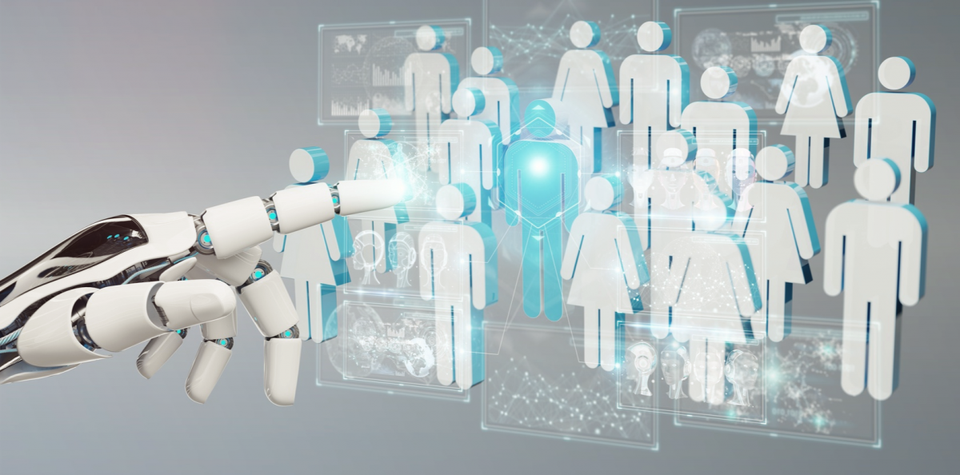
AI And Societal Impact – Addressing Large, Complex Unresolved Problems With AI
Add Your Heading Text Here
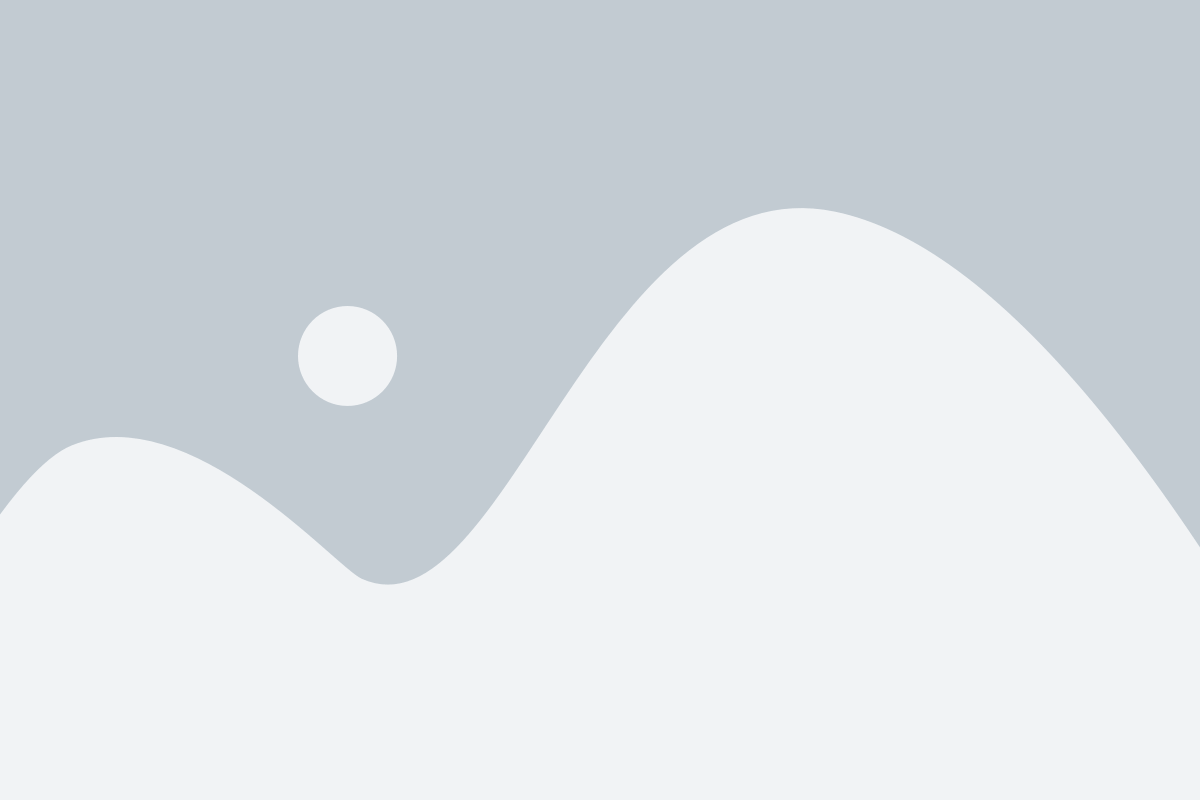
The idea that AI will conjure up an apocalyptic, robot-ruled future, where mechanical overlords govern humans is an extremely low probability event, even in the very distant future. In fact, not only are AI-driven interventions accelerating business outcomes – AI is also helping nations around the world find new avenues for enabling positive social outcomes for their people.
For all the evolution and development of humanity and technology over the years, our world still faces pressing systemic challenges that affect humanity at a large scale. From our complex and labyrinthine legal systems to the inefficiencies in our healthcare sector, large-scale problems still abound. The need of the hour is to better connect the people with the basic facilities they require. AI may not be a panacea in and of itself, but it offers a huge potential to improve the quality of life of people across the globe. Thankfully, today multiple nations have the intellectual capital – our peers in the software engineering and AI domains – that can bring substantial dividends for the population at large.
In this article, I will attempt to touch upon how AI can be used to address large, complex and unsolved problems and contribute to improving the quality of life for humanity. In keeping with WTISD’s topic for this year – Enabling the Positive Use of AI for All – I’ll share a social perspective on how AI-powered innovations can be hugely transformational to the world:
Improve Access to Healthcare Facilities
Available statistics show that over 45% of WHO Member States report to have less than 1 physician per 1000 people. (World Health Organization recommends a ratio of 1:1000). When you add to that the inequitable spread of doctors across certain countries, we have a poorly served population. While the life expectancy at a global level is 72 years (average across both males and females), the disparity between regions can be startling. For instance, the average in the WHO’s Africa region is a low 61.2 years. By imbibing AI, we can deliver an exponential improvement in health outcomes by improving medical adherence to reduce readmissions, tracking patient medical histories, improving access to physicians, reducing the time spent in clinics and prescribing personalized treatment pathways. Using AI, we can:
- Identify high population density areas that are currently underserved by hospitals. This can provide policymakers with inputs on how they can improve the deployment and availability of doctors, medical equipment and medication
- Leverage early warning signals through alternative mediums such as social media tracking for public health studies to provide guided diagnosis and interventions
- Create a digital record of patients’ medical histories and their clinical notes and use that as a reference for prescribing evidence-based treatment options and developing tailored treatment pathways
- Improve patient medical adherence by identifying individuals without health insurance, providing coverage and incentivizing the use of appropriate medication and treatment
- Speed up routine clinical processes such as scanning and annotating X-Rays and CT-Scans using computer vision and prescribing actions to physicians.
Revamp the Education System
The education system is undeniably critical for shaping future generations. However, both quality of and access to education is incredibly disparate across the developed and developing worlds. Curricula can often be outdated, thus not providing students with the skills they need for their careers. Problems abound in the education sector – from a high level of student dropouts, quality and methodology of teaching and lack of workforce readiness among students. While policymakers mull over how education can be made more contemporary and effective, AI can help provide guided interventions in the field of education by:
- Mapping the aptitude and interest of students in schools and universities with skills that are demanded by the market. This will help provide prescriptive career guidance that will be beneficial to both the employers and the future workforce
- Tracking the demand for skills in the market and the educational infrastructure available to supply those skills, through a Skills Repository. This will help keep education concurrent with current market demands and ensure much better alignment between academia and corporates
- Automate routine, time-consuming tasks – from creating and grading test papers, developing personalized benchmarks for each student, identifying gaps in student development, tracking aptitude and attentiveness within each subject, and enabling teachers to focus on curriculum development, coaching and mentoring, and improving behavioral and personality aspects of students
- Identify potential school and university-level dropouts and their root-causes so educational institutions can take proactive steps to ensure student retention and course completion.
