redirecting users to the source. AI-related features also enable services, offers, and insights in line with the user’s behaviour and requirements. The cognitive machine is trained to advise and communicate by analysing users’ data. Online wealth management services and other services are powered by integrating AI advancements to the app by capturing relevant data.
The tested example of answering simple questions that the users have and redirecting them to the relevant resource has proven successful. Routine and basic operations i.e. opening or closing the account, transfer of funds, can be enabled with the help of chatbots.
Fraud and risk management
Online fraud is an area of massive concern for businesses as they digitise at scale. Risk management at internet scale cannot be managed manually or by using legacy information systems. Most banks are looking to deploy machine or deep learning and predictive analytics to examine all transactions in real-time. Machine learning can play an extremely critical role in the bank’s middle office.
The primary uses include mitigating fraud by scanning transactions for suspicious patterns in real-time, measuring clients for creditworthiness, and enabling risk analysts with right recommendations for curbing risk.
Trading and Securities
Robotic Process Automation (RPA) plays a key role in security settlement through reconciliation and validation of information in the back office with trades enabled in the front office. Artificial intelligence facilitates the overall process of trade enrichment, confirmation and settlement.
Credit Assessment
Lending is a critical business for banks, which directly and indirectly touches almost all parts of the economy. At its core, lending can be seen as a big data problem. This makes it an effective case for machine learning. One of the critical aspects is the validation of creditworthiness of individuals or businesses seeking such loans. The more data available about the borrower, the better you can assess their creditworthiness.
Usually, the amount of a loan is tied to assessments based on the value of the collateral and taking future inflation into consideration. The potential of AI is that it can analyse all of these data sources together to generate a coherent decision. In fact, banks today look at creditworthiness as one of their everyday applications of AI.
Portfolio Management
Banks are increasingly relying on machine learning to make smarter, real-time investment decisions on behalf of their investors and clients.
These algorithms can progress across distinct ways. Data becomes an integral part of their decision-making tree, this enables them to experiment with different strategies on the fly to broaden their focus to consider a more diverse range of assets.
Banks are focussed to leverage an AI and machine learning-based technology platforms that make customised portfolio profiles of customers based on their investment limits, patterns and preferences.
Banking and artificial intelligence are at a vantage position to unleash the next wave of digital disruption. A user-friendly AI ecosystem has the potential for creating value for the banking industry, but the desire to adopt such solutions across all spectrums can become roadblocks. Some of the issues can be long implementation timelines, limitations in the budgeting process, reliance on legacy platforms, and the overall complexity of a bank’s technology environment.
To overcome the above challenges of introducing and building an AI-enabled environment. Banks need to enable incremental adoption methods and technologies. The critical part is ensuring that the transition allows them to overcome the change management/behavioural issues. The secret sauce of successful deployment is to ensure a seamless fit into the existing technology architecture landscape, making an effective AI enterprise environment.
Related Posts
AIQRATIONS
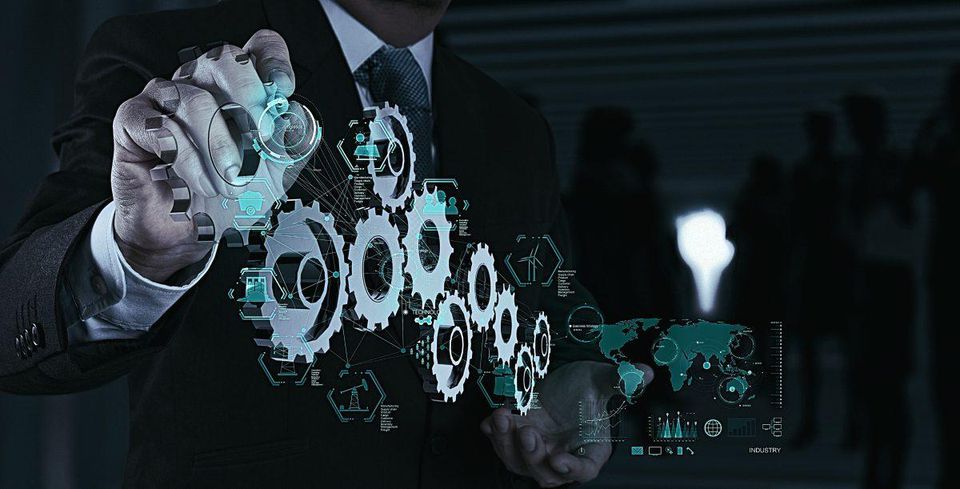
AI is the new MVP in sports
Add Your Heading Text Here
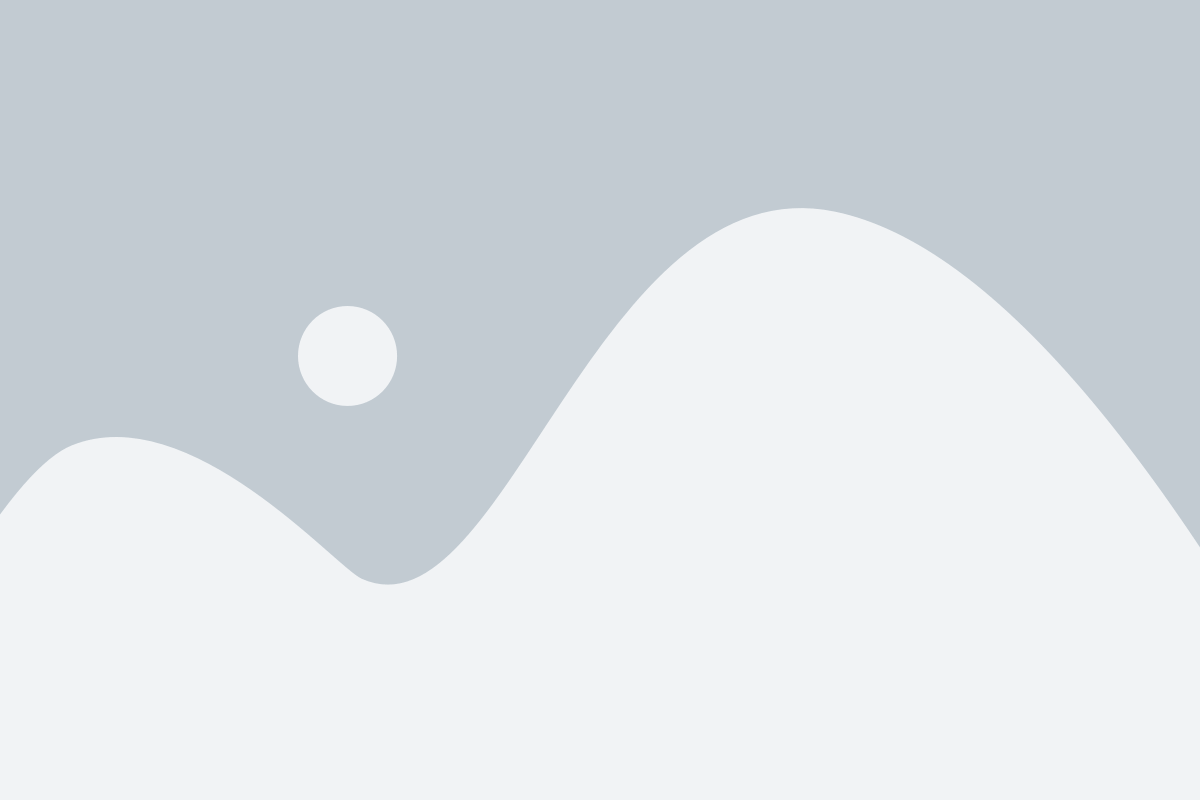
Over the last few years, technology has been rapidly permeating the sporting arena — from DRS in cricket to VAR (video assistant referee) and goal-line technology in football. Moneyball – the seminal book and movie on the use of analytics for smarter player acquisition – was the tipping point in how analytics and AI could be gainfully used in managing the business of sports better.
For years, avid sports fans around the world have had the topline analytics for their favourite players and teams at their fingertips. From there, today carrying analytics on player performance is seeing a massive data detonation. Sports analytics is no longer a mere water-cooler conversation. It is increasingly a specialised science that is rapidly informing the way professional sports team pick, monitor and coach players – in the process, even transforming the way a game is played.
As sports become more competitive, the margin for error is becoming smaller than ever. Teams need to incorporate every possible scientific element that helps improve player performance for a sustained improvement in their rankings. One such science being co-opted in sports is Artificial Intelligence – which is now a key component in the way teams identify players with high potential, monitor in-game progress to provide feedback and provide coaching for long-term success to players. Let us unpack these three crucial areas and see how AI is playing a key role in managing sports teams.
1. Identifying Top Stars
With a wealth of data available right from lower rungs to youth team records, AI can be hugely decisive in scouting for players likely to be mainstays of the senior levels of sport for years to come. By ingesting performance data for each player from their very first game, AI can uncover youth players who have a strong potential or can complement existing players in the team.
Union Minister of State for Youth Affairs and Sports Rajyavardhan Rathore believes that AI will help sports pick future stars. His plan is for the Indian government to create a database of nearly 3 crore young children in the age group of 5 to 18 years, who will be further refined and trained based on their abilities in different sports.
2. Performance and Insights
The major chunk of identified AI use cases falls in the arena of monitoring key performance indicators of sportspersons and providing insights for improvement. A lot of investment has already gone into the development of various sensors and devices that can track speed, accuracy, motion during practise sessions and games.
These monitoring devices – coupled with computer vision and machine learning – will be crucial interventions in the way coaches and players refine their approaches to their sport. Whether it be identifying potential areas to work on during training, or an analysis of situations within the game, AI can combine well with coaches to uncover how to extract the best performance from their teams and players.
An example of this in action is Dutch company SciSports. The company has developed a product called BallJames. Leveraging computer vision and machine learning, the product uses 3D images to provide insights on movements and tactics for football players.
3. Coaching for Success
Augmenting coaches and long-term training programme is the third and rapidly emerging use case of AI in sports. Depending on the sport being played, elite athletes spend between 10 years and 20 years at the very senior level, and possibly 10 years before at junior levels before becoming elite pros. All of which means that these athletes require continuous mental and physical conditioning to stay at the top of their game for nearly 30 years – depending on the sport they are playing.
AI can be an important intervention in building customised training routines for sportspersons – helping them take a long-term view of their health and diet. For instance, using AI, players could identify areas of improvement on the physical side and strengthen muscles, which may be adjudged as below-par for their sport. On similar lines, AI-led apps could also help suggest dietary options based on the conditioning required and provide personalised pathways for these athletes depending on their unique physical characteristics.
Mental Health
The other equally, if not more important aspect of managing the long-term well-being of athletes, is mental health. Sports players often live away from their families, go through swings in form alongside enormous highs and lows of success and failure. Through all of this, it is critical that we also manage the long-term psychological health of athletes to ensure that they stay at the top of their game. Apps such as jolt.ai – chatbots for motivation and tracking workout adherence – could be instrumental if created specifically to manage the mentality of sports players.
