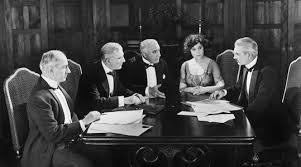
AI led strategy for business transformation : A guided approach for CXOs
Add Your Heading Text Here
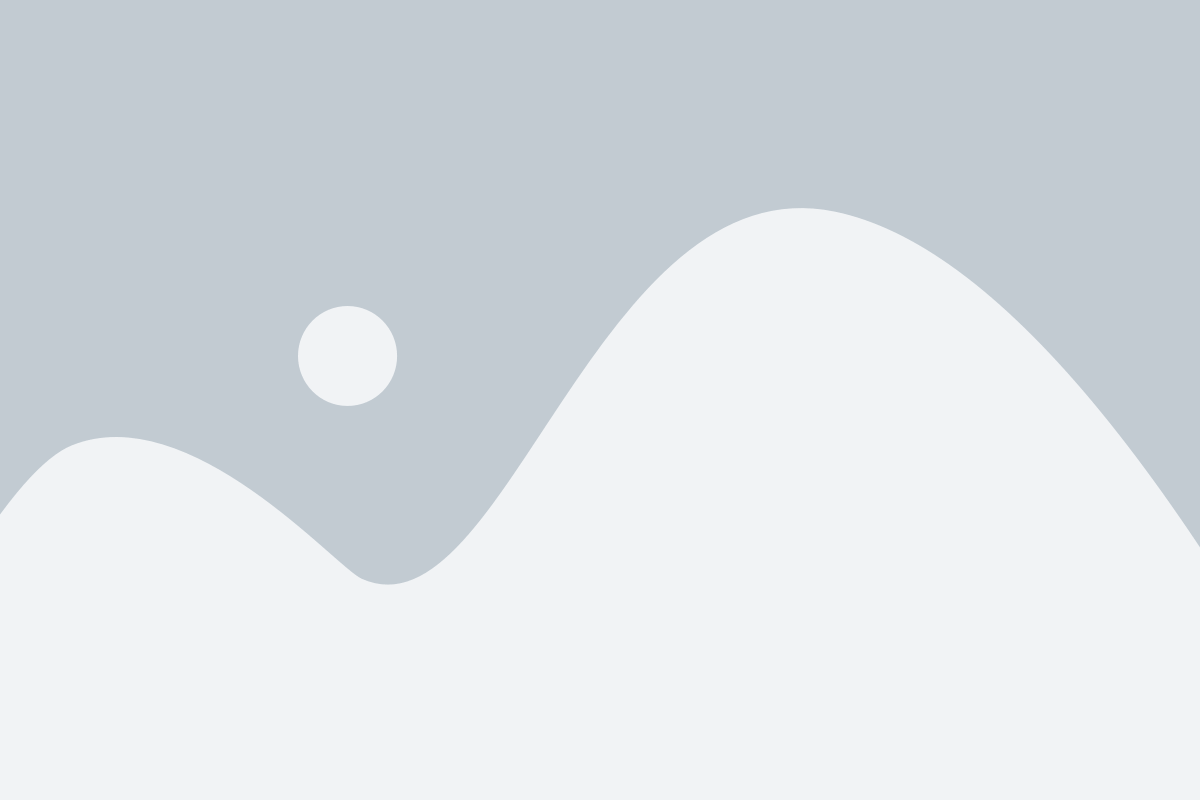
Business transformation programs have long focused on productivity enhancements —taking a “better, faster, cheaper” approach to how the enterprise works. And for good reason: disciplined efforts can boost productivity as well as accountability, transparency, execution, and the pace of decision making. When it comes to delivering fast results to the bottom line, it’s a proven recipe that works.
The problem is, it’s no longer enough. Artificial Intelligence enabled disruption are upending industry after industry, pressuring incumbent companies not only to scratch out stronger financial returns but also to remake who and what they are as enterprises.
Doing the first is hard enough. Tackling the second—changing what your company is and does—requires understanding where the value is shifting in your industry (and in others), spotting opportunities in the inflection points, and taking purposeful actions to seize them. The prospect of doing both jobs at once is sobering.
How realistic is it to think your company can pull it off? The good news is that AIQRATE can demonstrate that it’s entirely possible for organizations to ramp up their bottom-line performance even as they secure game-changing portfolio wins that redefine what a company is and does. What’s more, AL led transformations that focus on the organization’s performance and portfolio appear to load the dice in favor of transformation results. By developing these two complementary sets of muscles, companies can aspire to flex them in a coordinated way, using performance improvements to carry them to the next set of portfolio moves, which in turn creates momentum propelling the company to the next level.
Strategic Steps towards AI led Transformation:
This aspect covers AI led “portfolio-related” moves. The first is active resource reallocation towards building AI led transformation units, which I define as the company shifting more than 20 percent of its capital spending across its businesses or markets over ten years. Such firms create 50 percent more value than counterparts that shift resources at a slower clip.
Meanwhile, a big move in programmatic M&A driven by AI led spot trending—the type of deal making that produces more reliable performance boosts than any other—requires the company to execute at least one deal per year, cumulatively amounting to more than 30 percent of a company’s market capitalization over ten years, and with no single deal being more than 30 percent of its market capitalization.
Making big moves tends to reduce the risk profile and adds more upside than downside. The way I explain this to senior executives is that when you’re parked on the side of a volcano, staying put is your riskiest move.
AI led Transformations that go ‘all in’ by addressing both a company’s performance and its portfolio yield the highest odds.
The implication of these transformation stories is clear: approaches that go all in by addressing both a company’s performance and its portfolio yield the highest odds of lasting improvement. Over the course of a decade, companies that followed this path nearly tripled their likelihood of reaching the top quin tile of the AI transformation power curve relative to the average company in the middle.
Play to win with AI
Life would be simpler if story ended here. However, you’re not operating in a competitive vacuum. As I described earlier, other forces influence your odds of success in significant ways—in particular, how your industry is performing. Research studies have indicated that companies facing competitive headwinds would face longer odds of success than those with tailwinds.
Companies that combined big performance moves with big portfolio moves (including capital expenditures, when not the only portfolio move employed) saw a big lift in their odds. Life is still challenging for these companies—their net odds are dead even—yet this is superior to the negative odds of the other situations.
Winning thru competitive advantage with AI
In an improving industry, the returns to performance improvement are amplified massively. This runs contrary to the very human tendency of equating performance transformations with turnaround cases
The takeaway from all this is that two big rules stand out as commonly and powerfully true whatever your context: first, get moving with AI , don’t be static; second, go all in if you can with AI led transformation programs —it’s always the best outcome (and also the rarest).
Running the AI led transformation program
In my experience, the companies that are most successful at transforming themselves with AI ,sequence their moves so that the rapid lift of performance improvement provides oxygen and confidence for big moves in M&A, capital investment, and resource reallocation. And when the right portfolio moves aren’t immediately available or aren’t clear, the improved performance helps buy a company time until the strategy can catch up.
To illustrate this point, consider the anecdote about Apple that Professor Richard Rumelt describes in his book, Good Strategy/Bad Strategy. It was the late 1990s; Steve Jobs had returned to Apple and cleaned house through productivity-improving cutbacks and a radically simplified product line. Apple was much stronger, yet it remained a niche player in its industry. When Rumelt asked Jobs how he planned to address this fact, Jobs just smiled and said, ‘I am going to wait for the next big thing.’
While no one can guarantee that your “next big thing” will be an iPod-size breakthrough, there’s nothing stopping you from laying the groundwork for a successful AI led transformation. To see how prepared, you are for such an undertaking, ask yourself—and your team—the following five questions. I sincerely hope they provoke productive and transformative discussion among your team.
1.Where is the new business value chain that’s driven by AI
Achieving success with big, portfolio-related moves requires understanding where the business value flows in your business and why. The structural attractiveness of markets, and your position in them, can and does change over time. Ignore this and you might be shifting deck chairs on the Titanic. Meanwhile, to put this thinking into action, you must also view the company as an ever-changing portfolio. This represents a sea change for managers who are used to plodding, once-a-year strategy sessions that are more focused on “getting to yes” and on protecting turf than on debating real alternatives. Get high-powered decision-making algorithms to navigate you thru this transformation.
2. Put your money in building an AI led strategy
Only 10% of the US fortune 200 companies have AI led strategy; this is an impending strategic aspect that cannot be ignored. The dimensions of reimagining customer experience, building innovative products and services and transforming the businesses need to have an AI led strategy move by the CXOs
3.Are you ready for disruption?
Increasingly, incumbent organizations are getting to the pointy end of disruption, where they must accelerate the transition from legacy business models to new ones and even allow potentially cannibalizing businesses to flourish. Sometimes this requires a very deliberate two-speed approach where legacy assets are managed for cash while new businesses are nurtured for growth.
