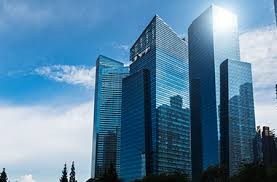
Is your Enterprise AI Ready: Strategic considerations for the CXOs
Add Your Heading Text Here
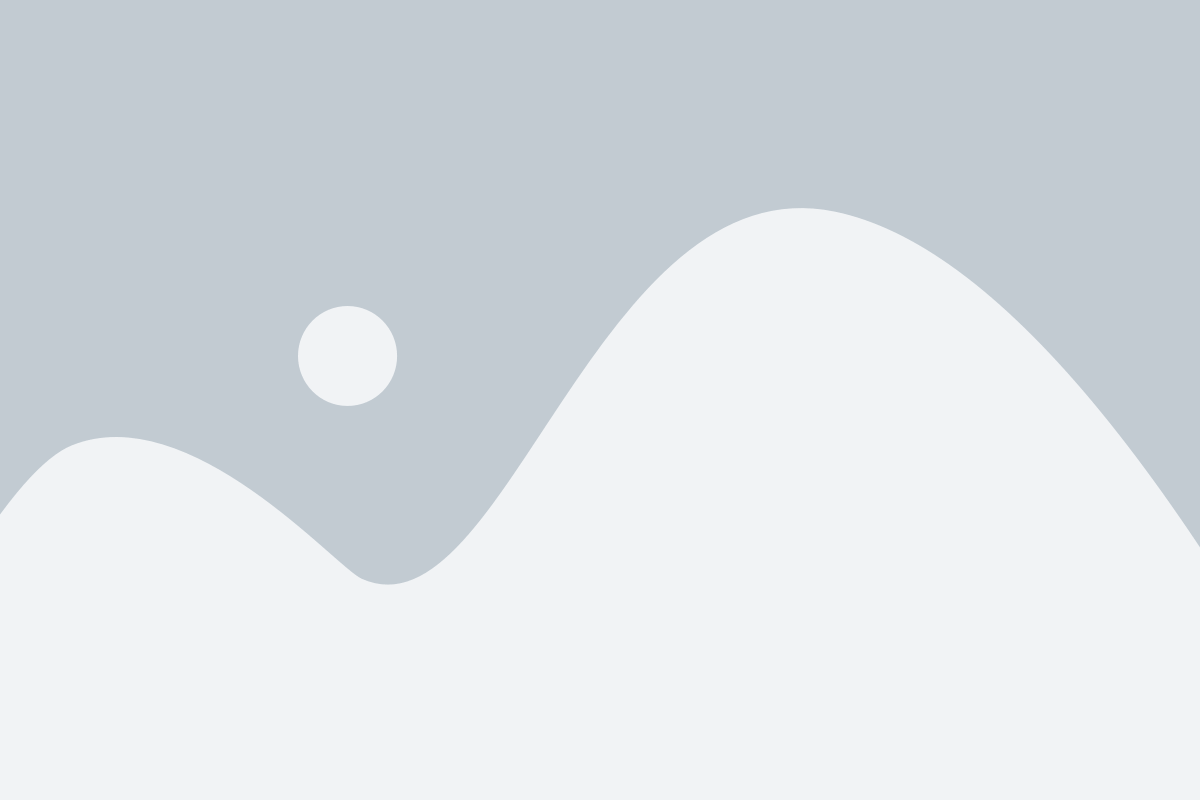
At the enterprise level, AI assumes enormous power and potential , it can disrupt, innovate, enhance, and in many cases totally transform businesses . Multiple reports predicts a 300% increase in AI investment in 2020-2022 and estimates that the AI market amongst several exponential technologies will be the highest . There are solid instances that the AI investment can pay off—if CEO’s can adopt the right strategy. Organizations that deploy AI strategically enjoy advantages ranging from cost reductions and higher productivity to top-line benefits such as increasing revenue and profits, enhanced customer experiences, and working-capital optimization. Multiple surveys also shows that the companies winning at AI are also more likely to enjoy broader businesses.
So How to make your Enterprise AI Ready?
72 % of the organizations say they are getting significant impact from AI. But these enterprises have taken clear, practical steps to get the results they want. Here are five of their strategic orientation to embark on the process to make AI Enterprise Ready :
- Core AI A-team assimilation with diversified skill sets
- Evangelize AI amongst senior management
- Focus on process, not function
- Shift from system-of-record to system-of-intelligence apps, platforms
- Encourage innovation and transformation
Core AI A-team assimilation with diversified skill sets
Through 2022, organization using cognitive ergonomics and system design in new AI projects will achieve long term success four times more often than others
With massive investments in AI startups in 2021 alone, and the exponential efficiencies created by AI, this evolution will happen quicker than many business leaders are prepared for. If you aren’t sure where to start, don’t worry – you’re not alone. The good news is that you still have options:
- You can acquire, or invest in a company applying AI/ML in your market, and gain access to new product and AI/ML talent.
- You can seek to invest as a limited partner in a few early stage AI focused VC firms, gaining immediate access and exposure to vetted early stage innovation, a community of experts and market trends.
- You can set out to build an AI-focused division to optimize your internal processes using AI, and map out how AI can be integrated into your future products. But recruiting in the space is painful and you will need a strong vision and sense of purpose to attract and retain the best.
Process Based Focus Rather than Function Based
One critical element differentiates AI success from AI failure: strategy. AI cannot be implemented piecemeal. It must be part of the organization’s overall business plan, along with aligned resources, structures, and processes. How a company prepares its corporate culture for this transformation is vital to its long-term success. That includes preparing talent by having senior management that understands the benefits of AI; fostering the right skills, talent, and training; managing change; and creating an environment with processes that welcome innovation before, during, and after the transition.
The challenge of AI isn’t just the automation of processes—it’s about the up-front process design and governance you put in to manage the automated enterprise. The ability to trace the reasoning path AI use to make decisions is important. This visibility is crucial in banking & financial services, where auditors and regulators require firms to understand the source of a machine’s decision.
Evangelize AI amongst senior management
One of the biggest challenges to enterprise transformation is resistance to change. Surveys have found that senior management is the inertia led to AI implementation. C-suite executives may not have warmed up to it either. There is such a lack of understanding about the benefits which AI can bring that the C-suite or board members simply don’t want to invest in it, nor do they understand that failing to do so will adversely affect their top & bottom line and even cause them to go out of business. Regulatory uncertainty about AI, rough experiences with previous technological innovation, and a defensive posture to better protect shareholders, not stakeholders, may be contributing factors.
Pursuing AI without senior management support is difficult. Here the numbers again speak for themselves. The majority of leading AI companies (68%) strongly agree that their senior management understands the benefits AI offers. By contrast, only 7% of laggard firms agree with this view. Curiously, though, the leading group still cites the lack of senior management vision as one of the top two barriers to the adoption of AI.
The Dawn of System-of-Intelligence Apps & Platforms
Analysts report predicts that an Intelligence stack will gain rapid adoption in enterprises as IT departments shift from system-of-record to system-of-intelligence apps, platforms, and priorities. The future of enterprise software is being defined by increasingly intelligent applications today, and this will accelerate in the future.
By 2022, AI platform services will cannibalize revenues for 30% of market leading companies
It will be commonplace for enterprise apps to have machine learning algorithms that can provide predictive insights across a broad base of scenarios encompassing a company’s entire value chain. The potential exists for enterprise apps to change selling and buying behaviour, tailoring specific responses based on real-time data to optimize discounting, pricing, proposal and quoting decisions.
The Process of Supporting Innovation
Besides developing capabilities among employees, an organization’s culture and processes must also support new approaches and technologies. Innovation waves take a lot longer because of the human element. You can’t just put posters on the walls and say, ‘Hey, we have become an AI-enabled company, so let’s change the culture.’ The way it works is to identify and drive visible examples of adoption. Algorithmic trading, image recognition/tagging, and patient data processing are predicted to the top AI uses cases by 2025. It is forecasted that predictive maintenance and content distribution on social media will be the fourth and fifth highest revenue producing AI uses cases over the next eight years.
In the End, it’s about Transforming Enterprise
AI is part of a much bigger process of re-engineering enterprises. That is the major difference between the automation attempts of yesteryear and today’s AI: AI is completely integrated into the fabric of business, allowing private and public-sector organizations to transform themselves and society in profound ways. Enterprises that will deploy AI at full scale will reap tangible benefits at both strategic & operational levels.
Related Posts
AIQRATIONS
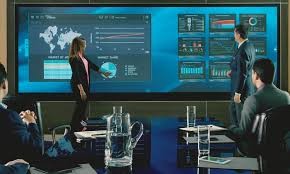
Reimagine Business Strategy & Operating Models with AI : The CXO’s Playbook
Add Your Heading Text Here
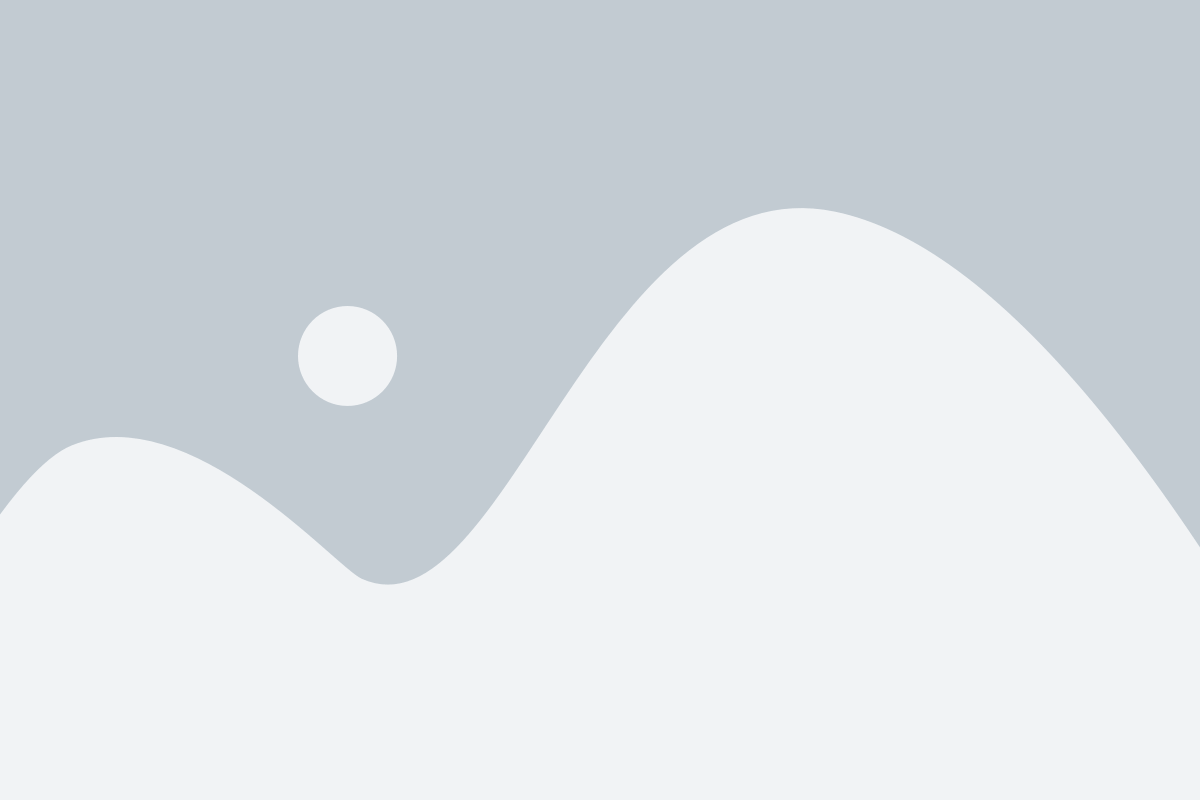
AlphaGo caused a stir by defeating 18-time world champion Lee Sedol in Go, a game thought to be impenetrable by AI for another 10 years. AlphaGo’s success is emblematic of a broader trend: An explosion of data and advances in algorithms have made technology smarter than ever before. Machines can now carry out tasks ranging from recommending movies to diagnosing cancer — independently of, and in many cases better than, humans. In addition to executing well-defined tasks, technology is starting to address broader, more ambiguous problems. It’s not implausible to imagine that one day a “strategist in a box” could autonomously develop and execute a business strategy. I have spoken to several CXOs and leaders who express such a vision — and they would like to embed AI in the business strategy and their operating models
Business Processes – Increasing productivity by reducing disruptions
AI algorithms are not natively “intelligent.” They learn inductively by analyzing data. Most leaders are investing in AI talent and have built robust information infrastructures, Airbus started to ramp up production of its new A350 aircraft, the company faced a multibillion-euro challenge. The plan was to increase the production rate of that aircraft faster than ever before. To do that, they needed to address issues like responding quickly to disruptions in the factory. Because they will happen. Airbus turned to AI , It combined data from past production programs, continuing input from the A350 program, fuzzy matching, and a self-learning algorithm to identify patterns in production problems.AI led to rectification of about 70% of the production disruptions for Airbus, by matching to solutions used previously — in near real time.