Address Legal and Law Enforcement Challenges
Globally, we face structural issues in areas of law enforcement and jurisprudence. Globally the average police-to-people ratio is 1 police personnel per 604 people, which is lower than the UN recommended standard of 1 per 454. Poor law enforcement eventually lends itself to a high crime rate and an overburdened legal system. AI can be a hugely pertinent gamechanger for global governance systems and help law enforcement officers improve surveillance by augmenting police efforts, automate a variety of routinized legal tasks and improve transparency in governance. By bringing the potential of AI in law enforcement, we can offer:
- Surveillance and identification of wrongdoers; areas recognized for high criminal activity can be done through computer vision
- Review and summary-creation of long drawn cases and their history can be done through natural language processing and voice recognition
- Routing Right-to-Information and governance-related citizen requests through intelligent bots, thus making it more efficient to get critical information
- Employ Anomaly Detection frameworks to surface fraudulent transactions – especially among land deals.
A global population of over 7.7 billion people, distributed across a huge landmass throws up a sizeable challenge when it comes to scalability. With many individual nations crippled by the inability to serve their populations, their public services need technology-centric solutions that are scalable and intelligent at the same time. Artificial intelligence will effectively address a number of these problems which are of socio-economic importance, and will go a long way in improving the quality of life of humanity at large. To enable this, public services need to act sooner rather than later and ramp up their data sets, identify and onboard technology, innovation and research partners for ideating and applying AI techniques that can power humanity’s next big leap.
Related Posts
AIQRATIONS
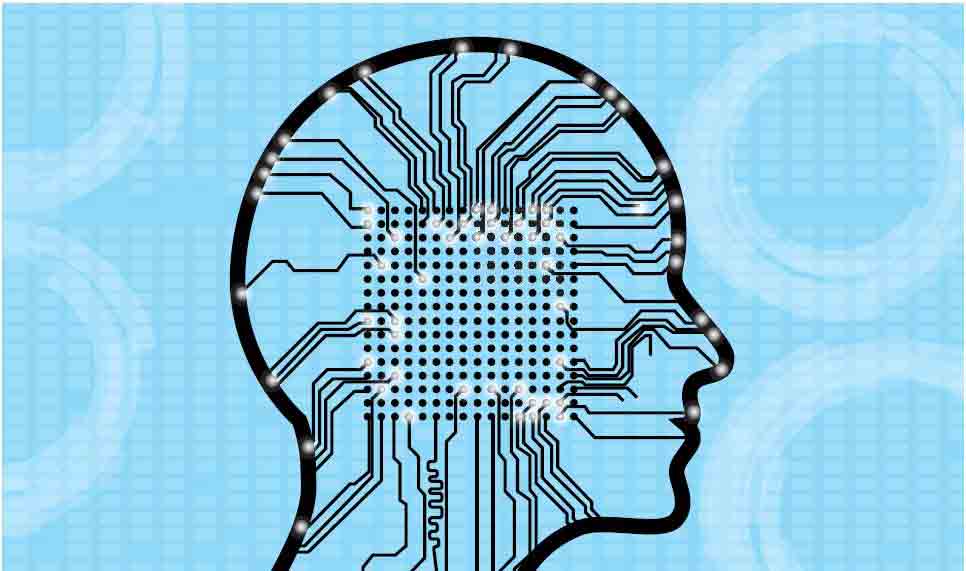
Detecting depression early with AI
Add Your Heading Text Here
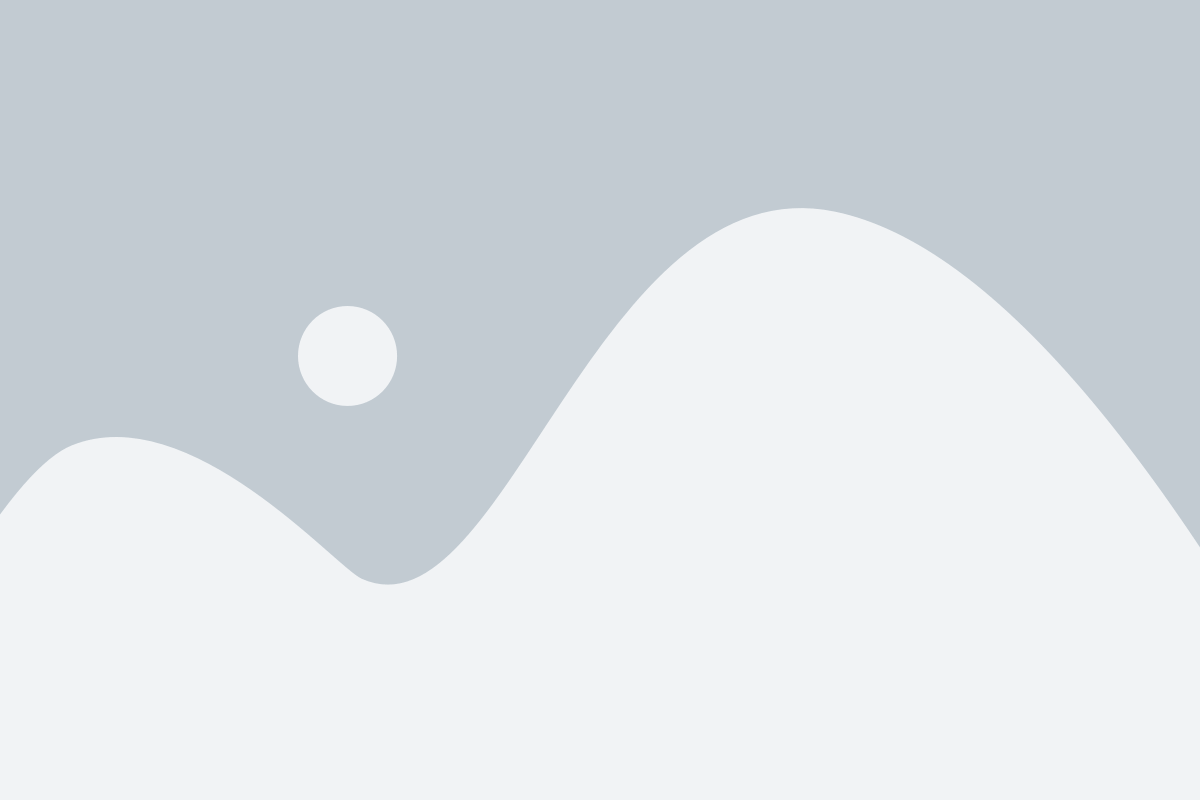
Can artificial intelligence be used in conjunction with mental health practitioners to better discover and address cases of depression and other mental illnesses? We have seen impactful examples of AI being applied to a range of health and wellness use cases, with the potential to help improve the lives of millions of people. Recent advances in this breakthrough technology have also shown the great promise that AI holds in helping individuals and doctors better manage cases and mitigate adverse health impacts of depression and other illnesses that plague the human mind.
Depression is a key problem faced by humans across the world — from milder forms of repetitive depressive mood states to more deeper-seated clinical depression. While we have seen significant improvements in our theoretical understanding of clinical depression, its causes and how to effectively mitigate it, the problem continues to affect millions of people. Psychiatric conditions such as this do not have vaccines or proven preventive medication. So much of the success in helping those with clinical depression hinges on early detection, continuous monitoring and scientific counselling and – in certain cases with a physician’s prescription – medication.
Health Data
At the macro-level, the downside of not managing clinical depression, especially for big populations, can be devastating. According to a study in 2015, it is estimated that approximately 216 million individuals across the globe (3% of total global population) have been affected with clinical depression – with a usual onset in the 20s and 30s. A lack of concerted focus on overall mental health and well-being can lead to outcomes as diverse as high suicide and crime rates to a reduction in national productivity and high levels of substance abuse and addiction.