While the above mostly dealt with the ‘game’ aspects of sports management, there is also a plethora of topical applications that can be utilised in the management of sports franchisees (fan loyalty and engagement, player acquisition and abilities matching and uplift from sponsorship deals) and sports administrators (spend and procurement management, asset management and tracking legal compliance and fraud). When you combine these two areas, it is evident that Artificial Intelligence could very well be the next MVP in sports!
Related Posts
AIQRATIONS
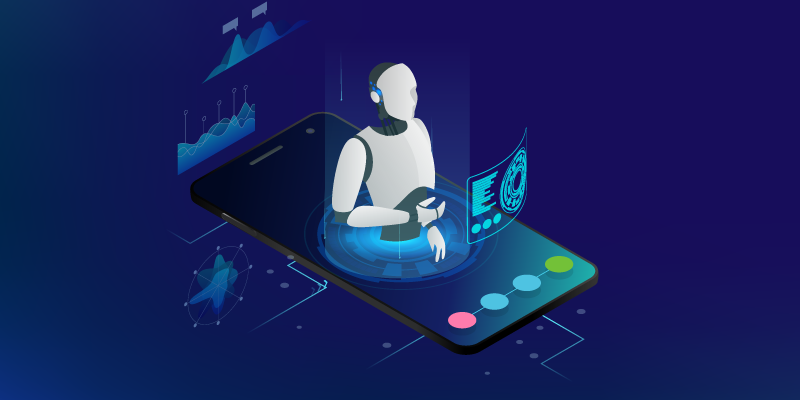
The Power of AI can radically improve the Engineering & Construction industry
Add Your Heading Text Here
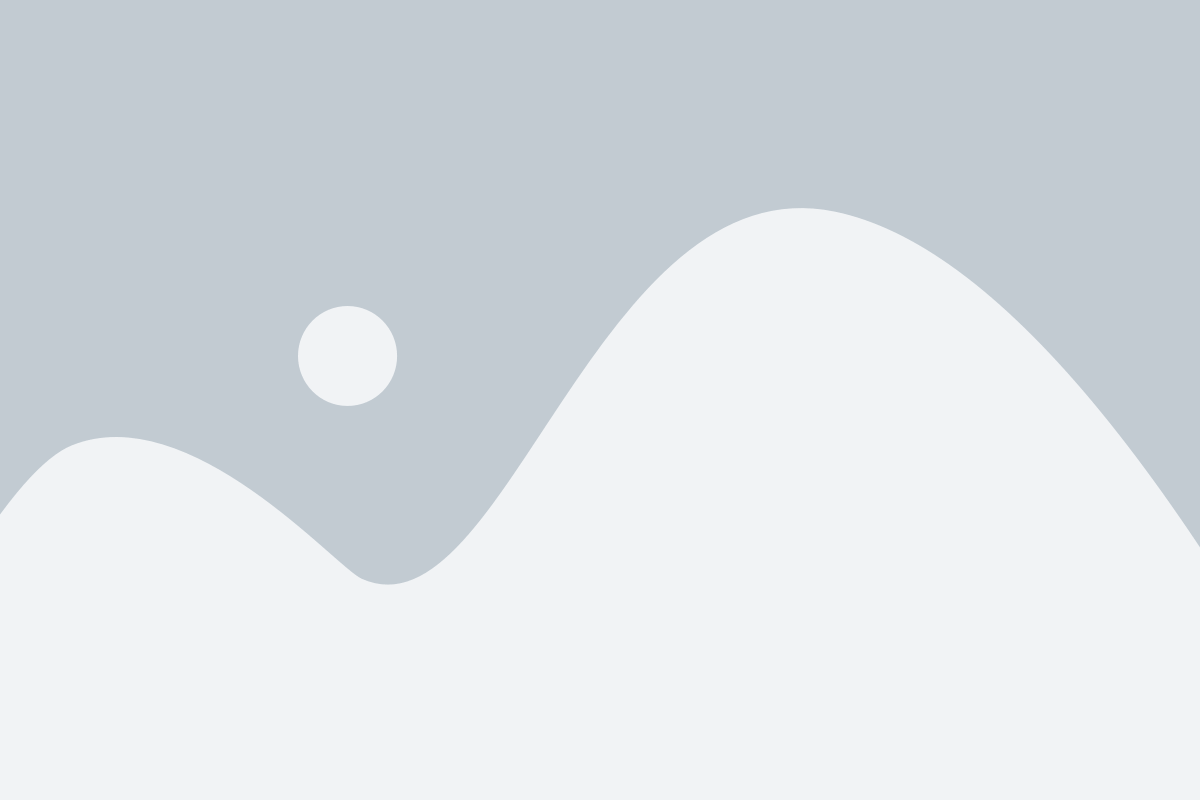
Why AI in construction?
Of all the game-changing innovations driven by technology and artificial intelligence in the world today, the potential of one key sector remains untapped – the construction industry.
According to McKinsey, the engineering and construction sector globally is valued to be worth $10 tn per year. While that is a respectable size, the construction industry overall has largely been slow in the uptake of inventions in the technology arena. In fact, several construction business houses in India tend to be family-owned and extremely traditionally-run, and have tremendous inertia in embracing new age technologies.
However, the past few years is seeing a change in the way construction firms operate. With well-funded global start-ups such as WeWork entering the fray with an AI and analytics forward approach to real estate development; industry incumbents need to up their game in order to stay relevant. While McKinsey expects the permeation of AI in the construction industry to be modest right now, it does represent an opportunity for early adopters to catch the bull by the horns and build a sizeable competitive advantage. Those from this industry that have a ponderous and slow uptake of new technology will surely be eaten up by their competitors. Through this article, we explore some artificial intelligence interventions that can be transformative for the construction and real estate industry at large.
Image recognition for managing risk, safety and quality
The construction industry would do well to adopt these techniques and apply them to manage risk and worker safety. Working conditions in the construction industry for labourers tend to be managed mediocrely at present. We hear of numerous cases of mortality and severe injuries where workers do not follow established safety procedures. Other cases also include unsafe working environments where certain infrastructure in overall construction projects are unsafe for human work.
Construction companies could employ drones to capture images and videos of their construction sites on a continuous basis. By applying deep learning and other AI techniques, firms would be able to identify unsafe workplace behaviour as well as unsafe working environments and run training interventions to improve the safety quotient of their workplaces.
Continuous design optimisation
Construction activity has largely been seen as a waterfall-like process where all the designs, construction materials and their feasibility are evaluated at the start of the project. While this is undoubtedly a watertight approach to construction, it does cause delays in planning, leading to lost revenue opportunity in the near term.
Today, with data readily available for analysis, AI can help continuously optimise the design of each project. A recommender system-like approach would help contractors and engineers identify the right design as well as the materials required to execute it. Additionally, AI-powered technology could also help recommend architectural finishes based on the proposed design – thus enabling construction firms to finalise the design and material requirements early in the schedule, and finish construction faster.
Increasing talent retention and development
The construction sector is remarkably disorganised and heavily relies on contract labour for executing a project. While minor, the cost and time involved in fulfilling positions left by ex-labourers and training new entrants really adds up and reduces the overall efficiency in project management. In India, contract labour can often also be seasonal, with numerous workers migrating to their hometowns in droves leading to longer gestation periods for projects.
AI has been applied to talent retention and talent development use cases in multiple industries, and the same can be applied to the construction industry with relative ease. With unsupervised machine learning algorithms, contractors and their parent companies will be able to forecast talent shortage accurately, and plan to backfill labour resources efficiently. AI can also enable improved labour retention strategies by recommending best options for ensuring improved talent retention and availability.
Project schedule optimisation
Construction projects are typically long drawn with a sizeable period elapsing between envisioning the project to having it commercially ready. In this period, we often see many niggles with respect to the project schedule. Overuse of materials, time-consuming nature of restocking, people availability issues – all these can throw the overall project plan into disarray.
Preventive maintenance through AI
Maintenance in the construction industry happens largely at two levels. Firstly, it is the maintenance of a partially and incrementally developing property. The second is when the builder organisation is responsible for the continuing maintenance after it has been leased out to tenants. At both levels, maintenance can be a hugely cumbersome and time-consuming activity, albeit critical, that the construction company must perform in order for operations to move smoothly.
We live in a world of sufficiently advanced technology and AI can complement human effort in the process of preventive maintenance. By using sensors and cameras as the data capture layer, and applying machine learning algorithms over the data, facility managers can monitor their property with greater ease and identify guided interventions on where maintenance activity is required. Using this data can be doubly productive as it will provide the system inputs on when routine maintenance activity for all the working components of a modern property are required, and schedule accordingly.
A technology-driven paradigm shift is fast coming for the construction industry. As things stand right now, the industry employs close to 7 percent of the global labour workforce. The strong uptake of infrastructure projects notwithstanding, the sector has grown only 1 percent per year for the past decades – with a flatlining per worker productivity, incumbents would do well to embrace the wave of Artificial Intelligence to power their next phase of growth. Using AI techniques, engineering and construction industry giants would be able to accelerate productivity, increase business efficiency and bring a much-needed technology facelift to the industry.