Just as it is enabling speed and efficiency at Airbus, AI capabilities are leading directly to new, better processes and results at other pioneering organizations. Other large companies, such as BP, Wells Fargo, and Ping , an Insurance, are already solving important business problems with AI. Many others, however, have yet to get started.
Integrated Strategy Machine – The Implementation Scope of AI @ scale
The integrated strategy machine is the AI analogy of what new factory designs were for electricity. In other words, the increasing intelligence of machines could be wasted unless businesses reshape the way they develop and execute their strategies. No matter how advanced technology is, it needs human partners to enhance competitive advantage. It must be embedded in what we call the integrated strategy machine. An integrated strategy machine is the collection of resources, both technological and human, that act in concert to develop and execute business strategies. It comprises a range of conceptual and analytical operations, including problem definition, signal processing, pattern recognition, abstraction and conceptualization, analysis, and prediction. One of its critical functions is reframing, which is repeatedly redefining the problem to enable deeper insights.
Amazon represents the state-of-the-art in deploying an integrated strategy machine. It has at least 21 AI systems, which include several supply chain optimization systems, an inventory forecasting system, a sales forecasting system, a profit optimization system, a recommendation engine, and many others. These systems are closely intertwined with each other and with human strategists to create an integrated, well-oiled machine. If the sales forecasting system detects that the popularity of an item is increasing, it starts a cascade of changes throughout the system: The inventory forecast is updated, causing the supply chain system to optimize inventory across its warehouses; the recommendation engine pushes the item more, causing sales forecasts to increase; the profit optimization system adjusts pricing, again updating the sales forecast.
Manufacturing Operations – An AI assistant on the floor
CXOs at industrial companies expect the largest effect in operations and manufacturing. BP plc, for example, augments human skills with AI in order to improve operations in the field. They have something called the BP well advisor that takes all of the data that’s coming off of the drilling systems and creates advice for the engineers to adjust their drilling parameters to remain in the optimum zone and alerts them to potential operational upsets and risks down the road. They are also trying to automate root-cause failure analysis to where the system trains itself over time and it has the intelligence to rapidly assess and move from description to prediction to prescription.
Customer-facing activities – Near real time scoring
Ping An Insurance Co. of China Ltd., the second-largest insurer in China, with a market capitalization of $120 billion, is improving customer service across its insurance and financial services portfolio with AI. For example, it now offers an online loan in three minutes, thanks in part to a customer scoring tool that uses an internally developed AI-based face-recognition capability that is more accurate than humans. The tool has verified more than 300 million faces in various uses and now complements Ping An’s cognitive AI capabilities including voice and imaging recognition.
AI for Different Operational Strategy Models
To make the most of this technology implementation in various business operations in your enterprise, consider the three main ways that businesses can or will use AI:
- Insights enabled intelligence
Now widely available, improves what people and organizations are already doing. For example, Google’s Gmail sorts incoming email into “Primary,” “Social,” and “Promotion” default tabs. The algorithm, trained with data from millions of other users’ emails, makes people more efficient without changing the way they use email or altering the value it provides. Assisted intelligence tends to involve clearly defined, rules-based, repeatable tasks.
Insights based intelligence apps often involve computer models of complex realities that allow businesses to test decisions with less risk. For example, one auto manufacturer has developed a simulation of consumer behaviour, incorporating data about the types of trips people make, the ways those affect supply and demand for motor vehicles, and the variations in those patterns for different city topologies, marketing approaches, and vehicle price ranges. The model spells out more than 200,000 variations for the automaker to consider and simulates the potential success of any tested variation, thus assisting in the design of car launches. As the automaker introduces new cars and the simulator incorporates the data on outcomes from each launch, the model’s predictions will become ever more accurate.
2. Recommendation based Intelligence
Recommendation based Intelligence, emerging today, enables organizations and people to do things they couldn’t otherwise do. Unlike insights enabled intelligence, it fundamentally alters the nature of the task, and business models change accordingly.
Netflix uses machine learning algorithms to do something media has never done before: suggest choices customers would probably not have found themselves, based not just on the customer’s patterns of behaviour, but on those of the audience at large. A Netflix user, unlike a cable TV pay-per-view customer, can easily switch from one premium video to another without penalty, after just a few minutes. This gives consumers more control over their time. They use it to choose videos more tailored to the way they feel at any given moment. Every time that happens, the system records that observation and adjusts its recommendation list — and it enables Netflix to tailor its next round of videos to user preferences more accurately. This leads to reduced costs and higher profits per movie, and a more enthusiastic audience, which then enables more investments in personalization (and AI).
3. Decision enabled Intelligence
Being developed for the future, Decision enabled intelligence creates and deploys machines that act on their own. Very few intelligence systems — systems that make decisions without direct human involvement or oversight — are in widespread use today. Early examples include automated trading in the stock market (about 75 percent of Nasdaq trading is conducted autonomously) and facial recognition. In some circumstances, algorithms are better than people at identifying other people. Other early examples include robots that dispose of bombs, gather deep-sea data, maintain space stations, and perform other tasks inherently unsafe for people.
As you contemplate the deployment of artificial intelligence at scale , articulate what mix of the three approaches works best for you.
a) Are you primarily interested in upgrading your existing processes, reducing costs, and improving productivity? If so, then start with insights enabled intelligence with a clear AI strategy roadmap
b) Do you seek to build your business around something new — responsive and self-driven products, or services and experiences that incorporate AI? Then pursue an decision enabled intelligence approach, probably with more complex AI applications and robust infrastructure
c) Are you developing a genuinely new platform ? In that case, think of building first principles of AI led strategy across the functionalities and processes of the platform .
CXO’s need to create their own AI strategy playbook to reimagine their business strategies and operating models and derive accentuated business performance.
Related Posts
AIQRATIONS
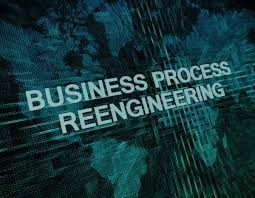
“RE-ENGINEERING” BUSINESSES – THINK “AI” led STRATEGY
Add Your Heading Text Here
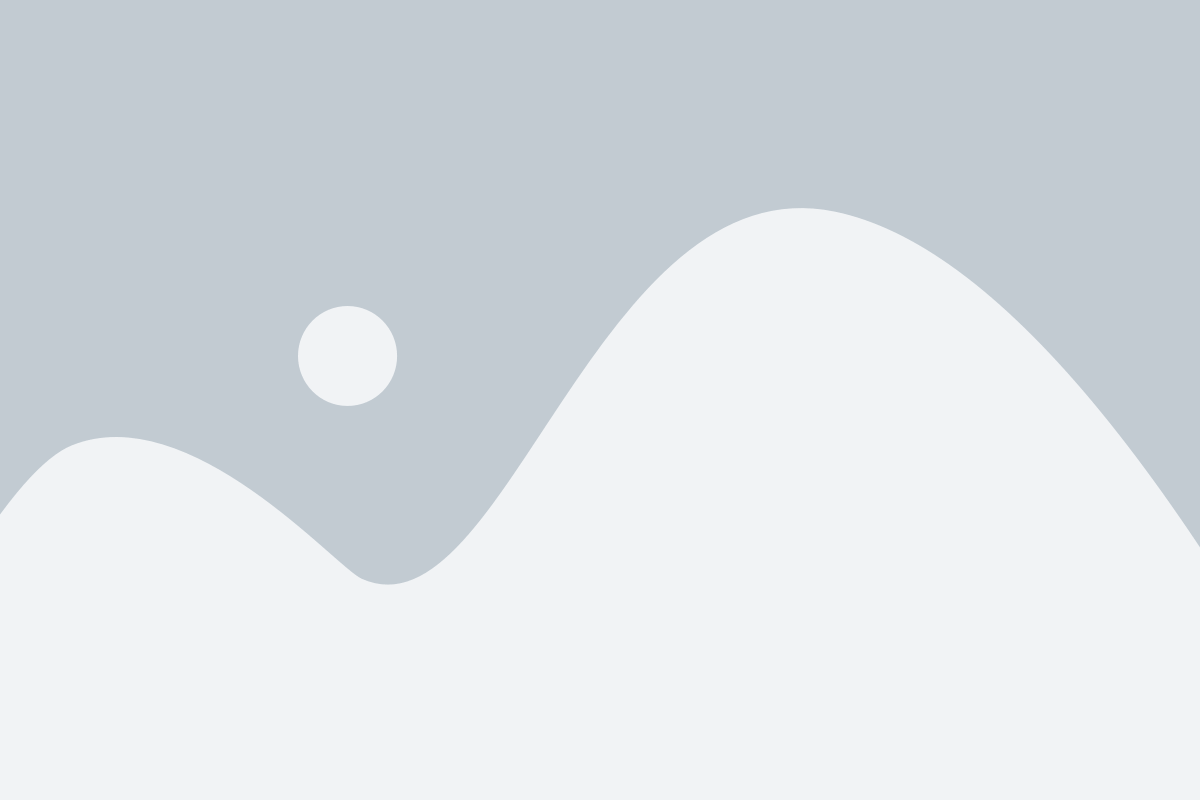
AI adoption across industries is galloping at a rapid pace and resulting benefits are increasing by the day, some businesses are challenged by the complexity and confusion that AI can generate. Enterprises can get stuck trying to analyse all that’s possible and all that they could do through Ai, when they should be taking that next step of recognizing what’s important and what they should be doing — for their customers, stakeholders, and employees. Discovering real business opportunities and achieving desired outcomes can be elusive. To overcome this, enterprises should pursue a constant attempt to re-engineer their AI strategy to generate insights & intelligence that leads to real outcomes
Re-engineering Data Architecture & Infrastructure
To successfully derive value from data immediately, there is a need for faster data analysis than is currently available using traditional data management technology. With the explosion of digital analytics, social media, and the “Internet of things” (IoT) there is an opportunity to radically re-engineer data architecture to provide organizations with a tiered approach to data collection, with real-time and historical data analyses. Infrastructure-as-a-service for AI is the combination of components that enables architecture that delivers the right business outcomes. Developing this architecture involves aspects of design of the cluster computing power, networking, and innovations in software that enable advanced technology services and interconnectivity. Infrastructure is the foundation for optimal processing and storage of data and is an important which is also the foundation for any data farm.
