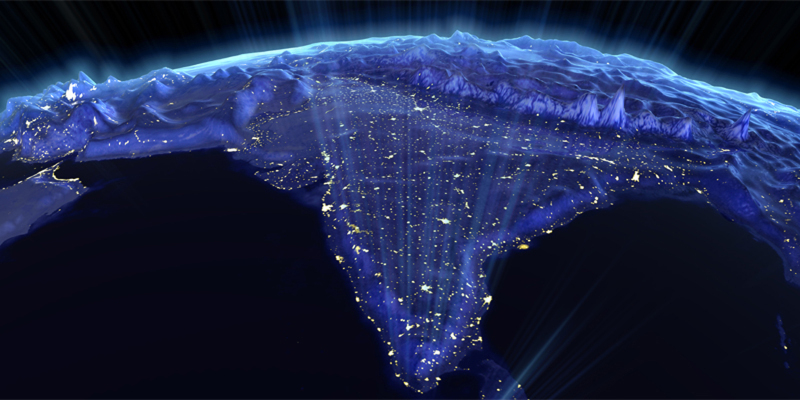
How India can Emerge as a Premier Destination for AI; Watch out China, USA…
Add Your Heading Text Here
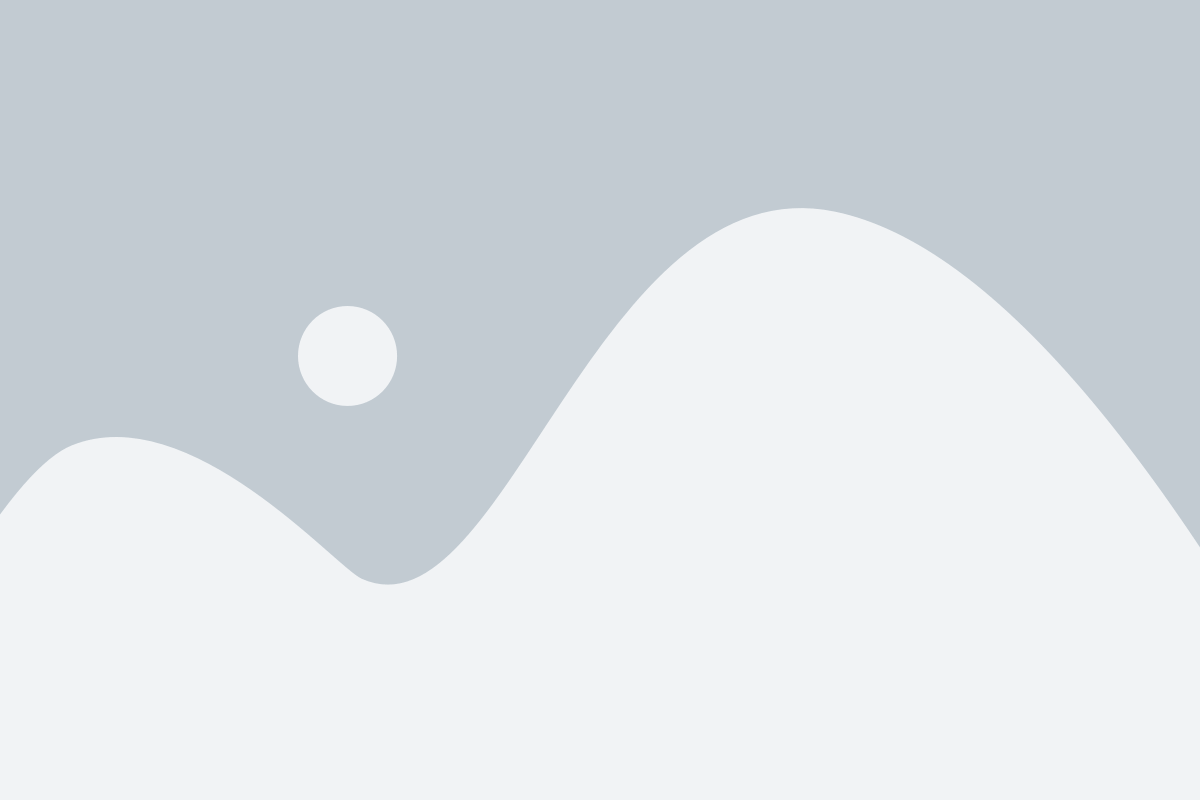
This detailed primer will provide you crucial overview of the AI’s increasing prevalence amongst Indian industry, government and peripheral ecosystem and the significant impact AI will have in your organizations to remodel strategic and business models accordingly. The ensuing details also highlights the relative comparison amongst India, China and USA on the steady progress being done in AI adoption.
VC’s, PE funds and investors attempting to understand where to target investment, what offerings and capabilities would lead to better performance and gains, and how to capitalize on AI opportunities, it’s crucial for them to understand the International economic potential of AI for now and projections in the coming years.
Cutting across all these considerations is how to build responsible AI operating models and keep it transparent enough to maintain the confidence of customers and wider stakeholders.
International AI Capitalization Report – China & NA Leads, India hot in the heels
Without doubt, AI is going to be a big game changer in the international setting. A recent PwC report concludes that AI could contribute up to $15.7 trillion to the global economy in 2030, more than the current output of China and India combined. Of this, $6.6 trillion is likely to come from increased productivity and $9.1 trillion is likely to come from consumption-side effects.
Global GDP will be up to 14% higher in 2030 as a result of the accelerating development and take-up of AI
from the standpoint of direct economic impact of AI, China and North America will have greatest gains in GDP. Even though NA will reach its peak of AI led growth faster due to huge opportunities in parallel technologies implementations and advanced customer readiness for AI. NA is supposed to reach the peak of macroeconomic gains by 2020, following which there would be a relative slowdown in the growth.
Figure 1: Souce – PwC Analysis
China, on the other hand will have a slower but stable rise in GDP gains, even post mid 2020s because a large portion of Chinese GDP comes from manufacturing, a sector which is highly susceptible to AI disruption in its operation, and also a higher rate of capital re-investment within Chinese economy compared to EU and NA. As productivity in China eventually catches up with North America, NA will focus more on importing AI-enabled products from China due to economically cheap alternative China provides. Hence by 2030, China will see much larger impact in its GDP.
Sector-wise AI Consumption Impact Index – The sector-wise impact of AI and its constituent offerings space will give crucial overview for investors to get a clear understanding of opportunities and threats in AI investment space. AI is set to be the key source of transformation, disruption and competitive advantage in today’s fast changing economy. Drawing on the findings of our AI Impact Index, we look at how quickly change is coming and where your business can expect the greatest return.