Related Posts
AIQRATIONS
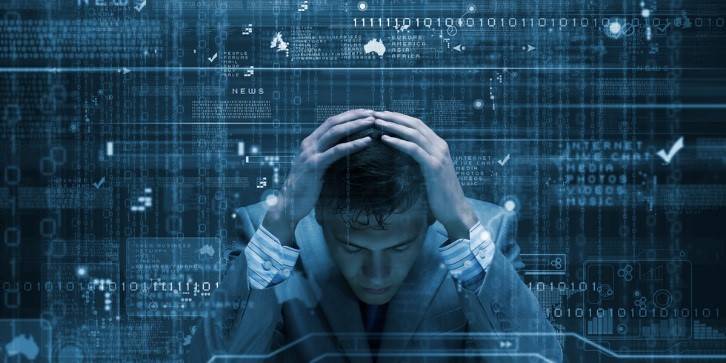
Data Glut to Data Abundance; The Fight for Data Supremacy – Enter the Age of Algorithm Ascendancy
Add Your Heading Text Here
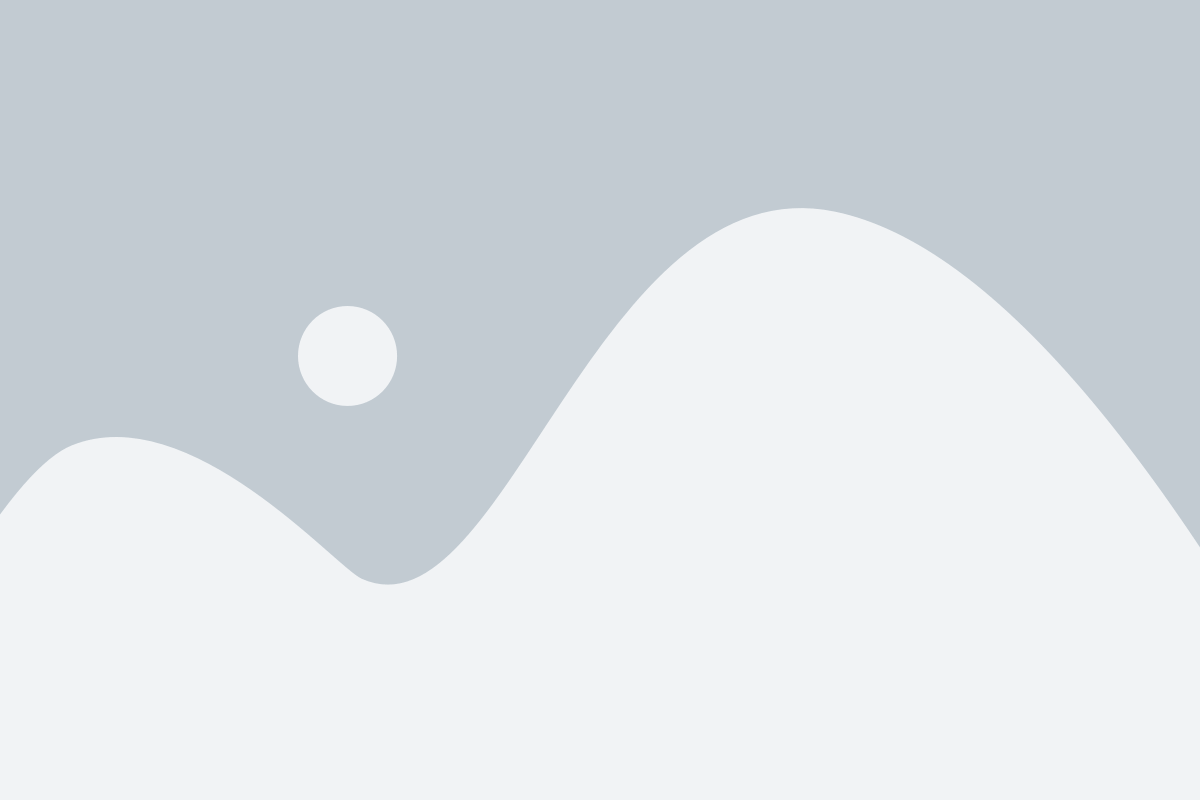
The definition of Data Breaches in current times have evolved from, happening under ‘malicious intent’, to also cover those which have been occurring as a consequences of bad data policies and regulation oversight. This means even policies that have been deemed legally screened might end up, in certain circumstances, in opening doors to some significant breach of data, user privacy and ultimately user trust.
For example, recently, Facebook banned data analytics company Cambridge Analytica from buying ads from its platform. The voter profiling firm allegedly procured 50 million physiological profiles of people through a research application developer Aleksandr Kogan, who broke Facebook’s data policies by sharing data from his personality-prediction app, that mined information from the social network’s users.
Kogan’s app, ‘thisisyourdigitallife’ harvested data not only from the individuals participating in the game, but also from everyone on their friend list. Since Facebook’s terms of services weren’t so clear back in 2014 the app allowed Kogan to share the data with third parties like Cambridge Analytica. This means policy wise it is a grey area whether the breach could be considered ‘unauthorized’, but it is clear that it happened without any express authorization from Facebook. This personal information was subsequently used to target voters and sway public opinion
This is different than the site hackings where credit card information was actually stolen at major retailers, the company in question, Cambridge Analytica, actually had the right to use this data. The problem is they used this information without permission in a way that was overtly deceptive to both Facebook users and Facebook itself.
Fallouts of Data Breaches: Developers left to deal with Tighter Controls
Facebook will become less attractive to app developers if it tightens norms for data usage as a fallout of the prevailing controversy over alleged misuse of personal information mined from its platform, say industry members.
India has the second largest developer base for Facebook, a community that builds apps and games on the platform and engage its users. With 241 million users, the country last July over took the US as the largest userbase for the social network platform.
There will be more scrutiny now. When you do, say, a sign on. The basic data (you can get) is the user’s name and email address, even which will undergo tremendous scrutiny before they approve it. That will have an impact on the timeline. The viral effect) could decrease. Now, without explicit rights from users, you cannot reach out to his/her contacts. Thus, the overhead goes on to the developers because of such data breaches, which shouldn’t have occurred in the first place had the policies surrounding user data were more distinct and clear.
Renewed Focus to Conflicting Data Policies and Human Factors
These kinds of passive breaches that happen because of unclear and conflicting policies instituted by Facebook provides us a very clear example of how active breaches (involving malicious attacks) and passive breaches (involving technically authorized but legally unsavoury data sharing) need to be given equal priority and should both be considered pertinent focus of data protection.
While Facebook CEO Mark Zuckerberg has vowed to make changes to prevent these types of information grabs from happening in the future, many of those tweaks will be presumably made internally. Individuals and companies still need to take their own action to ensure their information remains as protected and secure as possible.
Humans are the weakest link in data protection, and potentially even the leading cause for the majority of incidents in recent years. This debacle demonstrates that cliché to its full extent. Experts believe that any privacy policy needs to take into account all third parties who get access to the data too. While designing a privacy policy one needs to keep the entire ecosystem in mind. For instance, a telecom player or a bank while designing their privacy policy will have to take into account third parties like courier agencies, teleworking agencies, and call centers who have access to all their data and what kind of access they have.
Dealing with Privacy in Analytics: Privacy-Preserving Data Mining Algorithms
The problem of privacy-preserving data mining has become more important in recent years because of the increasing ability to store personal data about users, and the increasing sophistication of data mining algorithms to leverage this information. A number of algorithmic techniques such as randomization and k-anonymity, have been suggested in recent years in order to perform privacy-preserving data mining. Different communities have explored parallel lines of work in regards to privacy preserving data mining:
Privacy-Preserving Data Publishing: These techniques tend to study different transformation methods associated with privacy. These techniques include methods such as randomization, k-anonymity, and l-diversity. Another related issue is how the perturbed data can be used in conjunction with classical data mining methods such as association rule mining.
Changing the results of Data Mining Applications to preserve privacy: In many cases, the results of data mining applications such as association rule or classification rule mining can compromise the privacy of the data. This has spawned a field of privacy in which the results of data mining algorithms such as association rule mining are modified in order to preserve the privacy of the data.
Query Auditing: Such methods are akin to the previous case of modifying the results of data mining algorithms. Here, we are either modifying or restricting the results of queries.
Cryptographic Methods for Distributed Privacy: In many cases, the data may be distributed across multiple sites, and the owners of the data across these different sites may wish to compute a common function. In such cases, a variety of cryptographic protocols may be used in order to communicate among the different sites, so that secure function computation is possible without revealing sensitive information.
Privacy Engineering with AI
Privacy by Design is a policy concept was introduced the Data Commissioner’s Conference in Jerusalem, and over 120 different countries agreed they should contemplate privacy in the build, in the design. That means not just the technical tools you buy and consume, [but] how you operationalize, how you run your business; how you organize around your business and data.
Privacy engineering is using the techniques of the technical, the social, the procedural, the training tools that we have available, and in the most basic sense of engineering to say, “What are the routinized systems? What are the frameworks? What are the techniques that we use to mobilize privacy-enhancing technologies that exist today, and look across the processing lifecycle to build in and solve for privacy challenges?”
It’s not just about individual machines making correlations; it’s about different data feeds streaming in from different networks where you might make a correlation that the individual has not given consent to with personally identifiable information. For AI, it is just sort of the next layer of that. We’ve gone from individual machines, networks, to now we have something that is looking for patterns at an unprecedented capability, that at the end of the day, it still goes back to what is coming from what the individual has given consent to? What is being handed off by those machines? What are those data streams?
Also, there is the question of ‘context’. The simplistic policy of asking users if an application can access different venues of their data is very reductive. This does not, in an measure give an understanding of how that data is going to be leveraged and what other information about the users would the application be able to deduce and mine from the said data? The concept of privacy is extremely sensitive and not only depends on what data but also for what purpose. Have you given consent to having it used for a particular purpose? So, I think AI could play a role in making sense of whether data is processed securely.
The Final Word: Breach of Privacy as Crucial as Breach of Data
It is undeniably so that we are slowly giving equal, if not more importance to breach of privacy as compared to breach of data, which will eventually target even the policies which though legally acceptable or passively mandated but resulted in compromise of privacy and loss of trust. Because there is no point claiming one is legally safe in their policy perusal if the end result leads to the users being at the receiving end.
This would require a comprehensive analysis of data streams, not only internal to an application ecosystem, like Facebook, but also the extended ecosystem involving all the players it is channeling the data sharing to, albeit in a policy-protected manner. This will require AI enabled contextual decision making to come to terms as what policies could be considered as eventually breaching the privacy in certain circumstances.
Longer-term, though, you’ve got to write that ombudsman. We need to be able to engineer an AI to serve as an ombudsman for the AI itself.