Today we have a huge repository of health data. By applying machine learning and deep learning algorithms, AI can help individuals and physicians detect early indications of the onset of depression, monitor the progress of patients using medical and other therapeutic options and provide continuous support.
Early Detection
By using AI to understand and highlight speech patterns, use of specific words in text communication and even facial expression, we could possibly improve the speed at which such cases are uncovered. For achieving this, it is important that doctors and machine learning experts work together to identify patterns that have a strong correlation with clinical depression.
A research led by Dr Fei-Fei Li of Stanford University found that using facial and speech recognition data and algorithmic models, it might be possible to detect cases where there is an onset of clinical depression. By leveraging a combination of facial expressions, voice intonation and spoken words, their research was able to diagnose if an individual was suffering from depression with 80% accuracy.
Facebook too has begun piloting its own machine learning algorithm to go through posts to identify linguistic red flags that are indicative of depression. In early testing, the algorithm was said to perform just as well as existing questionnaires that are used to identify depression.
Therapist Interaction
Can AI inform therapists and help them do their job better? With the high and growing number of mental illnesses, the limited number of therapists need AI interventions to help make their job easier while helping improve health outcomes. AI could change the game in the therapy arena by augmenting therapists in identifying subtle signs showcased by patients – such as intonations in voice and facial expressions – which therapists might sometimes miss. Further, AI can also provide guided suggestions to them in providing effective treatment options.
An interesting innovation in this area comes from Ginger. This technology company combines a strong clinician network with machine learning to provide patients with the emotional support that they need at the right time – offering 24/7 availability for cognitive behaviour therapy (CBT), alongside mindfulness and resilience training.
On the back-end, AI is using each patient’s progress to inform its capabilities and make it smarter and more scalable. AI also helps Ginger match its users with a team of three emotional support coaches that it determines to be best suited for the individual.
Continuous Support
The stigma associated with mental illnesses often acts as a bottleneck in uncovering which individuals are suffering from it. Having a non-human companion that can provide human-like compassion can be a possible game changer in helping counsel patients suffering from depression and help them in the rehabilitation process. There are also advantages associated with AI being that counsellor – as it offers higher availability at a much lower cost.
Woebot is an excellent example and a bellwether for what AI could accomplish in the future for this use case. Created by Dr Alison Darcy, Woebot is a computer program that can be integrated with Facebook and is aimed at replicating the typical conversation a patient would have with a therapist. The tool asks about a patient’s mood and thoughts and offers evidence-based CBT. Just like a therapist would in a real-life situation, Woebot ‘listens’ actively in a conversation and learns about the patient to offer interactions more tailored to an individual’s unique situation.
Last year, researchers from the Massachusetts Institute of Technology presented a paper detailing a neural-network model that can be run over raw text and audio data to discover speech patterns that could be indicative of depression, without the need for a pre-set questionnaire. This immense breakthrough will hopefully ripple into more similar research, which could help aid those who suffer from cognitive conditions such as dementia.
While the potential for AI in the field of mental health is massive, it is also imperative that we consider the aspect of protecting the privacy and identity of those included in studies pertaining to this domain. Maintaining data security norms is an extremely crucial part of any AI exercise and in this case, it is even more paramount. To protect the sanctity of AI interventions and participating patients providing the data, it is extremely important to address concerns around data and information security first, before addressing any future use cases in this technology.
Related Posts
AIQRATIONS

3 Ways To Human Centric AI
Add Your Heading Text Here
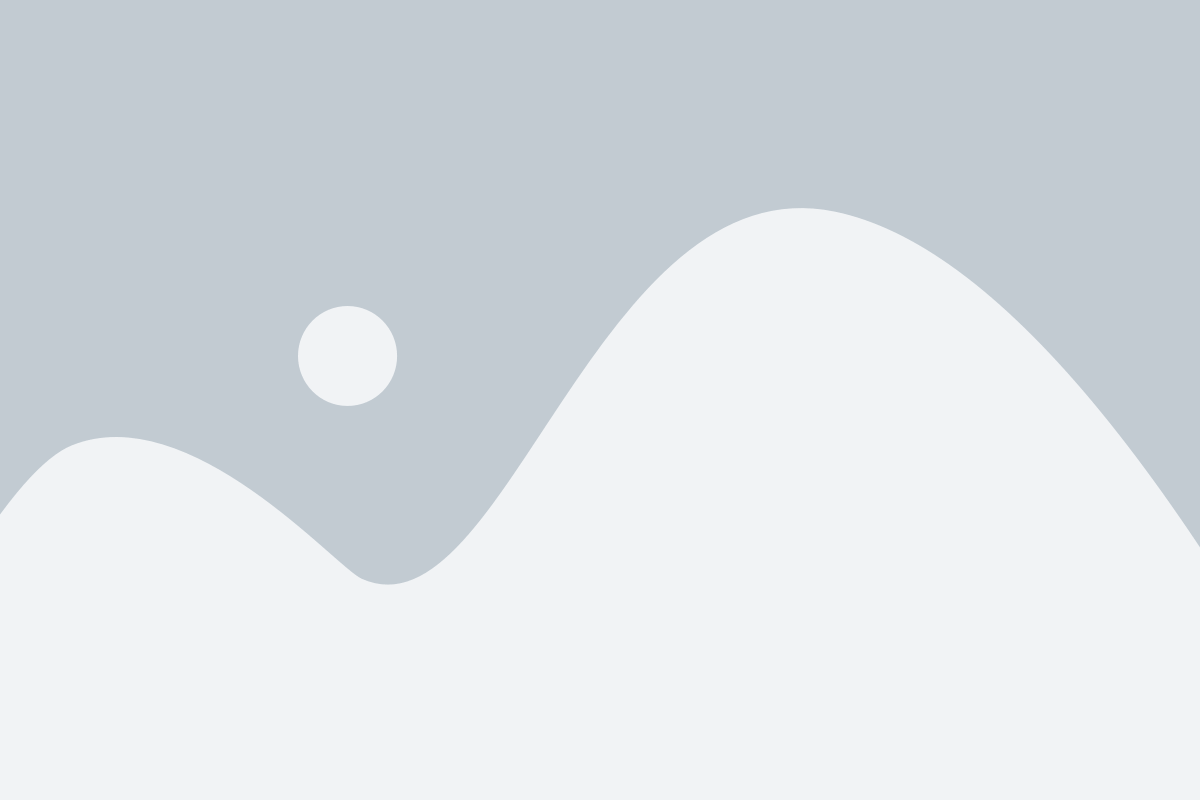
Three Ways to Facilitate a Symbiotic Relationship Between Cognitive Intelligence and Behavioral Sciences
After every conference I speak at about the transformative power of Artificial Intelligence and its potential to unlock untold business value, the one question that often crops up from the audience is.. if AI is expected to perform much of the grunt work in the enterprise world, what scope is there for the so-called ‘human’ qualities?
Is the future of business and technology so deeply intertwined that it leaves virtually no scope in the future for the vagaries of human intelligence and behavior? The answer is quick and simple – absolutely not. Artificial Intelligence, while a great paradigm-shifter in the world of business, is still one of the tools that will be used by humans for making better decisions. At the end of the day, AI will still be developed and used by humans. And maintaining the ‘human element’ in the way it is made, delivered, used and improved will most certainly make it a lot more successful. AI exists to make human life simpler and richer – and hence it is critical that AI practitioners and data scientists adopt a human-centric approach to its development, deployment and adoption. Even the best AI will become quickly redundant without inputs from real humans on how to accelerate strategic decisions and processes.
How do we then build in that ‘human element’ into our Artificial Intelligence tools? This is where humanities-centric subjects of design and behavioral science come into the picture. What is behavioral science? Simply put, it is the study of internal cognitive processes of humans and societies and how these processes manifest into external perceptible and imperceptible actions and interactions. Behavioral science typically stands at a nexus of various subjects – borrowing aspects from sociology, anthropology, psychology and even economics and political science. Its interdisciplinary nature precludes the scale of impact it can have if applied correctly. In technology, and specifically in AI, behavioral science will and should impact how we build, use and interact with technology.