Figure 2: AI Consumption Impact (Src: PwC Analysis)
Deriving from the detailed PwC analysis report, which includes an AI impact index rating which gives an indication of enhancing quality and personalization for consumers and freeing up their time to invest their preferences in other endeavours.
The areas with the biggest potential representation will help your business target investment in the short to medium term. Some aspects could be even more disruptive or even revolutionary, such as robotic doctors, but they would be further off in the timeline of mainstream implementation.
Is the Differential for Developing countries like India too steep in catching up with AI? – AI is still at its early stages, which means that irrespective of the fact that the technology landscape is skewed towards the developed economies as compared to developing, the developing economies and their markets could still lead the developed markets from AI standpoint. This makes countries like India, the second largest economy with a strong focus in IT sector, a strong contender.
The economic impact of AI in GDP for developing countries, will be driven by:
- Productivity gains from businesses automating processes (including use of robots and autonomous vehicles).
- Productivity gains from businesses augmenting their existing labour force with AI technologies (assisted and augmented intelligence).
- Increased consumer demand resulting from the availability of personalised and/or higher-quality AI-enhanced products and services.
The consumer revolution set off by AI opens the way for massive disruption as both established businesses and new entrants drive innovation and develop new business models. A key part of the impact of AI will come from its ability to make the most of parallel developments such as IoT connectivity.
India’s Macroeconomic Landscape of AI
India is already way ahead of many other countries in implementing artificial intelligence (AI). More than half of the companies are going beyond pilot and test projects and adopting the technology at a larger scale. This statistic is largely driven by American firms such as Accenture, Microsoft, the successful implementers’ toolkit, said. Last year, India was the second-largest global site for new centres, after the US.
Well over 58% of the companies that are using AI in India are working with the technology at significant scale
The Indian government’s Digital India initiative, too, has created a favourable regulatory environment for increased use of AI.
Recipe for AI Success in India – Digital & Data Bedrock
As India undergoes rapid digital transformation, data centres and the intelligence behind the data collected will enable the government and industry to make effective decisions based on algorithms. This means increasing opportunities for using (and investing over) AI in the country.
For example, Intel is betting on Artificial Intelligence (AI) to drive demand for its electronic chips, for which it is aiming to train 15,000 scientists, developers, engineers and students on AI in India over the next one year. The company will host 60 courses under its ‘AI Developer Education Program’. These will train people on ways they can adopt AI for better research, testing or even building of products. Intel is looking at India due to the country’s large base of technical talent. The country is the third largest global site for AI companies.
As India’s largest e-commerce marketplace Flipkart closes in on completing a decade in the business, it is looking to put in use its mammoth pile of data to predict sales of products months in advance. The company is working on an artificial intelligence (AI) solution that will give it an edge over rivals by helping it make smarter decisions in ordering, distribution and pricing products on its platform. Ultimately, the AI system will allow Flipkart to boost efficiency and reduce the cost of products for customers.
While rival Amazon, which has around a 10-year headstart over Flipkart, is known to have some of the most advanced sales prediction engines, the Indian company has the advantage of having a bigger data set of the country’s online consumer market.
AI Inroads in the Private Sector
AI has now a significant impact in the day to day lives of the regular mass of the country. Now that the Indian IT sector has reached a certain intermediary peak of digitization, the focus, now , is more on automating the repetitive problems and finding more optimized, efficient or refined methods of performing the same tasks, with less time duration and lesser manpower. The result is the standardization of some very critical app based services like virtual assistants, cab aggregators, shopping recommendations etc. This will eventually lead to AI solutions to real world problems.
The AI Startups Sphere of India- Startups are clearly playing a major role in innovating faster than corporates, which has led to several curious partnerships. SAP India has invested in Niki.ai, a bot that improves the ordering experience. Then there’s Ractrack.AI, where a bot improves customer engagement and provides insights; it functions as a virtual communications assistant to convert the customer into a client. Racetrack is helping companies turn leads into meaningful engagements by using AI. Another startup, LUCEP, converts all potential queries into leads with their AI engine.
The objective is to generate insights from data and simplify customer interaction with a business and also convert them into leads.
Indian startups saw $4 billion in risk capital being deployed across 1,040 angel and VC/PE deals between January and December 2016.
Disclosed funding announcements have shown a decreased value of 55 percent from the same period last year (2015) and a decrease of 20 percent from 2014. About $9 billion in VC/PE capital had been invested in 2015. The number of deals in 2016, however, has increased by 3 percent over the last year. On an average, four startup deals were announced every weekday throughout 2016. VCs predict that going forward machine learning and AI would be key themes to invest in.
AI in Public Sector– Ripe for Digital Revamp and AI Adoption
A Blue Ocean for AI Investment due to Digital India Initiatives – Though both corporates and startups are making significant inroads in instituting AI in their service architecture and product offerings, and sometimes as part of their core business strategy itself, the challenges in the public sector in instituting AI can be quickly overcome due to huge Digital Movements instituted by the Indian Govt. like Digital India, Skill India and Make in India. This will create a solid bedrock of Data and Digital Footprint which will act as a foundational infrastructure to base AI implementation on, opening a huge blue ocean in public sector, rich for AI investment.
A New Workaround for Regulatory Challenges in Public Sector AI Implementation – One of the peculiar problems the public sector faces in mainstream implementation of AI is the fact that since AI is a continuously self-learning system, capable of analytical or creative decision making and autonomous implementation of actions, who will then be accountable in taking responsibility for its actions, should they turn out to be not so favourable. This is because of the fact that since AI has a degree of autonomous decision making, it makes it difficult to pre-meditate its consequence. The AI systems are meant to augment and enrich the life of the consumers. In such a situation, deciding liability of AI system’s actions will be difficult. Therefore, a lot of deliberation will be required to carefully come to a precise conclusion surrounding implementing these systems with ethical foundation and propriety.
Although many countries like US and some European countries are in the verge of implementing regulations and laws surrounding concepts like driverless vehicles, India still don’t have the regulations sanctioned. This, but need not be a bad news. India is cut to establish a completely revamped legal infrastructure, thereby completely circumventing the need for continuous regulatory intervention. Also, there is a favourable atmosphere in India as far as AI is concerned which will foster a spike in activities in that avenue.