Related Posts
AIQRATIONS
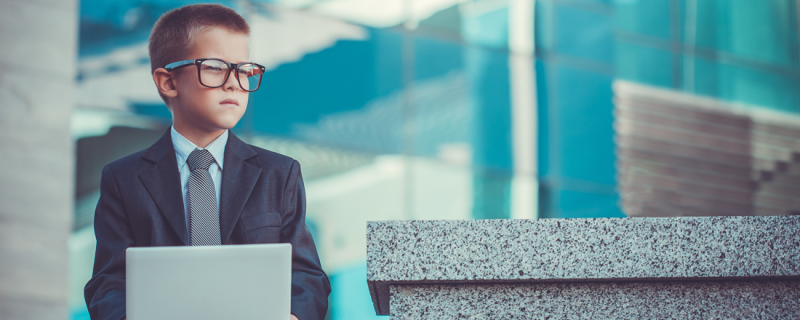
How Rise of Exponential Technologies – AI, RPA, Blockchain, Cybersecurity will Redefine Talent Demand & Supply Landscape
Add Your Heading Text Here
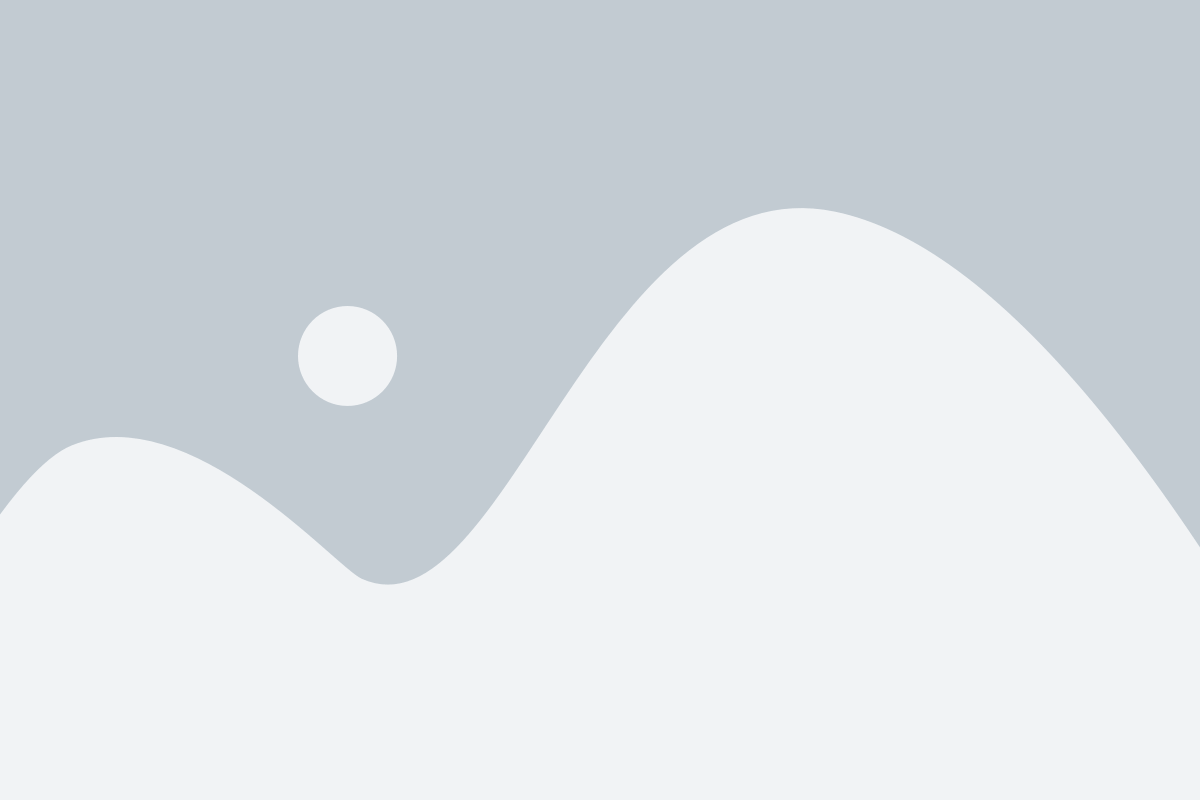
The current boom of exponential technologies of today is causing strong disruption in the talent availability landscape, with traditional, more mechanical roles being wiped out and paving way for huge demand for learning and design thinking based skills and professions. The World Economic Forum said in 2016 that 60% of children entering school today will work in jobs that do not yet exist.
While there is a risk to jobs due to these trends, the good news is that a huge number of new jobs are getting created as well in areas like AI, Machine Learning, Robotic Process Automation (RPA), Blockchain, Cybersecurity, etc. It is clearly a time of career pivot for IT professionals to make sure they are where the growth is.
AI and Machine Learning upending the traditional IT Skill Requirement
AI and Machine Learning will create a new demand for skills to guide its growth and development. These emerging areas of expertise will likely be technical or knowledge-intensive fields. In the near term, the competition for workers in these areas may change how companies focus their talent strategies.
At a time when the demand for data scientists and engineers will grow 39% by 2020, employers are seeking out leaders who can effectively work with technologists to ask the right questions and apply the insight to solve business problems. The business schools are, hence, launching more programs to equip graduates with the skills they need to succeed. Toronto’s Rotman School of Management, for example, last week launched a nine-month program which provides recent college graduates with advanced data management, analytical and communication skills.
According to the Organization of Economic Cooperation and Development, only 5-10% of labor would be displaced by intelligent automation, and new job creation will offset losses.
The future will increase the value of workers with a strong learning ability and strength in human interaction. On the other hand, today’s highly paid, experienced, and skilled knowledge workers may be at risk of losing their jobs to automation.
Many occupations that might appear to require experience and judgment — such as commodity traders — are being outdone by increasingly sophisticated machine-learning programs capable of quickly teasing subtle patterns out of large volumes of data. If your job involves distracting a patient while delivering an injection, guessing whether a crying baby wants a bottle or a diaper change, or expressing sympathy to calm an irate customer, you needn’t worry that a robot will take your job, at least for the foreseeable future.
Ironically, the best qualities for tomorrow’s worker may be the strengths usually associated with children. Learning has been at the centre of the new revival of AI. But the best learners in the universe, by far, are still human children. At first, it was thought that the quintessential preoccupations of the officially smart few, like playing chess or proving theorems — the corridas of nerd machismo — would prove to be hardest for computers. In fact, they turn out to be easy. Things every dummy can do like recognizing objects or picking them up are much harder. And it turns out to be much easier to simulate the reasoning of a highly trained adult expert than to mimic the ordinary learning of every baby. The emphasis on learning is a key change from previous decades and rounds of automation.
According to Pew Research, 47% of all employment opportunities will be occupied by machines within the next two decades.
What types of skills will be needed to fuel the development of AI over the next several years? These prospects include:
- Ethics: The only clear “new” job category is that of AI ethicist, a role that will manage the risks and liabilities associated with AI, as well as transparency requirements. Such a role might be imagined as a cross between a data scientist and a compliance officer.
- AI Training: Machine learning will require companies to invest in personnel capable of training AI models successfully, and then they must be able to manage their operations, requiring deep expertise in data science and an advanced business degree.
- Internet of Things (IoT): Strong demand is anticipated for individuals to support the emerging IoT, which will require electrical engineering, radio propagation, and network infrastructure skills at a minimum, plus specific skills related to AI and IoT.
- Data Science: Current shortages for data scientists and individuals with skills associated with human/machine parity will likely continue.
- Additional Skill Areas: Related to emerging fields of expertise are a number of specific skills, many of which overlap various fields of expertise. Examples of potentially high-demand skills include modeling, computational intelligence, machine learning, mathematics, psychology, linguistics, and neuroscience.
In addition to its effect on traditional knowledge workers and skilled positions, AI may influence another aspect of the workplace: gender diversity. Men hold 97 percent of the 2.5 million U.S. construction and carpentry jobs. These male workers stand more than a 70 percent chance of being replaced by robotic workers. By contrast, women hold 93 percent of the registered nurse positions. Their risk of obsolescence is vanishingly small: .009 percent.
RPA disrupting the traditional computing jobs significantly
RPA is not true AI. RPA uses traditional computing technology to drive its decisions and responses, but it does this on a scale large and fast enough to roughly mimic the human perspective. AI, on the other hand, applies machine and deep learning capabilities to go beyond massive computing to understand, learn, and advance its competency without human direction or intervention — a truly intelligent capability. RPA is delivering more near-term impact, but the future may be shaped by more advanced applications of true AI.
In 2016, a KPMG study estimated that 100 million global knowledge workers could be affected by robotic process automation by 2025.
The first reaction would be that in the back office and the middle office, all those roles which are currently handling repetitive tasks would become redundant. 47% of all American job functions could be automated within 20 years, according to the Oxford Martin School on Economics in a 2013 report.
Indeed, India’s IT services industry is set to lose 6.4 lakh low-skilled positions to automation by 2021, according to U.S.-based HfS Research. It said this was mainly because there were a large number of non-customer facing roles at the low-skill level in countries like India, with a significant amount of “back office” processing and IT support work likely to be automated and consolidated across a smaller number of workers.
Automation threatens 69% of the jobs in India, while it’s 77% in China, according to a World Bank research.
Job displacement would be the eventual outcome however, there would be several other situations and dimensions which need to be factored. Effective automation with the help of AI should create new roles and new opportunities hitherto not experienced. Those who currently possess traditional programming skills have to rapidly acquire new capabilities in machine learning, develop understanding of RPA and its integration with multiple systems. Unlike traditional IT applications, planning and implementation could be done in small patches in shorter span of time and therefore software developers have to reorient themselves.
For those entering into the workforce for the first time, there would be a demand for talent with traditional programming skills along with the skills for developing RPA frameworks or for customising the frameworks. For those entering the workforce for being part of the business process outsourcing functions, it would be important to develop capability in data interpretation and analysis as increasingly more recruitment at the entry level would be for such skills and not just for their communication or transaction handling skills.
Blockchain – A blue ocean of a New kind of Financial Industry Skillset
A technology as revolutionary as blockchain will undoubtedly have a major impact on the financial services landscape. Many herald blockchain for its potential to demystify the complex financial services industry, while also reducing costs, improving transparency to reduce the regulatory burden on the industry. But despite its potential role as a precursor to extend financial services to the unbanked, many fear that its effect on the industry may have more cons than pros.
30–60% of jobs could be rendered redundant by the simple fact that people are able to share data securely with a common record, using Blockchain
Industries including payments, banking, security and more will all feel the impact of the growing adoption of this technology. Jobs potentially in jeopardy include those involving tasks such as processing and reconciling transactions and verifying documentation. Profit centers that leverage financial inefficiencies will be stressed. Companies will lose their value proposition and a loss of sustainable jobs will follow. The introduction of blockchain to the finance industry is similar to the effect of robotics in manufacturing: change in the way we do things, leading to fewer jobs, is inevitable.
Nevertheless, the nature of such jobs is likely to evolve. While Blockchain creates an immutable record that is resistant to tampering, fraud may still occur at any stage in the process but will be captured in the record and there easily detected. This is where we can predict new job opportunities. There could be a whole class of professions around encryption and identity protection.
So far, the number of jobs created by the industry appears to exceed the number of available professionals qualified to fill them, but some aren’t satisfied this trend will continue. Still, the study of the potential impact of blockchain tech on jobs has been largely qualitative to date. Aite Group released a report that found the largest employers in the blockchain industry each employ about 100 people.