I see primarily three key areas where the symbiosis of AI-led cognitive intelligence systems and behavioral science can unlock massive value for enterprises that marry these two starkly different, but extremely complementary fields of study:
Appeal to the Non-Conscious
We have known for nearly a century now that a large majority of human biases, inferences, preferences and reactions are largely controlled by the dark recesses of our non-conscious brain. For technologists to build successful AI products, that are widely adopted and used they need to reach out inside the non-conscious parts of the human brain and orchestrate responses from there.
In AI technology specifically, user adoption is often the difference between make and break for products. Numerous AI products are mostly informed by the data they gather from human actions and their preferences. This data feeds the algorithms running in the background and makes them more sophisticated to better understand their human overlords. To that end, AI products need to have a strong underpinning in behavioral science, so that they can appeal to the non-conscious and improve adoption.
Take for example the work done by Nir Eyal for his book, ‘Hooked: How to Build Habit Forming Products.’ In the book, Eyal writes about multiple ways in which human subjects get applied to technology development. One of them is the Hook canvas – a loop comprising triggers, actions, rewards and investments – which are the cornerstone features of every addictive software you’ve used – from Facebook to Instagram, Snapchat and YouTube. Another is the idea of using the trinity of emotion, features and incentives – extremely relevant ideas to anyone working on building AI products. Another example comes from Worxogo – an Indian startup that employs behavioral design, neuroscience in tandem with predictive analytics to enhance employee performance through nudges to the non-conscious.
Build with Humans
Not only is AI built to serve humans, it is also built by humans. To that end, it becomes extremely important to consider what emotional triggers help define what we build and how we build it. Again, behavioral science practitioners have a key role to play in order to engage empathy in defining the requirements and going about the development of AI. Learnings from behavioral science can bring to light immeasurably important interventions for how we manage and lead teams, collaborate between a team and across multiple teams – all while maintaining a high level of motivation by appealing to a higher sense of purpose. It is worth examining how something as simple as empathy can be extremely valuable in how we build software. For instance, with improved self-awareness and empathy, developers can feel an intrinsic desire to write cleaner code while maintaining proper documentation. Also, given that AI is largely deployed using the DevOps methodology – empathy can be the difference between whether we can build a trust-based bridge between how we build, deploy and automate releases faster.
Beyond the ‘how’ of AI development, behavioral science can also contribute meaningfully to the ‘what’. Currently a lot of concern around AI is related to ethics – will AI lead to loss of meaningful work for humans? What data privacy issues can rear their head when we deploy large-scale data capture systems to improve our algorithms? We need to move the dial from apathy to empathy in the process of conceptualizing software – and knowledge of behavioral science will undoubtedly help AI practitioners develop more responsible AI.
Artificial Emotional Intelligence
The third key application of behavioral science – and possibly the most game-changing of the lot is – how can we apply behavioral science to make our systems more ‘human’? Is it possible to add a dash of EQ to these high IQ systems?
I certainly think there is a huge scope for developing AI that has a strong human bent. Consider the applications we are building today with AI and robotics – companions for the elderly, coaching apps for autistic children, even something as comparatively mundane is chatbots for customer service. Behavioral science holds the key to achieving the holy grail of how we can better balance the human-machine equation, by infusing human qualities into artificial systems.
To enable this, it is important to know who we are building for and what are their intrinsic and non-conscious needs. Behavioral science holds the clues that can complement AI’s ability to eliminate biases, while serving the emotional needs of humans. For example, StressSense tracks when people are highly stressed and helps them avoid anxious situations. This kind of breakthrough research can help in multiple AI applications, teaching them how to behave with humans, while ensuring a strong impact.
As technology providers and businesses work together to build transformational artificial intelligence systems and data science teams, it is very important to consider the human element. These teams would do well to develop a better understanding of whom the AI is built for and how it is used – through techniques offered by behavioral science. Balancing the human-machine equation and powering a complementary relationship between AI systems and the people who use them necessitates an infusion of behavioral science into the process. Ultimately, for AI to succeed, we need both – the foresight of technology as well as the insight of humans.
Related Posts
AIQRATIONS
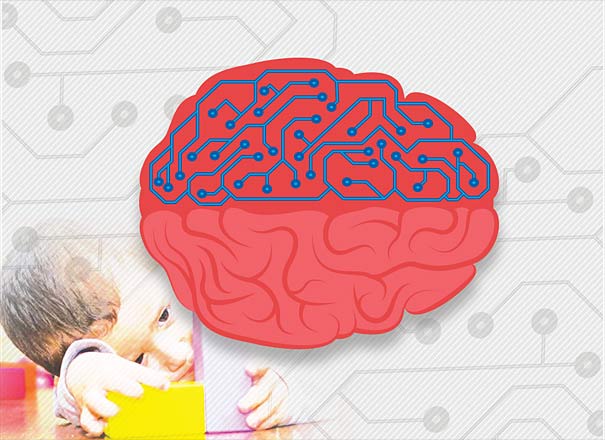
Autism care with AI
Add Your Heading Text Here
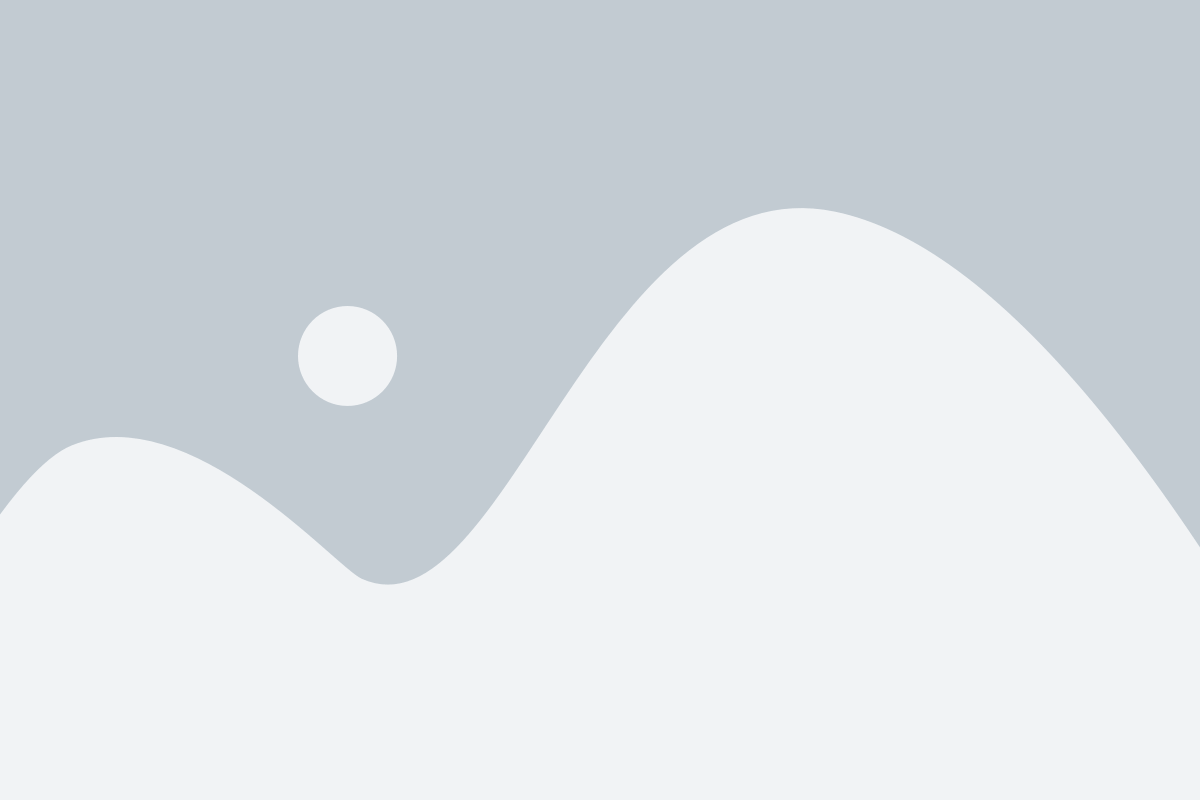
In these columns, I have earlier attempted to highlight the possibilities of multiple game-changing applications in the field of artificial intelligence (AI) that hold the potential to deliver positive benefits to humanity. AI can do much more beyond enabling a transformative business impact. Every technological advancement brings with it an opportunity to deliver positive changes to society. It is no different with AI. With massive increases in computing power and data deluge, it is possible for AI to be a harbinger of change for the society we live in.