Indian Governance Initiatives – Huge Scope for Investment of AI – As India emerges as a premier destination for AI, scope for investment opens in the governance aspect, in several ways. Governance schemes have a unique trait of the baggage of large volume and large scale implementation need, which can be tackled with Deep learning. For example, in Swachh Bharat Initiative, specifically construction of toilets in rural India, public servants are tasked with uploading images of these toilet constructions to a central server for assessment. Image recognition can be used to target unfinished toilets. It can also be used to identify whether the same official appears in multiple images or if photos were uploaded from a different location other than the intended place.
Other initiatives such as the Make in India, Digital India & Skill India can be augmented with AI to deal with scale. The range of application for AI techniques could range from crop insurance schemes, tax fraud detection, and detecting subsidy leakage and defence and security strategy.
An AI system can improve and enrich the agriculture of India by enhancing the bodies like The Department of Agriculture Cooperation and Farmers Welfare, Ministry of Agriculture runs the Kisan Call Centers across the country etc. It can help assist the call centre by linking various available information like soil reports from government agencies and link them to the environmental conditions. It will then provide advice on the optimal crop that can be sown in that land pocket.
As the need for large scale implementation and monitoring of governance initiative becomes more pronounced, the need for AI becomes absolute and it will open doors to considerable AI investment in the future of India.
Finally, Looking Ahead – A Collaborative Innovation Environment due to AI
AI innovations which fall under assisted, augmented and autonomous intelligence will help users understand and decide which level of intelligence is helpful and required in their context, thereby making AI Acceptance easier for the people. At the same time, this AI continuum can be used to understand economic ramifications, usage complexity and decision-making implications. While academia and the private sector conduct research on various AI problems with diverse implications in mind, the public sector with its various digital initiatives (Digital India, Make in India, etc.) can identify areas where parts of the AI continuum can be utilised to increase reach, effectiveness and efficiency, thereby giving direction to AI Innovative Research.
A collaborative innovation environment between academia and the private and public sectors will help provide holistic and proactive advisory delivery to the population, for eg. through public call centres, linking information from various government sources. At the same time, the rich data generated from these interactions can be used to draw deep conclusions. Collaboration between the three pillars could further help get a comprehensive view of problems and find intelligent and innovative ways to increase the efficiency and effectiveness of services delivered to society.
Related Posts
AIQRATIONS
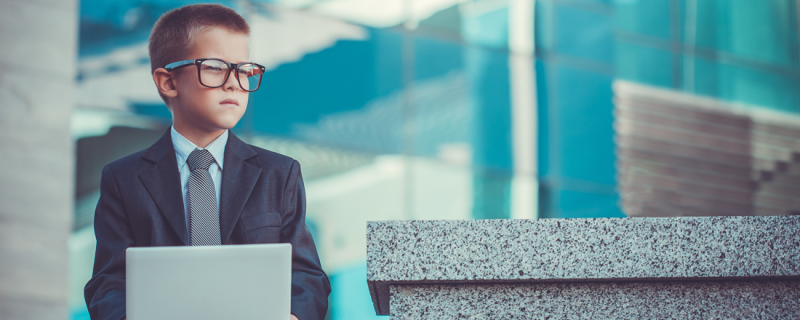
How Rise of Exponential Technologies – AI, RPA, Blockchain, Cybersecurity will Redefine Talent Demand & Supply Landscape
Add Your Heading Text Here
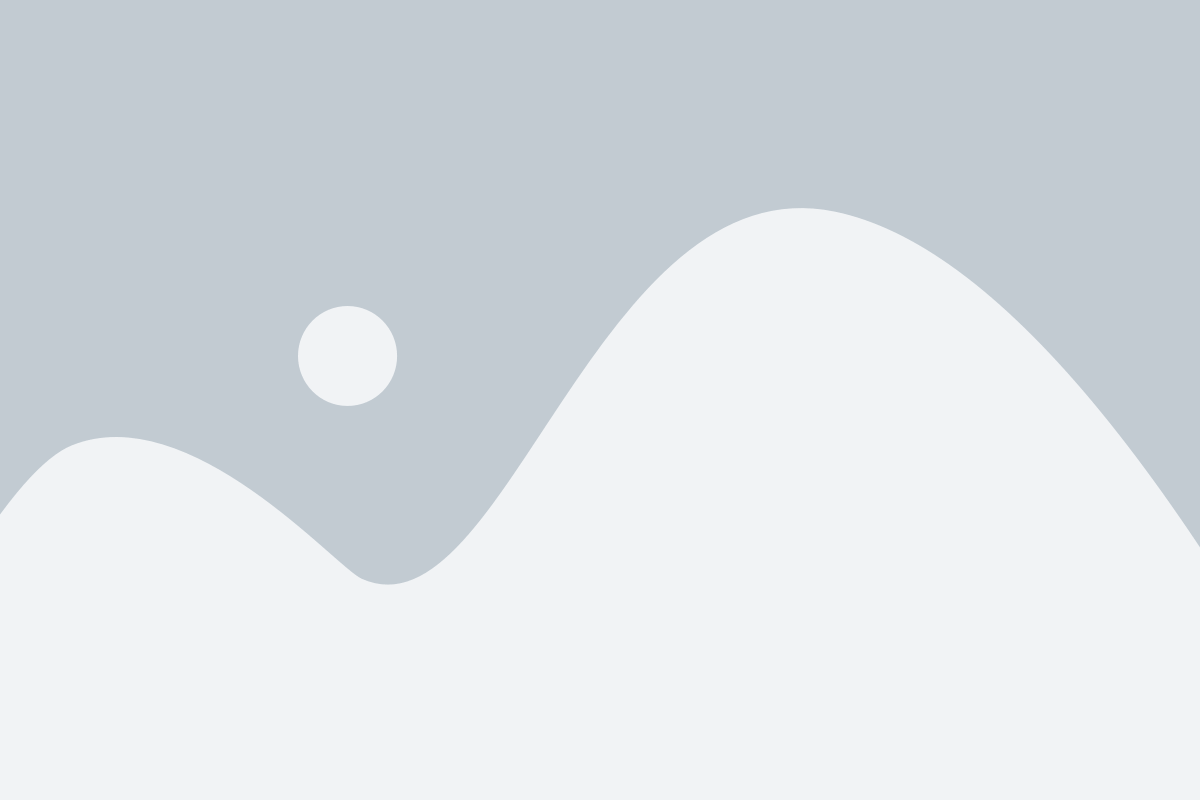
The current boom of exponential technologies of today is causing strong disruption in the talent availability landscape, with traditional, more mechanical roles being wiped out and paving way for huge demand for learning and design thinking based skills and professions. The World Economic Forum said in 2016 that 60% of children entering school today will work in jobs that do not yet exist.