Related Posts
AIQRATIONS
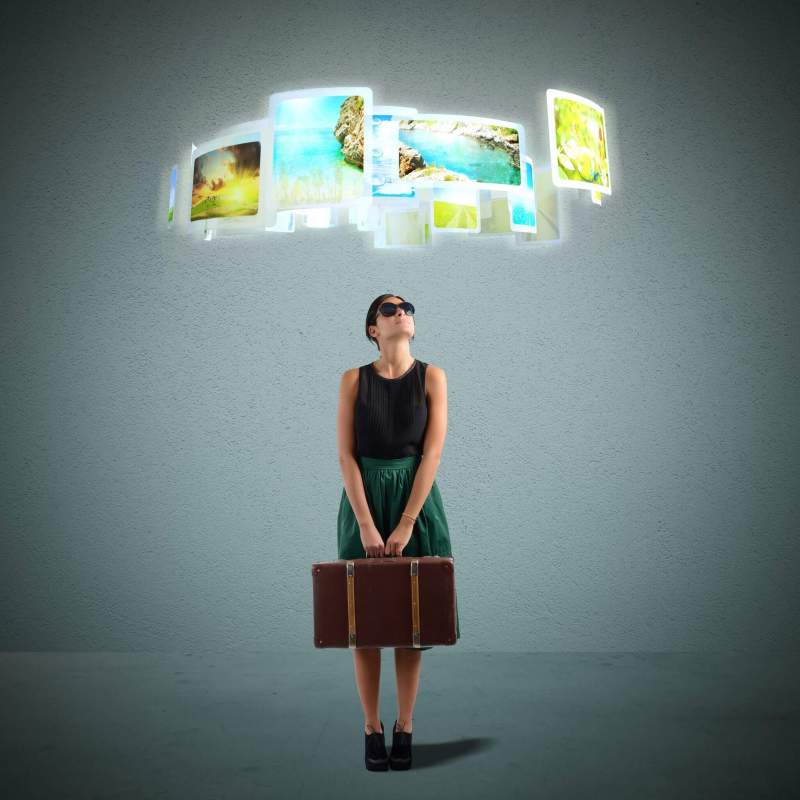
Travel & Hospitality Industry Transformation: Served Fast with AI
Add Your Heading Text Here
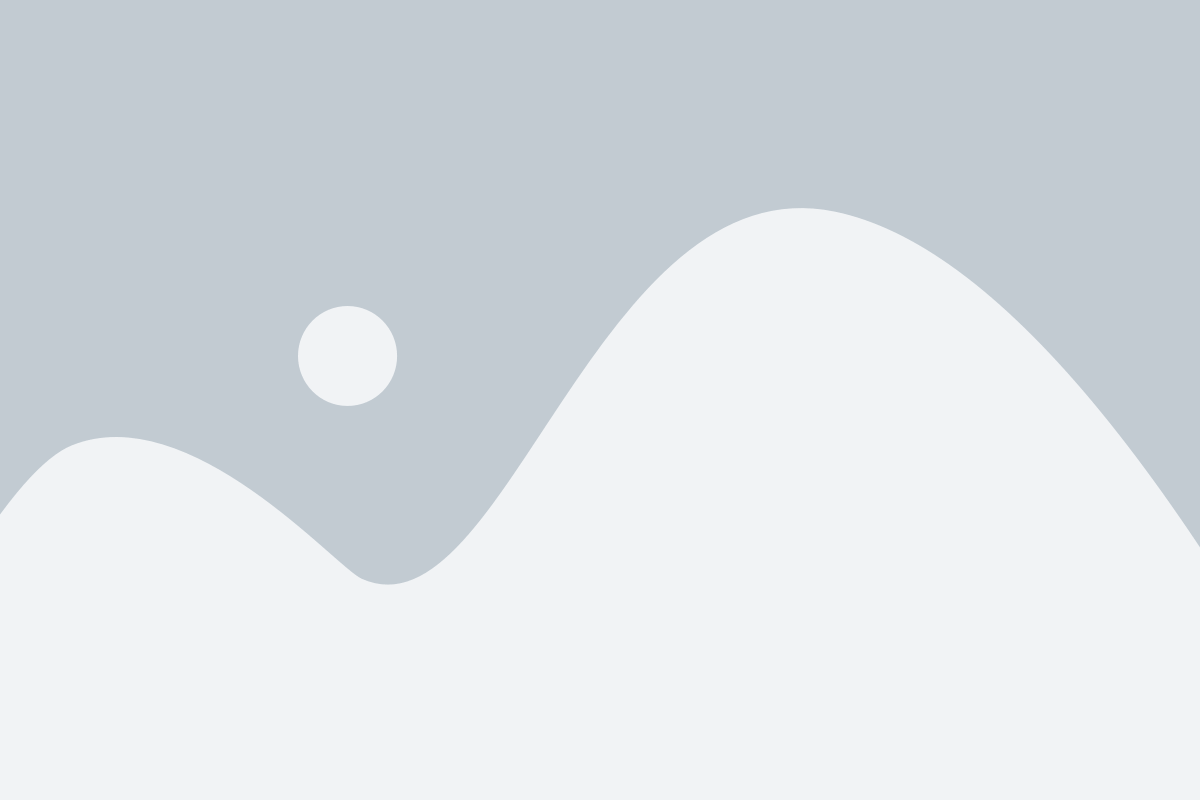
Over the years, the influence of AI has spread to almost every aspect of the travel and the hospitality industry. 30% of hospitality businesses use artificial intelligence to augment at least one of their primary sales processes and most customer personalization is done using AI.
30% of hospitality businesses use artificial intelligence to augment at least one of their primary sales processes.
The sudden popularity of Artificial Intelligence in the Travel industry can be credited to the humongous amount of data being generated today. Artificial Intelligence helps analyse unstructured data, brings value in partnership with Big Data and turns it into meaningful and actionable insights. Trends, outliers and patterns are figured out using this smart data which helps in guiding a Travel company to make informed decisions. The discounts, schemes, tour packages, seasons to target and people to target are formulated using this data. Usually, surveys and social media sensing are done to know customer’s insights and behaviour.
Let’s look at how AI has influenced each aspect of the business
Bleisure – Personalized Experience
There are always a few who are up for a new challenge and adopt to new technology. Many hotels have started using an AI concierge. One great example of an AI concierge is Hilton World wide’s Connie, who is the first true AI-powered concierge bot.
Connie stands at 2 feet high and guests can interact with it during their check-in. Connie is powered by IBM’s Watson AI and uses WayBlazer travel database. It can provide information to guests on local attractions, places to visit, etc. Being an AI, it can learn and adapt and respond to each guest.
In the Travel business, Mezi, using Artificial Intelligence and Natural Language Processing, provides a personalized experience to Business travellers who usually are strapped for time. It talks about bringing on a concept of bleisure (business+leisure) to address the needs of the workforce. A research done by them states that 84% of business travellers return feeling frustrated, burnt out and unmotivated. The kind of tedious and monotonous planning that goes into the travel booking could be the reason for it. With AI and NLP, Mezi collects preferences and generates suggestions so that a customized and streamlined experience is given and the issues faced by them are addressed properly.
Increased Productivity – Instant Connectivity
Increased productivity now begins with the search for the hotel, and technology has paved its way for the customer to access more data than ever before. Booking sites like Lola (www.lola.com) who provide on-demand travel services have developed technologies that can not only instantly connects people to their team of travel agents who find and book flights, hotels, and cars but have been able to empower their agents with tremendous technology to make research and decisions an easy process.
Intelligent Travel Assistants – Chatbots
Chatbot technology is another big strand of AI, and unsurprisingly, many travel brands have already launched their own versions in the past year or so. Skyscanner is just one example, creating a bot to help consumers find flights in Facebook Messenger. Users can also use it to request travel recommendations and random suggestions. Unlike ecommerce or retail brands using chatbots, which can appear gimmicky, there is an argument that examples like Skyscanner are much more relevant and useful for everyday consumers.
After all, with the arrival of many more travel search websites, consumers are being overwhelmed by choice – not necessarily helped by it. Consequently, a bot like Skyscanner is able to cut through the noise, connecting with consumers in their own time and in the social media spaces they most frequently visit.
Recently, Aeromexico started using Facebook Messenger chatbot to answer the very generic questions by the customers. The main idea was to cater to 80% of questions which are usually the repeated ones and about common topics. Thus, to avoid a repetitive process, artificial intelligence is of great application. Airlines hugely benefit from this. KLM Royal Dutch Airlines uses artificial intelligence to respond to the queries of customers on twitter and facebook. It uses an algorithm from a company called Digital Genius which is trained on 60,000 questions and answers. Not only this, Deutsche Lufthansa’s bot Mildred can help in searching the cheapest fares.
Discovery & Data Analysis – Intelligent Recommendations
International hotel search engine Trivago acquired Hamburg, Germany machine learning startup, Tripl, as it ramps up its product with recommendation and personalization technology, giving them a customer-centric approach.
The AI algorithm gives tailored travel recommendations by identifying trends in users’ social media activities and comparing it with in-app data of like-minded users. With its launch in July 2015, users could sign up only through Facebook, potentially sharing oodles of profile information such as friends, relationship status, hometown, and birthday.
Persona based travel recommendations, use of customised pictures and text are now gaining ground to entice travellers to book your hotels. KePSLA’s travel recommendation platform is one of the first in the world to do this by using deep learning and NLP solutions.
With 81% of people believing that robots would be better at handling data than humans, there is also a certain level of confidence in this area from consumers.
Knowing your Travellers – Deep Customer Behaviour
Dorchester Collection is another hotel chain to make use of AI. However, instead of using it to provide a front-of-house service, it has adopted it to interpret and analyse customer behaviour in the form of raw data.
Partnering with technology company, RicheyTX, Dorchester Collection has helped to develop an AI platform called Metis.
Delving into swathes of customer feedback such as surveys and reviews (which would take an inordinate amount of time to manually find and analyse) it is able to measure performance and instantly discover what really matters to guests.
For example, Metis helped Dorchester to discover that breakfast it not merely an expectation – but something guests place huge importance on. As a result, the hotels began to think about how they could enhance and personalise the breakfast experience.
Flight Fare and Hotel Price Forecasting
Flight fares and hotel prices are ever-changing and vary greatly depending on the provider. No one has time to track all those changes manually. Thus, smart tools which monitor and send out timely alerts with hot deals are currently in high demand in the travel industry.
The AltexSoft data science team has built such an innovative fare predictor tool for one of their clients, a global online travel agency, Fareboom.com. Working on its core product, a digital travel booking website, they could access and collect historical data about millions of fare searches going back several years. Armed with such information, they created a self-learning algorithm, capable of predicting the future price movements based on a number of factors, such as seasonal trends, demand growth, airlines special offers, and deals.
With the average confidence rate at 75 percent, the tool can make short-term (several days) as well as long-term (a couple of months) forecasts.
Optimized Disruption Management
While the previous case is focused mostly on planning trips and helping users navigate most common issues while traveling, automated disruption management is somewhat different. It aims at resolving actual problems a traveler might face on his/her way to a destination point.
Mostly applied to business and corporate travel, disruption management is always a time-sensitive task, requiring instant response. While the chances to get impacted by a storm or a volcano eruption are very small, the risk of a travel disruption is still quite high: there are thousands of delays and several hundreds of canceled flights every day.
With the recent advances in technology, it became possible to predict such disruptions and efficiently mitigate the loss for both the traveler and the carrier. The 4site tool, built by Cornerstone Information Systems, aims at enhancing the efficiency of enterprise travel. The product caters to travelers, travel management companies, and enterprise clients, providing a unique set of features for real-time travel disruption management.
For example, if there is a heavy snowfall at your destination point and all flights are redirected to another airport, a smart assistant can check for available hotels there or book a transfer from your actual place of arrival to your initial destination.
