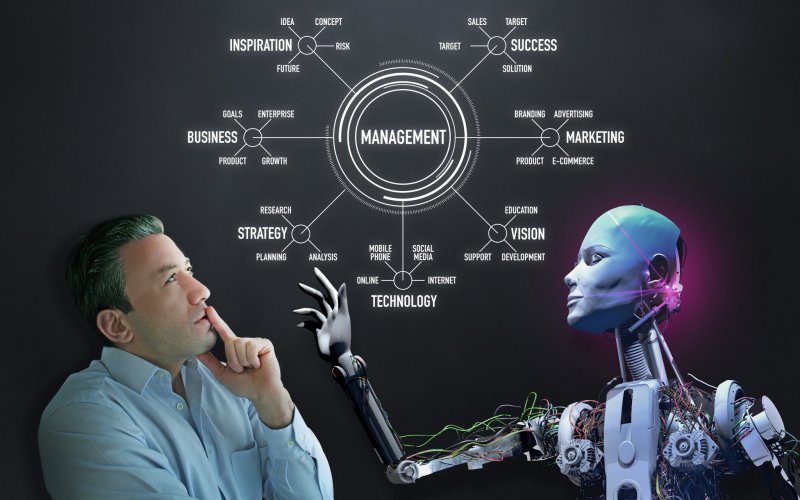
How AI is Challenging Management Theories and Disrupting Conventional Strategic Planning Processes
Add Your Heading Text Here
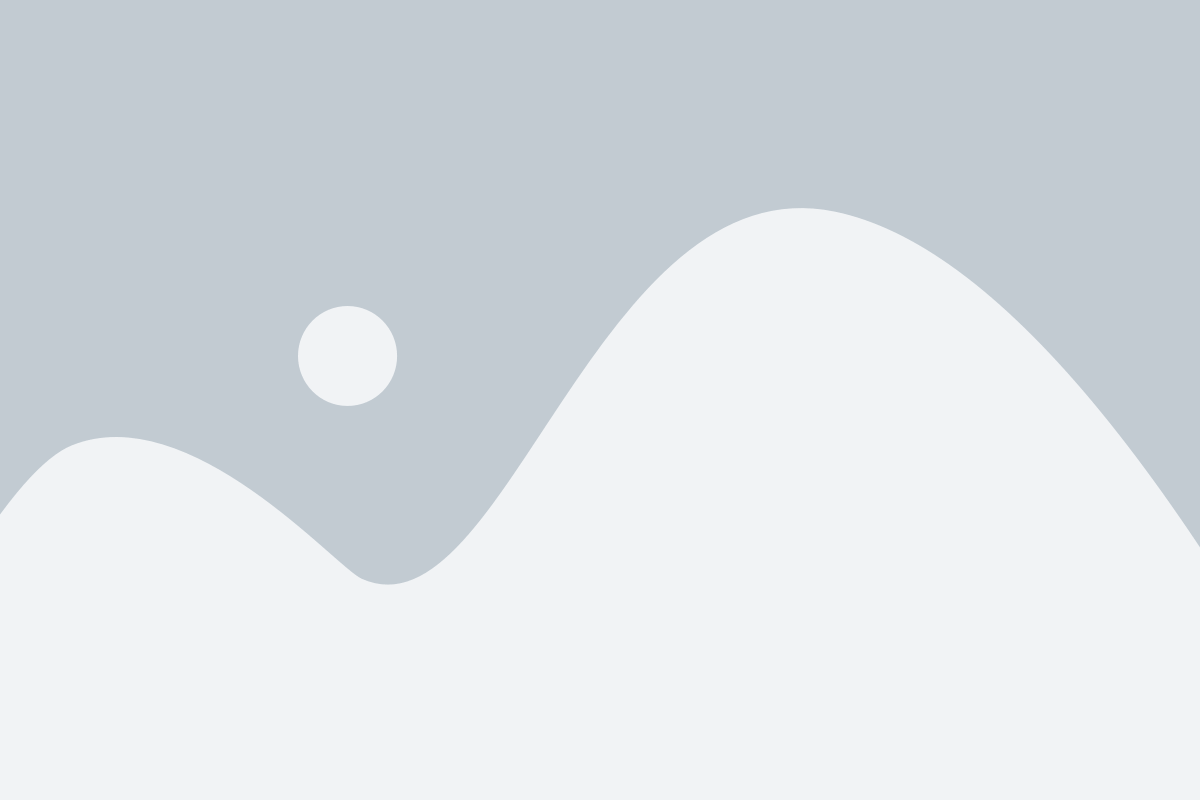
When it comes to AI, businesses think ambitiously. Nearly 85% of executives believe AI will allow their company to obtain or sustain a competitive advantage in the marketplace. Contrastingly, just one in five companies have incorporated AI into their organization and less than 39% of companies have an AI strategy.
Exactly why is AI so disruptive to traditional business models and traditional notions of industry competition? A useful way to analyse the situation is by looking at Porter’s model of the five forces of industry competition and exploring how artificial intelligence is impacting each of the various forces.
According to Michael E. Porter, in one of his landmark books, titled Competitive Strategy, “In any industry, whether it is domestic or international or produces a product or a service, the rules of competition are embodied in five competitive forces: the entry of new competitors, the threat of substitutes, the bargaining power of buyers, the bargaining power of suppliers, and the rivalry among the existing competitors.”
Figure 1: Porter’s Five Forces
Let’s look at each of these five forces and examine the role and impact of AI:
The entry of new competitors
There’s no doubt that AI is changing the nature of competition. Today, it’s not just traditional industry competitors you need to worry about, but new entrants from outside your industry, equipped with new AI based business models and value propositions.
This is often tech giants and startups that have envisioned and built a new business model from the ground up, powered by a new platform ecosystem for AI. They’re leveraging the familiar social, mobile, analytics and cloud technologies, but are often adding in personas and context, intelligent automation, chatbots and the Internet of Things, to further enhance the value proposition of their platform.
Why can new entrants move in so easily? Digital business changes the rules by lowering the traditional barriers to entry. A digitally based business model requires far less capital and can bring large economies of scale for example. Read more about how AI Startups are creating disruptive competition here.
The threat of substitutes
The threat of substitutes is high in many industries since switching costs are low and buyer propensity to substitute is high. For example, In the taxi services, customers can easily switch from traditional models to the new digital app based taxi services, employing AI routines to create differential pricing and intelligent route mapping to increase margin as well as decrease price for the customers. Propensity to switch from the traditional model is high due to consumer wait times for taxis, lack of visibility into taxi location and so on.
In case of BPO industry, the advent of AI has been extremely disruptive, with their clients completely substituting their services with building in-house automation offerings and circumventing their need, sometimes completely. Read more in detail about the disruption of BPO/BPM by AI here.
The bargaining power of buyers
Perhaps the strongest of the five forces impacting industry competition is the bargaining power of buyers since the biggest driver of AI and digital business comes from the needs and expectations of consumers and customers themselves.
This bargaining power lays out a new set of expectations for the AI and digital customer experience and necessitates continual corporate innovation across business models, processes, operations, products and services.
For example, the most used instances of chatbots are through customer support, and now they are heading in the direction of changing the retail sector altogether. The expectations of the Millennials are directing the course of this new technology. This is why chatbots have the burden to exceed the expectations in the retail sector.
Also, in another example, in the customer facing marketing aspect, AI is causing circular rise in customer expectations as rise of expectations, mostly from millennials, has forced the companies to adopt an AI solution to the problem, which further has emboldened their expectations. Amazon, the company that wants to eat everyone’s lunch, is already driving a third of its business from a AI-powered function: its recommended purchases. Read more about how AI is accentuating customer experience to address rising expectations Here.
The bargaining power of suppliers
Suppliers can accelerate or slow down the adoption of a AI based business model based upon how it impacts their own situation. Those pursuing AI models themselves, such as the use of APIs to streamline their ability to form new partnerships and manage existing ones, may help accelerate your own model.
Those who are suppliers to the traditional models, and who question or are still determining their new role in the digital equivalent, may use their bargaining power to slow down or dispute the validity or legality of the new model.
Good examples are the legal and business issues surfacing around the digital-sharing economy (i.e. ride-sharing, room-sharing etc.) where suppliers and other constituents work to ensure the AI based business model and process innovations (like route optimization, or deep customer behaviour analysis using private data) still adhere to established rules, regulations, privacy, security and safety. This is a positive and needed development since, coupled with bargaining power of buyers, it can help to keep new models “honest” in terms of how they operate.
The rivalry among the existing competitors
A lot of organisations are in exploratory stages as they realise that their strategy and customer engagement needs to get smarter. The combination of optimism and fear that clients today have shows that for them it is a competitive necessity to adopt AI and digital technologies.