A complex problem that we see is Autism Spectrum Disorder. As of 2015, this neurodevelopment disorder is estimated to have affected the lives of 24.8 million people globally. Worse, despite all the advances in medical sciences, there is no conclusive understanding of its causes and cures. While we do see cases where autism gets resolved, we are still unable to point concretely to any medical option that works better than others.
At best, autism can be managed – possibly through a mix of early diagnosis and continuous therapy. Thankfully, we live in an era where the awareness of autism is on the rise and the associated stigma is declining. By harnessing the power of AI to detect and manage cases of autism, we could potentially help those suffering from it lead a fulfilling life of dignity and respect.
While not conclusively proven, it is likely that an early diagnosis of autism is hugely beneficial for managing it in the future. An early detection would also help the people around these children be better attuned to their condition and enable them to set an environment that is conducive to their development.
One such application is applying AI to analyse vocal and behavioural cues exhibited by children. Stephen Sheinkopf — an autism researcher and psychologist at the Brown University Center for the Study of Children at Risk — believes anomalous crying patterns of babies might serve as an early warning sign of autism.
Neurological cues present in the acoustic features of cries — pitch, energy and resonance — might hold the key to early detection. Combining vocal, behavioural and physiological data, we might be able to piece together a pathway to early detection. That’s where AI and machine learning can add really great value, in integrating these disparate pieces of information that might otherwise be hard to make sense of.
An excellent example of this in action is Chatterbaby — conceptualised and developed by Ariana Anderson, a computational neuropsychologist at UCLA. Earlier, functionality enabled identification of potential causes for why a baby is crying by monitoring crying patterns. In addition, Chatterbaby is also attempting to identify if there are discernible differences in the crying patterns of autistic children and neurotypical ones — ultimately aiming to isolate the characteristics of each group to detect autism early.
Early ‘Testing’
Researchers in the UK and Italy have turned to AI for developing what might be the world’s first ‘test’ for autism. In their study, they leveraged AI to compare the protein levels in the blood samples of two groups of children — one group comprising 38 children diagnosed with autism, and the other with 31 children without the diagnosis. Their findings helped develop an algorithm that could test autism — with a 90% accuracy for which children have autistic spectrum disorder and an 87% accuracy for which children do not have it.
Another example is through tracking changes in brain function of six-month-old babies, which researchers from UNC Chapel Hill and Washington University’s School of Medicine believe can help early detection. They recently published a paper wherein they examined the brain scans of 59 high-risk babies to understand the connections and interactions between different regions of the brain. Post this, they analysed the brain scans of the 11 babies that were eventually diagnosed with autism.
By combining the data with AI and deep learning, they developed an algorithm able to detect the possibility of autism with an accuracy of 9 out of 11.
Chatbots and Virtual Assistants
Across studies, we see children with ASD have high levels of comfort with computers due to their predictable and logical nature. Autistic children can perceive humans to be emotional and unpredictable but computer-based systems (even those with human expression) to be rational and non-judgmental.
Systems such as chatbots and social robots can help capture and track the progress of autistic children, continuously monitor their social behaviours and make quick, informal assessments in school and at home. The big promise of these systems is allowing autistic children the opportunity to navigate social interactions, unfamiliar environments while aiding them to reach the developmental goals usually set for neurotypical children.
An example of this is an app called Companion, featuring a virtual assistant named Abby. Abby helps identify interests and needs of autistic children and provides support throughout the day. Beyond this app, Identifier identifies talents of autistic children through interactive game-play; eventual results are compiled onto a dashboard detailing the skills and shortcomings of the child.
A Strict No-No
While AI can bring these benefits, it is also important to ensure that these systems are designed with empathy for the autistic population. For instance, the use of bright, jarring colours is an absolute no-no for the design of such applications. Secondly, virtual agents should be enabled to speak in simple language, without the use of idioms, euphemisms or figures of speech to help such people understand instructions much better.
For a problem that has no apparent cause or cure, AI could be a breakthrough in improving the quality of life of those who suffer from autism and the people around them. By using AI for early diagnosis, disease management and people enablement, we would be able to help bring dignity to the lives of affected people.
Related Posts
AIQRATIONS
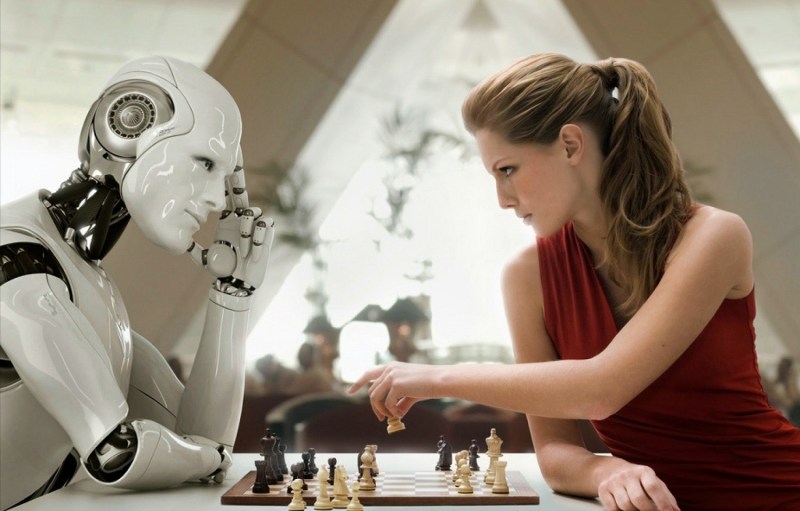
AI & Humanity – Existential Threat or Co-exist Attainability?