The new era of AI led infrastructure is virtualized (analytics) environments also can be referred to as the next Big “V” of big data. The virtualization infrastructure approach has several advantages, such as scalability, ease of maintenance, elasticity, cost savings due better utilization of resources, and the abstraction of the external layer from the internal implementation (back-end) of a service or resource. Containers are the trending technology making headlines recently, which is an approach to virtualization and cloud-enabled data centres. Fortune 500 companies have begun to “containerize” their servers, data centre and cloud applications with Docker. Containerization excludes all of the problems of virtualization by eliminating hypervisor and its VMs. Each application is deployed in its own container, which runs on the “bare metal” of the server plus a single, shared instance of the operating system.
AI led Business Process Re-Engineering
The BPR methodologies of the past have significantly contributed to the development of today’s enterprises. However, today’s business landscape has become increasingly complex and fast-paced. The regulatory environment is also constantly changing. Consumers have become more sophisticated and have easy access to information, on-the-go. Staying competitive in the present business environment requires organizations to go beyond process efficiencies, incremental improvements and enhancing transactional flow. Now, organizations need to have a comprehensive understanding of its business model through an objective and realistic grasp of its business processes. This entails having organization-wide insights that show the interdependence of various internal functions while taking into consideration regulatory requirements and shifting consumer tastes.
Data is the basis on which fact-based analysis is performed to obtain objective insights of the organization. In order to obtain organization-wide insights, management needs to employ AI capabilities on data that resides both inside and outside its organization. However, an organization’s AI capabilities are primarily dependent on the type, amount and quality of data it possesses.
The integration of an organization’s three key dimensions of people, process and technology is also critical during process design. The people are the individuals responsible and accountable for the organization’s processes. The process is the chain of activities required to keep the organization running. The technology is the suite of tools that support, monitor and ensure consistency in the application of the process. The integration of all these, through the support of a clear governance structure, is critical in sustaining a fact-based driven organizational culture and the effective capture, movement and analysis of data. Designing processes would then be most effective if it is based on data-driven insights and when AI capabilities are embedded into the re-engineered processes. Data-driven insights are essential in gaining a concrete understanding of the current business environment and utilizing these insights is critical in designing business processes that are flexible, agile and dynamic.
Re-engineering Customer Experience (CX) – The new paradigm
It’s always of great interest to me to see new trends emerge in our space. One such trend gaining momentum is enterprise looking at solving customer needs & expectations with what I’d describe as re-engineering customer experience . Just like everything else in our industry, changes in consumer behaviour caused by mobile and social trends are disrupting the CX space. Just a few years ago, web analytics solutions gave brands the best view into performance of their digital business and user behaviours. Fast-forward to today, and this is often not the case. With the growth in volume and importance of new devices, digital channels and touch points, CX solutions are now just one of the many digital data silos that brands need to deal with and integrate into the full digital picture. While some vendors may now offer ways for their solutions to run in different channels and on a range of devices, these capabilities are often still a work in progress. Many enterprises today find their CX solution as another critical set of insights that must be downloaded daily into a omni-channel AI data store and then run visualization to provide cross-channel business reporting.
Re-shaping Talent Acquisition and Engagement with AI
AI s is causing disruption in virtually every function but talent acquisition t is one of the more recent to get a business refresh. A new data driven approach to talent management is reshaping the way organizations find and hire staff, while the power of talent analytics is also changing how HR tackles employee retention and engagement. The implications for anyone hoping to land a job, and for businesses that have traditionally relied on personal relationships are extreme, but robots and algorithms will not yet completely replace human interaction.AI will certainly help to identify talent in specific searches. rather than relying on a rigorous interview process and resume, employers are able to “mine” through deep reserves of information, including from your online footprint. The real value will be in identifying personality types, abilities, and other strengths to help create well-rounded teams. Also, companies are also using people analytics to understand the stress levels of their employees to ensure long-term productiveness and wellness.
The Final Word
Based on my experiences with clients across enterprises , GCCs ,start-ups ; alignment among the three key dimensions of talent, process and AI led technology within a robust governance structure are critical to effectively utilize AI and remain competitive in the current business environment. AI is able to open doors to growth & scalability through insights & intelligence resulting in the identification of industry white spaces. It enhances operational efficiency through process improvements based on relevant and fact-based data. It is able to enrich human capital through workforce analysis resulting in more effective human capital management. It is able to mitigate risks by identifying areas of regulatory and company policy non-compliance before actual damage is done. AI led re-engineering approach unleashes the potential of an organization by putting the facts and the reality into the hands of the decision makers.
(AIQRATE, A bespoke global AI advisory and consulting firm. A first in its genre, AIQRATE provides strategic AI advisory services and consulting offerings across multiple business segments to enable clients on their AI powered transformation & innovation journey and accentuate their decision making and business performance.
AIQRATE works closely with Boards, CXOs and Senior leaders advising them on navigating their Analytics to AI journey with the art of possible or making them jumpstart to AI rhythm with AI@scale approach followed by consulting them on embedding AI as core to business strategy within business functions and augmenting the decision-making process with AI. We have proven bespoke AI advisory services to enable CXO’s and Senior Leaders to curate & design building blocks of AI strategy, embed AI@scale interventions and create AI powered organizations.
AIQRATE’s path breaking 50+ AI consulting frameworks, assessments, primers, toolkits and playbooks enable Indian & global enterprises, GCCs, Startups, SMBs, VC/PE firms, and Academic Institutions enhance business performance and accelerate decision making.
AIQRATE also consults with Consulting firms , Technology service providers , Pure play AI firms , Technology behemoths & Platform enterprises on curating differentiated & bespoke AI capabilities & offerings , market development scenarios & GTM approaches
Visit www.aiqrate.ai to experience our AI advisory services & consulting offerings)
Related Posts
AIQRATIONS
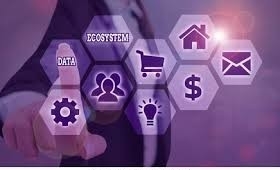
Data Driven Enterprise – Part II: Building an operative data ecosystems strategy
Add Your Heading Text Here
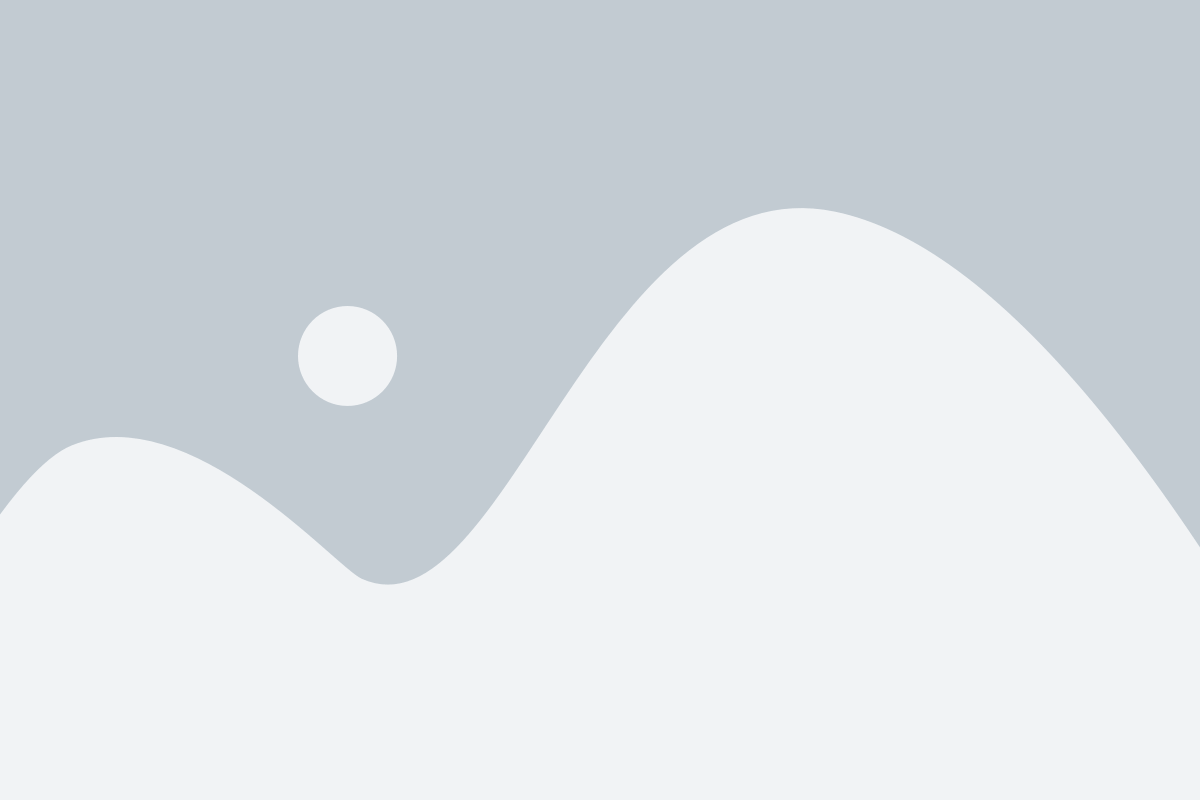
Ecosystems—interconnected sets of services in a single integrated experience—have emerged across a range of industries, from financial services to retail to healthcare. Ecosystems are not limited to a single sector; indeed, many transcend multiple sectors. For traditional incumbents, ecosystems can provide a golden opportunity to increase their influence and fend off potential disruption by faster-moving digital attackers. For example, banks are at risk of losing half of their margins to fintechs, but they have the opportunity to increase margins by a similar amount by orchestrating an ecosystem.
In my experience, many ecosystems focus on the provision of data: exchange, availability, and analysis. Incumbents seeking to excel in these areas must develop the proper data strategy, business model, and architecture.
What is a data ecosystem?
Simply put, a data ecosystem is a platform that combines data from numerous providers and builds value through the usage of processed data. A successful ecosystem balances two priorities:
Building economies of scale by attracting participants through lower barriers to entry. In addition, the ecosystem must generate clear customer benefits and dependencies beyond the core product to establish high exit barriers over the long term.Cultivating a collaboration network that motivates a large number of parties with similar interests (such as app developers) to join forces and pursue similar objectives. One of the key benefits of the ecosystem comes from the participation of multiple categories of players (such as app developers and app users).
What are the data-ecosystem archetypes?
As data ecosystems have evolved, five archetypes have emerged. They vary based on the model for data aggregation, the types of services offered, and the engagement methods of other participants in the ecosystem.
- Data utilities. By aggregating data sets, data utilities provide value-adding tools and services to other businesses. The category includes credit bureaus, consumer-insights firms, and insurance-claim platforms.
- Operations optimization and efficiency centers of excellence. This archetype vertically integrates data within the business and the wider value chain to achieve operational efficiencies. An example is an ecosystem that integrates data from entities across a supply chain to offer greater transparency and management capabilities.
- End-to-end cross-sectorial platforms. By integrating multiple partner activities and data, this archetype provides an end-to-end service to the customers or business through a single platform. Car reselling, testing platforms, and partnership networks with a shared loyalty program exemplify this archetype.
- Marketplace platforms. These platforms offer products and services as a conduit between suppliers and consumers or businesses. Amazon and Alibaba are leading examples.
- B2B infrastructure (platform as a business). This archetype builds a core infrastructure and tech platform on which other companies establish their ecosystem business. Examples of such businesses are data-management platforms and payment-infrastructure providers.
The ingredients for a successful data ecosystem : Data ecosystems have the potential to generate significant value. However, the entry barriers to establishing an ecosystem are typically high, so companies must understand the landscape and potential obstacles. Typically, the hardest pieces to figure out are finding the best business model to generate revenues for the orchestrator and ensuring participation.
If the market already has a large, established player, companies may find it difficult to stake out a position. To choose the right partners, executives need to pinpoint the value they can offer and then select collaborators who complement and support their strategic ambitions. Similarly, companies should look to create a unique value proposition and excellent customer experience to attract both end customers and other collaborators. Working with third parties often requires additional resources, such as negotiating teams supported by legal specialists to negotiate and structure the collaboration with potential partners. Ideally, partnerships should be mutually beneficial arrangements between the ecosystem leader and other participants.