While there is a risk to jobs due to these trends, the good news is that a huge number of new jobs are getting created as well in areas like AI, Machine Learning, Robotic Process Automation (RPA), Blockchain, Cybersecurity, etc. It is clearly a time of career pivot for IT professionals to make sure they are where the growth is.
AI and Machine Learning upending the traditional IT Skill Requirement
AI and Machine Learning will create a new demand for skills to guide its growth and development. These emerging areas of expertise will likely be technical or knowledge-intensive fields. In the near term, the competition for workers in these areas may change how companies focus their talent strategies.
At a time when the demand for data scientists and engineers will grow 39% by 2020, employers are seeking out leaders who can effectively work with technologists to ask the right questions and apply the insight to solve business problems. The business schools are, hence, launching more programs to equip graduates with the skills they need to succeed. Toronto’s Rotman School of Management, for example, last week launched a nine-month program which provides recent college graduates with advanced data management, analytical and communication skills.
According to the Organization of Economic Cooperation and Development, only 5-10% of labor would be displaced by intelligent automation, and new job creation will offset losses.
The future will increase the value of workers with a strong learning ability and strength in human interaction. On the other hand, today’s highly paid, experienced, and skilled knowledge workers may be at risk of losing their jobs to automation.
Many occupations that might appear to require experience and judgment — such as commodity traders — are being outdone by increasingly sophisticated machine-learning programs capable of quickly teasing subtle patterns out of large volumes of data. If your job involves distracting a patient while delivering an injection, guessing whether a crying baby wants a bottle or a diaper change, or expressing sympathy to calm an irate customer, you needn’t worry that a robot will take your job, at least for the foreseeable future.
Ironically, the best qualities for tomorrow’s worker may be the strengths usually associated with children. Learning has been at the centre of the new revival of AI. But the best learners in the universe, by far, are still human children. At first, it was thought that the quintessential preoccupations of the officially smart few, like playing chess or proving theorems — the corridas of nerd machismo — would prove to be hardest for computers. In fact, they turn out to be easy. Things every dummy can do like recognizing objects or picking them up are much harder. And it turns out to be much easier to simulate the reasoning of a highly trained adult expert than to mimic the ordinary learning of every baby. The emphasis on learning is a key change from previous decades and rounds of automation.
According to Pew Research, 47% of all employment opportunities will be occupied by machines within the next two decades.
What types of skills will be needed to fuel the development of AI over the next several years? These prospects include:
- Ethics: The only clear “new” job category is that of AI ethicist, a role that will manage the risks and liabilities associated with AI, as well as transparency requirements. Such a role might be imagined as a cross between a data scientist and a compliance officer.
- AI Training: Machine learning will require companies to invest in personnel capable of training AI models successfully, and then they must be able to manage their operations, requiring deep expertise in data science and an advanced business degree.
- Internet of Things (IoT): Strong demand is anticipated for individuals to support the emerging IoT, which will require electrical engineering, radio propagation, and network infrastructure skills at a minimum, plus specific skills related to AI and IoT.
- Data Science: Current shortages for data scientists and individuals with skills associated with human/machine parity will likely continue.
- Additional Skill Areas: Related to emerging fields of expertise are a number of specific skills, many of which overlap various fields of expertise. Examples of potentially high-demand skills include modeling, computational intelligence, machine learning, mathematics, psychology, linguistics, and neuroscience.
In addition to its effect on traditional knowledge workers and skilled positions, AI may influence another aspect of the workplace: gender diversity. Men hold 97 percent of the 2.5 million U.S. construction and carpentry jobs. These male workers stand more than a 70 percent chance of being replaced by robotic workers. By contrast, women hold 93 percent of the registered nurse positions. Their risk of obsolescence is vanishingly small: .009 percent.
RPA disrupting the traditional computing jobs significantly
RPA is not true AI. RPA uses traditional computing technology to drive its decisions and responses, but it does this on a scale large and fast enough to roughly mimic the human perspective. AI, on the other hand, applies machine and deep learning capabilities to go beyond massive computing to understand, learn, and advance its competency without human direction or intervention — a truly intelligent capability. RPA is delivering more near-term impact, but the future may be shaped by more advanced applications of true AI.
In 2016, a KPMG study estimated that 100 million global knowledge workers could be affected by robotic process automation by 2025.
The first reaction would be that in the back office and the middle office, all those roles which are currently handling repetitive tasks would become redundant. 47% of all American job functions could be automated within 20 years, according to the Oxford Martin School on Economics in a 2013 report.
Indeed, India’s IT services industry is set to lose 6.4 lakh low-skilled positions to automation by 2021, according to U.S.-based HfS Research. It said this was mainly because there were a large number of non-customer facing roles at the low-skill level in countries like India, with a significant amount of “back office” processing and IT support work likely to be automated and consolidated across a smaller number of workers.
Automation threatens 69% of the jobs in India, while it’s 77% in China, according to a World Bank research.
Job displacement would be the eventual outcome however, there would be several other situations and dimensions which need to be factored. Effective automation with the help of AI should create new roles and new opportunities hitherto not experienced. Those who currently possess traditional programming skills have to rapidly acquire new capabilities in machine learning, develop understanding of RPA and its integration with multiple systems. Unlike traditional IT applications, planning and implementation could be done in small patches in shorter span of time and therefore software developers have to reorient themselves.
For those entering into the workforce for the first time, there would be a demand for talent with traditional programming skills along with the skills for developing RPA frameworks or for customising the frameworks. For those entering the workforce for being part of the business process outsourcing functions, it would be important to develop capability in data interpretation and analysis as increasingly more recruitment at the entry level would be for such skills and not just for their communication or transaction handling skills.
Blockchain – A blue ocean of a New kind of Financial Industry Skillset
A technology as revolutionary as blockchain will undoubtedly have a major impact on the financial services landscape. Many herald blockchain for its potential to demystify the complex financial services industry, while also reducing costs, improving transparency to reduce the regulatory burden on the industry. But despite its potential role as a precursor to extend financial services to the unbanked, many fear that its effect on the industry may have more cons than pros.
30–60% of jobs could be rendered redundant by the simple fact that people are able to share data securely with a common record, using Blockchain
Industries including payments, banking, security and more will all feel the impact of the growing adoption of this technology. Jobs potentially in jeopardy include those involving tasks such as processing and reconciling transactions and verifying documentation. Profit centers that leverage financial inefficiencies will be stressed. Companies will lose their value proposition and a loss of sustainable jobs will follow. The introduction of blockchain to the finance industry is similar to the effect of robotics in manufacturing: change in the way we do things, leading to fewer jobs, is inevitable.