Add Your Heading Text Here
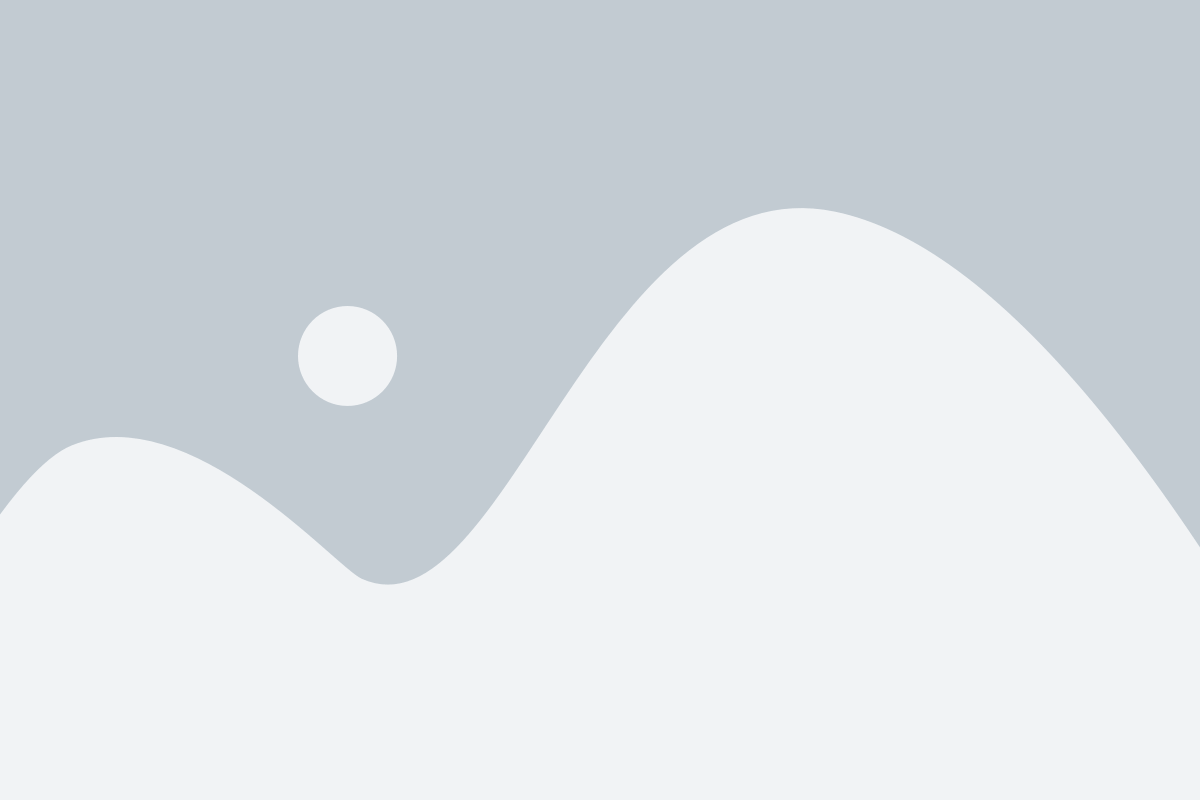
While some predict mass unemployment or all-out war between humans and artificial intelligence, others foresee a less bleak future. A future looks promising, in which humans and intelligent systems are inseparable, bound together in a continual exchange of information and goals, a “symbiotic autonomy.” If you may. It will be hard to distinguish human agency from automated assistance — but neither people nor software will be much use without the other.
Mutual Co-existence – A Symbiotic Autonomy
In the future, I believe that there will be a co-existence between humans and artificial intelligence systems that will be hopefully of service to humanity. These AI systems will involve software systems that handle the digital world, and also systems that move around in physical space, like drones, and robots, and autonomous cars, and also systems that process the physical space, like the Internet of Things.
I don’t think at AI will become an existential threat to humanity. Not that it’s impossible, but we would have to be very stupid to let that happen. Others have claimed that we would have to be very smart to prevent that from happening, but I don’t think it’s true.
If we are smart enough to build machine with super-human intelligence, chances are we will not be stupid enough to give them infinite power to destroy humanity. Also, there is a complete fallacy due to the fact that our only exposure to intelligence is through other humans. There are absolutely no reason that intelligent machines will even want to dominate the world and/or threaten humanity. The will to dominate is a very human one (and only for certain humans).
Even in humans, intelligence is not correlated with a desire for power. In fact, current events tell us that the thirst for power can be excessive (and somewhat successful) in people with limited intelligence.
You will have more intelligent systems in the physical world, too — not just on your cell phone or computer, but physically present around us, processing and sensing information about the physical world and helping us with decisions that include knowing a lot about features of the physical world. As time goes by, we’ll also see these AI systems having an impact on broader problems in society: managing traffic in a big city, for instance; making complex predictions about the climate; supporting humans in the big decisions they have to make.
Intelligence of Accountability
A lot of companies are working hard on making machines to be able to explain themselves — to be accountable for the decisions they make, to be transparent. A lot of the research we do is letting humans or users query the system. When Cobot, my robot, arrives to my office slightly late, a person can ask , “Why are you late?” or “Which route did you take?”
So they are working on the ability for these AI systems to explain themselves, while they learn, while they improve, in order to provide explanations with different levels of detail. People want to interact with these robots in ways that make us humans eventually trust AI systems more. You would like to be able to say, “Why are you saying that?” or “Why are you recommending this?” Providing that explanation is a lot of the research that is being done, and I believe robots being able to do that will lead to better understanding and trust in these AI systems. Eventually, through these interactions, humans are also going to be able to correct the AI systems. So they are trying to incorporate these corrections and have the systems learn from instruction. I think that’s a big part of our ability to coexist with these AI systems.
The Worst Case Contingency
A lot of the bad things humans do to each other are very specific to human nature. Behavior like becoming violent when we feel threatened, being jealous, wanting exclusive access to resources, preferring our next of kin to strangers, etc were built into us by evolution for the survival of the species. Intelligent machines will not have these basic behavior unless we explicitly build these behaviors into them. Why would we?
Also, if someone deliberately builds a dangerous and generally-intelligent AI, other will be able to build a second, narrower AI whose only purpose will be to destroy the first one. If both AIs have access to the same amount of computing resources, the second one will win, just like a tiger a shark or a virus can kill a human of superior intelligence.
In October 2014, Musk ignited a global discussion on the perils of artificial intelligence. Humans might be doomed if we make machines that are smarter than us, Musk warned. He called artificial intelligence our greatest existential threat.
Musk explained that his attempt to sound the alarm on artificial intelligence didn’t have an impact, so he decided to try to develop artificial intelligence in a way that will have a positive affect on humanity
Brain-machine interfaces could overhaul what it means to be human and how we live. Today, technology is implanted in brains in very limited cases, such as to treat Parkinson’s Disease. Musk wants to go farther, creating a robust plug-in for our brains that every human could use. The brain plug-in would connect to the cloud, allowing anyone with a device to immediately share thoughts.
Humans could communicate without having to talk, call, email or text. Colleagues scattered throughout the globe could brainstorm via a mindmeld. Learning would be instantaneous. Entertainment would be any experience we desired. Ideas and experiences could be shared from brain to brain.
We would be living in virtual reality, without having to wear cumbersome goggles. You could re-live a friend’s trip to Antarctica — hearing the sound of penguins, feeling the cold ice — all while your body sits on your couch.
Final Word – Is AI Uncertainty really about AI ?
I think that the research that is being done on autonomous systems — autonomous cars, autonomous robots — it’s a call to humanity to be responsible. In some sense, it has nothing to do with the AI. The technology will be developed. It was invented by us — by humans. It didn’t come from the sky. It’s our own discovery. It’s the human mind that conceived such technology, and it’s up to the human mind also to make good use of it.
I’m optimistic because I really think that humanity is aware that they need to handle this technology carefully. It’s a question of being responsible, just like being responsible with any other technology every conceived, including the potentially devastating ones like nuclear armaments. But the best thing to do is invest in education. Leave the robots alone. The robots will keep getting better, but focus on education, people knowing each other, caring for each other. Caring for the advancement of society. Caring for the advancement of Earth, of nature, improving science. There are so many things we can get involved in as humankind that could make good use of this technology we’re developing.