As companies look to enable data pooling and the benefits it can generate, they must be aware of laws regarding competition. Companies that agree to share access to data, technology, and collection methods restrict access for other companies, which could raise anti-competition concerns. Executives must also ensure that they address privacy concerns, which can differ by geography.
Other capabilities and resources are needed to create and build an ecosystem. For example, to find and recruit specialists and tech talent, organizations must create career opportunities and a welcoming environment. Significant investments will also be needed to cover the costs of data-migration projects and ecosystem maintenance.
Ensuring ecosystem participants have access to data
Once a company selects its data-ecosystem archetype, executives should then focus on setting up the right infrastructure to supports its operation. An ecosystem can’t deliver on its promise to participants without ensuring access to data, and that critical element relies on the design of the data architecture. We have identified five questions that incumbents must resolve when setting up their data ecosystem.
How do we exchange data among partners in the ecosystem?
Industry experience shows that standard data-exchange mechanisms among partners, such as cookie handshakes, for example, can be effective. The data exchange typically follows three steps: establishing a secure connection, exchanging data through browsers and clients, and storing results centrally when necessary.
How do we manage identity and access?
Companies can pursue two strategies to select and implement an identity-management system. The more common approach is to centralize identity management through solutions such as Okta, OpenID, or Ping. An emerging approach is to decentralize and federate identity management—for example, by using blockchain ledger mechanisms.
How can we define data domains and storage?
Traditionally, an ecosystem orchestrator would centralize data within each domain. More recent trends in data-asset management favor an open data-mesh architecture . Data mesh challenges conventional centralization of data ownership within one party by using existing definitions and domain assets within each party based on each use case or product. Certain use cases may still require centralized domain definitions with central storage. In addition, global data-governance standards must be defined to ensure interoperability of data assets.
How do we manage access to non-local data assets, and how can we possibly consolidate?
Most use cases can be implemented with periodic data loads through application programming interfaces (APIs). This approach results in a majority of use cases having decentralized data storage. Pursuing this environment requires two enablers: a central API catalog that defines all APIs available to ensure consistency of approach, and strong group governance for data sharing.
How do we scale the ecosystem, given its heterogeneous and loosely coupled nature?
Enabling rapid and decentralized access to data or data outputs is the key to scaling the ecosystem. This objective can be achieved by having robust governance to ensure that all participants of the ecosystem do the following:
- Make their data assets discoverable, addressable, versioned, and trustworthy in terms of accuracy
- Use self-describing semantics and open standards for data exchange
- Support secure exchanges while allowing access at a granular level
The success of a data-ecosystem strategy depends on data availability and digitization, API readiness to enable integration, data privacy and compliance—for example, General Data Protection Regulation (GDPR)—and user access in a distributed setup. This range of attributes requires companies to design their data architecture to check all these boxes.
As incumbents consider establishing data ecosystems, we recommend they develop a road map that specifically addresses the common challenges. They should then look to define their architecture to ensure that the benefits to participants and themselves come to fruition. The good news is that the data-architecture requirements for ecosystems are not complex. The priority components are identity and access management, a minimum set of tools to manage data and analytics, and central data storage.Truly mentioning , Developing an operative data ecosystem strategy is far more difficult than getting the tech requirements right.
Related Posts
AIQRATIONS
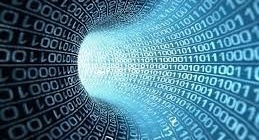
Data Driven Enterprise – Part I: Building an effective Data Strategy for competitive edge
Add Your Heading Text Here
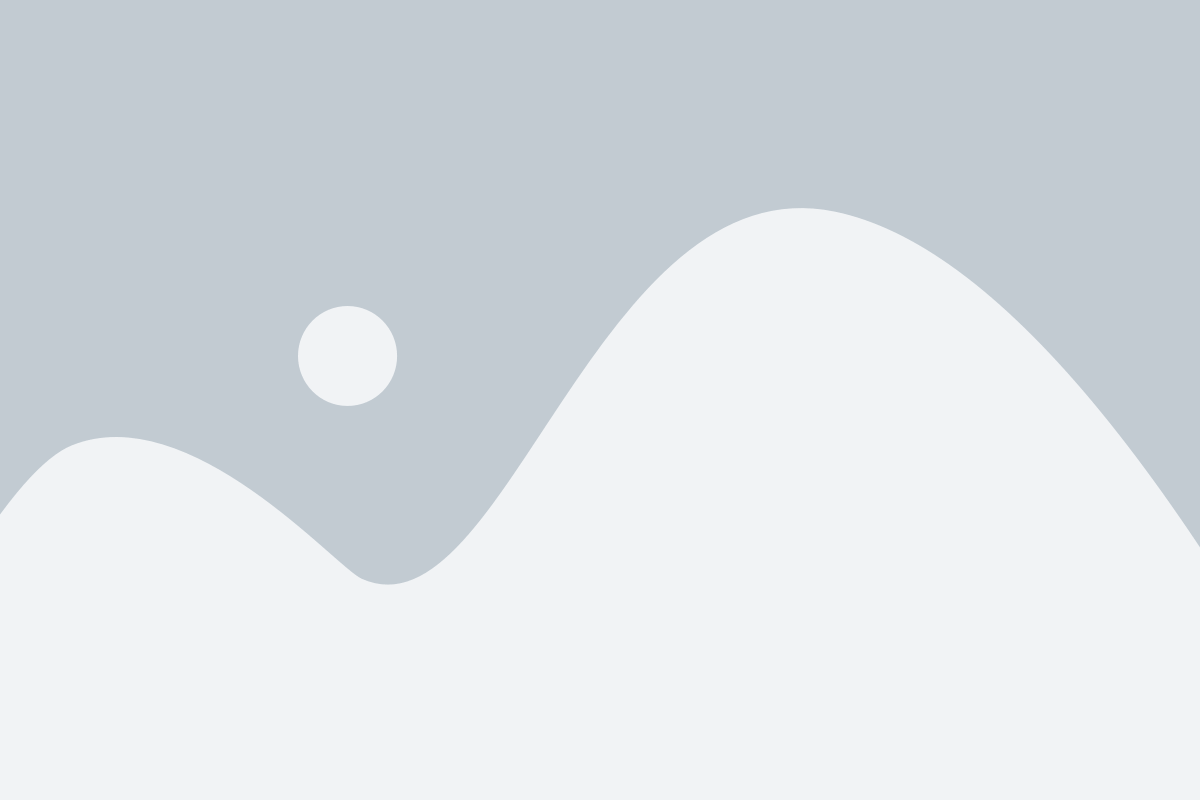
Few Enterprises take full advantage of data generated outside their walls. A well-structured data strategy for using external data can provide a competitive edge. Many enterprises have made great strides in collecting and utilizing data from their own activities. So far, though, comparatively few have realized the full potential of linking internal data with data provided by third parties, vendors, or public data sources. Overlooking such external data is a missed opportunity. Organizations that stay abreast of the expanding external-data ecosystem and successfully integrate a broad spectrum of external data into their operations can outperform other companies by unlocking improvements in growth, productivity, and risk management.
The COVID-19 crisis provides an example of just how relevant external data can be. In a few short months, consumer purchasing habits, activities, and digital behavior changed dramatically, making preexisting consumer research, forecasts, and predictive models obsolete. Moreover, as organizations scrambled to understand these changing patterns, they discovered little of use in their internal data. Meanwhile, a wealth of external data could—and still can—help organizations plan and respond at a granular level. Although external-data sources offer immense potential, they also present several practical challenges. To start, simply gaining a basic understanding of what’s available requires considerable effort, given that the external-data environment is fragmented and expanding quickly. Thousands of data products can be obtained through a multitude of channels—including data brokers, data aggregators, and analytics platforms—and the number grows every day. Analyzing the quality and economic value of data products also can be difficult. Moreover, efficient usage and operationalization of external data may require updates to the organization’s existing data environment, including changes to systems and infrastructure. Companies also need to remain cognizant of privacy concerns and consumer scrutiny when they use some types of external data.
These challenges are considerable but surmountable. This blog series discusses the benefits of tapping external-data sources, illustrated through a variety of examples, and lays out best practices for getting started. These include establishing an external-data strategy team and developing relationships with data brokers and marketplace partners. Company leaders, such as the executive sponsor of a data effort and a chief data and analytics officer, and their data-focused teams should also learn how to rigorously evaluate and test external data before using and operationalizing the data at scale.
External-data success stories: Companies across industries have begun successfully using external data from a variety of sources . The investment community is a pioneer in this space. To predict outcomes and generate investment returns, analysts and data scientists in investment firms have gathered “alternative data” from a variety of licensed and public data sources, many of which draw from the “digital exhaust” of a growing number of technology companies and the public web. Investment firms have established teams that assess hundreds of these data sources and providers and then test their effectiveness in investment decisions.
A broad range of data sources are used, and these inform investment decisions in a variety of ways:
- Investors actively gather job postings, company reviews posted by employees, employee-turnover data from professional networking and career websites, and patent filings to understand company strategy and predict financial performance and organizational growth.
- Analysts use aggregated transaction data from card processors and digital-receipt data to understand the volume of purchases by consumers, both online and offline, and to identify which products are increasing in share. This gives them a better understanding of whether traffic is declining or growing, as well as insights into cross-shopping behaviors.
- Investors study app downloads and digital activity to understand how consumer preferences are changing and how effective an organization’s digital strategy is relative to that of its peers. For instance, app downloads, activity, and rating data can provide a window into the success rates of the myriad of live-streaming exercise offerings that have become available over the last year.
Corporations have also started to explore how they can derive more value from external data . For example, a large insurer transformed its core processes, including underwriting, by expanding its use of external-data sources from a handful to more than 40 in the span of two years. The effort involved was considerable; it required prioritization from senior leadership, dedicated resources, and a systematic approach to testing and applying new data sources. The hard work paid off, increasing the predictive power of core models by more than 20 percent and dramatically reducing application complexity by allowing the insurer to eliminate many of the questions it typically included on customer applications.
Three steps to creating value with external data:
Use of external data has the potential to be game changing across a variety of business functions and sectors. The journey toward successfully using external data has three key steps.
1. Establish a dedicated team for external-data sourcing
To get started, organizations should establish a dedicated data-sourcing team. Per our understanding at AIQRATE , a key role on this team is a dedicated data scout or strategist who partners with the data-analytics team and business functions to identify operational, cost, and growth improvements that could be powered by external data. This person also would be responsible for building excitement around what can be made possible through the use of external data, planning the use cases to focus on, identifying and prioritizing data sources for investigation, and measuring the value generated through use of external data. Ideal candidates for this role are individuals who have served as analytics translators and who have experience in deploying analytics use cases and in working with technology, business, and analytics profiles.
The other team members, who should be drawn from across functions, would include purchasing experts, data engineers, data scientists and analysts, technology experts, and data-review-board members . These team members typically spend only part of their time supporting the data-sourcing effort. For example, the data analysts and data scientists may already be supporting data cleaning and modeling for a specific use case and help the sourcing work stream by applying the external data to assess its value. The purchasing expert, already well versed in managing contracts, will build specialization on data-specific licensing approaches to support those efforts.
Throughout the process of finding and using external data, companies must keep in mind privacy concerns and consumer scrutiny, making data-review roles essential peripheral team members. Data reviewers, who typically include legal, risk, and business leaders, should thoroughly vet new consumer data sets—for example, financial transactions, employment data, and cell-phone data indicating when and where people have entered retail locations. The vetting process should ensure that all data were collected with appropriate permissions and will be used in a way that abides by relevant data-privacy laws and passes muster with consumer.This team will need a budget to procure small exploratory data sets, establish relationships with data marketplaces (such as by purchasing trial licenses), and pay for technology requirements (such as expanded data storage).