Nevertheless, the nature of such jobs is likely to evolve. While Blockchain creates an immutable record that is resistant to tampering, fraud may still occur at any stage in the process but will be captured in the record and there easily detected. This is where we can predict new job opportunities. There could be a whole class of professions around encryption and identity protection.
So far, the number of jobs created by the industry appears to exceed the number of available professionals qualified to fill them, but some aren’t satisfied this trend will continue. Still, the study of the potential impact of blockchain tech on jobs has been largely qualitative to date. Aite Group released a report that found the largest employers in the blockchain industry each employ about 100 people.
Related Posts
AIQRATIONS
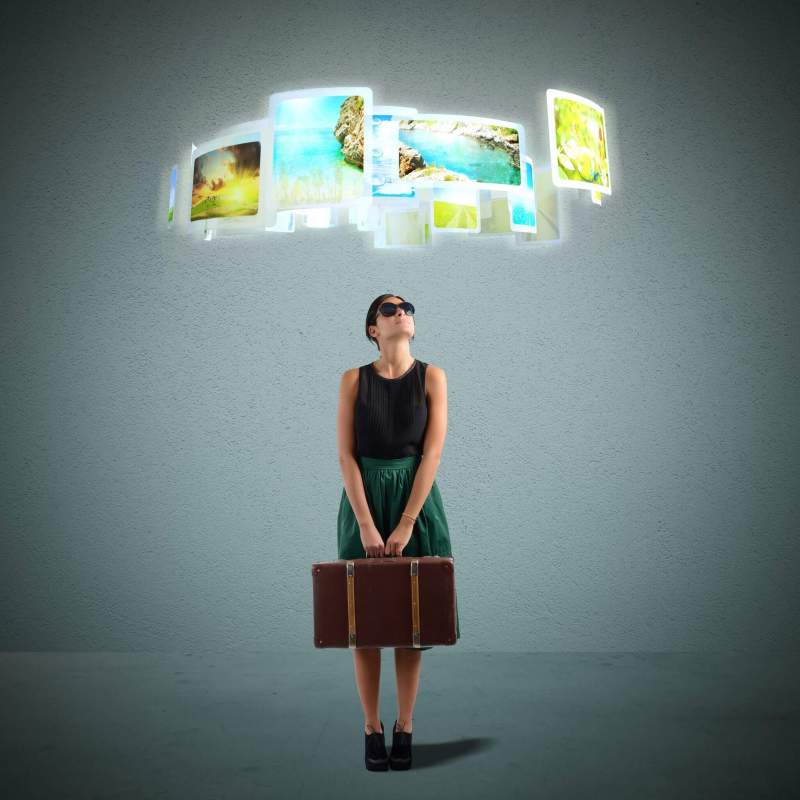
Travel & Hospitality Industry Transformation: Served Fast with AI
Add Your Heading Text Here
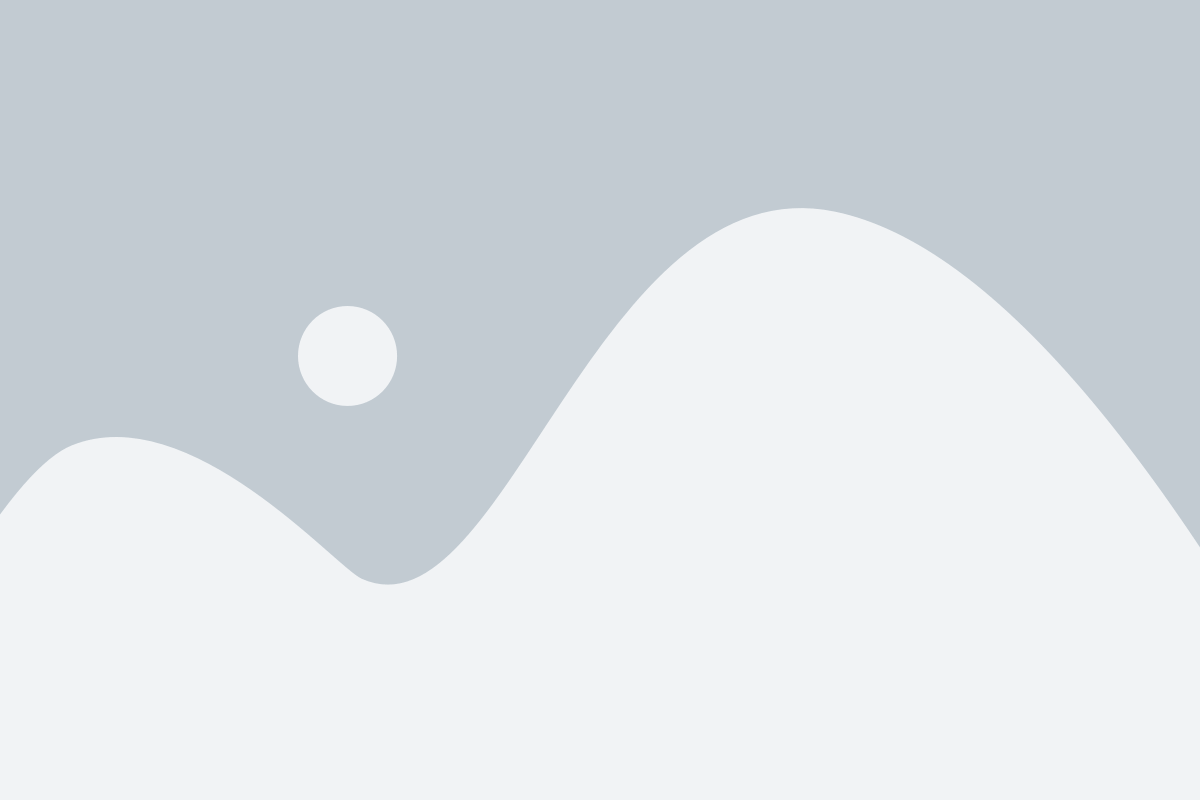
Over the years, the influence of AI has spread to almost every aspect of the travel and the hospitality industry. 30% of hospitality businesses use artificial intelligence to augment at least one of their primary sales processes and most customer personalization is done using AI.
30% of hospitality businesses use artificial intelligence to augment at least one of their primary sales processes.