Related Posts
AIQRATIONS
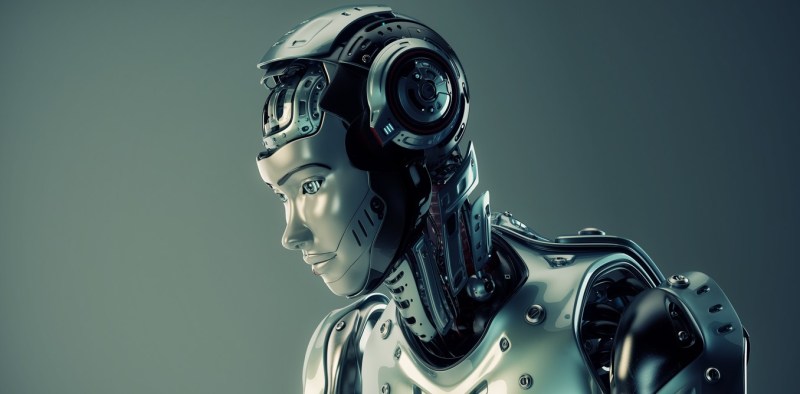
Can AI and Eternal Humanity both Co-exist
Add Your Heading Text Here
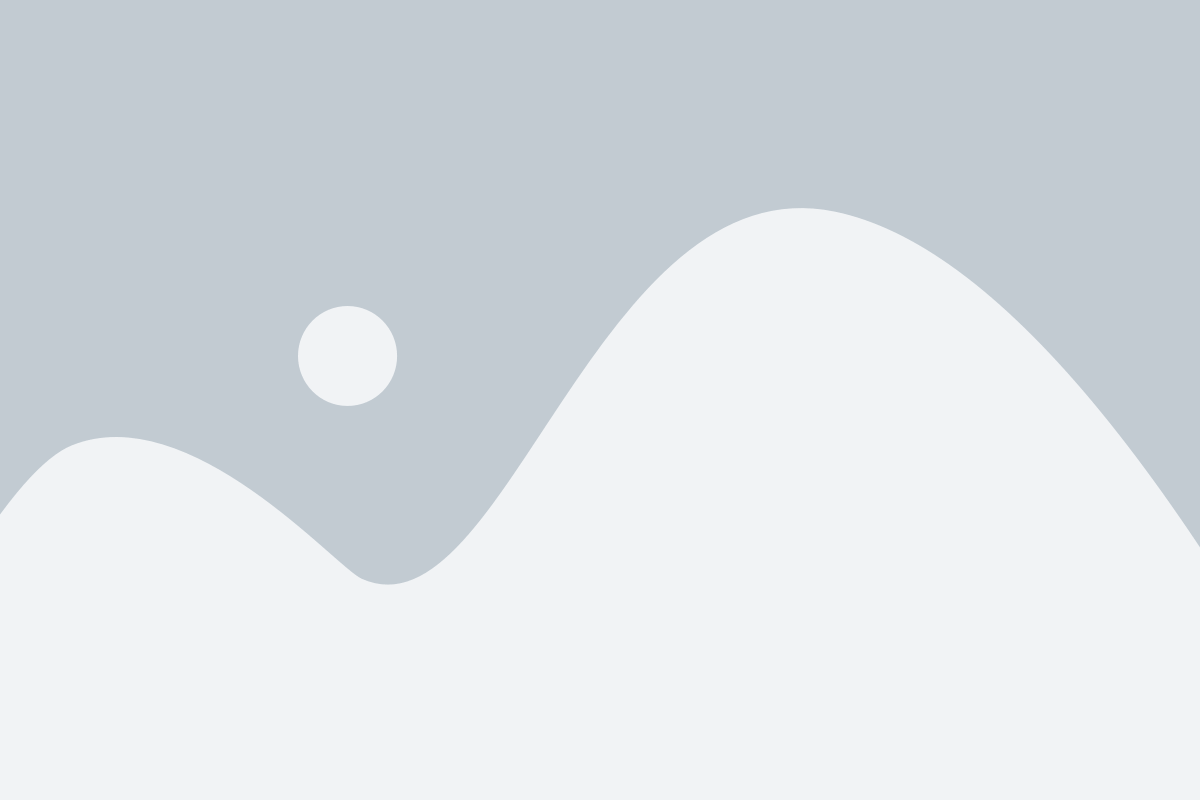
At the turn of the century, it’s likely few, if any, could anticipate the many ways artificial intelligence would later affect our lives.
Take Emotional Robot with Intelligent Network, or ERWIN, for example. He’s designed to mimic human emotions like sadness and happiness in order to help researchers understand how empathy affects human-robot connections. When ERWIN works with Keepon—a robot who looks eerily similar to a real person—scientists gather data on how emotional responses and body language can foster meaningful relationships in an inevitably droid-filled society. Increasingly, robots are integrating into our lives as laborers, therapeutic and medical tools, assistants and more.
While some predict mass unemployment or all-out war between humans and artificial intelligence, others foresee a less bleak future.
The Machine-Man Coexistence
Professor Manuela Veloso, head of the machine learning department at Carnegie Mellon University, is already testing out the idea on the CMU campus, building roving, segway-shaped robots called “cobots” to autonomously escort guests from building to building and ask for human help when they fall short. It’s a new way to think about artificial intelligence, and one that could have profound consequences in the next five years.
There will be a co-existence between humans and artificial intelligence systems that will be hopefully of service to humanity. These AI systems will involve software systems that handle the digital world, and also systems that move around in physical space, like drones, and robots, and autonomous cars, and also systems that process the physical space, like the Internet of Things.
You will have more intelligent systems in the physical world, too — not just on your cell phone or computer, but physically present around us, processing and sensing information about the physical world and helping us with decisions that include knowing a lot about features of the physical world. As time goes by, we’ll also see these AI systems having an impact on broader problems in society: managing traffic in a big city, for instance; making complex predictions about the climate; supporting humans in the big decisions they have to make.
Digital – The Ultimate Catalyst to Accelerate AI
A lot of [AI] research in the early days was actually acquiring [that] knowledge. We would have to ask humans. We would have to go to books and manually enter that information into the computer.
in the last few years, more and more of this information is digital. It seems that the world reveals itself on the internet. So AI systems are now about the data that’s available and the ability to process that data and make sense of it, and we’re still figuring out the best ways to do that. On the other hand, we are very optimistic because we know that the data is there.
The question now becomes, how do we learn from it? How do you use it? How do you represent it? How do you study the distributions — the statistics of the data? How do you put all these pieces together? That’s how you get deep learning and deep reinforcement learning and systems that do automatic translation and robots that play soccer. All these things are possible because we can process all this data so much more effectively and we don’t have to take the enormous step of acquiring that knowledge and representing it. It’s there.
Rules of Coexistence
As of late, discussions have run rampant about the impact of intelligent systems on the nature of work, jobs and the economy. Whether it is self-driving cars, automated warehouses, intelligent advisory systems, or interactive systems supported by deep learning, these technologies are rumored to first take our jobs and eventually run the world.
There are many points of view with regard to this issue, all aimed at defining our role in a world of highly intelligent machines but also aggressively denying the truth of the world to come. Below are the critical arguments of how we’ll coexist with machines in the future:
Machines Take Our Jobs, New Jobs Are Created
Some arguments are driven by the historical observation that every new piece of technology has both destroyed and created jobs. The cotton gin automated the cleaning of cotton. This meant that people no longer had to do the work because a machine enabled the massive growth of cotton production, which shifted the work to cotton picking. For nearly every piece of technology, from the steam engine to the word processor, the argument is that as some jobs were destroyed, others were created.
Machines Only Take Some Of Our Jobs
A variant of the first argument is that even if new jobs are not created, people will shift their focus to those aspects of work that intelligent systems are not equipped to handle. This includes areas requiring the creativity, insight and personal communication that are hallmarks of human abilities, and ones that machines simply do not possess. The driving logic is that there are certain human skills that a machine will never be able to master.
A similar, but more nuanced argument portrays a vision of man-machine partnerships in which the analytical power of a machine augments the more intuitive and emotional skills of the human. Or, depending on how much you value one over the other, human intuition will augment a machine’s cold calculations.
Machines Take Our Jobs, We Design New Machines
Finally, there is the view that as intelligent machines do more and more of the work, we will need more and more people to develop the next generation of those machines. Supported by historical parallels (i.e. cars created the need for mechanics and automobile designers), the argument is that we will always need someone working on the next generation of technology. This is a particularly presumptuous position as it is essentially technologists arguing that while machines will do many things, they will never be able to do what technologists do.
But Could Coexistence Exist Eternally?
These are all reasonable arguments above, and each one has its merits. But they are all based on the same assumption: Machines will never be able to do everything that people can do, because there will always be gaps in a machine’s ability to reason, be creative or intuitive. Machines will never have empathy or emotion, nor have the ability to make decisions or be consciously aware of themselves in a way that could drive introspection.
These assumptions have existed since the earliest days of A.I. They tend to go unquestioned simply because we prefer to live in a world in which machines cannot be our equals, and we maintain control over those aspects of cognition that, to this point at least, make us unique.