In 20 years, probably every job will be touched by AI. The technology is growing universally. WhatsApp and Facebook — everything is driven by AI. And what this means is that on the job front, there may be blood. Once AI, ML, and virtual and augmented reality go mainstream, these technologies will prove to be a huge job creator.
But currently, the most competitive space in AI adoption is in the implementation of chatbots across industries and functions. While we might see chatbots starting to appear through the likes of Facebook Messenger and WhatsApp platforms in the coming 12 months, and will be dedicating teams of engineers to train the platforms, rather than relying on the general public. Read more about the competitive atmosphere and underlying need to better customer experience using chatbot here.
How AI will transform Strategic Planning Process
How can managers — from the front lines to the C-suite — thrive in the age of AI? In many ways, the lack of understanding when it comes to AI is due to the variety of ways AI can be implemented as a part of strategic planning for a business. Different industries, or even different companies within the same industry, may use AI in different ways. Ping An, which employs 110 data scientists, has launched about 30 CEO-sponsored AI initiatives that support, in part, its vision – that technology will be the key driver to deliver top-line growth for the company in the years to come. Yet in sharp contrast, elsewhere in the insurance industry, other large companies’ AI initiatives are limited to experimenting with chatbots. Obviously, integrating AI is not going to be simple. There will be a massive learning curve for organizations before they’re able to start implementing AI effectively. But the core shift in strategic planning will happen in the following ways:
AI will take over almost all Administrative Tasks
According to an HBR report, managers across all levels spend more than half of their time on administrative coordination and control tasks. (For instance, a typical store manager or a lead nurse at a nursing home must constantly juggle shift schedules because of staff members’ illnesses, vacations, or sudden departures.) These are the very responsibilities that the same managers expect to see AI affecting the most. And they are correct: AI will automate many of these tasks.
Figure 2: Source – HBR (How Artificial Intelligence Will Redefine Management)
For example, in case of report writing The Associated Press expanded its quarterly earnings reporting from approximately 300 stories to 4,400 with the help of AI-powered software robots. In doing so, technology freed up journalists to conduct more investigative and interpretive reporting.
Strategy Managers will focus more on Judgement-oriented Creative Thinking Work
The human factor, which AI still cannot permeate – the application of experience, expertise and a capacity to improvise, to critical business decisions and practices – need to be focused on by strategy managers. Many decisions require insight beyond what artificial intelligence can squeeze from data alone. Managers use their knowledge of organizational history and culture, as well as empathy and ethical reflection. Managers we surveyed have a sense of a shift in this direction and identify the creative thinking skills and experimentation, data analysis and interpretation, and strategy development as three of the four top new skills that will be required to succeed in the future. And since the potential of machine learning is the ability to help make decisions, the AI technology would be better placed as an assisting hand than administrative mind.
Think of AI not as Machines, but Colleagues
Managers who view AI as a kind of colleague will recognize that there’s no need to “race against a machine.” While human judgment is unlikely to be automated, intelligent machines can add enormously to this type of work, assisting in decision support and data-driven simulations as well as search and discovery activities. In fact, 78% of the surveyed managers believe that they will trust the advice of intelligent systems in making business decisions in the future.
Not only will AI augment managers’ work, but it will also enable managers to interact with intelligent machines in collegial ways, through conversation or other intuitive interfaces.
For example, Kensho Technologies, a provider of next-generation investment analytics, allows investment managers to ask investment-related questions in plain English, such as, “What sectors and industries perform best three months before and after a rate hike?” and get answers within minutes.
Design Thinking needs to be adopted both ways – Managers & AI
While managers’ own creative abilities are vital, perhaps even more important is their ability to harness others’ creativity. Manager-designers bring together diverse ideas into integrated, workable, and appealing solutions. Creative thinking and experimentation is a key skill area that managers need to learn to stay successful as AI increasingly takes over administrative work. ‘Collaborative Creativity’ is the operating word here.
But this doesn’t mean that design thinking necessarily need to become a forte exclusive to managers. Even though AI engines may not have reached radical thinking and improvisation as humans, AI algorithms should be viewed as cognitive tools capable of augmenting human capabilities and integrated into systems designed to go with the grain of human—and organizational—psychology. This calls for Divergence from More Powerful Intelligence To More Creative Intelligence in AI.
To make design thinking meaningful for consumers, companies can benefit from carefully selecting use cases and the information they feed into AI technologies. In determining which available data is likely to generate desired results, enterprises can start by focusing on their individual problems and business cases, create cognitive centres of excellence, adopt common platforms to digest and analyze data, enforce strong data governance practices, and crowdsource ideas from employees and customers alike. Read more about Design Thinking in AI here.
Create New Business Processes manifested from Augmented Working Strategy
Simply put, my recommendation is to adopt AI in order to automate administration and to augment but not replace human judgment. If the current shortage of analytical talent is any indication, organizations can ill afford to wait and see whether their managers are equipped to work alongside AI. This calls for change in business processes, and the way they are implemented itself. To navigate in an uncertain future, managers must explore early, and experiment with AI and apply their insights to the next cycle of experiments.
AI augmentation will drive the adoption of new key performance indicators. AI will bring new criteria for success: collaboration capabilities, information sharing, experimentation, learning and decision-making effectiveness, and the ability to reach beyond the organization for insights.
Accordingly, organizations need to develop training and recruitment strategies for creativity, collaboration, empathy, and judgment skills. Leaders should develop a diverse workforce and team of managers that balance experience with creative and social intelligence — each side complementing the other to support sound collective judgment.
Final Word
While oncoming AI disruptions in Management Principles and Strategic Planning space won’t arrive all at once, the pace of development is faster and the implications more far-reaching than most executives and managers realize. Those managers capable of assessing what the workforce of the future will look like can prepare themselves for the arrival of AI.
Related Posts
AIQRATIONS
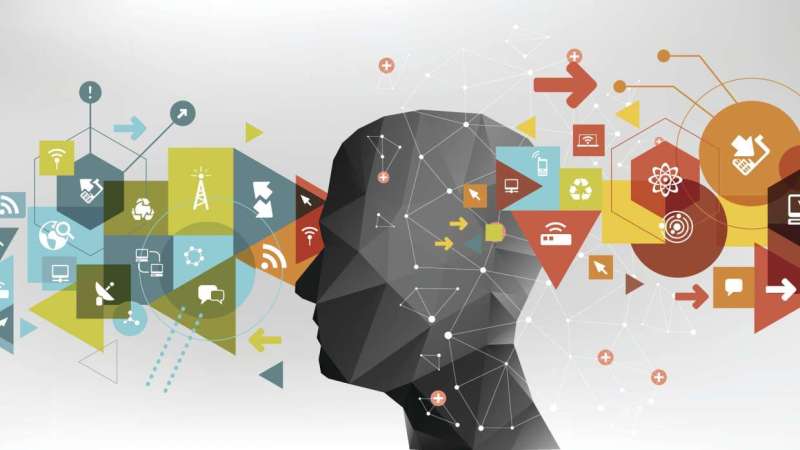
Design Thinking | Behavioural Sciences: Strategic Elements to Building a Successful AI Enterprise
Add Your Heading Text Here
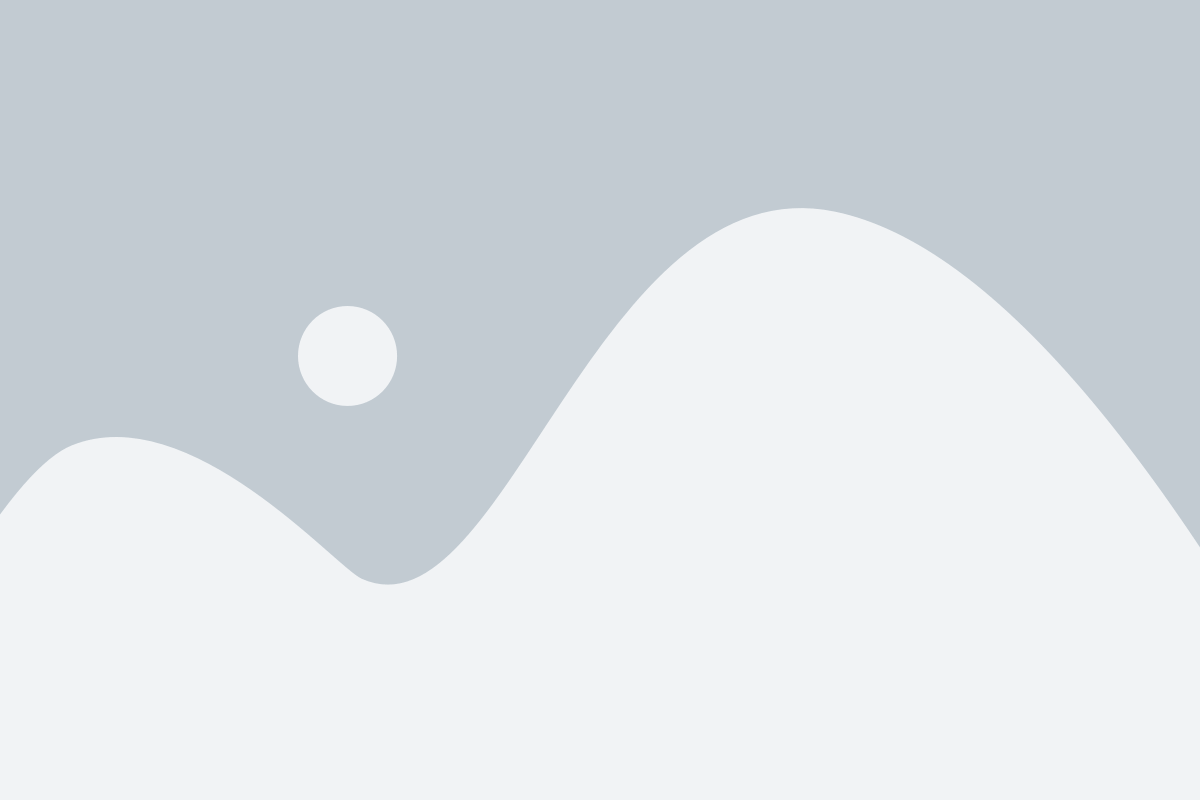
Today’s artificial intelligence (AI) revolution has been made possible by the algorithm revolution. The machine learning algorithms researchers have been developing for decades, when cleverly applied to today’s web-scale data sets, can yield surprisingly good forms of intelligence. For instance, the United States Postal Service has long used neural network models to automatically read handwritten zip code digits. Today’s deep learning neural networks can be trained on millions of electronic photographs to identify faces, and similar algorithms may increasingly be used to navigate automobiles and identify tumors in X-rays. The IBM Watson information retrieval system could triumph on the game show “Jeopardy!” partly because most human knowledge is now stored electronically.