4.Will our company take this seriously?
Embracing AI led transformative change requires commitment, and gaining commitment requires a compelling change story that everyone in the company can embrace. Philips recognized this in 2011 when it launched its “Accelerate” program. Along with productivity improvements and portfolio changes (including a big pivot from electronics to health tech), the company shaped its change story around improving three billion lives annually by 2030, as part of a broader goal of making the world healthier and more sustainable through innovation. Massive thrust and investment was laid by Phillips leadership team on AI led transformation programs.
5.Is the leadership ready for the transformation?
Leading a successful AI led transformation requires a lot more than just picking the right moves and seeing them through. Among your other priorities: build momentum, engage your workforce, and make the change personal for yourself and your company. All of this means developing new leadership skills and ways of working, while embracing a level of commitment as a leader that may be unprecedented for you.
In the end, AI led strategy for transformation is a process and start of a journey …. embrace it or feel the heat of leaving behind. The new age competition is agile and nimble and AI led transformation strategy is a right move to thwart the competition.
Related Posts
AIQRATIONS

AI For CXOs — Redefining The Future Of Leadership In The AI Era
Add Your Heading Text Here
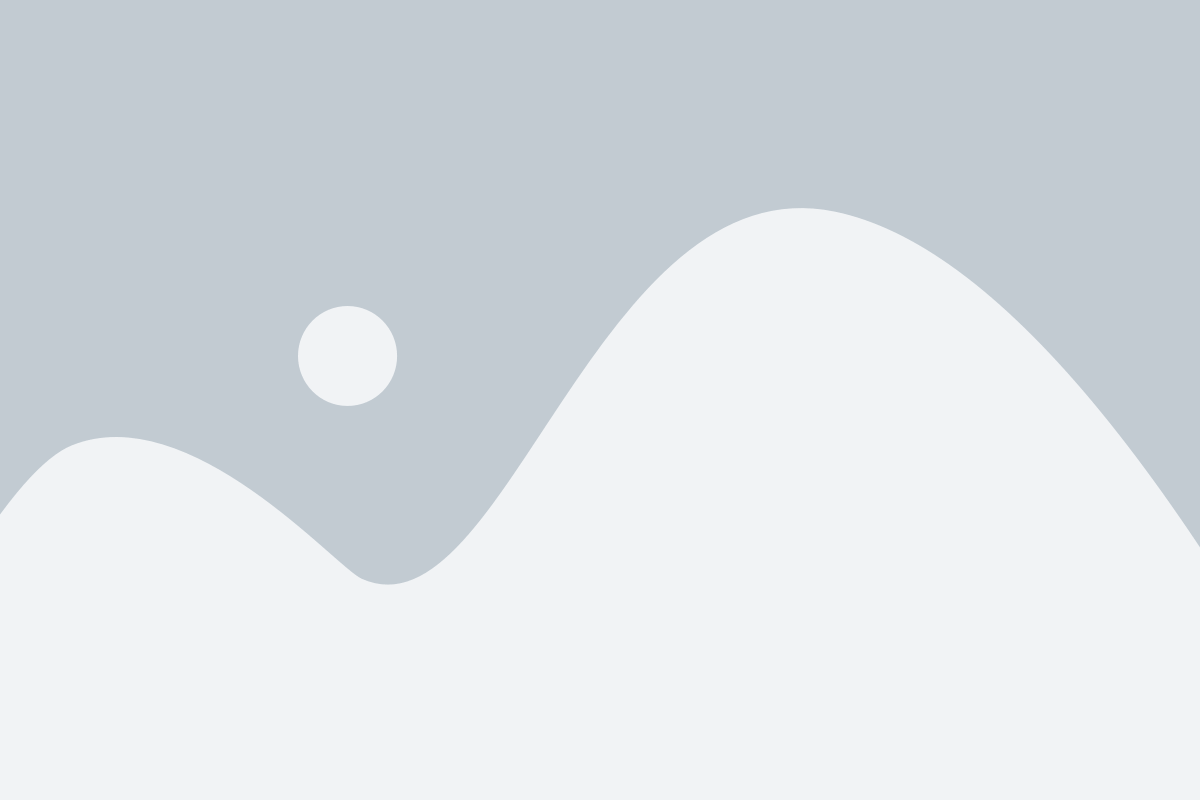
Artificial intelligence is getting ubiquitous and is transforming organizations globally. AI is no longer just a technology. It is now one of the most important lenses that business leaders need to look through to identify new business models, new sources of revenue and bring in critical efficiencies in how they do businesses.
Artificial intelligence has quickly moved beyond bits and pieces of topical experiments in the innovation lab. AI needs to be weaved into the fabric of business. Indeed, if you see the companies leading with AI today, one of the common denominators is that there is a strong executive focus around artificial intelligence. AI transformation can be successful when there is a strong mandate coming from the top and leaders make it a strategic priority for their enterprise.
Given AI’s importance to the enterprise, it is fair to say that AI will not only shape the future of the enterprise, but also the future for those that lead the enterprise mandate on artificial intelligence.
Curiosity and Adaptability
To lead with AI in the enterprise, top executives will need to demonstrate high levels of adaptability and agility. Leaders need to develop a mindset to harness the strategic shifts that AI will bring in an increasingly dynamic landscape of business – which will require extreme agility. Leaders that succeed in this AI era will need to be able to build capable, agile teams that can rapidly take cognizance of how AI can be a game changer in their area of business and react accordingly. Agile teams across the enterprise will be a cornerstone of better leadership in this age of AI.
Leading with AI will also require leaders to be increasingly curious. The paradigm of conducting business in this new world is evolving faster than ever. Leaders will need to ensure that they are on top of the recent developments in the dual realms of business and technology. This requires CXOs to be positively curious and constantly on the lookout for game changing solutions that can have a discernible impact on their topline and bottom-line.
Clarity of Vision
Leadership in the AI era will be strongly characterized by the strength and clarity with which leaders communicate their vision. Leaders with an inherently strong sense of purpose and an eye for details will be forged as organizations globally witness AI transformation.
It is not only important for those that lead with AI to have a clear vision. It is equally important to maintain a razor sharp focus on the execution aspect. When it comes to scaling artificial intelligence in the organization, the devil is very often in the details – the data and algorithms that disrupt existing business processes. For leaders to be successful, they must remain attentive to the trifecta of factors – completeness of their vision for AI transformation, communication of said vision to relevant stakeholders and monitoring the entire execution process. While doing so, it is important to remain agile and flexible as mentioned in my earlier section – in order to be aware of possible business landscape shifts on the horizon.
Engage with High EQ
AI transformation can often seem to be all about hard numbers and complex algorithms. However, leaders need to also infuse the human element to succeed in their efforts to deliver AI @ Scale. The third key for top executives to lead in the age of AI is to ensure that they marry high IQs with equally or perhaps higher levels of EQ.
Why is this so very important? Given the state of this technology today, it is important that we build systems that are completely free of bias and are fair in how they arrive at strategic and tactical decisions. AI learns from the data that it is provided and hence it is important to ensure that the data it is fed is free from bias – which requires a human aspect. Secondly, AI causes severe consternation among the working population – with fears of job loss abounding. It is important to ensure that these irrational fears of an ‘AI Takeover’ are effectively abated. For AI to be successful, it is important that both types of intelligence – artificial and human – symbiotically coexist to deliver transformational results.
AI is undoubtedly going to become one of the sources of lasting competitive advantage for enterprises. According to research, 4 out of 5 C-level executives believe that their future business strategy will be informed through opportunities made available by AI technology. This requires a leadership mindset that is AI-first and can spot opportunities for artificial intelligence solutions to exploit. By democratizing AI solutions across the organization, enterprises can ensure that their future leadership continues to prioritize the deployment of this technology in use cases where they can deliver maximum impact.