2. Develop relationships with data marketplaces and aggregators
While online searches may appear to be an easy way for data-sourcing teams to find individual data sets, that approach is not necessarily the most effective. It generally leads to a series of time-consuming vendor-by-vendor discussions and negotiations. The process of developing relationships with a vendor, procuring sample data, and negotiating trial agreements often takes months. A more effective strategy involves using data-marketplace and -aggregation platforms that specialize in building relationships with hundreds of data sources, often in specific data domains—for example, consumer, real-estate, government, or company data. These relationships can give organizations ready access to the broader data ecosystem through an intuitive search-oriented platform, allowing organizations to rapidly test dozens or even hundreds of data sets under the auspices of a single contract and negotiation. Since these external-data distributors have already profiled many data sources, they can be valuable thought partners and can often save an external-data team significant time. When needed, these data distributors can also help identify valuable data products and act as the broker to procure the data.
Once the team has identified a potential data set, the team’s data engineers should work directly with business stakeholders and data scientists to evaluate the data and determine the degree to which the data will improve business outcomes. To do so, data teams establish evaluation criteria, assessing data across a variety of factors to determine whether the data set has the necessary characteristics for delivering valuable insights . Data assessments should include an examination of quality indicators, such as fill rates, coverage, bias, and profiling metrics, within the context of the use case. For example, a transaction data provider may claim to have hundreds of millions of transactions that help illuminate consumer trends. However, if the data include only transactions made by millennial consumers, the data set will not be useful to a company seeking to understand broader, generation-agnostic consumer trends.
3. Prepare the data architecture for new external-data streams
Generating a positive return on investment from external data calls for up-front planning, a flexible data architecture, and ongoing quality-assurance testing.Up-front planning starts with an assessment of the existing data environment to determine how it can support ingestion, storage, integration, governance, and use of the data. The assessment covers issues such as how frequently the data come in, the amount of data, how data must be secured, and how external data will be integrated with internal data. This will provide insights about any necessary modifications to the data architecture.
Modifications should be designed to ensure that the data architecture is flexible enough to support the integration of a continuous “conveyor belt” of incoming data from a variety of data sources—for example, by enabling application-programming-interface (API) calls from external sources along with entity-resolution capabilities to intelligently link the external data to internal data. In other cases, it may require tooling to support large-scale data ingestion, querying, and analysis. Data architecture and underlying systems can be updated over time as needs mature and evolve.The final process in this step is ensuring an appropriate and consistent level of quality by constantly monitoring the data used. This involves examining data regularly against the established quality framework to identify whether the source data have changed and to understand the drivers of any changes (for example, schema updates, expansion of data products, change in underlying data sources). If the changes are significant, algorithmic models leveraging the data may need to be retrained or even rebuilt.
Minimizing risk and creating value with external data will require a unique mix of creative problem solving, organizational capability building, and laser-focused execution. That said, business leaders who demonstrate the achievements possible with external data can capture the imagination of the broader leadership team and build excitement for scaling beyond early pilots and tests. An effective route is to begin with a small team that is focused on using external data to solve a well-defined problem and then use that success to generate momentum for expanding external-data efforts across the organization.
Related Posts
AIQRATIONS
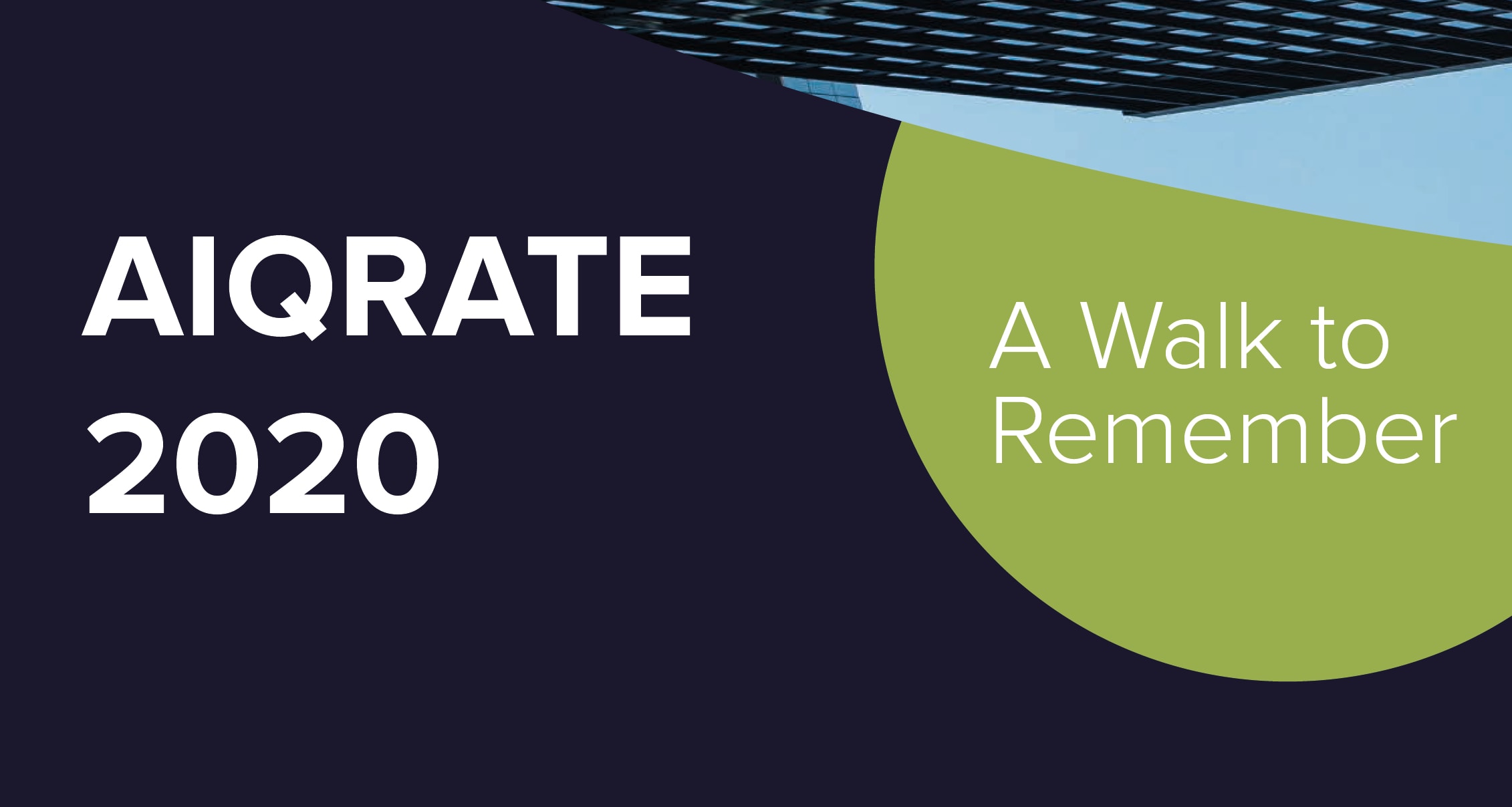
AIQRATE in 2020 ….A walk to remember
Add Your Heading Text Here
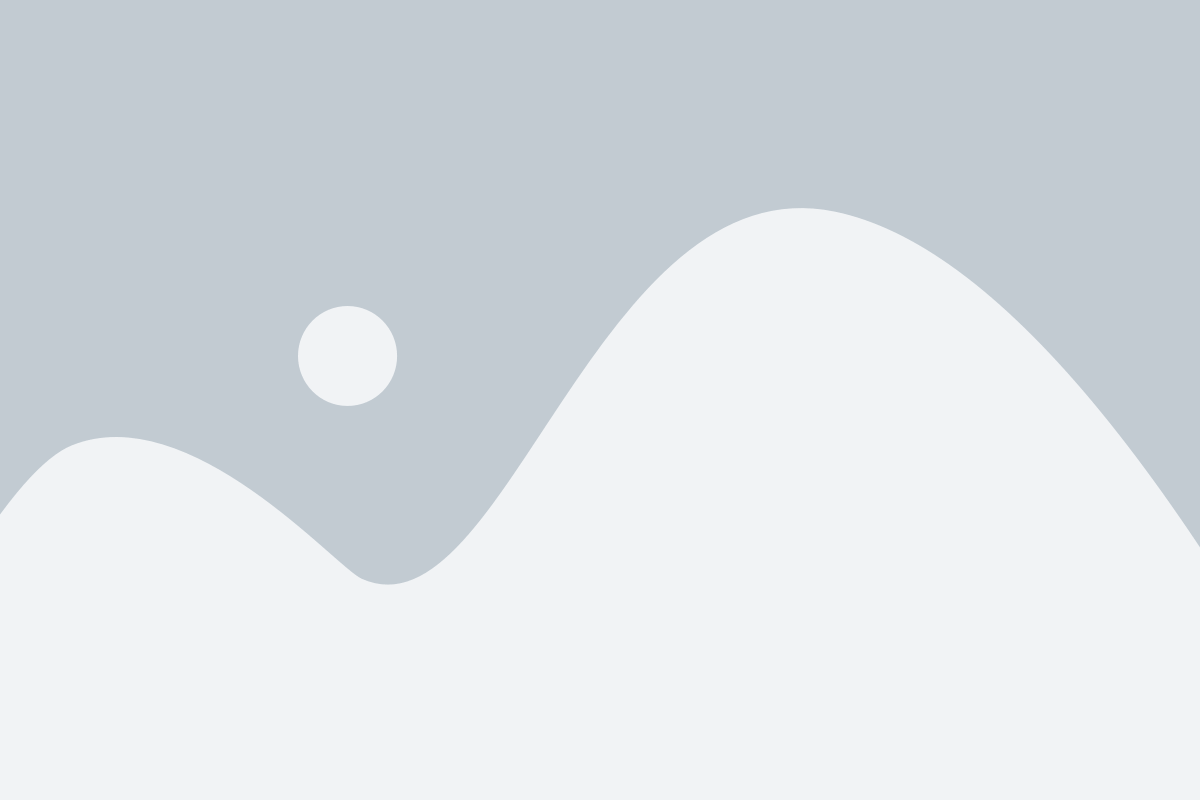
“Enabling clients reimagine their decision making & accentuate the business performance with AI strategy in a transformation, innovation and disruption driven world”
In today’s fast paced & volatile VUCA world, leaders face unprecedented challenges. They need to navigate through volatility while staying focused on strategy, business performance and culture. Artificial Intelligence is fast becoming a game changing catalyst and a strategic differentiator and almost a panacea to solve large, complex and unresolved problems. To be an AI powered organization, leaders not only need to have a broad understanding of AI strategy, they need to know how and where to use it. AIQRATE advisory services and consulting offerings are designed to enable leaders and decision makers from Enterprises, GCCs, Cloud Providers, Technology players, Startups, SMBs, VC/PE firms, Public Institutions and Academic Institutions to become AI ready and reduce the risk associated with curating, deploying AI strategy and ensuing interventions and increase the predictability of a durable leader’s success.
In the age of the bionic enterprises, AI continues to dominate the technology & business landscape. Under the aegis of transformation, disruption and innovation, AI has several applications and impact areas which usher a new change in how we make decisions in the enterprise and personal spheres. Traditionally, human decisions are to a large extent based on intuition, gut and historical data. In the age of AI, several of our decisions will be taken by algorithms. Leveraging AI, the ability to mimic the human brain and the ensuing ability to sense, comprehend and act will significantly go up and will result in emergence of augmented intelligence in decision making. Enterprises, GCCs, SMBs, Startups and Government Institutions are attempting to harness the power of AI to change the way they do business. All these industry segments are looking at AI becoming the secret sauce behind making them gain a competitive advantage. If you have not started yet, you are already behind the competition, however large or pedigreed you might be.