But current AI technologies are a collection of big data-driven point solutions, and algorithms are reliable only to the extent that the data used to train them is complete and appropriate. One-off or unforeseen events that humans can navigate using common sense can lead algorithms to yield nonsensical outputs.
Design thinking is defined as human-centric design that builds upon the deep understanding of our users (e.g., their tendencies, propensities, inclinations, behaviours) to generate ideas, build prototypes, share what you’ve made, embrace the art of failure (i.e., fail fast but learn faster) and eventually put your innovative solution out into the world. And fortunately for us humans (who really excel at human-centric things), there is a tight correlation between the design thinking and artificial intelligence.
Artificial intelligence technologies could reshape economies and societies, but more powerful algorithms do not automatically yield improved business or societal outcomes. Human-centered design thinking can help organizations get the most out of cognitive technologies.
Divergence from More Powerful Intelligence To More Creative Intelligence
While algorithms can automate many routine tasks, the narrow nature of data-driven AI implies that many other tasks will require human involvement. In such cases, algorithms should be viewed as cognitive tools capable of augmenting human capabilities and integrated into systems designed to go with the grain of human—and organizational—psychology. We don’t want to ascribe to AI algorithms more intelligence than is really there. They may be smarter than humans at certain tasks, but more generally we need to make sure algorithms are designed to help us, not do an end run around our common sense.
Design Thinking at Enterprise Premise
Although cognitive design thinking is in its early stages in many enterprises, the implications are evident. Eschewing versus embracing design thinking can mean the difference between failure and success. For example, a legacy company that believes photography hinges on printing photographs could falter compared to an internet startup that realizes many customers would prefer to share images online without making prints, and embraces technology that learns faces and automatically generates albums to enhance their experience.
To make design thinking meaningful for consumers, companies can benefit from carefully selecting use cases and the information they feed into AI technologies. In determining which available data is likely to generate desired results, enterprises can start by focusing on their individual problems and business cases, create cognitive centres of excellence, adopt common platforms to digest and analyze data, enforce strong data governance practices, and crowdsource ideas from employees and customers alike.
In assessing what constitutes proper algorithmic design, organizations may confront ethical quandaries that expose them to potential risk. Unintended algorithmic bias can lead to exclusionary and even discriminatory practices. For example, facial recognition software trained on insufficiently diverse data sets may be largely incapable of recognizing individuals with different skin tones. This could cause problems in predictive policing, and even lead to misidentification of crime suspects. If the training data sets aren’t really that diverse, any face that deviates too much from the established norm will be harder to detect. Accordingly, across many fields, we can start thinking about how we create more inclusive code and employ inclusive coding practices.
CXO Strategy for Cognitive Design Thinking
CIOs can introduce cognitive design thinking to their organizations by first determining how it can address problems that conventional technologies alone cannot solve. The technology works with the right use cases, data, and people, but demonstrating value is not always simple. However, once CIOs have proof points that show the value of cognitive design thinking, they can scale them up over time.
CIOs benefit from working with business stakeholders to identify sources of value. It is also important to involve end users in the design and conception of algorithms used to automate or augment cognitive tasks. Make sure people understand the premise of the model so they can pragmatically balance algorithm results with other information.
Enterprise Behavioral Science – From Insights to Influencing Business Decisions
Every January, how many people do you know say that they want to resolve to save more, spend less, eat better, or exercise more? These admirable goals are often proclaimed with the best of intentions, but are rarely achieved. If people were purely logical, we would all be the healthiest versions of ourselves.
However, the truth is that humans are not 100% rational; we are emotional creatures that are not always predictable. Behavioral economics evolved from this recognition of human irrationality. Behavioral economics is a method of economic analysis that applies psychological insights into human behavior to explain economic decision-making.
Decision making is one of the central activities of business – hundreds of billions of decisions are made everyday. Decision making sits at the heart of innovation, growth, and profitability, and is foundational to competitiveness. Despite this degree of importance, decision making is poorly understood, and badly supported by tools. A study by Bain & Company found that decision effectiveness is 95% correlated with companies’ financial performance.
Enterprise Behavioral Science is not only about understanding potential outcomes, but to completely change outcomes, and more specifically, change the way in which people behave. Behavioral Science tells us that to make a fundamental change in behavior that will affect the long-term outcome of a process, we must insert an inflection point.
As an example, you are a sales rep and two years ago your revenue was $1 million. Last year it was $1.1 million, and this year you expect $1.2 million in sales. The trend is clear, and your growth has been linear and predictable. However, there is a change in company leadership and your management has increased your quota to $2 million for next year. What is going to motivate you to almost double your revenues? The difference between expectations ($2 million) and reality ($1.2 million) is often referred to as the “behavioral gap” . When the behavioral gap is significant, an inflection point is needed to close that gap. The right incentive can initiate an inflection point and influence a change in behavior. Perhaps that incentive is an added bonus, President’s Club eligibility, a promotion, etc.
Cognitive Design Thinking – The New Indispensable Reskilling Avenue
Artificial intelligence, machine learning, big data analytics and mobile and software development will be the top technology areas where the need for re-skilling will be the highest. India will need 700 million skilled workforce by 2022 to meet the demands of a growing economy. Hence, while there is a high probability that machine learning and artificial intelligence will play an important role in whatever job you hold in the future, there is one way to “future-proof” your career…embrace the power of design thinking.
In fact, integrating design thinking and artificial intelligence can give you “super powers” that future-proof whatever career you decide to pursue. To meld these two disciplines together, one must:
- Understand where and how artificial intelligence and behavioural science can impact your business initiatives. While you won’t need to write machine learning algorithms, business leaders do need to learn how to “Think like a data scientist” in order understand how AI can optimize key operational processes, reduce security and regulatory risks, uncover new monetization opportunities.
- Understand how design thinking techniques, concepts and tools can create a more compelling and emphatic user experience with a “delightful” user engagement through superior insights into your customers’ usage objectives, operating environment and impediments to success.
Design thinking is a mindset. IT firms are trying to move up the curve. Higher-end services that companies can charge more is to provide value and for that you need to know that end-customers needs. For example, to provide value services to banking customers is to find out what the bank’s customer needs are in that country the banking client is based. Latent needs come from a design thinking philosophy where you observe customer data, patterns and provide a solution that the customer does not know. Therefore, Companies will hire design thinkers as they can predict what the consumer does not know and hence charge for the product/service from their clients. Idea in design thinking is to provide agile product creation or solutions.