Related Posts
AIQRATIONS
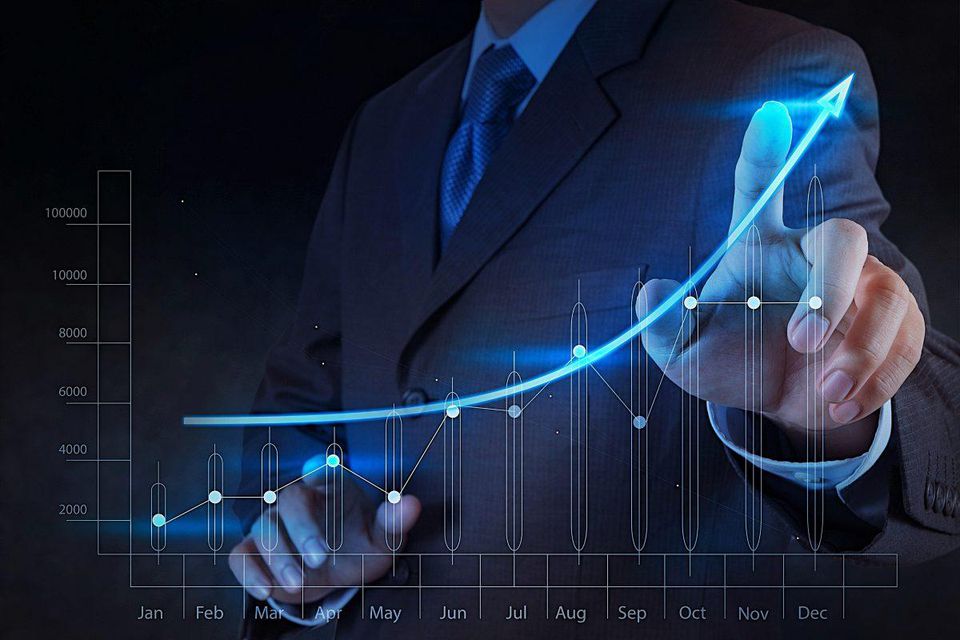
Delivering Business Value Through AI To Impact Top Line, Bottom Line And Unlock ROI
Add Your Heading Text Here
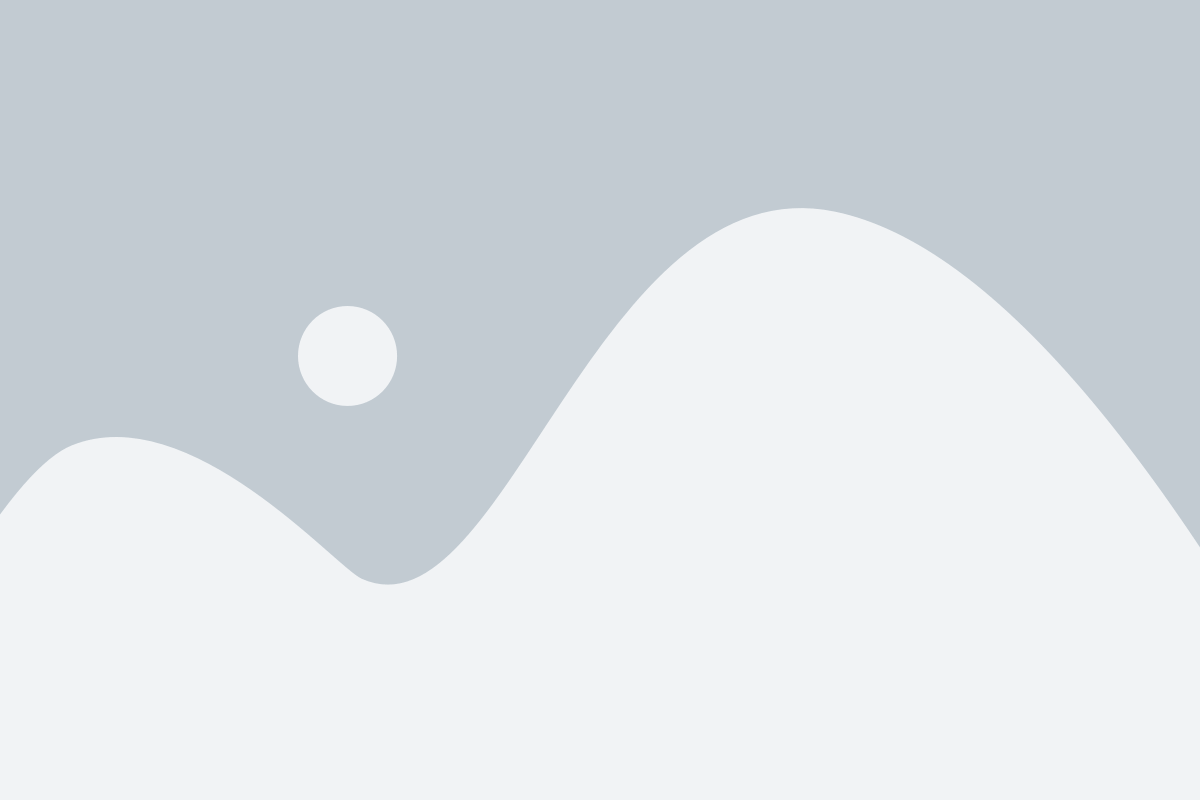
As is the case with investments in any other area of technology, AI needs to deliver demonstrable impact to business top line and bottom line. In today’s competitive landscape of business, enterprises are expected to measure the incremental ROI for every expense and every investment made – technology or otherwise. The case of Artificial Intelligence is no different. It is critical that technology and business leaders demand ROI impact for this technology in order to foster its growth and justify its proliferation in business.
To be sure, there are two key areas where Artificial Intelligence can contribute immense value; Increasing top line figures by unlocking new revenue streams and improving the bottom line through efficiencies in operations. Needless to say, top line gains eventually percolate their way into showcasing bottom line improvement – but for the purpose of this post, we’ll refer to bottom line impact as areas where AI brings in cost efficiencies by helping organizations reduce their overall cost of operations.
Artificial Intelligence driven applications can have a discernible impact on business top lines and bottom lines and help organizations unlock ROI from their implementation.
AI-Powered Topline Growth
Artificial Intelligence-led applications have huge potential to add to top line revenue growth for any organization. Typical AI interventions for this purpose range from improving the effectiveness of marketing and sales functions, improving customer loyalty through laser-guided customer experience initiatives and direct and indirect data monetization.
New Revenue Streams Enabled by Data Monetization:
Business leaders need to realize AI’s potential to unlock new sources of revenue in addition to improving customer targeting and loyalty. One of these ways is data monetization. What is data monetization? Simply put, data monetization refers to the act of generating measurable economic benefits from available data resources. According to Gartner, there are two distinct ways in which business leaders can monetize data. The most commonly seen method from the two is Direct Monetization. The way to realize value from this avenue involves directly adding AI as a feature to existing offerings. Companies like Nielsen, D&B, TransUnion, Equifax, Acxiom, Bloomberg and IMS run their business on licensing their data in a raw format or as part of their application infrastructure. With emerging Data-as-a-Service models and the application for direct insight delivery through intelligent application of AI, direct data monetization is simpler than ever. By wrapping insights alongside the data source, vendors can create a symbiotically powerful exchange of information for both the buyers and sellers of data. On the other hand, Indirect Monetization involves embedding AI into traditional business processes with a focus on driving increased revenue. A popular example of this is corporations who come out with branded, paid-for reports based on the data they own. For instance, professional services companies such as Aon, Deloitte, McKinsey, etc., regularly bring forward insightful industry and function-specific reports based on the data they collect as part of their consulting assignments.
Enabling Intelligent Marketing and Sales
Many of the most prominently cited successes of AI-enabled business transformation comes from the marketing and sales arena. Sales and marketing are constantly on the forefront for exciting inventions in AI since they contribute directly to top line growth. Use cases discovered in this arena span social media sentiment mining, programmatic selection of advertising properties, measuring effectiveness of marketing programs, ensuring customer loyalty and intelligent sales recommendations. AI also has huge potential to drive businesses to explore and exploit eCommerce platforms as a credible channel for sales and to help drive the digital agenda forward. Available tools are helping drive better customer conversions on eCommerce properties – by analysing the digital footprints (clickstream, etc.) of prospective customers, persuading them into making a purchase. In such use cases, AI helps improve personalization at the point-of-purchase, improve conversions and reduce cart abandonment. Marketing and sales use cases today are pretty much at the epicentre of an AI disruption and business leaders need to uncover more use cases that can help drive effective top line growth.
AI Redefining Customer Experience
Customers are the epicentre of every successful organization. Today, we live in times where customers have numerous competitor options to choose from while the switching costs for customers are increasingly lower. Given this scenario, for businesses to win with their customers they need to have a smarter approach to customer experience management.
We have progressed well beyond pre-programmed bots addressing frequently asked questions. AI-enabled systems today go further and provide customers with personalized guidance. The travel and hospitality industries, for instance, are ripe for such disruptive innovations. In many cases, we see chatbots that help customers identify and recommend interesting activities and events that tourists can avail. When applied with human creativity, AI can ensure this redefined understanding of customer experience, while maintaining a lower cost of delivering that experience.
AI for Improving Bottom Line Performance
At an operational level as well, AI can help organizations run a more efficient business. For instance, corporations across industries need to find innovative and fail-safe ways to reduce the cost of manufacturing as well as capping their outlay on the supply chain network. AI-centric solutions can drive down the turnaround time for talent acquisition and transform other facets of the Human Capital function too.
AI Driving Operational Efficiencies
Traditional manufacturing processes are now increasingly augmented by robotics and AI. These technologies are bringing increasing sophistication to the manufacturing process. The successes combine human and machine intelligence making AI-augmented manufacturing a pervasive phenomenon. Today, business leaders in the Industry 4.0 generation need to seriously consider planning a hybrid labour force powered by human and artificial intelligence – and ensure that the two coexist by implementing the right policies and plans in place.