The sudden popularity of Artificial Intelligence in the Travel industry can be credited to the humongous amount of data being generated today. Artificial Intelligence helps analyse unstructured data, brings value in partnership with Big Data and turns it into meaningful and actionable insights. Trends, outliers and patterns are figured out using this smart data which helps in guiding a Travel company to make informed decisions. The discounts, schemes, tour packages, seasons to target and people to target are formulated using this data. Usually, surveys and social media sensing are done to know customer’s insights and behaviour.
Let’s look at how AI has influenced each aspect of the business
Bleisure – Personalized Experience
There are always a few who are up for a new challenge and adopt to new technology. Many hotels have started using an AI concierge. One great example of an AI concierge is Hilton World wide’s Connie, who is the first true AI-powered concierge bot.
Connie stands at 2 feet high and guests can interact with it during their check-in. Connie is powered by IBM’s Watson AI and uses WayBlazer travel database. It can provide information to guests on local attractions, places to visit, etc. Being an AI, it can learn and adapt and respond to each guest.
In the Travel business, Mezi, using Artificial Intelligence and Natural Language Processing, provides a personalized experience to Business travellers who usually are strapped for time. It talks about bringing on a concept of bleisure (business+leisure) to address the needs of the workforce. A research done by them states that 84% of business travellers return feeling frustrated, burnt out and unmotivated. The kind of tedious and monotonous planning that goes into the travel booking could be the reason for it. With AI and NLP, Mezi collects preferences and generates suggestions so that a customized and streamlined experience is given and the issues faced by them are addressed properly.
Increased Productivity – Instant Connectivity
Increased productivity now begins with the search for the hotel, and technology has paved its way for the customer to access more data than ever before. Booking sites like Lola (www.lola.com) who provide on-demand travel services have developed technologies that can not only instantly connects people to their team of travel agents who find and book flights, hotels, and cars but have been able to empower their agents with tremendous technology to make research and decisions an easy process.
Intelligent Travel Assistants – Chatbots
Chatbot technology is another big strand of AI, and unsurprisingly, many travel brands have already launched their own versions in the past year or so. Skyscanner is just one example, creating a bot to help consumers find flights in Facebook Messenger. Users can also use it to request travel recommendations and random suggestions. Unlike ecommerce or retail brands using chatbots, which can appear gimmicky, there is an argument that examples like Skyscanner are much more relevant and useful for everyday consumers.
After all, with the arrival of many more travel search websites, consumers are being overwhelmed by choice – not necessarily helped by it. Consequently, a bot like Skyscanner is able to cut through the noise, connecting with consumers in their own time and in the social media spaces they most frequently visit.
Recently, Aeromexico started using Facebook Messenger chatbot to answer the very generic questions by the customers. The main idea was to cater to 80% of questions which are usually the repeated ones and about common topics. Thus, to avoid a repetitive process, artificial intelligence is of great application. Airlines hugely benefit from this. KLM Royal Dutch Airlines uses artificial intelligence to respond to the queries of customers on twitter and facebook. It uses an algorithm from a company called Digital Genius which is trained on 60,000 questions and answers. Not only this, Deutsche Lufthansa’s bot Mildred can help in searching the cheapest fares.
Discovery & Data Analysis – Intelligent Recommendations
International hotel search engine Trivago acquired Hamburg, Germany machine learning startup, Tripl, as it ramps up its product with recommendation and personalization technology, giving them a customer-centric approach.
The AI algorithm gives tailored travel recommendations by identifying trends in users’ social media activities and comparing it with in-app data of like-minded users. With its launch in July 2015, users could sign up only through Facebook, potentially sharing oodles of profile information such as friends, relationship status, hometown, and birthday.
Persona based travel recommendations, use of customised pictures and text are now gaining ground to entice travellers to book your hotels. KePSLA’s travel recommendation platform is one of the first in the world to do this by using deep learning and NLP solutions.
With 81% of people believing that robots would be better at handling data than humans, there is also a certain level of confidence in this area from consumers.
Knowing your Travellers – Deep Customer Behaviour
Dorchester Collection is another hotel chain to make use of AI. However, instead of using it to provide a front-of-house service, it has adopted it to interpret and analyse customer behaviour in the form of raw data.
Partnering with technology company, RicheyTX, Dorchester Collection has helped to develop an AI platform called Metis.
Delving into swathes of customer feedback such as surveys and reviews (which would take an inordinate amount of time to manually find and analyse) it is able to measure performance and instantly discover what really matters to guests.
For example, Metis helped Dorchester to discover that breakfast it not merely an expectation – but something guests place huge importance on. As a result, the hotels began to think about how they could enhance and personalise the breakfast experience.
Flight Fare and Hotel Price Forecasting
Flight fares and hotel prices are ever-changing and vary greatly depending on the provider. No one has time to track all those changes manually. Thus, smart tools which monitor and send out timely alerts with hot deals are currently in high demand in the travel industry.
The AltexSoft data science team has built such an innovative fare predictor tool for one of their clients, a global online travel agency, Fareboom.com. Working on its core product, a digital travel booking website, they could access and collect historical data about millions of fare searches going back several years. Armed with such information, they created a self-learning algorithm, capable of predicting the future price movements based on a number of factors, such as seasonal trends, demand growth, airlines special offers, and deals.
With the average confidence rate at 75 percent, the tool can make short-term (several days) as well as long-term (a couple of months) forecasts.
Optimized Disruption Management
While the previous case is focused mostly on planning trips and helping users navigate most common issues while traveling, automated disruption management is somewhat different. It aims at resolving actual problems a traveler might face on his/her way to a destination point.
Mostly applied to business and corporate travel, disruption management is always a time-sensitive task, requiring instant response. While the chances to get impacted by a storm or a volcano eruption are very small, the risk of a travel disruption is still quite high: there are thousands of delays and several hundreds of canceled flights every day.
With the recent advances in technology, it became possible to predict such disruptions and efficiently mitigate the loss for both the traveler and the carrier. The 4site tool, built by Cornerstone Information Systems, aims at enhancing the efficiency of enterprise travel. The product caters to travelers, travel management companies, and enterprise clients, providing a unique set of features for real-time travel disruption management.
For example, if there is a heavy snowfall at your destination point and all flights are redirected to another airport, a smart assistant can check for available hotels there or book a transfer from your actual place of arrival to your initial destination.
Not only passengers are affected by travel disruptions; airlines bear significant losses every time a flight is canceled or delayed. Thus, Amadeus, one of the leading global distribution systems (GDS), has introduced Schedule Recovery system, aiming to help airlines mitigate the risks of travel disruption. The tool helps airlines instantly address and efficiently handle any threats and disruptions in their operations.
Future potential
So, we’ve already seen the travel industry capitalise on AI to a certain extent. But how will it evolve in the coming year?