Without Design Thinking & Behavioural Science, AI Will be Only an Incremental Value
Though organizations understand the opportunity that big data presents, many struggles to find a way to unlock its value and use it in tandem with design thinking – making “big data a colossal waste of time & money.” Only by combining quantitative insights gathered using AI, machine/deep learning, and qualitative research through behavioural science, and finally design thinking to uncover hidden patterns and leveraging it to understand what the customer would want, will we be able to paint a complete picture of the problem at hand, and help drive towards a solution that would create value for all stakeholders.
Related Posts
AIQRATIONS
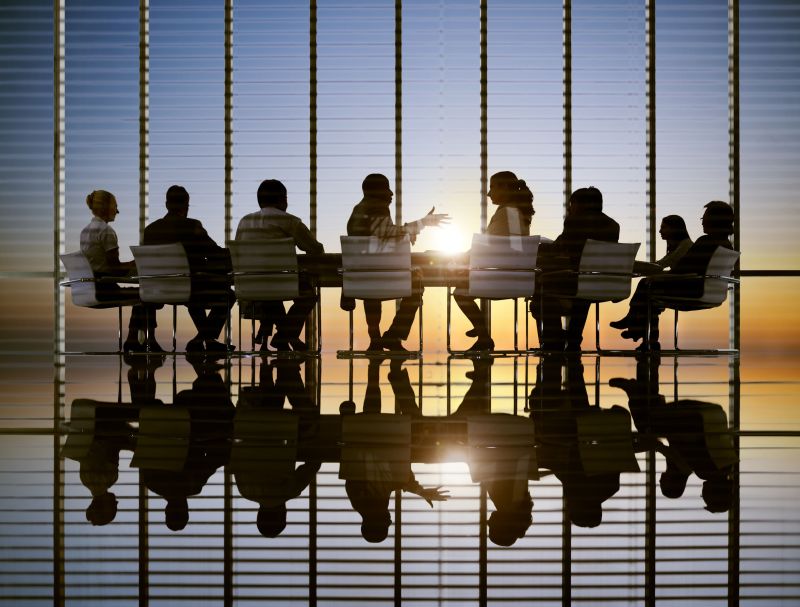
How CXOs are Leveraging AI to Pivot Business Strategy and Operational Models
Add Your Heading Text Here
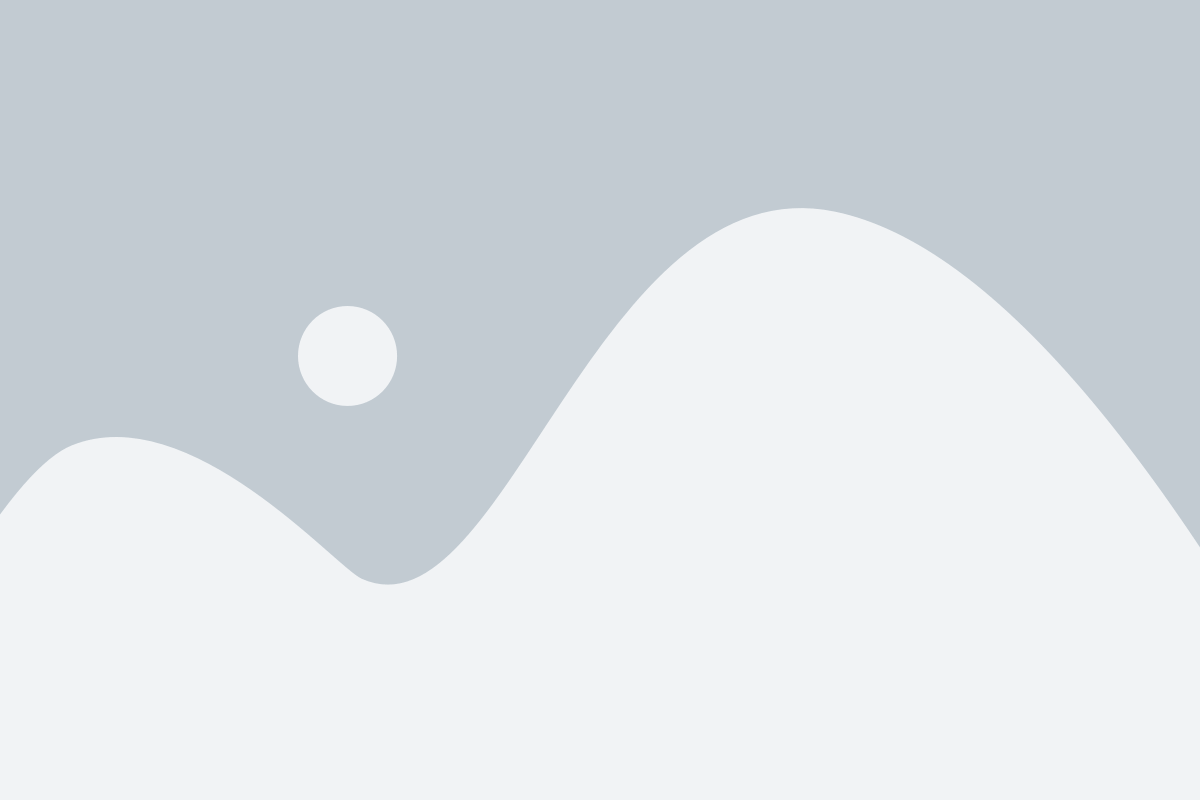
AlphaGo caused a stir by defeating 18-time world champion Lee Sedol in Go, a game thought to be impenetrable by AI for another 10 years. AlphaGo’s success is emblematic of a broader trend: An explosion of data and advances in algorithms have made technology smarter than ever before. Machines can now carry out tasks ranging from recommending movies to diagnosing cancer — independently of, and in many cases better than, humans. In addition to executing well-defined tasks, technology is starting to address broader, more ambiguous problems. It’s not implausible to imagine that one day a “strategist in a box” could autonomously develop and execute a business strategy. We’ve spoken to CXOs and leaders who express such a vision — and companies such as Amazon and Alibaba are already beginning to make it a reality.
Business Processes – Increasing productivity by reducing disruptions
AI algorithms are not natively “intelligent.” They learn inductively by analyzing data. While most leaders are investing in AI talent and have built robust information infrastructures,
As Airbus started to ramp up production of its new A350 aircraft, the company faced a multibillion-euro challenge. The plan was to increase the production rate of that aircraft faster than ever before. To do that, they needed to address issues like responding quickly to disruptions in the factory. Because they will happen. Airbus turned to artificial intelligence. It combined data from past production programs, continuing input from the A350 program, fuzzy matching, and a self-learning algorithm to identify patterns in production problems.
AI led to rectification of about 70% of the production disruptions for Airbus, by matching to solutions used previously — in near real time.
Just as it is enabling speed and efficiency at Airbus, AI capabilities are leading directly to new, better processes and results at other pioneering organizations. Other large companies, such as BP, Infosys, Wells Fargo, and Ping An Insurance, are already solving important business problems with AI. Many others, however, have yet to get started.
Integrated Strategy Machine – The Implementation Scope Augmented AI
The integrated strategy machine is the AI analog of what new factory designs were for electricity. In other words, the increasing intelligence of machines could be wasted unless businesses reshape the way they develop and execute their strategies. No matter how advanced technology is, it needs human partners to enhance competitive advantage. It must be embedded in what we call the integrated strategy machine. An integrated strategy machine is the collection of resources, both technological and human, that act in concert to develop and execute business strategies. It comprises a range of conceptual and analytical operations, including problem definition, signal processing, pattern recognition, abstraction and conceptualization, analysis, and prediction. One of its critical functions is reframing, which is repeatedly redefining the problem to enable deeper insights.
Amazon represents the state-of-the-art in deploying an integrated strategy machine. It has at least 21 data science systems, which include several supply chain optimization systems, an inventory forecasting system, a sales forecasting system, a profit optimization system, a recommendation engine, and many others. These systems are closely intertwined with each other and with human strategists to create an integrated, well-oiled machine. If the sales forecasting system detects that the popularity of an item is increasing, it starts a cascade of changes throughout the system: The inventory forecast is updated, causing the supply chain system to optimize inventory across its warehouses; the recommendation engine pushes the item more, causing sales forecasts to increase; the profit optimization system adjusts pricing, again updating the sales forecast.