Not only passengers are affected by travel disruptions; airlines bear significant losses every time a flight is canceled or delayed. Thus, Amadeus, one of the leading global distribution systems (GDS), has introduced Schedule Recovery system, aiming to help airlines mitigate the risks of travel disruption. The tool helps airlines instantly address and efficiently handle any threats and disruptions in their operations.
Future potential
So, we’ve already seen the travel industry capitalise on AI to a certain extent. But how will it evolve in the coming year?
Business travel
Undoubtedly, we’ll see many more brands using AI for data analysis as well as launching their own chatbots. There’s already been a suggestion that Expedia is next in line, but it is reportedly set to focus on business travel rather than holidaymakers. Due to the greater need for structure and less of a desire for discovery, it certainly makes sense that artificial intelligence would be more suited to business travellers.
Specifically, it could help to simplify the booking process for companies, as well as help eliminate discrepancies around employee expenses. With reducing costs and improving efficiency two of the biggest benefits, AI could start to infiltrate business travel even more so than leisure in the next 12 months.
Voice technology
Lastly, we can expect to see greater development in voice-activated technology.
With voice-activated search, the experience of researching and booking travel has the potential to become quicker and easier than ever before. Similarly, as Amazon Echo and Google Home start to become commonplace, more hotels could start to experiment with speech recognition to ramp up customer service.
This means devices and bots could become the norm for brands in the travel industry.
Related Posts
AIQRATIONS
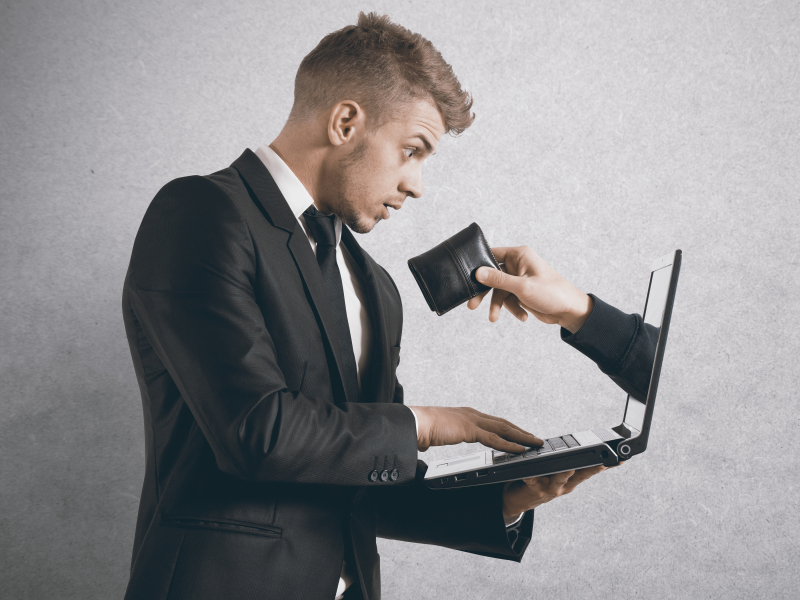
How AI is Enabling Mitigation of Fraud in the Banking, Insurance Enterprises
Add Your Heading Text Here
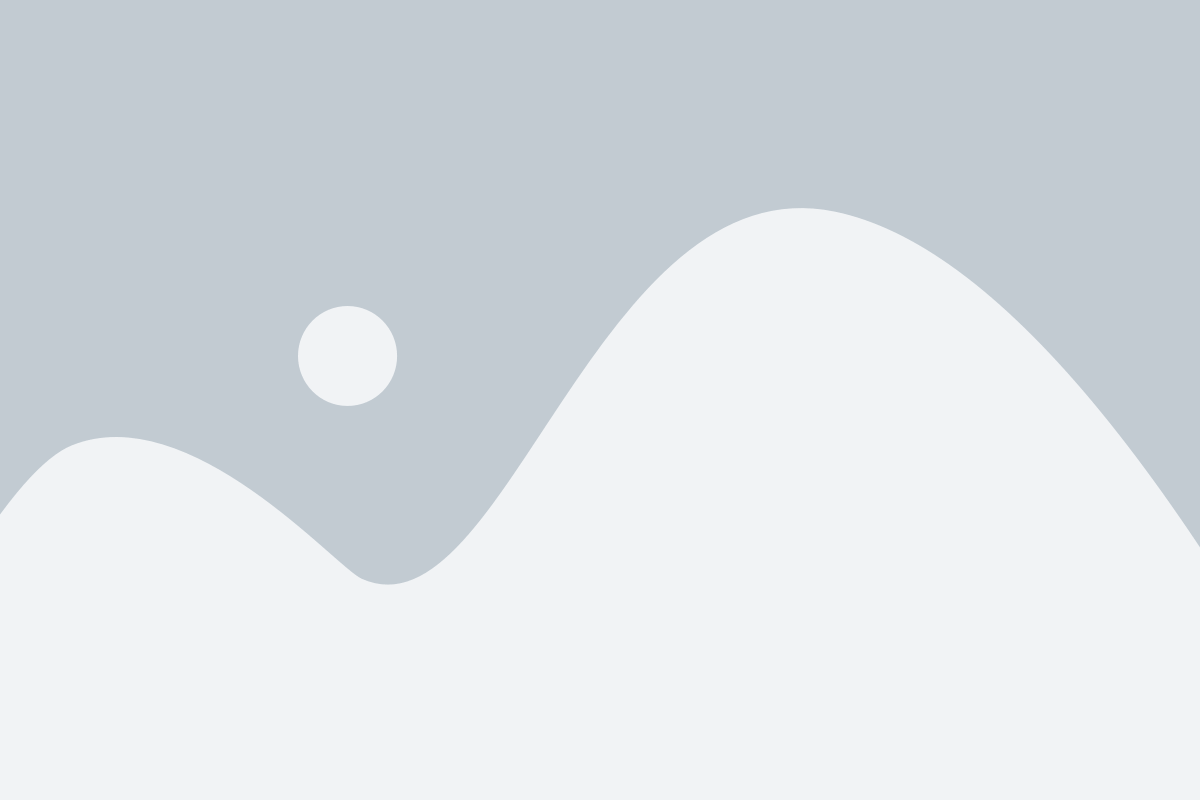
The Banking and Finance sector (BFSI) is witnessing one of its most interesting and enriching phases. Apart from the evident shift from traditional methods of banking and payments, technology has started playing a vital role in defining this change.
Mobile apps, plastic money, e-wallets and bots have aided the phenomenal swing from offline payments to online payments over the last two decades. Now, the use of Artificial Intelligence (AI) in BFSI is expediting the evolution of this industry.
But as the proliferation of digital continues, the number of ways one can commit fraud has also increased. Issuers, merchants, and acquirers of credit, debit, and prepaid general purpose and private label payment cards worldwide experienced gross fraud losses of US$11.27 billion in 2012, up 14.6% over the previous year1. Fraud losses on all general purpose and private label, signature and PIN payment cards reached US$5.33 billion in United States in the same period, up 14.5%1. These are truly big numbers, and present the single-biggest challenge to the trust reposed in banks by customers. Besides the risk of losing customers, direct financial impact for banks is also a significant factor.
Upon reporting of a fraudulent transaction by a customer, the bank is liable for the transaction cost, it has to refund merchant chargeback fee, as well as additional fee. Fraud also invites fines from regulatory authorities. The recently-passed Durbin Amendment caps processing fee that can be charged per transaction, and this increases the damage caused by unexpected fraud losses. The rapidly rising use of electronic payment modes has also increased the need for effective, efficient, and real-time methods to detect, deter, and prevent fraud.
Nuances of Banking Fraud Prevention Using AI
AI enables a computer to behave and take decisions like a human being. Coined in 1956 by John McCarthy at MIT, the term AI was little known to the layman and merely a subject of interest to academicians, researchers and technologists. However, over the past few years, it is more commonly seen in our everyday lives; in our smartphones, shopping experiences, hospitals, travel, etc.
Machine Learning, Deep Learning, NLP Platforms, Predictive APIs and Image and Speech Recognition are some core AI technologies used in BFSI today. Machine Learning recognises data patterns and highlights deviations in data observed. Data is analysed and then compared with existing data to look for patterns. This can help in fraud detection, prediction of spending patterns and subsequently, the development of new products.
Key Stroke Dynamics
Key Stroke Dynamics can be used for analysing transactions made by customers. They capture strokes when the key is pressed (dwell time) and released on a keyboard, along with vibration information.
As second factor authentication is mandatory for electronic payments, this can help detect fraud, especially if the user’s credentials are compromised. Deep Learning is a new area in Machine Learning research and consists of multiple linear and non-linear transformations. It is based on learning and improving representations of data. A common application of this can be found in the crypto-currency, Bitcoin.
Adaptive Learning
Adaptive Learning is another form of AI currently used by banks for fraud detection and mitigation. A model is created using existing rules or data in the bank’s system. Incremental learning algorithms are then used to update the models based on changes observed in the data patterns.
AI instances in Insurance for Fraud Prevention
Applying for Insurance
When a customer submits their application for insurance, there is an expectation that the potential policyholder provides honest and truthful information. However, some applicants choose to falsify information to manipulate the quote they receive.
To prevent this, insurers could use AI to analyse an applicant’s social media profiles and activity for confirmation that the information provided is not fraudulent. For example, in life insurance policies, social media pictures and posts may confirm whether an applicant is a smoker, is highly active, drinks a lot or is prone to taking risks. Similarly, social media may be able to indicate whether “fronting” (high-risk driver added as a named driver to a policy when they are in fact the main driver) is present in car insurance applications. This could be achieved by analysing posts to see if the named driver indicates that the car is solely used by them, or by assessing whether the various drivers on the policy live in a situation that would permit the declared sharing of the car.
Claims Management & Fraud Prevention
Insurance carriers can greatly benefit from the recent advances in artificial intelligence and machine learning. A lot of approaches have proven to be successful in solving problems of claims management and fraud detection. Claims management can be augmented using machine learning techniques in different stages of the claim handling process. By leveraging AI and handling massive amounts of data in a short time, insurers can automate much of the handling process, and for example fast-track certain claims, to reduce the overall processing time and in turn the handling costs while enhancing customer experience.
The algorithms can also reliably identify patterns in the data and thus help to recognize fraudulent claims in the process. With their self-learning abilities, AI systems can then adapt to new unseen cases and further improve the detection over time. Furthermore, machine learning models can automatically assess the severity of damages and predict the repair costs from historical data, sensors, and images.
Two companies tackling the management of claims are Shift Technology who offer a solution for claims management and fraud detection and RightIndem with the vision to eliminate friction on claims. Motionscloud offer a mobile solution for the claims handling process, including evidence collection and storage in various data formats, customer interaction and automatic cost estimation. ControlExpert handle claims for the auto insurance, with AI replacing specialized experts in the long-run. Cognotekt optimize business processes using artificial intelligence. Therefore the current business processes are analyzed to find the automation potentials. Applications include claims management, where processes are automated to speed up the circle time and for detecting patterns that would be otherwise invisible to the human eye, underwriting, and fraud detection, among others. AI techniques are potential game changers in the area of fraud. Fraudulent cases may be detected easier, sooner, more reliable and even in cases invisible to the human eye.