So, where are you placed on your AI journey? At AIQRATE, we can guide you on your journey of understanding what AI can do for you, embedding it within your business strategy, functional areas and augmenting the decision-making process.
At AIQRATE, we are here to help you with the art of the possible with AI. Through our bespoke AI strategy frameworks, methodologies, toolkits, playbooks and assessments, we will bring seamless Transformation, Innovation and Disruption to your businesses. Leveraging our proven repository of consulting templates and artifacts, we will curate your AI strategic approach roadmap. Our advisory offerings and consulting engagements are designed in alignment with your strategic growth, vision and competitive scenarios.
We are at an inflection point where AI will revolutionize the way we do business. The paradigms of customer, products, offerings, services and competition will change dramatically; and being AI-ready will become a true differentiator. AIQRATE will be your strategic partner to help you to prepare for what’s next in order to stay relevant.
Enclosing here our journey walk through of 2020.
Wish you a great 2021!
Best,
Sameer Dhanrajani
Chief Executive Officer
AIQRATE
Bangalore , India
Related Posts
AIQRATIONS
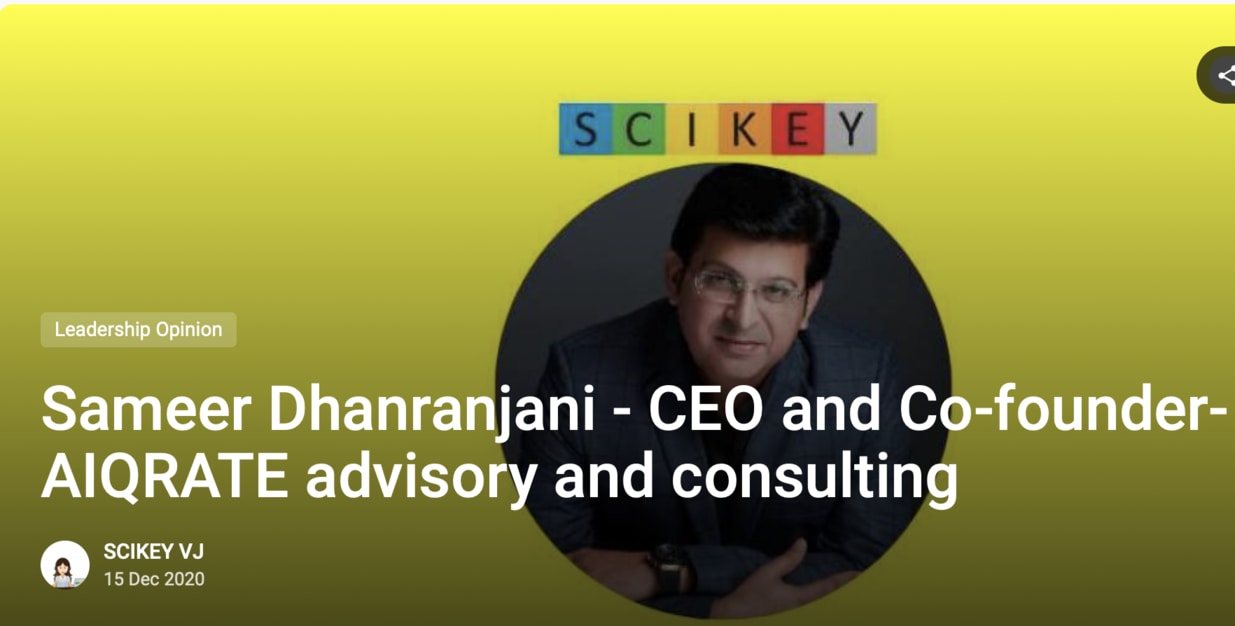
AI led Algorithms can decide on how we need to emote, behave, react, transact or interact with an individual – Sameer with SCIKEY
Add Your Heading Text Here
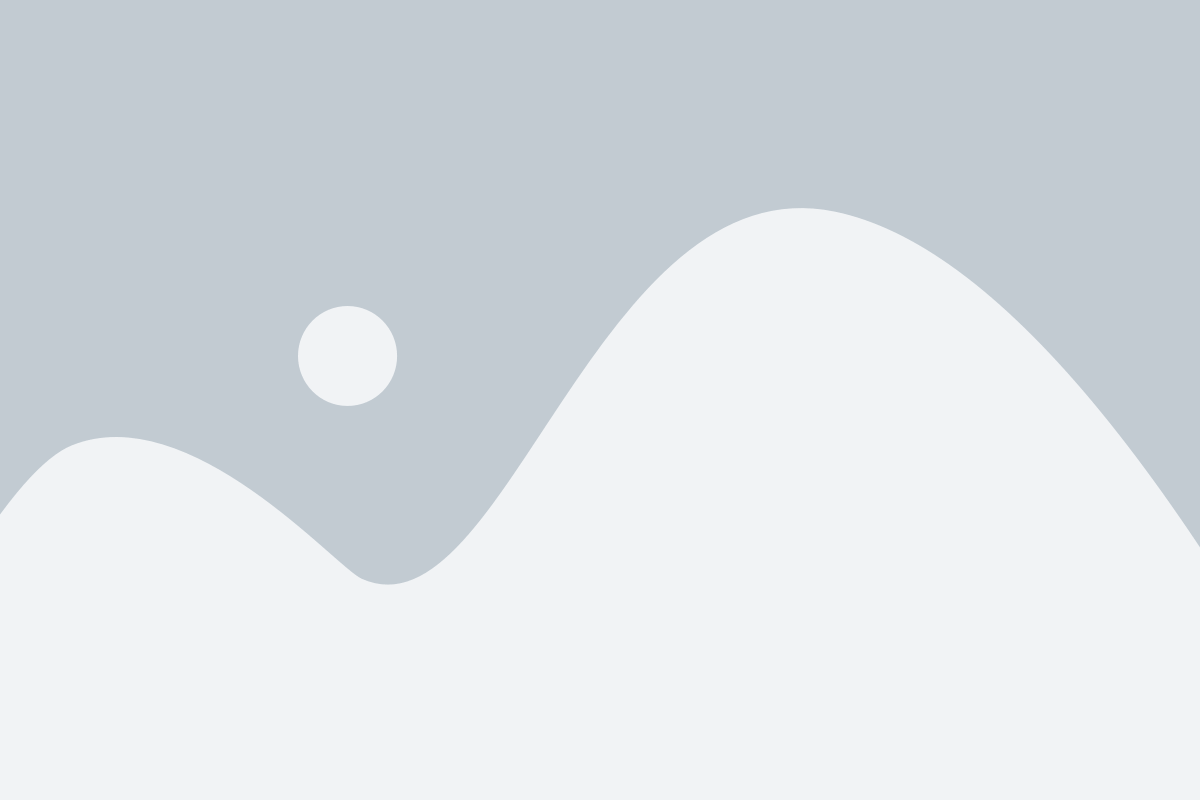
In an exclusive interaction with SCIKEY, Sameer Dhanrajani, CEO at AIQRATE Advisory & Consulting, speaks about how the future of work will look like enabled by AI, and it’s contribution in building productive teams and the emerging AI trends to watch out for in Post COVID scenario.
“AI led algorithms can decide on how we need to emote, behave, react, transact or interact with an individual,” Sameer Dhanranjani
Sameer is a globally recognized AI advisor, business builder, evangelist and thought leader known for his deep knowledge, strategic consulting approaches in AI space. Sameer has consulted with several Fortune 500 global enterprises, Indian corporations , GCCs, startups , SMBs, VC/PE firms, Academic Institutions in driving AI led strategic transformation and innovation strategies. Sameer is a renowned author, columnist, blogger and four times Tedx speaker. He is an author of bestselling book – AI and Analytics: accelerating business decisions.
In an exclusive interaction with SCIKEY, Sameer Dhanranjani, CEO at AIQRATE advisory consulting, speaks about how the future of work will look like enabled by AI, and it’s contribution in building productive teams and the emerging AI trends to watch out for in Post COVID scenario.
Mr Dhanranjani, you have consulted with several Fortune 500 enterprises, GCCs also start-ups in driving AI-led strategic transformation strategies. What according to you, are the topmost strategic considerations to weigh for managing accelerating business in Post COVID world for a start-up?
The unprecedented times of COVID-19 have brought the aspect of decision making under consideration. This includes tactical, strategic, and operational decision making that is crucial to make the venture more sustainable. Today the use of artificial intelligence is quite high amongst organizations. It can be used by start-up ventures and other outfits to make decisions irrespective of the area that needs decision making.
Most decisions that need to be made strategically are being passed on to artificial intelligence-enabled interventions. The algorithm makes similar decisions based on the previous decisions taken. Algorithms can decide how we need to emote, behave, react, transact or interact with the opposite individual This advancement in AI brings the challenge for organizations to create products and services specific to each customer through hyper-personalization and micro-segmenting. However, it can also be considered as an opportunity for organizations to emerge from the pandemic with newer business models and experiences for customers. Start-ups, especially, can make use of such advancements to reinvent and rejuvenate the organizational ecosystem.
You are known for your passion for Artificial Intelligence and are an author to the bestselling book – AI and Analytics: Accelerating Business Decisions. Tell us where how can AI be strategically significant while building productive teams.
My experience has led me to deal with engagements in the entire value chain of HR, ranging from hiring to engagement to incentivization that has leveraged using AI. It is phenomenal to see how AI can help build, engage, and sustain productive teams. AI can help in hiring through the detection emotions, facial expressions, tone modulations of the interviewee through computer vision and image classification techniques.
In the creation of productive teams, AI can gauge the engagement levels of an employee. It tries to look at the various interventions made by an employee regarding their attendance, participation in virtual meetings, and propensity to ask and engage themselves in conversations. It also keeps in check the number of pauses, intervals, and breaks taken by an employee. Every aspect of the employee is being marked to see how productive, inclusive, as an individual and in teams.
What are the top 5 AI trends to watch out for in Post COVID the scenario of the next one year?
When it comes to AI, the first trend emerging is that AI is not a tool or a technology, but it is now being touted as a strategic imperative for any organization. This means that AI strategies will become an intrinsic part and feature of every organisation.
The second trend is the democratization of AI. There is a possibility of the emergence of an AI marketplace where virtual exchanges related to business problems, demo runs etc. can be conducted. One would actually be able to figure out which algorithm is best for them in customer experience, supply chain etc.
The third trend being the cloud will act as a catalyst for AI proliferation. The propensity for cloud providers to enable AI companies with possible aspects of microservice API’s, Product Solutions will be created on the go. This means that the cloud enablers will have options to see various possibilities specific to their organisation when it comes to AI-specific use cases.
The fourth trend is linked to skilling. AI today is a part of a lot of course curriculums. But what is missing is the whole aspect of how does it get applied? The new courseware will be focused on how is AI implemented, adopted in the organization.
The last fifth trend is decision-making enabled by AI, which means humans will have no option but to upskill and reskill themselves to take a more rational, pragmatic and sanguine approach. So new models, new emerging realities of decision making will emerge.
How is AI powering the Future of Work, what are critical considerations for business and tech leaders considering the rapidly changing business dynamics due to COVID?
The future of work will be about AI and what we call AI plus a set of exponential technologies. This means that every aspect of our performance interaction and our responses will be gauged very manually through these technologies. This indicates that the level of performances in terms of how we go up-to-date needs to be worked upon. The future of work is an ecosystem where one particular employer cannot do it all.
This means that if learning must occur through an external player, it must come through the ecosystem of co-employees and the employer. In the future, we will not be caged as mere professionals doing our job but will be encouraged to push our boundaries to explore more at work. At the same time, transformation, innovation, and disruption will be a part of the future’s performance metrics. They will become a major parameter for the organization to create a mediocre versus proficient employee or a professional. This is where the onus will fall on the employees to ensure that they are not just doing what is being called out, but are going beyond to create what we call a value creation for the organisation.