But the reality is that from consciousness to intuition to emotion, there is no reason to believe that any one of them will hold. It is quite conclusive that the only alternative to the belief that human thought can be modeled on a machine is to believe that our minds are the product of “magic.” Either we are part of the world of causation or we are not. If we are, A.I. is possible.
Related Posts
AIQRATIONS
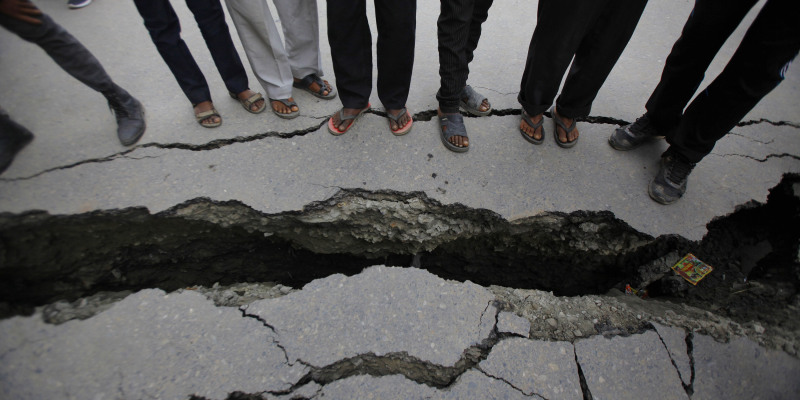
Using Analytics for Detection of Earthquakes and Intensity Forecasting
Add Your Heading Text Here
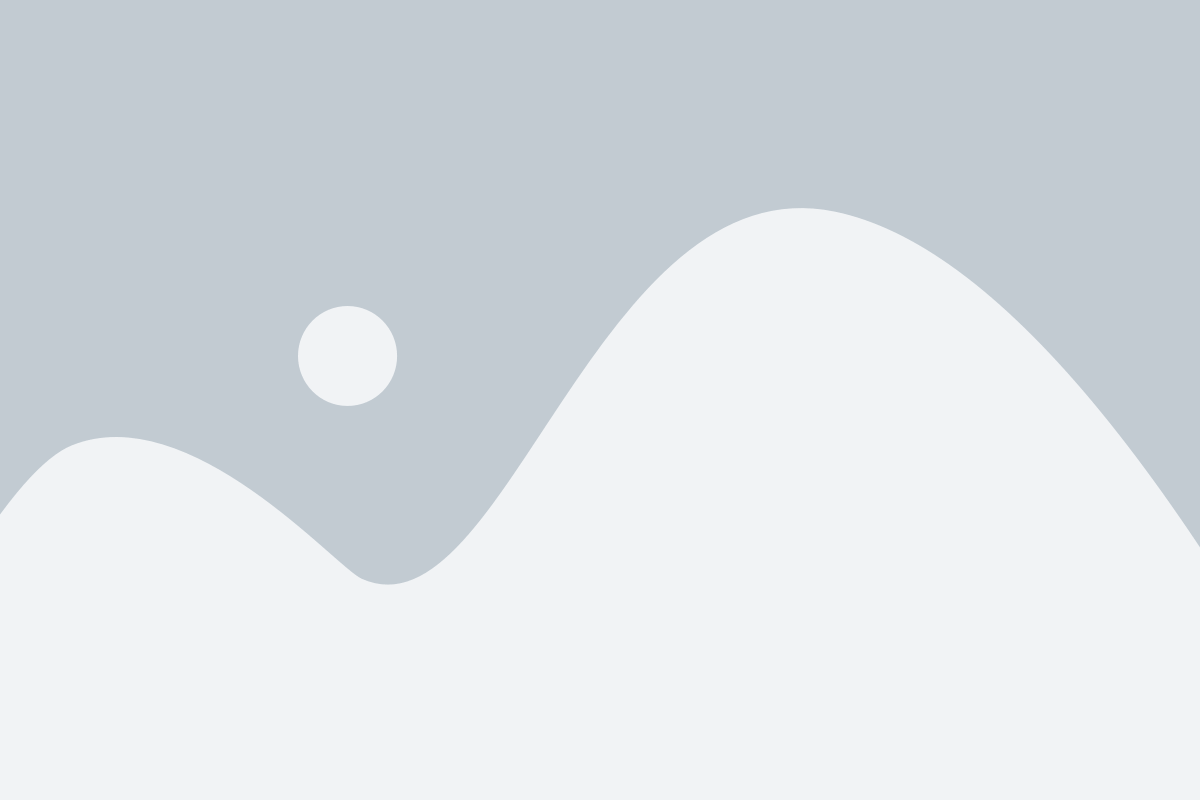
We know the quakes are coming. We just don’t know how to tell enough people early enough to avoid the catastrophe ahead. Around the world more than 13,000 people are killed each year by earthquakes, and almost 5 million have their lives affected by injury or loss of property. Add to that $12 billion a year in economic losses to the global economy (the average annual toll between 1980 and 2008). Understandably for some time scientists have been asking if earthquakes can be predicted more accurately.
Unfortunately, the conventional answer has often been “no”. For many years earthquake prediction relied almost entirely on monitoring the frequency of quakes or natural events in the surroundings and using this to establish when they were likely to reoccur. A case in point is the Haicheng earthquake that occurred in eastern China on February 4, 1975. Just prior to this earthquake, the temperatures were high and the pressure was abnormal. Many snakes and rodents also emerged from the ground as a warning sign. With this information, the State Seismological Bureau (SSB) was able to predict an earthquake that helped to save many lives. However, this prediction was issued on the day when the earthquake occurred, so it did cause heavy loss of property. Had this earthquake been predicted a few days earlier, it could have been possible to completely evacuate the affected cities, and this is exactly where big data fits in.
Nature is always giving cues about the occurrence of events, and it is simply up to us to tune in to these cues so that we can act accordingly. Since these cues are widespread, it is best to use big data to collectively bring in this data to a central location so that analysis and the resulting predictions are more accurate. Some common information that can be tracked by big data is the movement of animals and the atmospheric conditions preceding earthquakes.
Scientists today predict where major earthquakes are likely to occur, based on the movement of the plates in the Earth and the location of fault zones. They calculate quake probabilities by looking at the history of earthquakes in the region and detecting where pressure is building along fault lines. These can go wrong as a strain released along a section of the fault line can transfer strain to another section. This is also what happened in the recent quake, say French scientists, noting that the 1934 quake on the eastern segment had moved a part of the strain to the eastern section where the latest quake was triggered.
Academics often put forward arguments that accurate earthquake prediction is inherently impossible, as conditions for potential seismic disturbance exist along all tectonic fault lines, and a build-up of small-scale seismic activity can effectively trigger larger, more devastating quakes at any point. However all this is changing. Big Data analysis has opened up the game to a new breed of earthquake forecasters using satellite and atmospheric data combined with statistical analysis. And their striking results seem to be proving the naysayers wrong.
One of these innovators is Jersey-based Terra Seismic, which uses satellite data to predict major earthquakes anywhere in the world with 90% accuracy. It uses unparalleled satellite Big Data technology, in many cases they could forecast major (magnitude 6+) quakes from one to 30 days before they occur in all key seismic prone countries. It uses open source software written in Python and running on Apache web servers to process large volumes of satellite data, taken each day from regions where seismic activity is ongoing or seems imminent. Custom algorithms analyze the satellite images and sensor data to extrapolate risk, based on historical facts of which combinations of circumstances have previously led to dangerous quakes.
Of course plenty of other organizations have monitored these signs – but it is big data analytics which is now providing the leap in levels of accuracy. Monitored in isolation these particular metrics might be meaningless – due to the huge number of factors involved in determining where a quake will hit, and how severe it will be. But with the ability to monitor all potential quake areas, and correlate any data point on one quake, with any other – predictions can become far more precise, and far more accurate models of likely quake activity can be constructed, based on statistical likelihood.
So once again we see Big Data being put to use to make the impossible possible – and hopefully cut down on the human misery and waste of life caused by natural disasters across the globe.