Conclusion
Those who wish to defraud insurance companies currently do so by finding ways to “beat” the system. For some uses of AI, fraudsters can simply modify their techniques to “beat” the AI system. In these circumstances, whilst AI creates an extra barrier to prevent and deter fraud, it does not eradicate the ability to commit insurance fraud. However, with other uses of AI, the software is able to create larger blockades through its use of “big data”. It can therefore provide more preventative assistance. As AI continues to develop, this assistance will become of greater use to the insurance industry in their fight against fraud.
Related Posts
AIQRATIONS
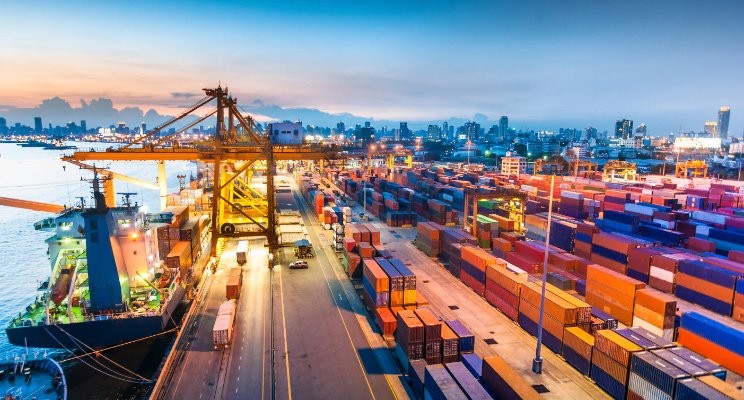
Fluid Supply Chain Transformation = AI + Automation
Add Your Heading Text Here
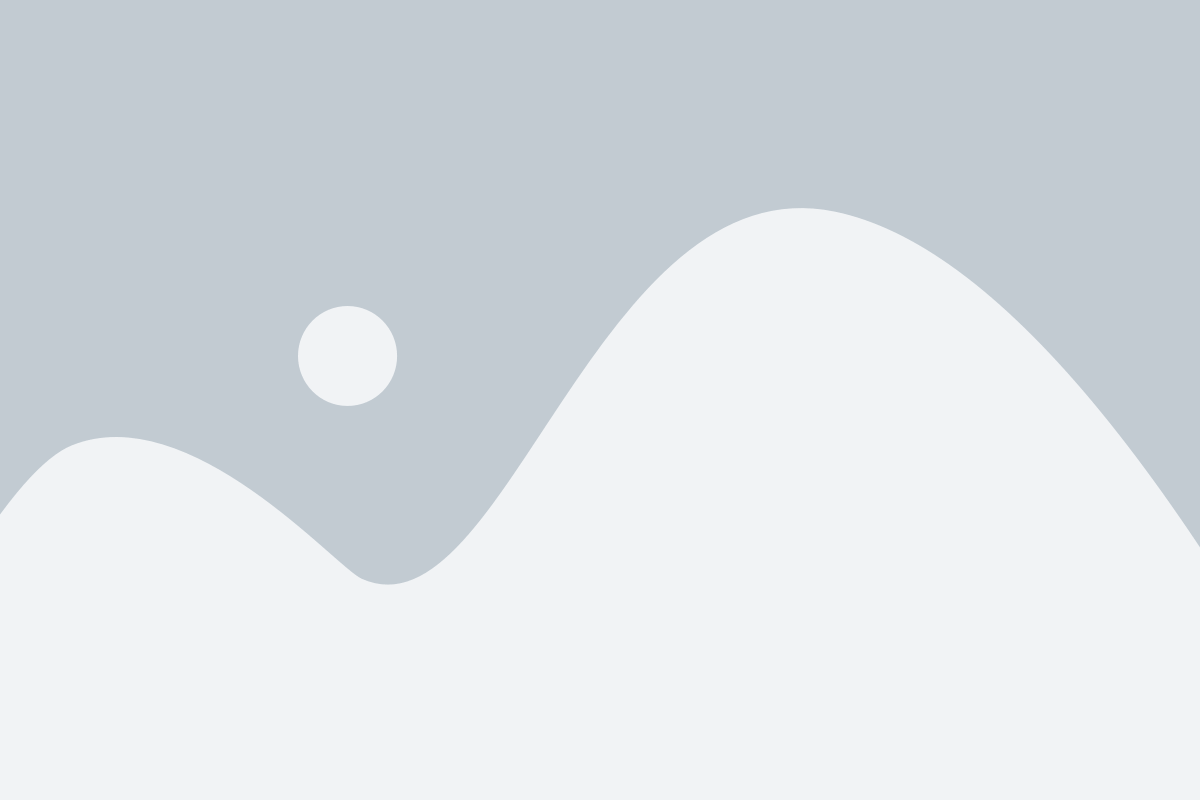
Rapidly evolving technology and a digitally focused world have opened the door for a new wave of automation to enter the workforce. Robots already stand side-by-side with their human counterparts on many manufacturing floors, adding efficiency, capacity (robots don’t need to sleep!) and dependability. Add in drones and self-driving vehicles and it’s no wonder many are questioning the role of humans going forward.
Supply chains, although automated to a degree, still face challenges brought about by the amount of slow, manual tasks required, and the daily management of a complex web of interdependent parts. The next generation of process efficiency gains and visibility could be on your doorstep with artificial intelligence in supply chain management, if only you’d let the robots automatically open it for you.
Robotic Process Automation
RPA works by automating the end-to-end supply chain, enabling the management of all tasks and sections in tandem. It allows you to spend less time on low value, high frequency activities like managing day-to-day processes, and provides more time to work on high value, exception-based requirements, which ultimately drives value for the entire business.
PwC estimates businesses could automate up to 45% of current work, saving $2 trillion in annual wages. “In addition to the cost and efficiency advantages, RPA can take a business to the next level of productivity optimization,” the firm says. Those ‘lights out’ factories and warehouses are becoming closer to a reality.
Four key elements need to be in place for you to take full advantage of robotic process automation in your supply chain:
- robots for picking orders and moving them through the facility;
- sensors to ensure product quality and stock;
- cognitive learning systems;
- and, artificial intelligence to turn processes into algorithms to guide the entire operation.
In addition, you’ll need strong collaboration internally and among suppliers and customers to tie all management systems back to order management and enterprise resource planning platforms.
Artificial Intelligence In Supply Chain Automation
AI is changing the traditional way in which companies are operating. Siemens in its “lights out” manufacturing plant, has automated some of its production lines to a point where they are run unsupervised for several weeks.
Siemens is also taking a step towards a larger goal of creating Industrie 4.0 or a fully self-organizing factory which will automate the entire supply chain. Here, the demand and order information would automatically get converted into work orders and be incorporated into the production process.
This would streamline manufacturing of highly customized products.
Artificial Intelligence In Supplier Management And Customer Service
Organizations are also increasingly leveraging AI for supplier management and customer management. IPsoft’s AI platform, Amelia automates work knowledge and is able to speak to the customers in more than 20 languages. A global oil and gas company has trained Amelia to help provide prompt and more efficient ways of answering invoicing queries from its suppliers. A large US-based media services organization taught Amelia how to support first line agents in order to raise the bar for customer service.
Artificial Intelligence In Logistics & Warehousing
Logistics function will undergo a fundamental change as artificial intelligence gets deployed to handle domestic and international movement of goods. DHL has stated that its use of autonomous fork lifts is “reaching a level of maturity” in warehouse operations. The next step would be driver less autonomous vehicles undertaking goods delivery operations.
Artificial Intelligence In Procurement
AI is helping drive cost reduction and compliance agenda through procurement by generating real time visibility of the spend data. The spend data is automatically classified by AI software and is checked for compliance and any exceptions in real time. Singapore government is carrying out trials of using artificial intelligence to identify and prevent cases of procurement fraud.
The AI algorithm analyzes HR and finance data, procurement requests, tender approvals, workflows, non-financial data like government employee’s family details and vendor employee to identify potentially corrupt or negligent practices. AI will also take up basic procurement activities in the near future thereby helping improve the procurement productivity.
Artificial Intelligence in new product development
AI has totally overhauled the new product development process.by reducing the time to market for new products. Instead of developing physical prototypes and testing the same, innovators are now creating 3D digital models of the product. AI facilitates interaction of the product developers in the digital space by recognizing the gestures and position of hand. For example, the act of switching on a button of a digital prototype can be accomplished by a gesture.
AI In Demand Planning And Forecasting
Getting the demand planning right is a pain point for many companies. A leading health food company leveraged analytics with machine learning capabilities to analyze their demand variations and trends during promotions.
The outcome of this exercise was a reliable, detailed model highlighting expected results of the trade promotion for the sales and marketing department. Gains included a rapid 20 percent reduction in forecast error and a 30 percent reduction in lost sales.
AI in Smart Logistics
The impact of data-driven and autonomous supply chains provides an opportunity for previously unimaginable levels of optimization in manufacturing, logistics, warehousing and last mile delivery that could become a reality in less than half a decade despite high set-up costs deterring early adoption in logistics.
Changing consumer behavior and the desire for personalization are behind two other top trends Batch Size One and On-demand Delivery: Set to have a big impact on logistics, on-demand delivery will enable consumers to have their purchases delivered where and when they need them by using flexible courier services.
A study by MHI and Deloitte found more than half (51%) of supply chain and logistics professionals believe robotics and automation will provide a competitive advantage. That’s up from 39% last year. While only 35% of the respondents said they’ve already adopted robotics, 74% plan to do so within the next 10 years. And that’s likely in part to keep up with key players like Amazon, who have been leading the robotics charge for the past few years.
What is the mantra ?
These examples showcase that in today’s dynamic world, AI embedded supply chains offer a competitive advantage. AI armed with predictive analytics can analyze massive amounts of data generated by the supply chains and help organizations move to a more proactive form of supply chain management.
Thus, in this digital age where the mantra is “evolve or be disrupted”, companies are leveraging AI to reinvent themselves and scale their businesses quickly. AI is becoming a key enabler of the changes that businesses need to make and is helping them manage complexity of the constant digital change.