Smarter Supply Chains Powered by AI
Orchestrating a leaner, more predictable supply chain is ripe for an AI-led disruption. We are witnessing not just new products and categories but also new formats of retailers proliferating the industry. This varied portfolio of offerings and channels requires corporations to manage their outlay efficiently on the overall network responsible for the network that manages the entire process from procurement and assembly to stocking and last mile delivery. Multiple use cases exist that leverage multi-source data from internal and external repositories, combining them with information from IOT sensors. AI algorithms are then applied over this combined data infrastructure with the objective of helping business users quickly identify possible weaknesses/flaws in the process such as delays and possible shortages. Business leaders are constantly on the lookout for solutions that can directly lift their bottom line by bringing in more intelligence and automation to their supply chain networks – thus unlocking savings for their businesses.
An Artificial Facelift for the Human Resources Function
The human resources function has historically been considered a cost-center in organizations. In addition to bringing down the costs associated with talent acquisition and management – AI would also help HR teams become leaner, more organized and reduce the turnaround time for talent acquisition. AI interventions are being seen in the areas of employee engagement and attrition management, but some of the most exciting use cases come from the talent acquisition area within the HR function. Multiple organizations are already working on solutions that can eliminate the need for HR staff to scan through each job application individually. By using AI intelligently, talent acquisition teams can determine the framework conditions for a job on offer and leave the creation of assessment tasks to Artificial Intelligence-powered systems. The AI-empowered system can then communicate the evaluation results and recommend the most suitable candidates for further interview rounds.
One of the key reasons why AI is in vogue today is the demonstrable ROI impact that it promises to bring to business processes. With greater computational power and more data, AI has become more practicable than before, but what will sustain its growth is how much incremental value it can eventually unlock for businesses across the globe and power new revenue models for businesses to tap into. It is critical that business and technology leaders earnestly kick off discussions around how to justify the impact of AI and mark down the key metrics that will be used to measure it. Partners and service providers too need to stay on top of finding ways to showcase measurable improvements that their software or services can bring to technology buyers. This will enable the entire AI ecosystem to flourish.
Related Posts
AIQRATIONS
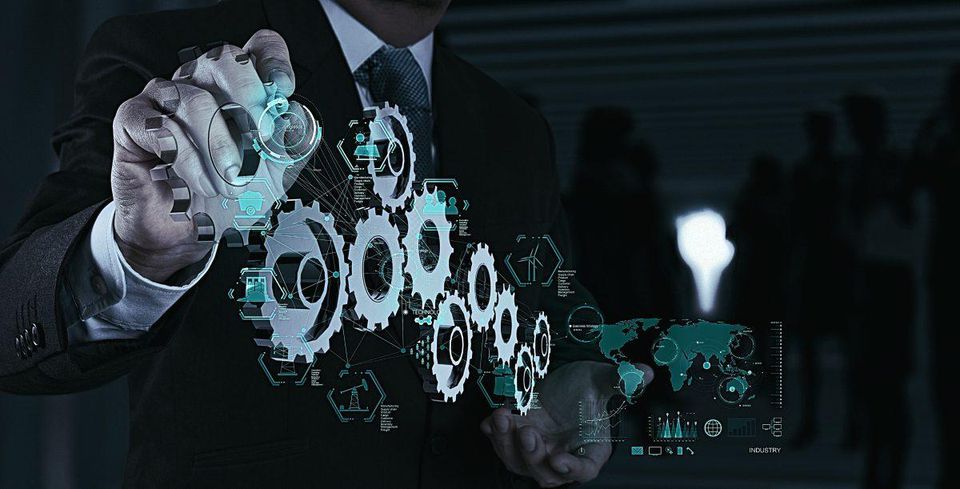
Building AI-enabled organisations
Add Your Heading Text Here
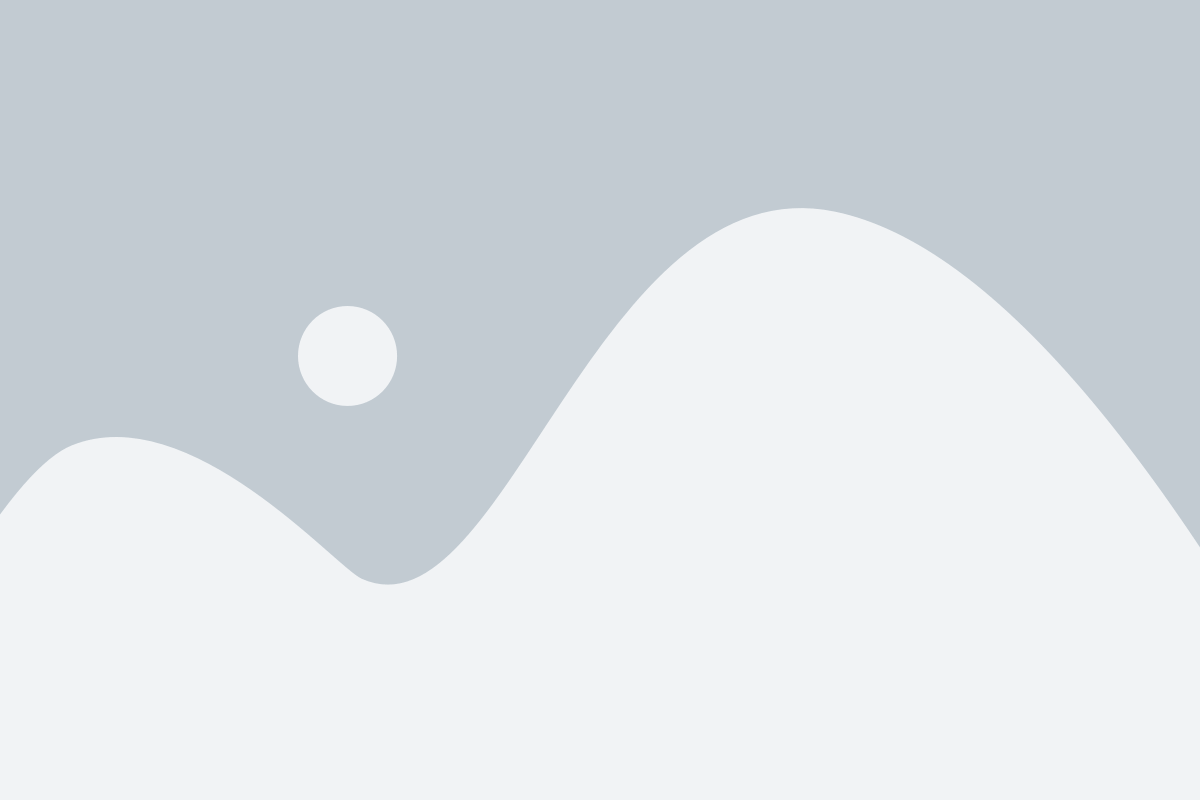
The adoption and benefit realisation from cognitive technologies is gaining increasing momentum. According to a PwC report, 72% of business executives surveyed believe that artificial intelligence (AI) will be a strong business advantage and 67% believe that a combination of human and machine intelligence is a more powerful entity than each one on its own.
Another survey conducted by Deloitte reports that on an average, 83% of respondents who have actively deployed AI in the enterprise see moderate to substantial benefits through AI – a number that goes further up with the number of AI deployments.
These studies make it abundantly clear that AI is occupying a high and increasing mindshare among business executives – who have a strong appreciation of the bottom line impact delivered by cognitive systems, through improved efficiencies.
AI-first Mindset
Having said that, with AI becoming more and more mainstream in an organisational setup, piecemeal implementations will deliver a lower marginal impact to organisations’ competitive advantage. While once early adopters were able to realise transformational benefits through siloed AI deployments, now that it is fast maturing as a must-have in the enterprise and we will need a different approach.
To realise true competitive advantage, organisations need to have an AI-first mindset. It is the new normal in accelerating business decisions. It was once said that every company is a technology company – meaning that all companies were expected to have mature technology backbones to deliver business impact and customer satisfaction. That dictum is now being amended to say – every company is a cognitive company.
To deliver on this promise, companies need to weave AI into the very fabric of their strategy. To realise competitive advantage tomorrow, we need to embed AI across the organisation today, with a strong, stable and scalable foundation. Here are three building blocks that are needed to create that robust foundation.
1. Enrich Data & Algorithm Repositories
If data is indeed the new oil (which it is), organisations that hold the deepest reserves and the most advanced refinery will be the ones that win in this new landscape. Companies having the most meaningful repository of data, along with fit-for-purpose proprietary algorithms will most likely enjoy a sizeable competitive advantage.
So, companies need to improve and re-invent their data generation and collection mechanisms. Data generation will help reduce their reliance on external data providers and help them own the data for conducting meaningful, real-time analysis by continuously enriching the data set.