Manufacturing Operations – An AI assistant on the floor
CXOs at industrial companies expect the largest effect in operations and manufacturing. BP plc, for example, augments human skills with AI in order to improve operations in the field. They have something called the BP well advisor that takes all of the data that’s coming off of the drilling systems and creates advice for the engineers to adjust their drilling parameters to remain in the optimum zone and alerts them to potential operational upsets and risks down the road. They are also trying to automate root-cause failure analysis to where the system trains itself over time and it has the intelligence to rapidly assess and move from description to prediction to prescription.
Customer-facing activities – near real time scoring
Ping An Insurance Co. of China Ltd., the second-largest insurer in China, with a market capitalization of $120 billion, is improving customer service across its insurance and financial services portfolio with AI. For example, it now offers an online loan in three minutes, thanks in part to a customer scoring tool that uses an internally developed AI-based face-recognition capability that is more accurate than humans. The tool has verified more than 300 million faces in various uses and now complements Ping An’s cognitive AI capabilities including voice and imaging recognition.
AI Strategy for Different Operational Models
To make the most of this technology implementation in various business operations in your enterprise, consider the three main ways that businesses can or will use AI:
Assisted intelligence
Now widely available, improves what people and organizations are already doing. For example, Google’s Gmail sorts incoming email into “Primary,” “Social,” and “Promotion” default tabs. The algorithm, trained with data from millions of other users’ emails, makes people more efficient without changing the way they use email or altering the value it provides. Assisted intelligence tends to involve clearly defined, rules-based, repeatable tasks.
Assisted intelligence apps often involve computer models of complex realities that allow businesses to test decisions with less risk. For example, one auto manufacturer has developed a simulation of consumer behavior, incorporating data about the types of trips people make, the ways those affect supply and demand for motor vehicles, and the variations in those patterns for different city topologies, marketing approaches, and vehicle price ranges. The model spells out more than 200,000 variations for the automaker to consider and simulates the potential success of any tested variation, thus assisting in the design of car launches. As the automaker introduces new cars and the simulator incorporates the data on outcomes from each launch, the model’s predictions will become ever more accurate.
Augmented intelligence
Augmented Intelligence, emerging today, enables organizations and people to do things they couldn’t otherwise do. Unlike assisted intelligence, it fundamentally alters the nature of the task, and business models change accordingly.
For example, Netflix uses machine learning algorithms to do something media has never done before: suggest choices customers would probably not have found themselves, based not just on the customer’s patterns of behavior, but on those of the audience at large. A Netflix user, unlike a cable TV pay-per-view customer, can easily switch from one premium video to another without penalty, after just a few minutes. This gives consumers more control over their time. They use it to choose videos more tailored to the way they feel at any given moment. Every time that happens, the system records that observation and adjusts its recommendation list — and it enables Netflix to tailor its next round of videos to user preferences more accurately. This leads to reduced costs and higher profits per movie, and a more enthusiastic audience, which then enables more investments in personalization (and AI).
Autonomous intelligence
Being developed for the future, Autonomous Intelligence creates and deploys machines that act on their own. Very few autonomous intelligence systems — systems that make decisions without direct human involvement or oversight — are in widespread use today. Early examples include automated trading in the stock market (about 75 percent of Nasdaq trading is conducted autonomously) and facial recognition. In some circumstances, algorithms are better than people at identifying other people. Other early examples include robots that dispose of bombs, gather deep-sea data, maintain space stations, and perform other tasks inherently unsafe for people.
As you contemplate the introduction of artificial intelligence, articulate what mix of the three approaches works best for you.
- Are you primarily interested in upgrading your existing processes, reducing costs, and improving productivity? If so, then start with assisted intelligence, probably with a small group of services from a cloud-based provider.
- Do you seek to build your business around something new — responsive and self-driven products, or services and experiences that incorporate AI? Then pursue an augmented intelligence approach, probably with more complex AI applications resident on the cloud.
- Are you developing a genuinely new technology? Most companies will be better off primarily using someone else’s AI platforms, but if you can justify building your own, you may become one of the leaders in your market.
The transition among these forms of AI is not clean-cut; they sit on a continuum. In developing their own AI strategy, many companies begin somewhere between assisted and augmented, while expecting to move toward autonomous eventually.
Related Posts
AIQRATIONS

The most strategic agenda in CEO’s mind – Is the enterprise AI ready ?
Add Your Heading Text Here
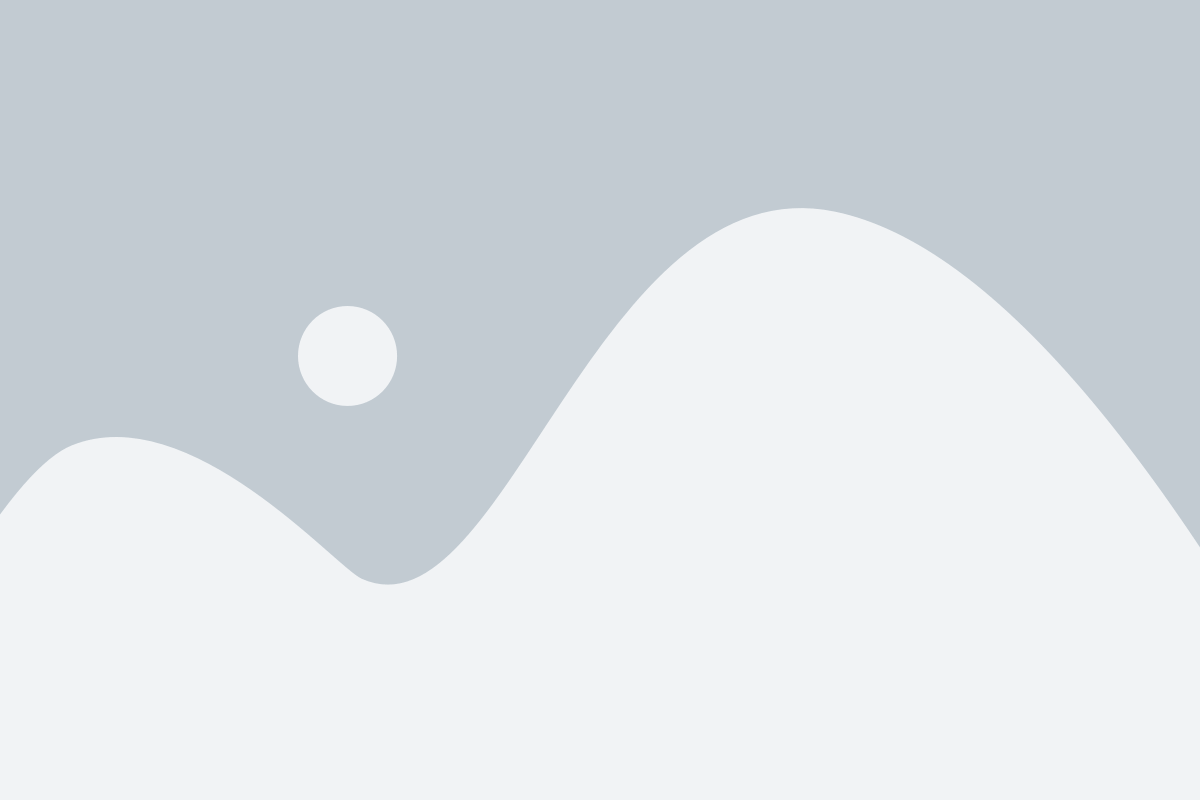
For the larger mass of professionals, the words “artificial intelligence,” or AI, often conjure up images of robots, the sorts of robots that might someday take their jobs. But at the enterprise level, AI means something different. It has enormous power and potential: it can disrupt, innovate, enhance, and in many cases totally transform a business. Forrester Research predicts a 300% increase in AI investment in 2017 from last year, and IDC estimates that the AI market will surge from about $8 billion in 2016 to more than $47 billion in 2020. There’s solid proof that the investment can pay off—if CEO’s can adopt the right strategy. Organizations that deploy AI strategically enjoy advantages ranging from cost reductions and higher productivity to top-line benefits such as increasing revenue and profits, richer customer experiences, and working-capital optimization. The survey shows that the companies winning at AI are also more likely to enjoy broader business success.
So How to make your Enterprise AI Ready?
just one quarter of organizations say they are getting significant impact from it. But these leading businesses have taken clear, practical steps to get the results they want. Here are five of their key strategies:
- Core AI Resource Assimilation using Funding or Acquisition
- Gain senior management support
- Focus on process, not function
- Reskill your teams and foster a learning culture
- Shift from system-of-record to system-of-intelligence apps, platforms
- Encourage innovation
Core AI Resource Assimilation using Funding or Acquisition
As per insights from Forbes and Cowen & Company, 81% of IT leaders are currently investing in or planning to invest in Artificial Intelligence (AI). Based on the study, CIOs have a new mandate to integrate AI into IT technology stacks. The study found that 43% are evaluating and doing a Proof of Concept (POC) and 38% are already live and planning to invest more. The following graphic provides an overview of company readiness for machine learning and AI projects.