Related Posts
AIQRATIONS
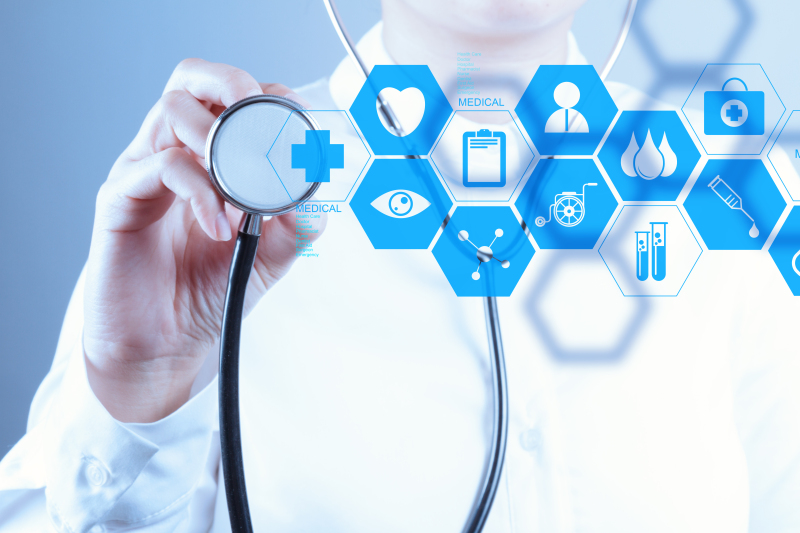
How Healthcare Industry will Benefit by Embracing Data Sciences
Add Your Heading Text Here
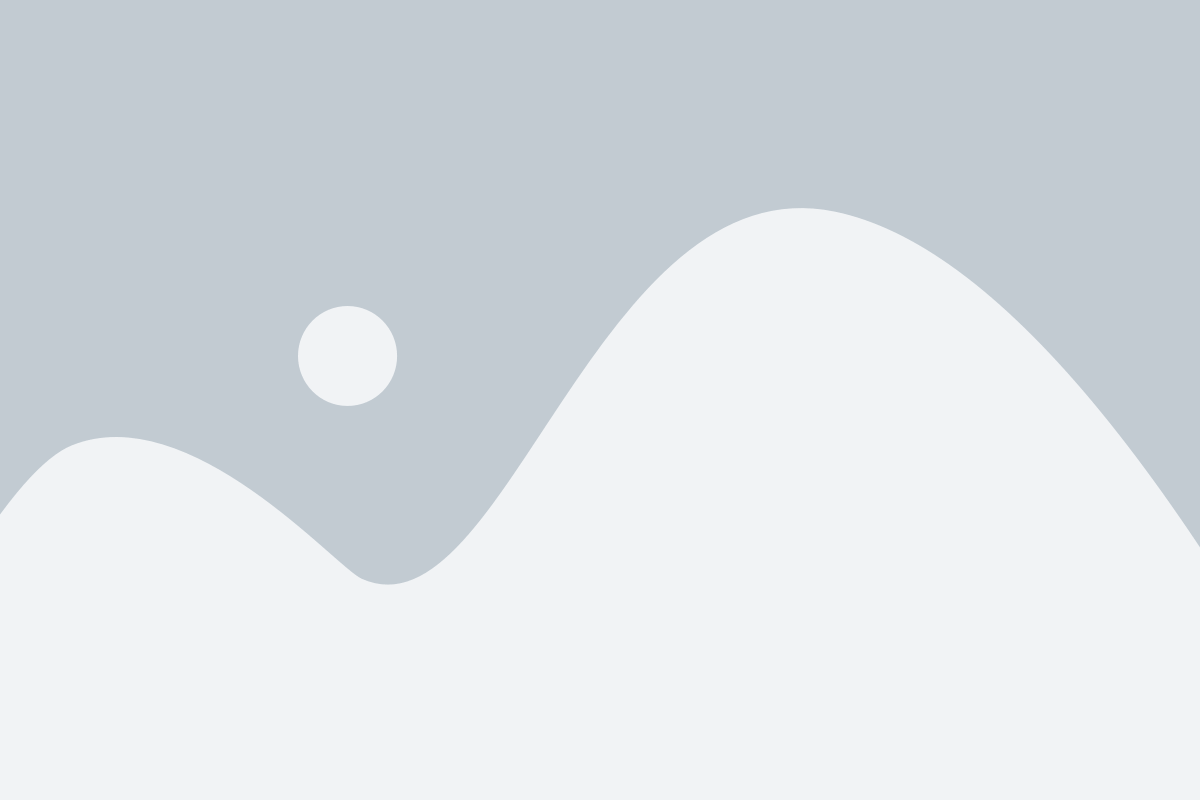
In the healthcare industry, what could be more important than having better healthcare outcomes? Each and every day healthcare workers around the globe are striving hard to find more ways of improving our lives. However, the world is changing, and frankly, at a faster rate than most of us can keep up. Intuition alone will no longer be enough for quality patient outcomes. The amount of healthcare data continues to mound every second, making it harder and harder to find any form of helpful information. Big Data is not to be romanticized; it can be a blessing and a curse. It can contribute to both the insight and the fog of visibility.
In truth, data science is proving invaluable to improving outcomes due to its ability to automate so much of the heavy lifting – in fast, scalable, and precise ways. All one has to do is look at our ability to predict epidemics, advance cures, and make patient stays in hospitals safer and more pleasant. In healthcare, data science should be seen as a beneficial intelligence rather than only artificial intelligence, providing an augmentation of services to the healthcare experts already in play.
Hospital Claims Data
In 2010, there were 35.1 million discharges with an average length of stay of 4.8 days according to the National Hospital Discharge Survey. That same survey went on to note that there were 51.4 million procedures performed. The National Hospital Ambulatory Medical Care Survey in 2011 stated the number of outpatient department visits were 125.7 million with 136.3 million emergency department visits. These are some of the basic figures showing the amount of care the U.S. health care system has provided. Using Data Science to annualize this sort of data allows healthcare providers to start building a new intuition built on a data narrative that could possibly help avoid the spread of diseases or address specific health threats. Using a combination of descriptive statistics, exploratory data analysis, and predictive analytics, it becomes relatively easy to identify the most cost-effective treatments for specific ailments and allows for a process to help reduce the number of duplicate or unnecessary treatments. The power in predicting a future state is in using that knowledge to change the behavior patterns of today.
Electronic Health Record (EHR)
Interoperable electronic health records (EHRs) for patient care hold tremendous potential to reduce the growth in costs. EHRs can help healthcare organizations improve chronic disease management, increase operating efficiencies, transform their finances, and improve patient outcomes. However, EHR implementations are in various stages of maturity across the country, and their benefits have not been fully realized. One of the primary challenges healthcare decision-makers face is how to make meaningful use of the data collected, available, and accessible in EHRs.
By optimizing the use of data accessible in EHRs, we can uncover hidden relationships and identify patterns and trends in this diverse and complex information to improve chronic disease management, increase operating efficiencies, and transform healthcare organizations’ finances.
Patient Behavior and Sentiment Data
A study by AMI Research suggests that “wearables” are expected to reach $52 million by 2019. Wearables monitor heart rates, sleep patterns, walking, and much more while providing new dimensions of context, geolocation, behavioral pattern, and biometrics. Combine this with the unstructured “lifestyle” data that comes across social media and you have a potent combination that is more than just numbers and tweets.
It is obvious that we will experience huge benefits from analyzing the in’s and out’s of healthcare data. In my judgment, we will continue to see a push for prevention over cure which puts predicting outcomes front and center. After all, catching things in the earlier stages is easier to treat and outbreaks can be more easily contained.
It may not resonate as widely today, but in the future we will look back on data science as something significant for healthcare. It is reasonable to expect that we will likely recover more quickly from illness and injury, live longer because of newly discovered drugs, and benefit from more efficient hospital surgeries – and in large part this will be because of how we analyze Big Data.
What makes living in the era of Big Data such a delight is that the healthcare industry is being pressed to find better tools, skills, and techniques to deal competently with the deluge of patient data and its inherent insights? When healthcare makes the choice to fully embrace data science, it will change the future for everyone.
Genomics
Inexpensive DNA sequencing and next-generation genomic technologies are changing the way healthcare providers do business. We now have the ability to map entire DNA sequences and measure tens of thousands of blood components to assess health.
Next-generation genomic technologies allow data scientists to drastically increase the amount of genomic data collected on large study populations. When combined with new informatics approaches that integrate many kinds of data with genomic data in Healthcare applications such as disease research, Prescription Effectiveness etc, we will better understand the genetic bases of drug response and disease. Researchers aim to achieve ultra-personalized healthcare. As a beginning, the FDA has already begun to issue medicine labels that specify different dosages for patients with particular genetic variants.
Predictive Analytics and Preventive Measures
Prevention is always better than cure. For the health-care industry, it also happens to save a lot of money. (The Centers for Medicaid and Medicare Services, for instance, can penalize hospitals that exceed average rates of readmission – indicating that they could be doing more to prevent medical problems.)
Take, for example, the partnership between Mount Sinai Medical Center and former Facebook guru Jeff Hammerbach. Mount Sinai’s problem was how to reduce readmission rates. Hammerbach’s solution was predictive analytics:
- In a pilot study, Hammerbach and his team combined data on disease, past hospital visits and other factors to determine a patient’s risk of readmission.
- These high-risk patients would then receive regular communication from hospital staff to help them avoid getting sick again.
Sinai isn’t alone. In 2008, Texas Health partnered with Healthways to merge and analyze clinical and insurance claims information. Their goal was the same – identify high-risk patients and offer them customized interventions.
Meanwhile, in 2013, data scientists at Methodist Health System are looking at accountable-care organization claims from 14,000 Medicare beneficiaries and 6,000 employees. Their aim? You guessed it. Predict which patients will need high-cost care in the future.
Patient Monitoring and Home Devices
Doctors can do a lot, but they can’t follow a patient around every minute of the day. Wearable body sensors – sensors tracking everything from heart rate to testosterone to body water – can.
Sensors are just one way in which medical technology is moving beyond the hospital bed. Home-use, medical monitoring devices and mobile applications are cropping up daily. A scanner to diagnose melanomas? A personal EEG heart monitor? No problem.
These gadgets are designed to help the patient, naturally, but they’re also busy harvesting data.
For example:
- Asthmapolis’s GPS-enabled tracker, already available by 2011, records inhaler usage by asthmatics. This information is collated, analyzed and merged with data on asthma catalysts from the CDC (e.g., high pollen counts in New England) to help doctors learn how best to prevent attacks.
- With Ginger.io’s mobile application, out in 2012, patients consent to have data about their calls, texts, location and movements monitored. These are combined with data on behavioral health from the NIH and other sources to pinpoint potential problems. Too many late-night phone calls, for instance, might signal a higher risk of anxiety attack.
- To improve patient drug compliance, Eliza, a Boston-based company, monitors which types of reminders work on which types of people. Smarter targeting means more compliance.
The Challenges Ahead
There are plenty of hurdles to creating a data-driven health care industry. Some are technical, some emotional. Health care providers have had decades to accumulate paper records, inefficiencies and entrenched routines. A remedy will not be quick.
And some say it shouldn’t. At least, not without a hard look at patient privacy, data ownership and the overall direction of U.S. health care.