Alongside, corporations also need to build an ‘algorithm factory’ – to speed up the development of accurate, fit-for-purpose and meaningful algorithms. The algorithm factory would need to push out data models in an iterative process in a way that improves the speed and accuracy.
This would enable the data and analysis capabilities of companies to grow in a scalable manner. While this task would largely fall under the aegis of data science teams, business teams would be required to provide timely interventions and feedback – to validate impact delivered by these models, and suggest course-corrections where necessary.
Another key aspect of this process is to enable a transparent cross-organisation view into these repositories. This will allow employees to collaborate and innovate rapidly by learning what is already been done and will reduce needless time and effort spent in developing something that’s already there.
2. AI Education for Workforce
Operationalising AI requires a convergence of different skill sets. According to the above-cited Deloitte survey, 37% of respondents felt that their managers didn’t understand cognitive technology – which was a hindrance to their AI deployments.
We need to mix different streams of people to build a scalable AI-centric organisation. For instance, business teams need to be continuously trained on the operational aspects of AI, its various types, use cases and benefits – to appreciate how AI can impact their area of business.
Technology teams need to be re-skilled around the development and deployment of AI applications. Data processing and analyst teams need to better understand how to build scalable computational models, which can run more autonomously and improve fast.
Unlike a typical technology transformation, AI transformation is a business reengineering exercise and requires cross-functional teams to collaborate and enrich their understanding of AI and how it impacts their functions, while building a scalable AI programme.
The implicit advantage of developing topical training programmes and involving a larger set of the workforce is to mitigate the FUD that is typically associated with automation initiatives. By giving employees the opportunity to learn and contribute in a meaningful way, we can eliminate bottlenecks, change-aversion and enable a successful AI transformation.
3. Ethical and Security Measures
The 4th Industrial Revolution will require a re-assessment of ethical and security practices around data, algorithms and applications that use the former two.
By introducing renewed standards and ethical codes, enterprises can address two important concerns people typically raise – how much power can/should AI exercise and how can we stay protected in cases of overreach.
We are already witnessing teething trouble – with accidents involving self-driving cars resulting in pedestrian deaths, and the continuing Facebook-Cambridge Analytica saga.
Building a strong grounding for AI systems will go a long way in improving customer and social confidence – that personal data is in safe hands and is protected from abuse – enabling them to provide an informed consent to their data. To that end, we need to continue refining our understanding around the ethical standards of AI implementations
AI and other cyber-physical systems are key components of the next generation of business. According to a report by semiconductor manufacturer, ARM, 61% of respondents believe that AI can make the world a better place. To increase that sentiment even further, and to make AI business-as-usual, and power the cognitive enterprise, it is critical that we subject machine intelligence to the same level of governance, scrutiny and ethical standards that we would apply to any core business process.
Related Posts
AIQRATIONS

The New Age Enterprise – Enabled by AI
Add Your Heading Text Here
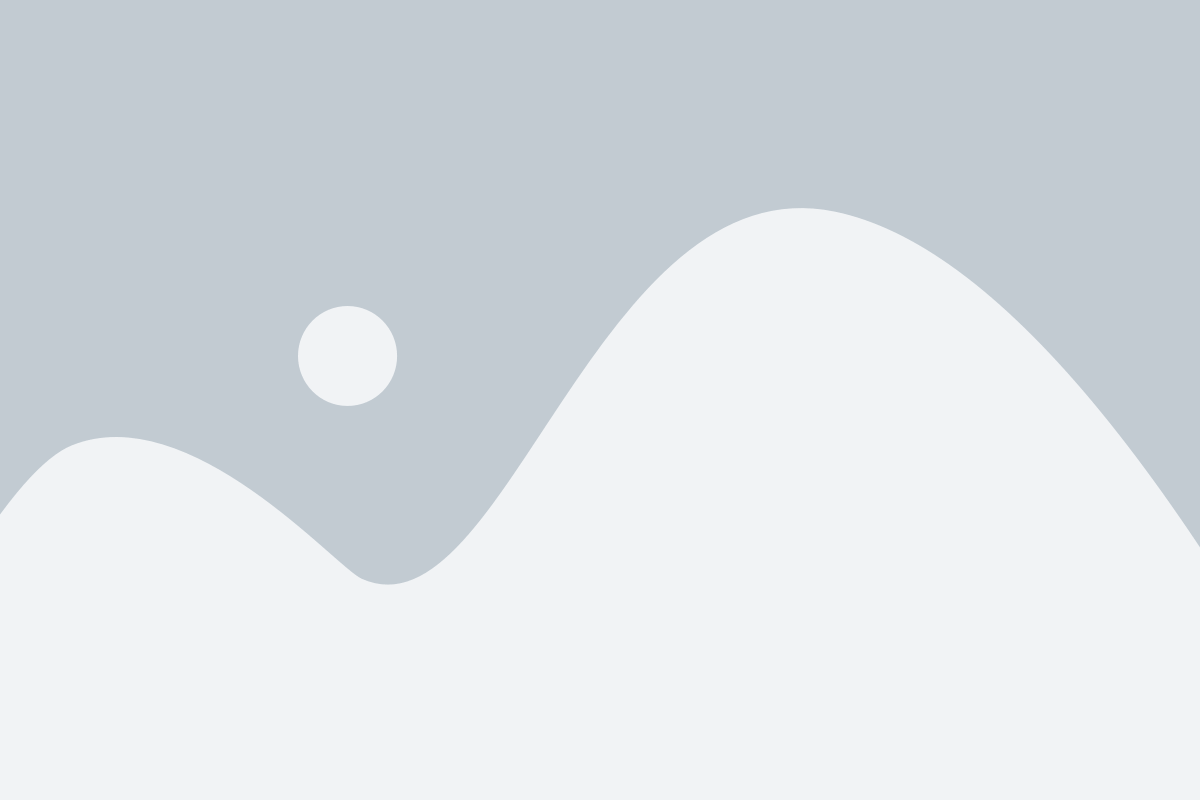
The excitement around artificial intelligence is palpable. It seems that not a day goes by without one of the giants in the industry coming out with a breakthrough application of this technology, or a new nuance is added to the overall body of knowledge. Horizontal and industry-specific use cases of AI abound and there is always something exciting around the corner every single day.
However, with the keen interest from global leaders of multinational corporations, the conversation is shifting towards having a strategic agenda for AI in the enterprise. Business heads are less interested in topical experiments and minuscule productivity gains made in the short term. They are more keen to understand the impact of AI in their areas of work from a long-term standpoint. Perhaps the most important question that they want to see answered is – what will my new AI-enabled enterprise look like?
The question is as strategic as it is pertinent. For business leaders, the most important issues are – improving shareholder returns and ensuring a productive workforce – as part of running a sustainable, future-ready business. Artificial intelligence may be the breakout technology of our time, but business leaders are more occupied with trying to understand just how this technology can usher in a new era of their business, how it is expected to upend existing business value chains, unlock new revenue streams, and deliver improved efficiencies in cost outlays. In this article, let us try to answer these questions.
AI is Disrupting Existing Value Chains
Ever since Michael Porter first expounded on the concept in his best-selling book, Competitive Advantage: Creating and Sustaining Superior Performance, the concept of the value chain has gained great currency in the minds of business leaders globally. The idea behind the value chain was to map out the interlinkages between the primary activities that work together to conceptualize and bring a product / service to market (R&D, manufacturing, supply chain, marketing, etc.), as well as the role played by support activities performed by other internal functions (finance, HR, IT etc.). Strategy leaders globally leverage the concept of value chains to improve business planning, identify new possibilities for improving business efficiency and exploit potential areas for new growth.
Now with AI entering the fray, we might see new vistas in the existing value chains of multinational corporations. For instance:
- Manufacturing is becoming heavily augmented by artificial intelligence and robotics. We are seeing these technologies getting a stronger foothold across processes requiring increasing sophistication. Business leaders need to now seriously consider workforce planning for a labor force that consists both human and artificial workers at their manufacturing units. Due attention should also be paid in ensuring that both coexist in a symbiotic and complementary manner.
- Logistics and Delivery are two other areas where we are seeing a steady growth in the use of artificial intelligence. Demand planning and fulfilment through AI has already reached a high level of sophistication at most retailers. Now Amazon – which handles some of the largest and most complex logistics networks in the world – is in advanced stages of bringing in unmanned aerial vehicles (drones) for deliveries through their Amazon Prime Air program. Business leaders expect outcomes to range from increased customer satisfaction (through faster deliveries) and reduction in costs for the delivery process.