Through 2020, organization using cognitive ergonomics and system design in new AI projects will achieve long term success four times more often than others
– Gartner
With $1.7 billion invested in AI startups in Q1 2017 alone, and the exponential efficiencies created by this sort of technology, this evolution will happen quicker than many business leaders are prepared for. If you aren’t sure where to start, don’t worry – you’re not alone. The good news is that you still have options:
- You can acquire, or invest in, an innovative technology company applying AI/ML in your market, and gain access to new product and AI/ML talent.
- You can seek to invest as a limited partner in a few early stage AI focused VC firms, gaining immediate access and exposure to vetted early stage innovation, a community of experts and market trends.
- You can set out to build an AI-focused division to optimize your internal processes using AI, and map out how AI can be integrated into your future products. But recruiting in the space is painful and you will need a strong vision and sense of purpose to attract and retain the best.
- You can use outside development-for-hire shops like new entrant Element.ai, who raised over $100M last June, or more traditional consulting firms, to fill the gaps or get the ball rolling.
Process Based Focus Rather than Function Based
One critical element differentiates AI success from AI failure: strategy. AI cannot be implemented piecemeal. It must be part of the organization’s overall business plan, along with aligned resources, structures, and processes. How a company prepares its corporate culture for this transformation is vital to its long-term success. That includes preparing people by having senior management that understands the benefits of AI; fostering the right skills, talent, and training; managing change; and creating an environment with processes that welcome innovation before, during, and after the transition.
The challenge of AI isn’t just the automation of processes—it’s about the up-front process design and governance you put in to manage the automated enterprise. The ability to trace the reasoning path AI technologies use to make decisions is important. This visibility is crucial in financial services, where auditors and regulators require firms to understand the source of a machine’s decision.
Taking down Resistance to change of Upper Management
One of the biggest challenges to digital transformation is resistance to change. The survey found that upper management is the group most strongly opposed to AI implementation. C-suite executives may not have warmed up to it either. There is such a lack of understanding about the benefits which the technology can bring that the C-suite or board members simply don’t want to invest in it, nor do they understand that failing to do so will adversely affect their bottom line and even cause them to go out of business. Regulatory uncertainty about AI, rough experiences with previous technological innovation, and a defensive posture to better protect shareholders, not stakeholders, may be contributing factors.
Pursuing AI without senior management support is difficult. Here the numbers again speak for themselves. The majority of leading AI companies (68%) strongly agree that their senior management understands the benefits AI offers. By contrast, only 7% of laggard firms agree with this view. Curiously, though, the leading group still cites the lack of senior management vision as one of the top two barriers to the adoption of AI.
Reskilling Teams and HR Redeployment
HR and corporate management will need to figure out new jobs for people to do. Redeployment is going to be a huge factor that the better companies will learn how to handle. The question of job losses is a sensitive one, most often played up in news headlines. But AI also creates numerous job opportunities in new and different areas, often enabling employees to learn higher-level skills. In healthcare for example, physicians are learning to work with AI-powered diagnostic tools to avoid mistakes and make better decisions. The question is who owns the data. If HR retains ownership of people data, it continues to have a role. If it loses that, all bets are off.
HR’s other role in an AI future will be to help make decisions about if and when to automate, whether to reskill or redeploy the human workforce, and the moral and ethical aspects of such decisions. Companies which are experimenting with bots and AI with no thought for the implications need to realize that HR should be central to the governance of AI automation.
Given the potential of AI to complement human intelligence, it is vital for top-level executives to be educated about reskilling possibilities. It is in the best interest of companies to train workers who are being moved from jobs that are automated by AI to jobs in which their work is augmented by AI.
The Dawn of System-of-Intelligence Apps & Platforms
Cowen predicts that an Intelligent App Stack will gain rapid adoption in enterprises as IT departments shift from system-of-record to system-of-intelligence apps, platforms, and priorities. The future of enterprise software is being defined by increasingly intelligent applications today, and this will accelerate in the future.
By 2019, AI platform services will cannibalize revenues for 30% of market leading companies -Gartner
Cowen predicts it will be commonplace for enterprise apps to have machine learning algorithms that can provide predictive insights across a broad base of scenarios encompassing a company’s entire value chain. The potential exists for enterprise apps to change selling and buying behaviour, tailoring specific responses based on real-time data to optimize discounting, pricing, proposal and quoting decisions.
The Process of Supporting Innovation
Besides developing capabilities among employees, an organization’s culture and processes must also support new approaches and technologies. Innovation waves take a lot longer because of the human element. You can’t just put posters on the walls and say, ‘Hey, we have become an AI-enabled company, so let’s change the culture.’ The way it works is to identify and drive visible examples of adoption.
Algorithmic trading, image recognition/tagging, and patient data processing are predicted to the top AI uses cases by 2025. Tractica forecasts predictive maintenance and content distribution on social media will be the fourth and fifth highest revenue producing AI uses cases over the next eight years.
In the End, it’s about Transforming Enterprise
AI is part of a much bigger process of re-engineering enterprises. That is the major difference between the sci-fi robots of yesteryear and today’s AI: the technologies of the latter are completely integrated into the fabric of business, allowing private and public-sector organizations to transform themselves and society in profound ways. You don’t have to turn to sci-fi. The story of human/machine collaboration is already playing at an enterprise near
Related Posts
AIQRATIONS
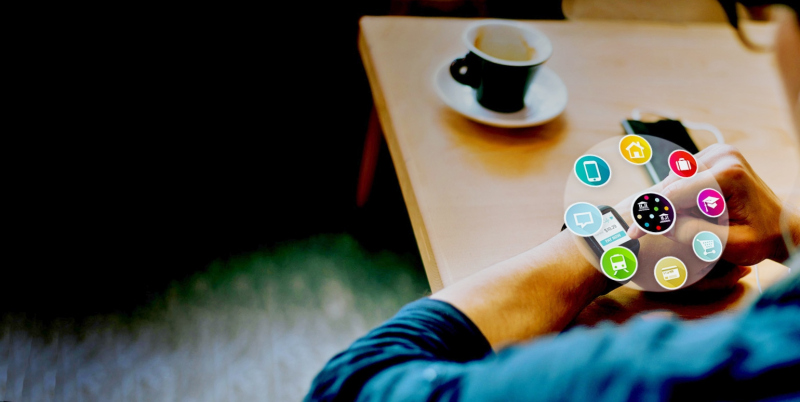
ACCELERATED DECISION MAKING AMPLIFIED BY REAL TIME ANALYTICS – A PERSPECTIVE
Add Your Heading Text Here
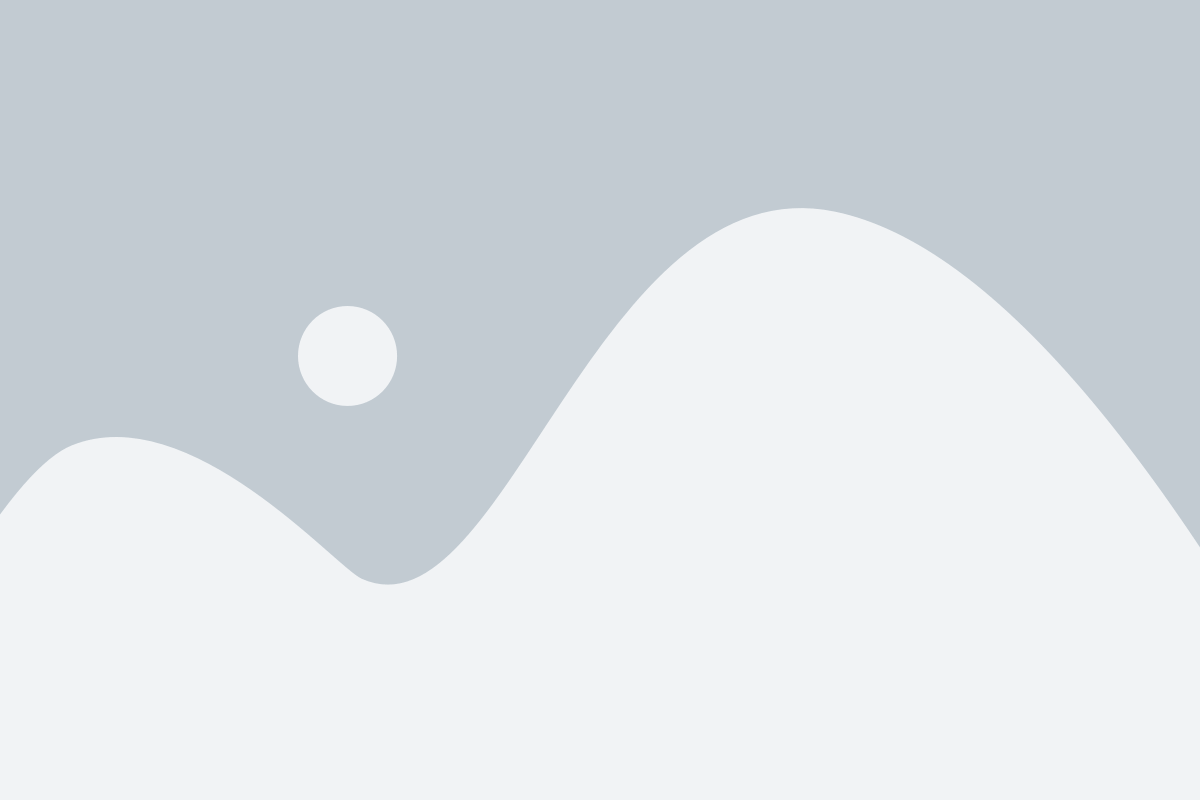
Companies are using more real-time analytics, because of the pressure to increase the speed and accuracy of business processes — particularly for digital business and the Internet of Things (IoT). Although data and analytics leaders intuitively understand the value of fast analytical insights, many are unsure how to achieve them.