About SCIKEY:
SCIKEY Market Network is a Digital Marketplace for Jobs, Work Business solutions, supported by a Professional Network and an integrated Services Ecosystem. It enables enterprises, businesses, job seekers, freelancers, and gig workers around the world. With its online events, learning certifications, assessments, ranking awards, content promotion tools, SaaS solutions for business, a global consulting ecosystem, and more, companies can get the best deals in one place.
‘SCIKEY Assured,’ a premium managed services offering by SCIKEY, delivers the best outcomes to enterprise customers globally for talent and technology solutions getting delivered offshore, remotely, or on-premise. We are super-proud to be working with some of the world’s most iconic Fortune1000 brands.
Better Work. Better Business. Better Life. Better World.
Related Posts
AIQRATIONS
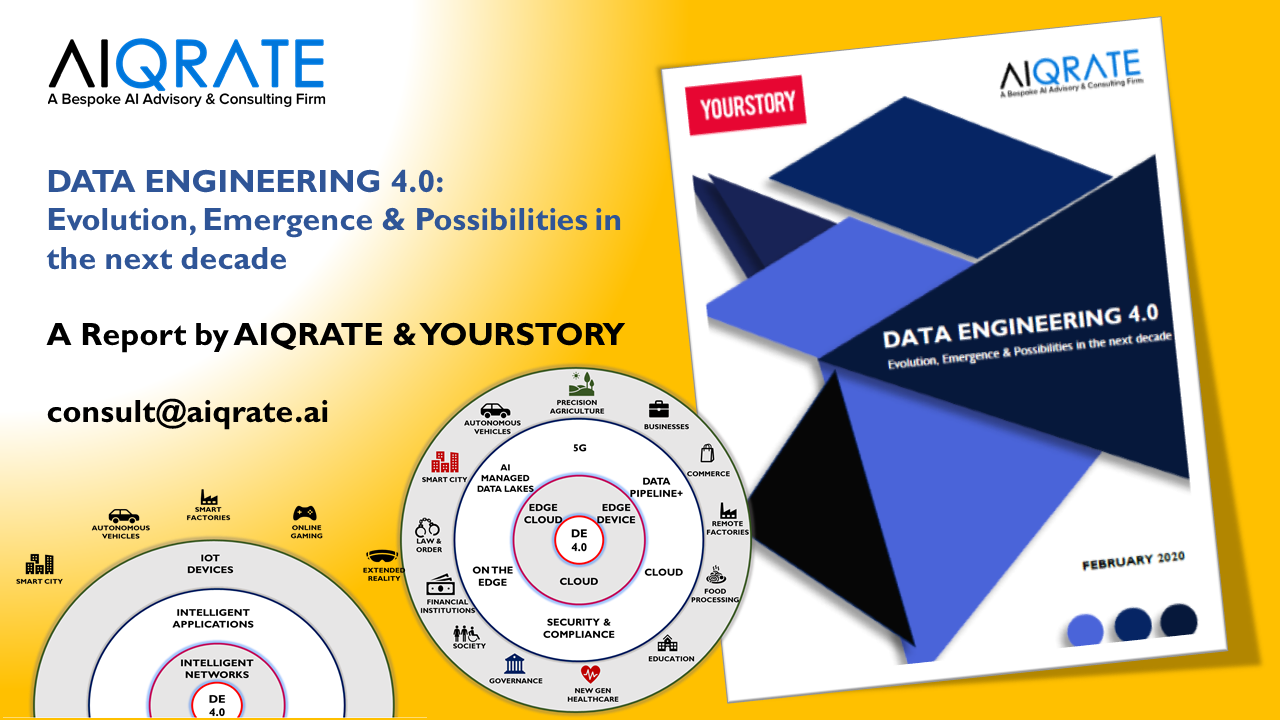
REPORT: Data Engineering 4.0: Evolution, Emergence and Possibilities in the next decade
Add Your Heading Text Here
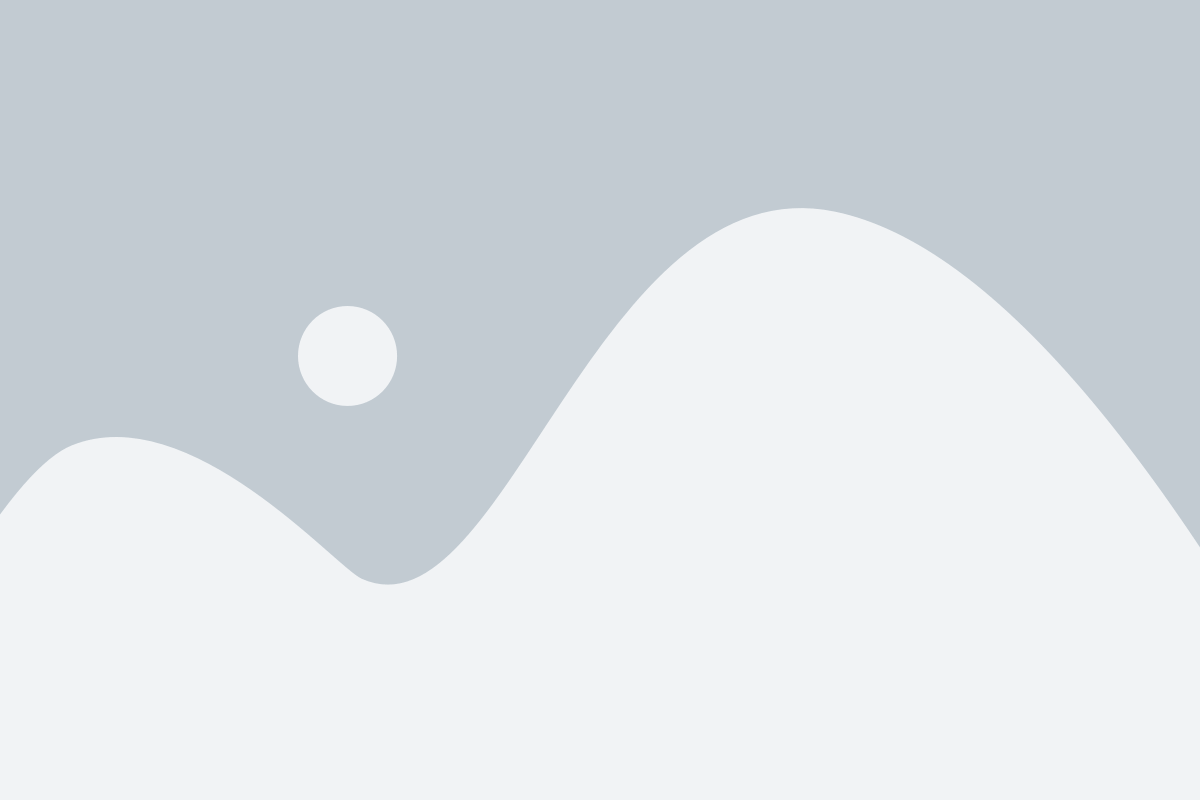
Today, most technology aficionados think of data engineering as the capabilities associated with traditional data preparation and data integration including data cleansing, data normalization and standardization, data quality, data enrichment, metadata management and data governance. But that definition of data engineering is insufficient to derive and drive new sources of society, business and operational value. The Field of Data Engineering brings together data management (data cleansing, quality, integration, enrichment, governance) and data science (machine learning, deep learning, data lakes, cloud) functions and includes standards, systems design and architectures.
There are two critical economic-based principles that will underpin the field of Data Engineering:
Principle #1: Curated data never depletes, never wears out and can be used an unlimited number of use cases at a near zero marginal cost.
Principle #2: Data assets appreciate, not depreciate, in value the more that they are used; that is, the more these data assets are used, the more accurate, more reliable, more efficient and safer they become.
There have been significant exponential technology advancements in the past few years ; data engineering is the most topical of them. Burgeoning data velocity , data trajectory , data insertion , data mediation & wrangling , data lakes & cloud security & infrastructure have revolutionized the data engineering stream. Data engineering has reinvented itself from being passive data aggregation tools from BI/DW arena to critical to business function. As unprecedented advancements are slated to occur in the next few years, there is a need for additional focus on data engineering. The foundations of AI acceleration is underpinned by robust data engineering capabilities.
YourStory & AIQRATE curated and unveiled a seminal report on “Data Engineering 4.0: Evolution , Emergence & Possibilities in the next decade.” A first in the area , the report covers a broad spectrum on key drivers of growth for Data Engineering 4.0 and highlights the incremental impact of data engineering in the time to come due to emergence of 5G , Quantum Computing & Cloud Infrastructure. The report also covers a comprehensive section on applications across industry segments of smart cities , autonomous vehicles , smart factories and the ensuing adoption of data engineering capabilities in these segments. Further , it dwells on the significance of incubating data engineering capabilities for deep tech startups for gaining competitive edge and enumerates salient examples of data driven companies in India that are leveraging data engineering prowess . The report also touches upon the data legislation and privacy aspects by proposing certain regulations and suggesting revised ones to ensure end to end protection of individual rights , security & safety of the ecosystem. Data Engineering 4.0 will be an overall trojan horse in the exponential technology landscape and much of the adoption acceleration that AI needs to drive ; will be dependent on the advancements in data engineering area.
Please fill in the below details to download the complete report.