- Marketing and Sales are constantly on the forefront for some of the most exciting inventions in AI. One of the most recent and evolved applications of AI is Reactful. A tool developed for eCommerce properties, Reactful helps drive better customer conversions by analyzing the clickstream and digital footprints of people who are on web properties and persuades them into making a purchase. Business leaders need to explore new ideas such as this that can help drive meaningful engagement and top line growth through these new AI-powered tools.
AI is Enabling New Revenue Streams
The second way business leaders are thinking strategically around AI is for its potential to unlock new sources of revenue. Earlier, functions such as internal IT were seen as a cost center. In today’s world, due to the cost and competitive pressure, areas of the business which were traditionally considered to be cost centers are require to reinvent themselves into revenue and profit centers. The expectation from AI is no different. There is a need to justify the investments made in this technology – and find a way for it to unlock new streams of revenue in traditional organizations. Here are two key ways in which business leaders can monetize AI:
- Indirect Monetization is one of the forms of leveraging AI to unlock new revenue streams. It involves embedding AI into traditional business processes with a focus on driving increased revenue. We hear of multiple companies from Amazon to Google that use AI-powered recommendation engines to drive incremental revenue through intelligent recommendations and smarter bundling. The action item for business leaders is to engage stakeholders across the enterprise to identify areas where AI can be deeply ingrained within tech properties to drive incremental revenue.
- Direct Monetization involves directly adding AI as a feature to existing offerings. Examples abound in this area – from Salesforce bringing in Einstein into their platform as an AI-centric service to cloud infrastructure providers such as Amazon and Microsoft adding AI capabilities into their cloud offerings. Business leaders should brainstorm about how AI augments their core value proposition and how it can be added into their existing product stack.
AI is Bringing Improved Efficiencies
The third critical intervention for a new AI-enabled enterprise is bringing to the fore a more cost-effective business. Numerous topical and early-stage experiments with AI have brought interesting success for reducing the total cost of doing business. Now is the time to create a strategic roadmap for these efficiency-led interventions and quantitatively measure their impact to business. Some food for thought for business leaders include:
- Supply Chain Optimization is an area that is ripe for AI-led disruption. With increasing varieties of products and categories and new virtual retailers arriving on the scene, there is a need for companies to reduce their outlay on the network that procures and delivers goods to consumers. One example of AI augmenting the supply chain function comes from Evertracker – a Hamburg-based startup. By leveraging IOT sensors and AI, they help their customers identify weaknesses such as delays and possible shortages early, basing their analysis on internal and external data. Business leaders should scout for solutions such as these that rely on data to identify possible tweaks in the supply chain network that can unlock savings for their enterprises.
- Human Resources is another area where AI-centric solutions can be extremely valuable to drive down the turnaround time for talent acquisition. One such solution is developed by Recualizer – which reduces the need for HR staff to scan through each job application individually. With this tool, talent acquisition teams need to first determine the framework conditions for a job on offer, while leaving the creation of assessment tasks to the artificial intelligence system. The system then communicates the evaluation results and recommends the most suitable candidates for further interview rounds. Business leaders should identify such game-changing solutions that can make their recruitment much more streamlined – especially if they receive a high number of applications.
- The Customer Experience arena also throws up very exciting AI use cases. We have now gone well beyond just bots answering frequently asked questions. Today, AI-enabled systems can also provide personalized guidance to customers that can help organizations level-up on their customer experience, while maintaining a lower cost of delivering that experience. Booking.com is a case in point. Their chatbot helps customers identify interesting activities and events that they can avail of at their travel destinations. Business leaders should explore such applications that provide the double advantage of improving customer experience, while maintaining strong bottom-line performance.
The possibilities for the new AI-enabled enterprises are as exciting as they are varied. The ideas shared in this article are by no means exhaustive, but hopefully seed in interesting ideas for powering improved business performance. Strategy leaders and business heads need to consider how their AI-led businesses can help disrupt their existing value chains for the better, and unlock new ideas for improving bottom-line and top-line performance. This will usher in a new era of the enterprise, enabled by AI.
Related Posts
AIQRATIONS
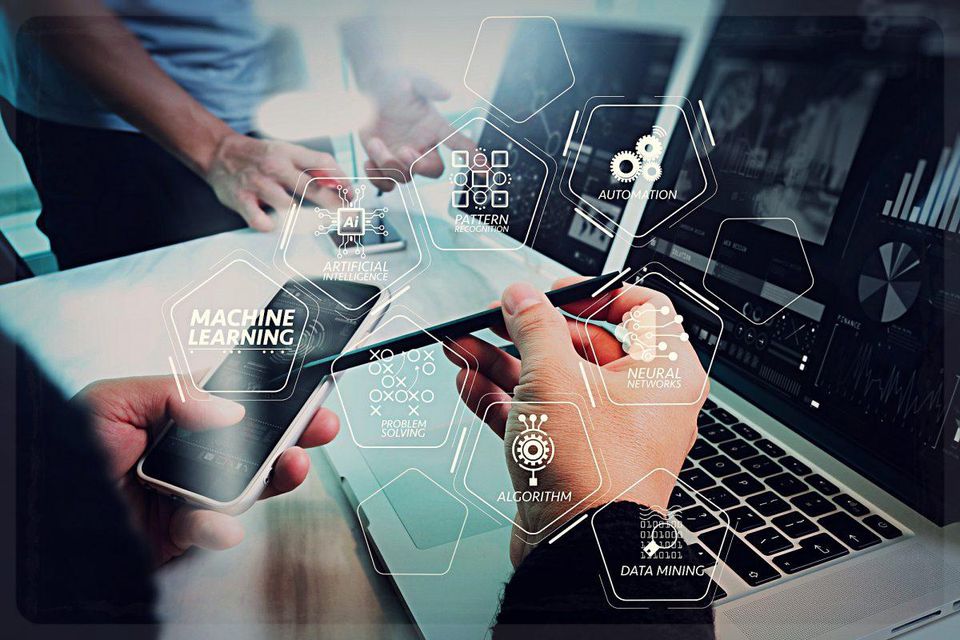
Reimagining Enterprise Decision-Making With Artificial Intelligence
Add Your Heading Text Here
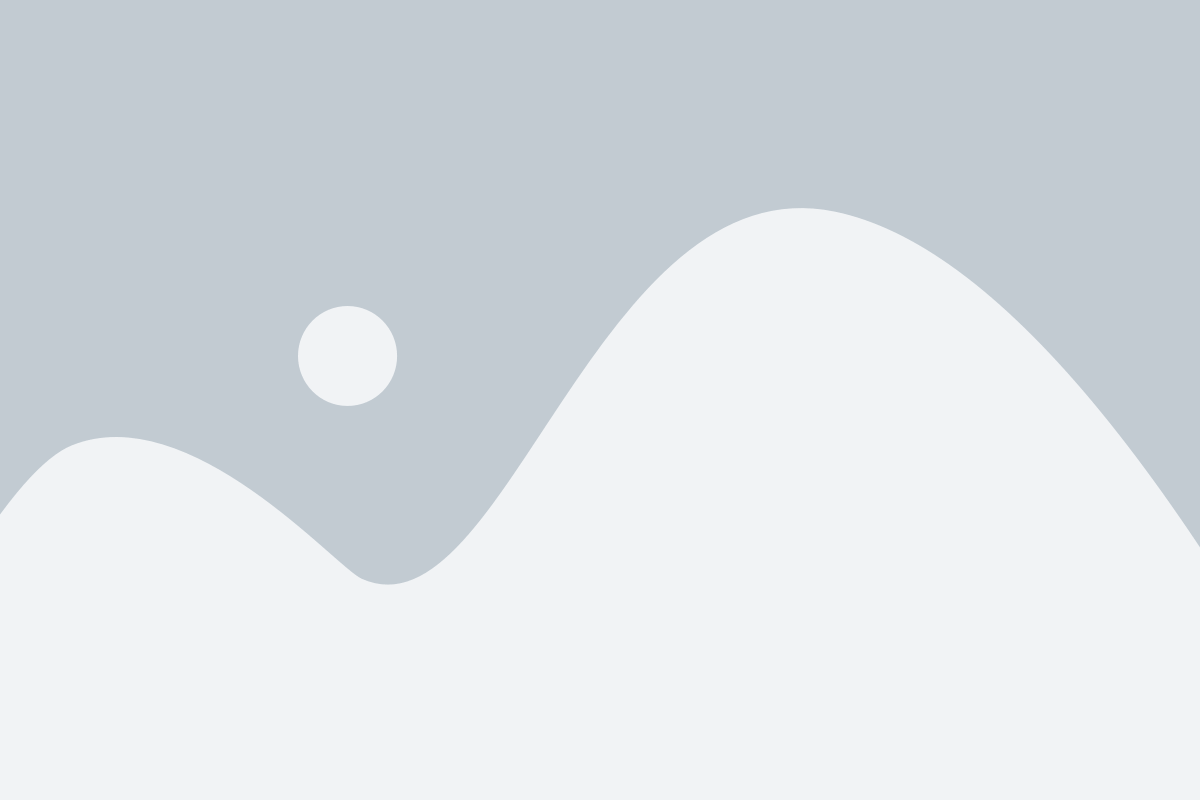
Artificial Intelligence will deliver revolutionary impact on how enterprises make decisions today. In the last few years alone, we have rapidly moved beyond heuristics-based decision-making to analytics-driven decision-support. In the VUCA phase, businesses globally are now pivoting to an AI-led, algorithm-augmented style of decision-making. With huge computing power and ever-increasing data storage and analytics prowess, we are entering a new paradigm, a probable and interesting scenario wherein, Artificial Intelligence will play a huge role in augmenting human intelligence and enabling decision-making with complete autonomy. The big hope is that this new paradigm will not only reduce human biases and errors that are common with heuristic decisions, but also reduce the time involved in making these critical decisions.