Every large company makes thousands of real-time decisions each minute. For example, when a potential customer calls the contact center or visits the company’s website to gather product information, the company has a few seconds to figure out the best-next-action offer to propose to maximize the chance of making a sale. Or, when a customer presents a credit card to buy something or submits a withdrawal transaction request to an automated teller machine, a bank has one second or less to determine if the customer is who they say they are and whether they are likely to pay the bill when it is due. Of course, not all real-time decisions are tied to customers. Companies also make real-time decisions about internal operations, such as dynamically rerouting delivery trucks when a traffic jam forms; calling in a mechanic to replace parts in a machine when it starts to fail; or adjusting their manufacturing schedules when incoming materials fail to arrive on time.
Many decisions will be made in real time, regardless of whether real-time analytics are available, because the world is event-driven and the company has to respond immediately as events unfold. Improved real-time responses that are informed by fact-based, real-time analytics are optional, but clearly desirable.
Real-time analytics can be confusing, because different people may be thinking of different concepts when they use the term “real time.” Moreover, it isn’t always simple to determine where real-time analytics are appropriate, because the “right time” for analytics in a given business situation depends on many considerations; real-time is not always appropriate, or even possible. Finally, data and analytics leaders and their staff typically know less about real-time analytics than about traditional business intelligence and analytics.
Find the certain concept of “Real Time” Relevant to Your Business Problem
Real-time analytics is defined as “the discipline that applies logic and mathematics to data to provide insights for making better decisions quickly.” Real time means different things to different people.
When engineers say “real time” they mean that a system will always complete the task within a specified time frame.
Each component and subtask within the system is carefully designed to provide predictable performance, avoiding anything that could take longer to occur than is usually the case. Real-time systems prevent random delays, such as Java garbage collection, and may run on real-time operating systems that avoid nondeterministic behavior in internal functions such as scheduling and dispatching. There is an implied service-level agreement or guarantee. Strictly speaking, a real-time system could take hours or more to do its work, but in practice, most real-time systems act in seconds, milliseconds or even microseconds.
The concept of engineering real time is most relevant when dealing with machines and fully automated applications that require a precise sequence and timing of interactions among multiple components. Control systems for airplanes, power plants, self-driving cars and other machines often use real-time design. Time-critical software applications, such as high-frequency trading (HFT), also leverage engineering real-time concepts although they may not be entirely real time.
Use Different Technology and Design Patterns for Real-Time Computation Versus Real-Time Solutions
Some people use the term real-time analytics to describe fast computation on historical data from yesterday or last year. It’s obviously better to get the answer to a query, or run a model, in a few seconds or minutes (business real time) rather than waiting for a multihour batch run. Real-time computation on small datasets is executed in-memory by Excel and other conventional tools. Real-time computation on large datasets is enabled by a variety of design patterns and technologies, such as:
- Preloading the data into an in-memory database or in-memory analytics tool with large amounts of memory
- Processing in parallel on multiple cores and chips
- Using faster chips or graphics processing units (GPUs)
- Applying efficient algorithms (for example, minimizing context switches)
- Leveraging innovative data architectures (for example, hashing and other kinds of encoding)
Most of these can be hidden within modern analytics products so that the user does not have to be aware of exactly how they are being used.
Real-time computation on historical data is not sufficient for end-to-end real-time solutions that enable immediate action on emerging situations. Analytics for real-time solutions requires two additional things:
- Data must be real time (current)
- Analytic logic must be predefined
If conditions are changing from minute to minute, a business needs to have situation awareness of what is happening right now. The decision must reflect the latest sensor readings, business transactions, web logs, external market data, social computing postings and other current information from people and things.
Real-time solutions use design patterns that enable them to access the input data quickly so that data movement does not become the weak link in the chain. There is no time to read large amounts of data one row or message at a time across a wide-area network. Analytics are run as close as possible to where the data is generated. For example, IoT applications run most real-time solutions on or near the edge, close to the devices. Also, HFT systems are co-located with the stock exchanges to minimize the distance that data has to travel. In some real-time solutions, including HFT systems, special high-speed networks are used to convey streaming data into the system.
Match the Speed of Analytics to the Speed of the Business Decision
Decisions have a range of natural timing, so “right time” is not always real time. Business analysts and solution architects should work with managers and other decision makers to determine how fast to make each decision. The two primary considerations are:
- How quickly will the value of the decision degrade?
- Decisions should be executed in real time if a customer is waiting on the phone for an answer; resources would be wasted if they sit idle; fraud would succeed; or physical processes would fail if the decision takes more than a few milliseconds or minutes. On the other hand, a decision on corporate strategy may be nearly as valuable in a month as it would be today, because its implementation will take place over months and years so starting a bit earlier may not matter much.
- How much better will a decision be if more time is spent?
- Simple, well-understood decisions on known topics, and for which data is readily available, can be made quickly without sacrificing much quality.
Lastly, Automate Decisions if Algorithms Can Represent the Entire Decision Logic
Algorithms offers the “last mile” of the decision. However, automating algorithms requires a well described process to code against. According to Gartner, “Decision automation is possible only when the algorithms associated with the applicable business policies can be fully defined.”
Final Word
Performing some analytics in real time is a goal in many analytics and business intelligence modernization programs. To operate in real time, data and analytics leaders must leverage predefined analytical models, rather than ad hoc models, and use current input data rather than just historical data.