Related Posts
AIQRATIONS
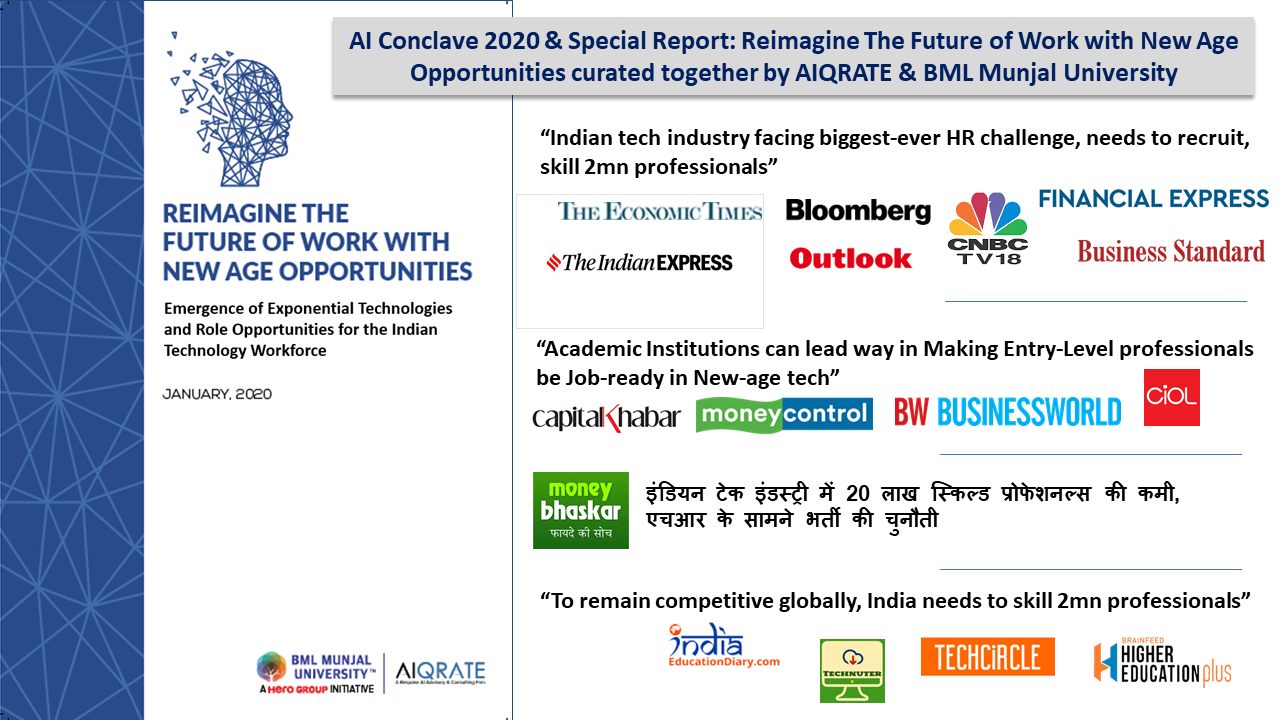
REPORT: Reimagine The Future of Work with New Age Opportunities
Add Your Heading Text Here
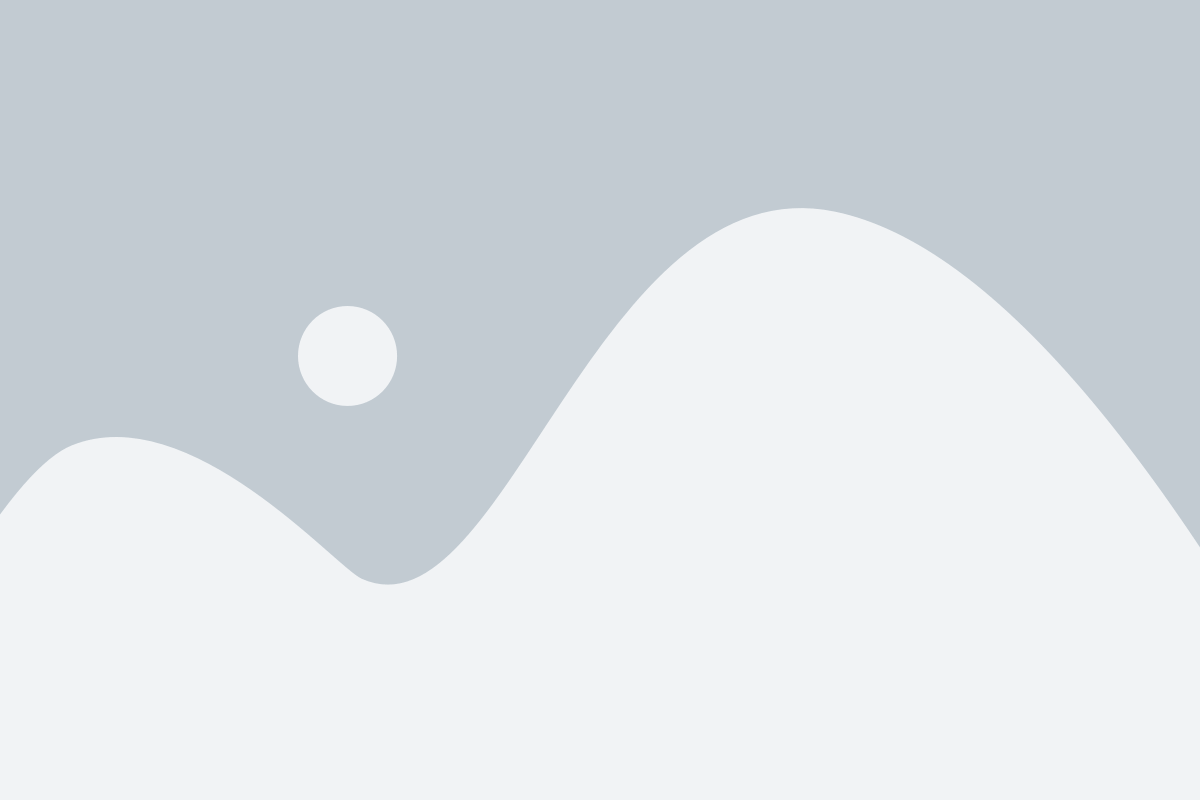
The management of talent has always been and continues to be a major challenge for most industries. This is particularly true for knowledge based industries like information technology. The dramatically changing dynamics of the Indian Technology industry compound the challenges and opportunities faced by the industry.
Never since the advent of mass production has an industry seen such dramatic volatility in such short period of time. The revolution before primarily added to the productivity of the labor and moved across the globe. The current revolution is not merely transcending national borders – it is redefining jobs, eliminating others and creating new opportunities.
Please fill in the below details to download the complete report.
Related Posts
AIQRATIONS
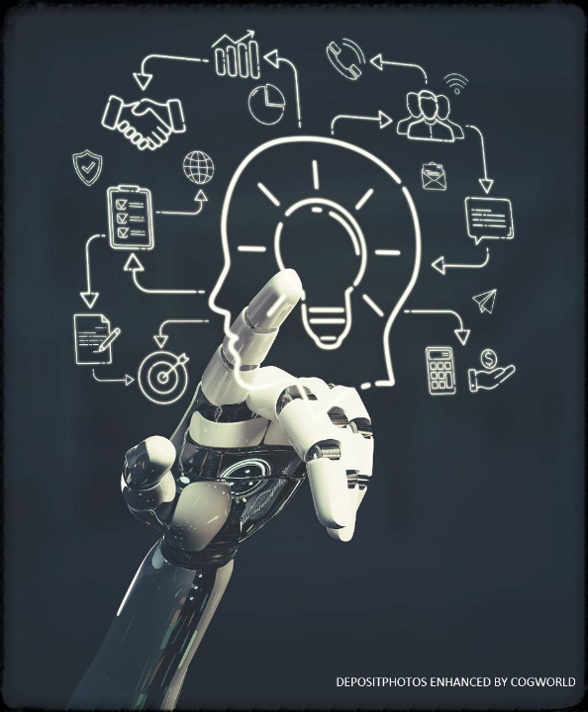
AI-Driven Disruption And Transformation: New Business Segments To Novel Market Opportunities
Add Your Heading Text Here
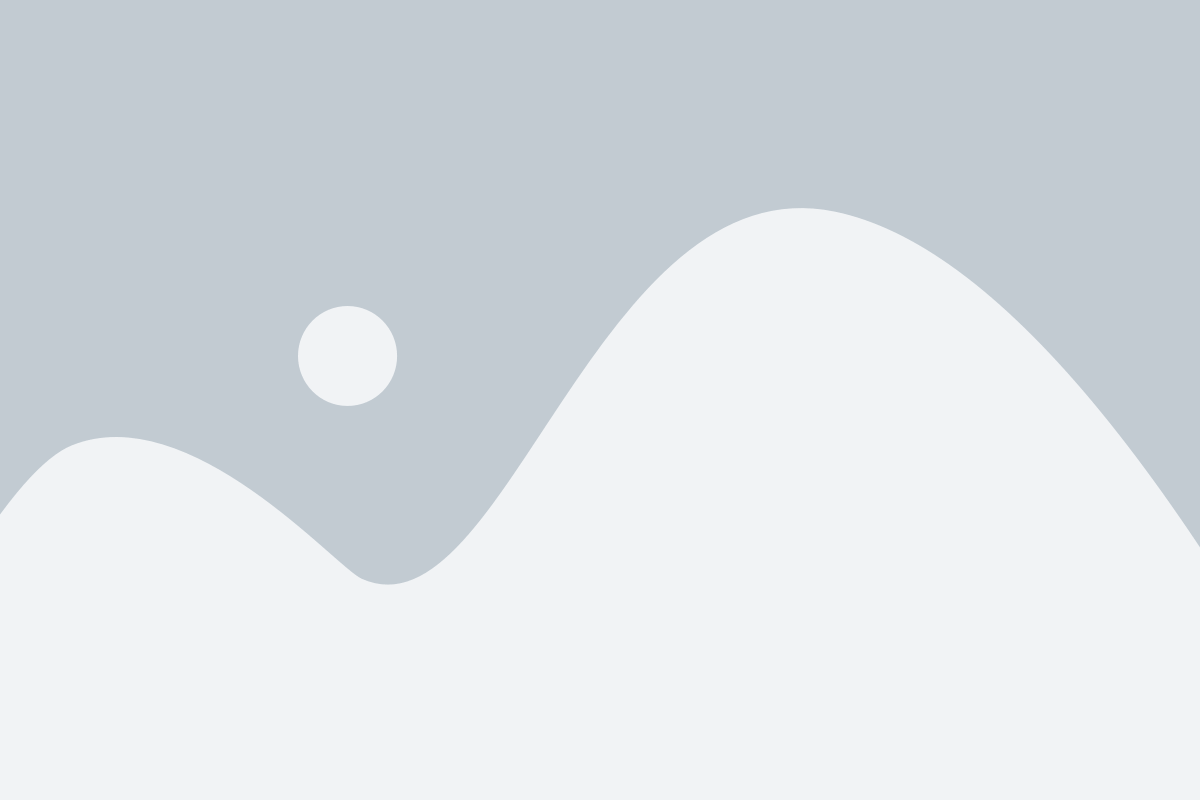
There’s little doubt that Artificial Intelligence (AI) is driving the decisive strategic elements in multiple industries, and algorithms are sitting at the core of every business model and in the enterprise DNA. Conventional wisdom, based on no small amount of research, holds that the rise of AI will usher radical, disruptive changes in the incumbent industries and sectors in the next five to 10 years.
Additionally, it’s never been a better time to launch an AI venture. Investments in AI-focused ventures have grown 1800% in just six years. The rationale behind these numbers comes, in part, from the fact that enterprises expect AI to enable them to move into new business segments, or to maintain a competitive edge in their industry.
Strategists believe this won’t come as a surprise to CXOs and decision-makers as acceleration of AI adoption and proliferation of smart, intuitive and ML algorithms spawn the creation of new industries and business segments and overall, trigger new opportunities for business monetization. However, a few questions loom large for CXOs: How will these new industries and business segments be created with AI? And, what strategic shifts can leadership make to monetize these new business opportunities?
The creation of new industries and business segments depends on dramatic advances in AI that can take a swift adoption journey to move from discovery to commercial application to a new industry. New industry segments around AI are in the making and are far from tapped. A cursory look at new age businesses: Micro-segmented, hyper-personalized online shopping platforms, GPS driven ride-sharing companies, recommendation-driven streaming channels, adaptive learning based EdTech companies, conversational AI-driven new and work scheduling are just a few of the imminent and visible examples. Yet a lot more can be done in this space.
AI adoption brings intentional efforts to adapt to this onslaught of algorithms and how it’s affecting customer and employee behavior. As algorithms become a permanent fixture in everyday life, organizations are forced to update legacy technology strategies and supporting methodologies to better reflect how the real world is evolving. And the need to do so is becoming increasingly obligatory.
On the other side, traditional and incumbent enterprises are reverse engineering investments, processes, and systems to better align with how markets are changing. Because it’s focusing on customer behavior, AI is actually in its own way, making businesses more human. As such, Artificial Intelligence is not specifically about technology, it’s empowered by it. Without an end in mind, self-learning algorithms continually seek out how to use technology in ways that improve customer experiences and relationships. It also represents an effort that introduces new models for business and, equally, creates a way of staying in business as customers become increasingly aware and selective.
Today, AI expertise is focused more on developing commercial applications that optimize efficiencies in existing industries and is focused less on developing patented algorithms that could lead to new industries. These efficiencies are accelerating the sectoral consolidation and convergence, and are less about new industry creation.
However, AI’s most potent, long-term economic use may just be to augment the discovery and pursuit of solving large, complex and unresolved problems that could be the foundations of new industry segments. Enterprises have started realizing the significance of having a long-term strategic interest and investments in using AI in this way. Yet few of the above mentioned examples are testimony to AI triggering new industry segments and business opportunities. The real winners in the algorithm-driven economy will be business leaders that align their strategies to augment AI expertise from ground zero, keep a continuous tab on blockbuster algorithms, and redefine new business segments that enable monetization of new opportunities.
AI has immense potential to jumpstart the creation of new industries and the disruption of existing ones. The curation of this as a strategic roadmap for business leaders is far from easy, but it carries great rewards for businesses. It takes a village to bring about change, and it also takes the spark and perseverance of an AI strategist to spot important trends and create a sense of urgency around new possibilities.