Here, I’ll attempt to focus on how we moved from simpler data driven decision-support to AI-powered decisions. The evolution of this technology has been breathtaking to behold and just might provide clues as to what we can expect in the future. Further, I’ll cover a few critical aspects that need to be inculcated by organizations on the AI transformation journey, and provide a few insightful cues that will make this journey exciting and fruitful.
Transformation of Decision-Making: From Analytics to AI
First, let us look at how we got here. Some truly pathbreaking events happened along the way while we were trying to make more accurate business decisions, leading us to reimagine how decisions will be made in the enterprise.
Organizations are Becoming Math Houses
With data deluge and digital detonation, combined with the appreciation of the fact that robust analytical capabilities lead to more informed decisions, we are witnessing AI savvy organizations rapidly maturing into ‘math houses.’ Data science – the ability to extract meaningful insights out of data has become de rigueur. Why? Because we now know that data, when seen in isolation, is inherently dumb. It is the ability to process this data and identify patterns and anomalies – using sophisticated algorithms and ensemble techniques – that makes all the difference. These self-intuitive algorithms are where real value resides – as they define the intelligence required to uncover insights and make smart recommendations. Organizations today are evolving into algorithm factories. There is a real understanding today that by enabling continuous advancement in mathematical algorithms, we can deliver consistent decisions based on prescribed as well as evolving business rules.
It is now an established reality that companies with robust mathematical capabilities possess a huge advantage over those that don’t. Indeed, it’s this math-house orientation that separates companies like Amazon and Google from the ones they leave in their wake, with their ability to understand their customers better, identify anomalies and recognize key patterns.
AI: From Predictive to Prescriptive
We saw a similar evolution in the age of analytics – wherein the science and value veered from descriptive analytics, providing diagnostics of past events to prescriptive analytics, helping see and shape the future. We are seeing a similar evolution in how AI gets leveraged in the enterprise and where its maximum value lies.
In early implementations, it was common to see AI as just a tool to predict and forecast future conditions, while accounting for the dynamism seen in the external environment. Today, AI-enabled decision-making is more prescriptive, with AI providing enterprises not just a look into the future, but also key diagnostics and suggestions on potential decision options and their payoffs. Such evolved applications of AI can help businesses make decisions that can potentially exploit more business opportunities, while averting potential threats much earlier.
Mr. Algorithm to Drive Decision Making
The culmination of this AI-era advancement would be the introduction of smart algorithms in every walk of life and business. Algorithms will become further mainstream leading to what will be the most sweeping business change since the industrial revolution. Organizations – those that already aren’t – will start developing a suite of algorithmic IP’s that will de-bias most enterprise decisions.
If Mr. Algorithm is going to drive most enterprise decisions of tomorrow, we need to create some checks and balances to ensure that it does not go awry. It is more critical today than ever before that the algorithmic suite developed by enterprises has a strong grounding in ethics and can handle situations appropriately for which explicit training may not have been provided.
How to Enable this AI Era of Change
Ushering into an AI-centric era of decision-making will require organizational transformation from business, cultural and technical standpoints. The following facets will be the enablers of this change:
Developing an Engineering Mindset
Instrumenting AI in the enterprise requires a combination of data scientists and computer scientists. As AI matures in the enterprise, the users, use cases and data will increase exponentially. To deliver impactful AI applications, scale and extensibility is critically important. This is where having an engineering mindset comes in. Imbibing an engineering mindset will help standardize the use of these applications while ensuring that they are scalable and extensible.
Learning, Unlearning, Relearning
The other critical aspect to a culture where AI can thrive is creating an environment supporting continuous unlearning and relearning. AI can succeed if the people developing and operating it are rewarded for continuous experimentation and exploration. And just like AI, people should be encouraged to incorporate feedback loops and learn continuously. As technology matures it’s important that the existing workforce keeps up. For one, it’s critical that the knowledge of algorithm theory, applied math alongside training on AI library and developer tools, is imparted into the workforce – and is continuously updated to reflect new breakthroughs in this space.
Embedding Design-Thinking and Behavioral Science at the Center of this Transformation
Finally, given the nature of AI applications, it’s critical that they are consumed voraciously. User input very often activates the learning cycles of artificial intelligence applications. To ensure high usage of these applications, it’s very important that we put the user at the center while designing these applications. This is where the application of behavioral sciences and human-centered design will deliver impact. By imparting empathy in these applications for the user, we will be able to design better and more useful AI applications.
As we augment decision-making with algorithmic, AI-centered systems and platforms – the big expectation is that they will bring untold efficiencies in terms of cost, alongside improvement in the speed and quality with which decisions get made. It’s time to reimagine and deliver on enterprise decision-making that is increasingly shaped through artificial intelligence. These aspects – how the AI is progressing and how to exploit its potential are of paramount importance to keep in mind for an AI transformation.
Related Posts
AIQRATIONS
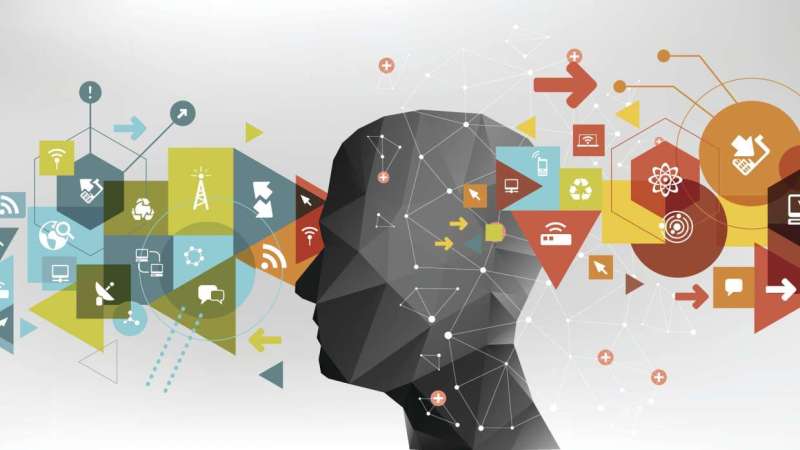
Design Thinking | Behavioural Sciences: Strategic Elements to Building a Successful AI Enterprise
Add Your Heading Text Here
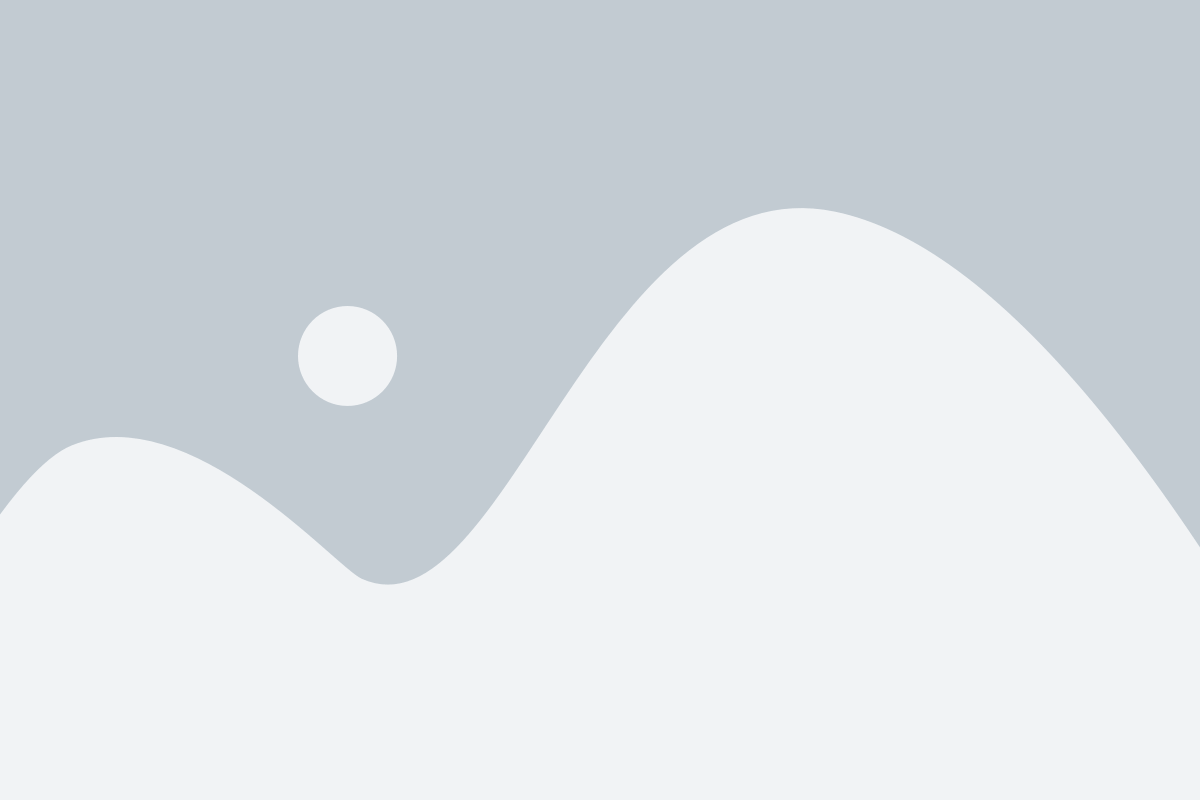
Today’s artificial intelligence (AI) revolution has been made possible by the algorithm revolution. The machine learning algorithms researchers have been developing for decades, when cleverly applied to today’s web-scale data sets, can yield surprisingly good forms of intelligence. For instance, the United States Postal Service has long used neural network models to automatically read handwritten zip code digits. Today’s deep learning neural networks can be trained on millions of electronic photographs to identify faces, and similar algorithms may increasingly be used to navigate automobiles and identify tumors in X-rays. The IBM Watson information retrieval system could triumph on the game show “Jeopardy!” partly because most human knowledge is now stored electronically.