Related Posts
AIQRATIONS
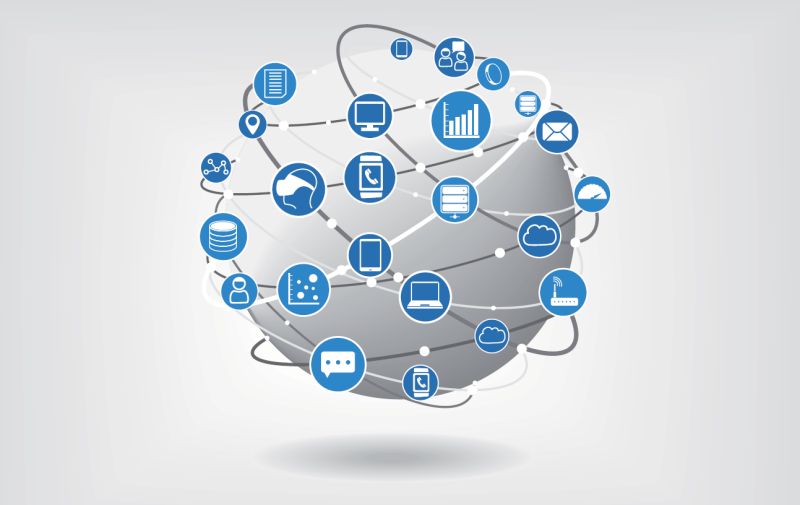
THE BEST PRACTICES FOR INTERNET OF THINGS ANALYTICS
Add Your Heading Text Here
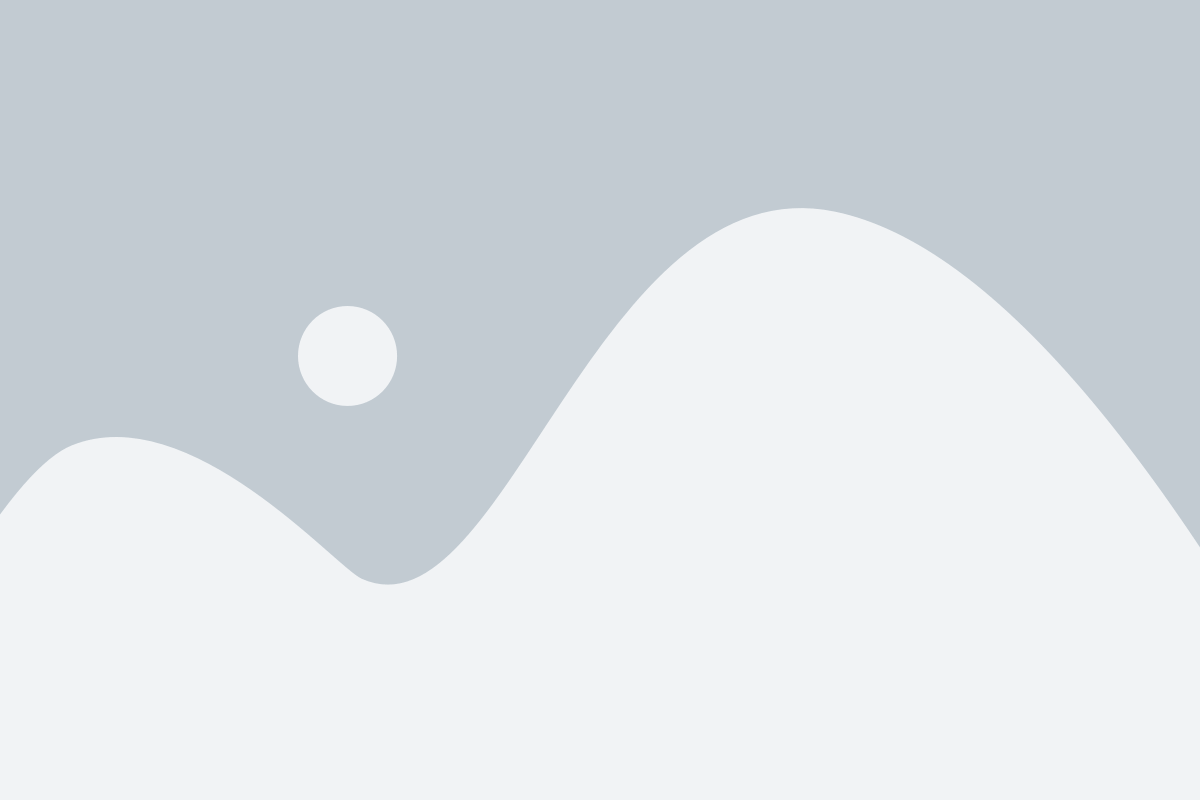
In most ways, Internet of Things analytics are like any other analytics. However, the need to distribute some IoT analytics to edge sites, and to use some technologies not commonly employed elsewhere, requires business intelligence and analytics leaders to adopt new best practices and software.
There are certain prominent challenges that Analytics Vendors are facing in venturing into building a capability. IoT analytics use most of the same algorithms and tools as other kinds of advanced analytics. However, a few techniques occur much more often in IoT analytics, and many analytics professionals have limited or no expertise in these. Analytics leaders are struggling to understand where to start with Internet of Things (IoT) analytics. They are not even sure what technologies are needed.
Also, the advent of IoT also leads to collection of raw data in a massive scale. IoT analytics that run in the cloud or in corporate data centers are the most similar to other analytics practices. Where major differences appear is at the “edge” — in factories, connected vehicles, connected homes and other distributed sites. The staple inputs for IoT analytics are streams of sensor data from machines, medical devices, environmental sensors and other physical entities. Processing this data in an efficient and timely manner sometimes requires event stream processing platforms, time series database management systems and specialized analytical algorithms. It also requires attention to security, communication, data storage, application integration, governance and other considerations beyond analytics. Hence it is imperative to evolve into edge analytics and distribute the data processing load all across.
Hence, some IoT analytics applications have to be distributed to “edge” sites, which makes them harder to deploy, manage and maintain. Many analytics and Data Science practitioners lack expertise in the streaming analytics, time series data management and other technologies used in IoT analytics.
Some visions of the IoT describe a simplistic scenario in which devices and gateways at the edge send all sensor data to the cloud, where the analytic processing is executed, and there are further indirect connections to traditional back-end enterprise applications. However, this describes only some IoT scenarios. In many others, analytical applications in servers, gateways, smart routers and devices process the sensor data near where it is generated — in factories, power plants, oil platforms, airplanes, ships, homes and so on. In these cases, only subsets of conditioned sensor data, or intermediate results (such as complex events) calculated from sensor data, are uploaded to the cloud or corporate data centers for processing by centralized analytics and other applications.
The design and development of IoT analytics — the model building — should generally be done in the cloud or in corporate data centers. However, analytics leaders need to distribute runtime analytics that serve local needs to edge sites. For certain IoT analytical applications, they will need to acquire, and learn how to use, new software tools that provide features not previously required by their analytics programs. These scenarios consequently give us the following best practices to be kept in mind:
Develop Most Analytical Models in the Cloud or at a Centralized Corporate Site
When analytics are applied to operational decision making, as in most IoT applications, they are usually implemented in a two-stage process – In the first stage, data scientists study the business problem and evaluate historical data to build analytical models, prepare data discovery applications or specify report templates. The work is interactive and iterative.
A second stage occurs after models are deployed into operational parts of the business. New data from sensors, business applications or other sources is fed into the models on a recurring basis. If it is a reporting application, a new report is generated, perhaps every night or every week (or every hour, month or quarter). If it is a data discovery application, the new data is made available to decision makers, along with formatted displays and predefined key performance indicators and measures. If it is a predictive or prescriptive analytic application, new data is run through a scoring service or other model to generate information for decision making.
The first stage is almost always implemented centrally, because Model building typically requires data from multiple locations for training and testing purposes. It is easier, and usually less expensive, to consolidate and store all this data centrally. Also, It is less expensive to provision advanced analytics and BI platforms in the cloud or at one or two central corporate sites than to license them for multiple distributed locations.
The second stage — calculating information for operational decision making — may run either at the edge or centrally in the cloud or a corporate data center. Analytics are run centrally if they support strategic, tactical or operational activities that will be carried out at corporate headquarters, at another edge location, or at a business partner’s or customer’s site.
Distribute the Runtime Portion of Locally Focused IoT Analytics to Edge Sites
Some IoT analytics applications need to be distributed, so that processing can take place in devices, control systems, servers or smart routers at the sites where sensor data is generated. This makes sure the edge location stays in operation even when the corporate cloud service is down. Also, wide-area communication is generally too slow for analytics that support time-sensitive industrial control systems.
Thirdly, transmitting all sensor data to a corporate or cloud data center may be impractical or impossible if the volume of data is high or if reliable, high-bandwidth networks are unavailable. It is more practical to filter, condition and do analytic processing partly or entirely at the site where the data is generated.
Train Analytics Staff and Acquire Software Tools to Address Gaps in IoT-Related Analytics Capabilities
Most IoT analytical applications use the same advanced analytics platforms, data discovery tools as other kinds of business application. The principles and algorithms are largely similar. Graphical dashboards, tabular reports, data discovery, regression, neural networks, optimization algorithms and many other techniques found in marketing, finance, customer relationship management and advanced analytics applications also provide most aspects of IoT analytics.
However, a few aspects of analytics occur much more often in the IoT than elsewhere, and many analytics professionals have limited or no expertise in these. For example, some IoT applications use event stream processing platforms to process sensor data in near real time. Event streams are time series data, so they are stored most efficiently in databases (typically column stores) that are designed especially for this purpose, in contrast to the relational databases that dominate traditional analytics. Some IoT analytics are also used to support decision automation scenarios in which an IoT application generates control signals that trigger actuators in physical devices — a concept outside the realm of traditional analytics.
In many cases, companies will need to acquire new software tools to handle these requirements. Business analytics teams need to monitor and manage their edge analytics to ensure they are running properly and determine when analytic models should be tuned or replaced.
Increased Growth, if not Competitive Advantage
The huge volume and velocity of data in IoT will undoubtedly put new levels of strain on networks. The increasing number of real-time IoT apps will create performance and latency issues. It is important to reduce the end-to-end latency among machine-to-machine interactions to single-digit milliseconds. Following the best practices of implementing IoT analytics will ensure judo strategy of increased effeciency output at reduced economy. It may not be suffecient to define a competitive strategy, but as more and more players adopt IoT as a mainstream, the race would be to scale and grow as quickly as possible.