Business travel
Undoubtedly, we’ll see many more brands using AI for data analysis as well as launching their own chatbots. There’s already been a suggestion that Expedia is next in line, but it is reportedly set to focus on business travel rather than holidaymakers. Due to the greater need for structure and less of a desire for discovery, it certainly makes sense that artificial intelligence would be more suited to business travellers.
Specifically, it could help to simplify the booking process for companies, as well as help eliminate discrepancies around employee expenses. With reducing costs and improving efficiency two of the biggest benefits, AI could start to infiltrate business travel even more so than leisure in the next 12 months.
Voice technology
Lastly, we can expect to see greater development in voice-activated technology.
With voice-activated search, the experience of researching and booking travel has the potential to become quicker and easier than ever before. Similarly, as Amazon Echo and Google Home start to become commonplace, more hotels could start to experiment with speech recognition to ramp up customer service.
This means devices and bots could become the norm for brands in the travel industry.
Related Posts
AIQRATIONS
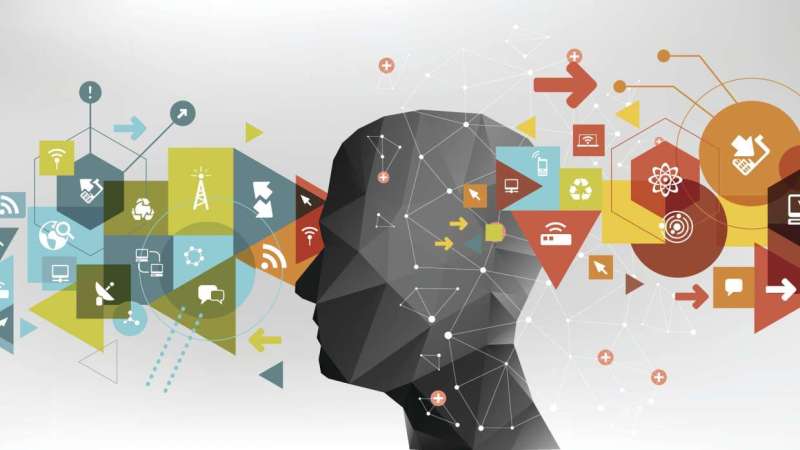
Design Thinking | Behavioural Sciences: Strategic Elements to Building a Successful AI Enterprise
Add Your Heading Text Here
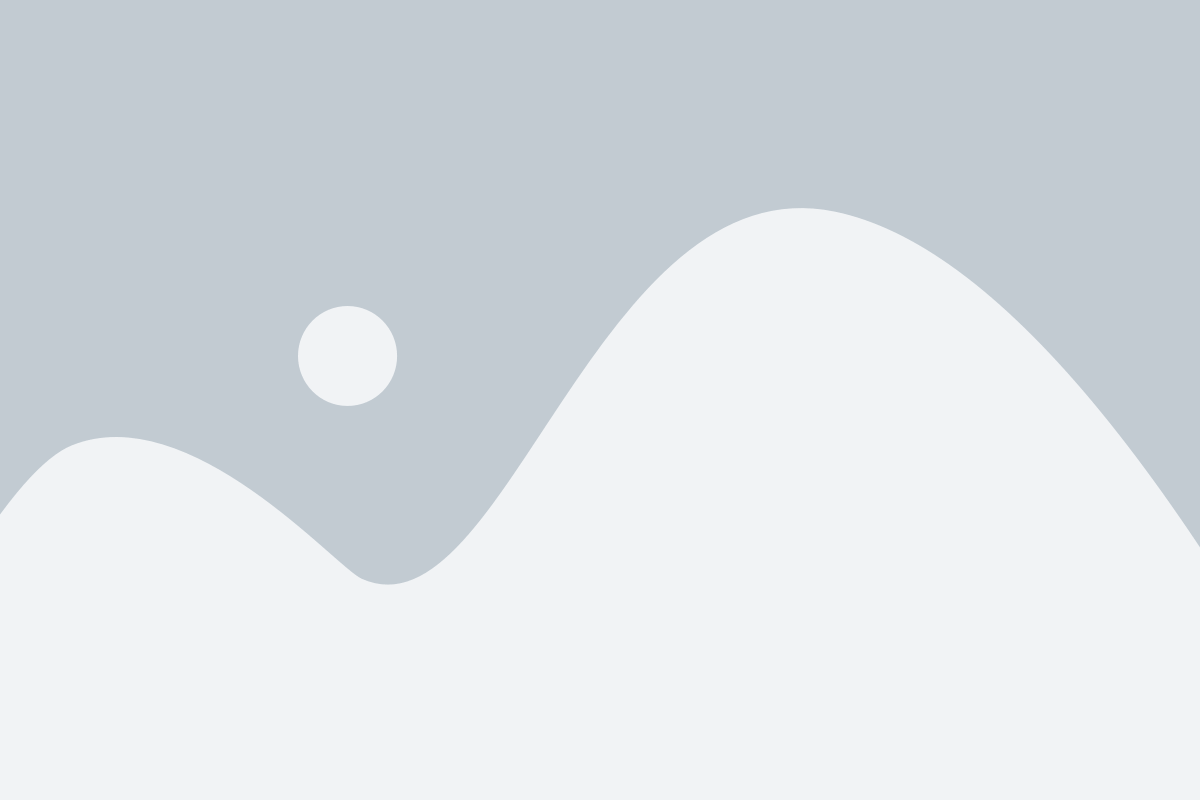
Today’s artificial intelligence (AI) revolution has been made possible by the algorithm revolution. The machine learning algorithms researchers have been developing for decades, when cleverly applied to today’s web-scale data sets, can yield surprisingly good forms of intelligence. For instance, the United States Postal Service has long used neural network models to automatically read handwritten zip code digits. Today’s deep learning neural networks can be trained on millions of electronic photographs to identify faces, and similar algorithms may increasingly be used to navigate automobiles and identify tumors in X-rays. The IBM Watson information retrieval system could triumph on the game show “Jeopardy!” partly because most human knowledge is now stored electronically.
But current AI technologies are a collection of big data-driven point solutions, and algorithms are reliable only to the extent that the data used to train them is complete and appropriate. One-off or unforeseen events that humans can navigate using common sense can lead algorithms to yield nonsensical outputs.
Design thinking is defined as human-centric design that builds upon the deep understanding of our users (e.g., their tendencies, propensities, inclinations, behaviours) to generate ideas, build prototypes, share what you’ve made, embrace the art of failure (i.e., fail fast but learn faster) and eventually put your innovative solution out into the world. And fortunately for us humans (who really excel at human-centric things), there is a tight correlation between the design thinking and artificial intelligence.