But current AI technologies are a collection of big data-driven point solutions, and algorithms are reliable only to the extent that the data used to train them is complete and appropriate. One-off or unforeseen events that humans can navigate using common sense can lead algorithms to yield nonsensical outputs.
Design thinking is defined as human-centric design that builds upon the deep understanding of our users (e.g., their tendencies, propensities, inclinations, behaviours) to generate ideas, build prototypes, share what you’ve made, embrace the art of failure (i.e., fail fast but learn faster) and eventually put your innovative solution out into the world. And fortunately for us humans (who really excel at human-centric things), there is a tight correlation between the design thinking and artificial intelligence.
Artificial intelligence technologies could reshape economies and societies, but more powerful algorithms do not automatically yield improved business or societal outcomes. Human-centered design thinking can help organizations get the most out of cognitive technologies.
Divergence from More Powerful Intelligence To More Creative Intelligence
While algorithms can automate many routine tasks, the narrow nature of data-driven AI implies that many other tasks will require human involvement. In such cases, algorithms should be viewed as cognitive tools capable of augmenting human capabilities and integrated into systems designed to go with the grain of human—and organizational—psychology. We don’t want to ascribe to AI algorithms more intelligence than is really there. They may be smarter than humans at certain tasks, but more generally we need to make sure algorithms are designed to help us, not do an end run around our common sense.
Design Thinking at Enterprise Premise
Although cognitive design thinking is in its early stages in many enterprises, the implications are evident. Eschewing versus embracing design thinking can mean the difference between failure and success. For example, a legacy company that believes photography hinges on printing photographs could falter compared to an internet startup that realizes many customers would prefer to share images online without making prints, and embraces technology that learns faces and automatically generates albums to enhance their experience.
To make design thinking meaningful for consumers, companies can benefit from carefully selecting use cases and the information they feed into AI technologies. In determining which available data is likely to generate desired results, enterprises can start by focusing on their individual problems and business cases, create cognitive centres of excellence, adopt common platforms to digest and analyze data, enforce strong data governance practices, and crowdsource ideas from employees and customers alike.
In assessing what constitutes proper algorithmic design, organizations may confront ethical quandaries that expose them to potential risk. Unintended algorithmic bias can lead to exclusionary and even discriminatory practices. For example, facial recognition software trained on insufficiently diverse data sets may be largely incapable of recognizing individuals with different skin tones. This could cause problems in predictive policing, and even lead to misidentification of crime suspects. If the training data sets aren’t really that diverse, any face that deviates too much from the established norm will be harder to detect. Accordingly, across many fields, we can start thinking about how we create more inclusive code and employ inclusive coding practices.
CXO Strategy for Cognitive Design Thinking
CIOs can introduce cognitive design thinking to their organizations by first determining how it can address problems that conventional technologies alone cannot solve. The technology works with the right use cases, data, and people, but demonstrating value is not always simple. However, once CIOs have proof points that show the value of cognitive design thinking, they can scale them up over time.
CIOs benefit from working with business stakeholders to identify sources of value. It is also important to involve end users in the design and conception of algorithms used to automate or augment cognitive tasks. Make sure people understand the premise of the model so they can pragmatically balance algorithm results with other information.
Enterprise Behavioral Science – From Insights to Influencing Business Decisions
Every January, how many people do you know say that they want to resolve to save more, spend less, eat better, or exercise more? These admirable goals are often proclaimed with the best of intentions, but are rarely achieved. If people were purely logical, we would all be the healthiest versions of ourselves.
However, the truth is that humans are not 100% rational; we are emotional creatures that are not always predictable. Behavioral economics evolved from this recognition of human irrationality. Behavioral economics is a method of economic analysis that applies psychological insights into human behavior to explain economic decision-making.
Decision making is one of the central activities of business – hundreds of billions of decisions are made everyday. Decision making sits at the heart of innovation, growth, and profitability, and is foundational to competitiveness. Despite this degree of importance, decision making is poorly understood, and badly supported by tools. A study by Bain & Company found that decision effectiveness is 95% correlated with companies’ financial performance.
Enterprise Behavioral Science is not only about understanding potential outcomes, but to completely change outcomes, and more specifically, change the way in which people behave. Behavioral Science tells us that to make a fundamental change in behavior that will affect the long-term outcome of a process, we must insert an inflection point.
As an example, you are a sales rep and two years ago your revenue was $1 million. Last year it was $1.1 million, and this year you expect $1.2 million in sales. The trend is clear, and your growth has been linear and predictable. However, there is a change in company leadership and your management has increased your quota to $2 million for next year. What is going to motivate you to almost double your revenues? The difference between expectations ($2 million) and reality ($1.2 million) is often referred to as the “behavioral gap” . When the behavioral gap is significant, an inflection point is needed to close that gap. The right incentive can initiate an inflection point and influence a change in behavior. Perhaps that incentive is an added bonus, President’s Club eligibility, a promotion, etc.
Cognitive Design Thinking – The New Indispensable Reskilling Avenue
Artificial intelligence, machine learning, big data analytics and mobile and software development will be the top technology areas where the need for re-skilling will be the highest. India will need 700 million skilled workforce by 2022 to meet the demands of a growing economy. Hence, while there is a high probability that machine learning and artificial intelligence will play an important role in whatever job you hold in the future, there is one way to “future-proof” your career…embrace the power of design thinking.
In fact, integrating design thinking and artificial intelligence can give you “super powers” that future-proof whatever career you decide to pursue. To meld these two disciplines together, one must:
- Understand where and how artificial intelligence and behavioural science can impact your business initiatives. While you won’t need to write machine learning algorithms, business leaders do need to learn how to “Think like a data scientist” in order understand how AI can optimize key operational processes, reduce security and regulatory risks, uncover new monetization opportunities.
- Understand how design thinking techniques, concepts and tools can create a more compelling and emphatic user experience with a “delightful” user engagement through superior insights into your customers’ usage objectives, operating environment and impediments to success.
Design thinking is a mindset. IT firms are trying to move up the curve. Higher-end services that companies can charge more is to provide value and for that you need to know that end-customers needs. For example, to provide value services to banking customers is to find out what the bank’s customer needs are in that country the banking client is based. Latent needs come from a design thinking philosophy where you observe customer data, patterns and provide a solution that the customer does not know. Therefore, Companies will hire design thinkers as they can predict what the consumer does not know and hence charge for the product/service from their clients. Idea in design thinking is to provide agile product creation or solutions.
Without Design Thinking & Behavioural Science, AI Will be Only an Incremental Value
Though organizations understand the opportunity that big data presents, many struggles to find a way to unlock its value and use it in tandem with design thinking – making “big data a colossal waste of time & money.” Only by combining quantitative insights gathered using AI, machine/deep learning, and qualitative research through behavioural science, and finally design thinking to uncover hidden patterns and leveraging it to understand what the customer would want, will we be able to paint a complete picture of the problem at hand, and help drive towards a solution that would create value for all stakeholders.