Artificial intelligence technologies could reshape economies and societies, but more powerful algorithms do not automatically yield improved business or societal outcomes. Human-centered design thinking can help organizations get the most out of cognitive technologies.
Divergence from More Powerful Intelligence To More Creative Intelligence
While algorithms can automate many routine tasks, the narrow nature of data-driven AI implies that many other tasks will require human involvement. In such cases, algorithms should be viewed as cognitive tools capable of augmenting human capabilities and integrated into systems designed to go with the grain of human—and organizational—psychology. We don’t want to ascribe to AI algorithms more intelligence than is really there. They may be smarter than humans at certain tasks, but more generally we need to make sure algorithms are designed to help us, not do an end run around our common sense.
Design Thinking at Enterprise Premise
Although cognitive design thinking is in its early stages in many enterprises, the implications are evident. Eschewing versus embracing design thinking can mean the difference between failure and success. For example, a legacy company that believes photography hinges on printing photographs could falter compared to an internet startup that realizes many customers would prefer to share images online without making prints, and embraces technology that learns faces and automatically generates albums to enhance their experience.
To make design thinking meaningful for consumers, companies can benefit from carefully selecting use cases and the information they feed into AI technologies. In determining which available data is likely to generate desired results, enterprises can start by focusing on their individual problems and business cases, create cognitive centres of excellence, adopt common platforms to digest and analyze data, enforce strong data governance practices, and crowdsource ideas from employees and customers alike.
In assessing what constitutes proper algorithmic design, organizations may confront ethical quandaries that expose them to potential risk. Unintended algorithmic bias can lead to exclusionary and even discriminatory practices. For example, facial recognition software trained on insufficiently diverse data sets may be largely incapable of recognizing individuals with different skin tones. This could cause problems in predictive policing, and even lead to misidentification of crime suspects. If the training data sets aren’t really that diverse, any face that deviates too much from the established norm will be harder to detect. Accordingly, across many fields, we can start thinking about how we create more inclusive code and employ inclusive coding practices.
CXO Strategy for Cognitive Design Thinking
CIOs can introduce cognitive design thinking to their organizations by first determining how it can address problems that conventional technologies alone cannot solve. The technology works with the right use cases, data, and people, but demonstrating value is not always simple. However, once CIOs have proof points that show the value of cognitive design thinking, they can scale them up over time.
CIOs benefit from working with business stakeholders to identify sources of value. It is also important to involve end users in the design and conception of algorithms used to automate or augment cognitive tasks. Make sure people understand the premise of the model so they can pragmatically balance algorithm results with other information.
Enterprise Behavioral Science – From Insights to Influencing Business Decisions
Every January, how many people do you know say that they want to resolve to save more, spend less, eat better, or exercise more? These admirable goals are often proclaimed with the best of intentions, but are rarely achieved. If people were purely logical, we would all be the healthiest versions of ourselves.
However, the truth is that humans are not 100% rational; we are emotional creatures that are not always predictable. Behavioral economics evolved from this recognition of human irrationality. Behavioral economics is a method of economic analysis that applies psychological insights into human behavior to explain economic decision-making.
Decision making is one of the central activities of business – hundreds of billions of decisions are made everyday. Decision making sits at the heart of innovation, growth, and profitability, and is foundational to competitiveness. Despite this degree of importance, decision making is poorly understood, and badly supported by tools. A study by Bain & Company found that decision effectiveness is 95% correlated with companies’ financial performance.
Enterprise Behavioral Science is not only about understanding potential outcomes, but to completely change outcomes, and more specifically, change the way in which people behave. Behavioral Science tells us that to make a fundamental change in behavior that will affect the long-term outcome of a process, we must insert an inflection point.
As an example, you are a sales rep and two years ago your revenue was $1 million. Last year it was $1.1 million, and this year you expect $1.2 million in sales. The trend is clear, and your growth has been linear and predictable. However, there is a change in company leadership and your management has increased your quota to $2 million for next year. What is going to motivate you to almost double your revenues? The difference between expectations ($2 million) and reality ($1.2 million) is often referred to as the “behavioral gap” . When the behavioral gap is significant, an inflection point is needed to close that gap. The right incentive can initiate an inflection point and influence a change in behavior. Perhaps that incentive is an added bonus, President’s Club eligibility, a promotion, etc.
Cognitive Design Thinking – The New Indispensable Reskilling Avenue
Artificial intelligence, machine learning, big data analytics and mobile and software development will be the top technology areas where the need for re-skilling will be the highest. India will need 700 million skilled workforce by 2022 to meet the demands of a growing economy. Hence, while there is a high probability that machine learning and artificial intelligence will play an important role in whatever job you hold in the future, there is one way to “future-proof” your career…embrace the power of design thinking.
In fact, integrating design thinking and artificial intelligence can give you “super powers” that future-proof whatever career you decide to pursue. To meld these two disciplines together, one must:
- Understand where and how artificial intelligence and behavioural science can impact your business initiatives. While you won’t need to write machine learning algorithms, business leaders do need to learn how to “Think like a data scientist” in order understand how AI can optimize key operational processes, reduce security and regulatory risks, uncover new monetization opportunities.
- Understand how design thinking techniques, concepts and tools can create a more compelling and emphatic user experience with a “delightful” user engagement through superior insights into your customers’ usage objectives, operating environment and impediments to success.
Design thinking is a mindset. IT firms are trying to move up the curve. Higher-end services that companies can charge more is to provide value and for that you need to know that end-customers needs. For example, to provide value services to banking customers is to find out what the bank’s customer needs are in that country the banking client is based. Latent needs come from a design thinking philosophy where you observe customer data, patterns and provide a solution that the customer does not know. Therefore, Companies will hire design thinkers as they can predict what the consumer does not know and hence charge for the product/service from their clients. Idea in design thinking is to provide agile product creation or solutions.
Without Design Thinking & Behavioural Science, AI Will be Only an Incremental Value
Though organizations understand the opportunity that big data presents, many struggles to find a way to unlock its value and use it in tandem with design thinking – making “big data a colossal waste of time & money.” Only by combining quantitative insights gathered using AI, machine/deep learning, and qualitative research through behavioural science, and finally design thinking to uncover hidden patterns and leveraging it to understand what the customer would want, will we be able to paint a complete picture of the problem at hand, and help drive towards a solution that would create value for all stakeholders.
Related Posts
AIQRATIONS
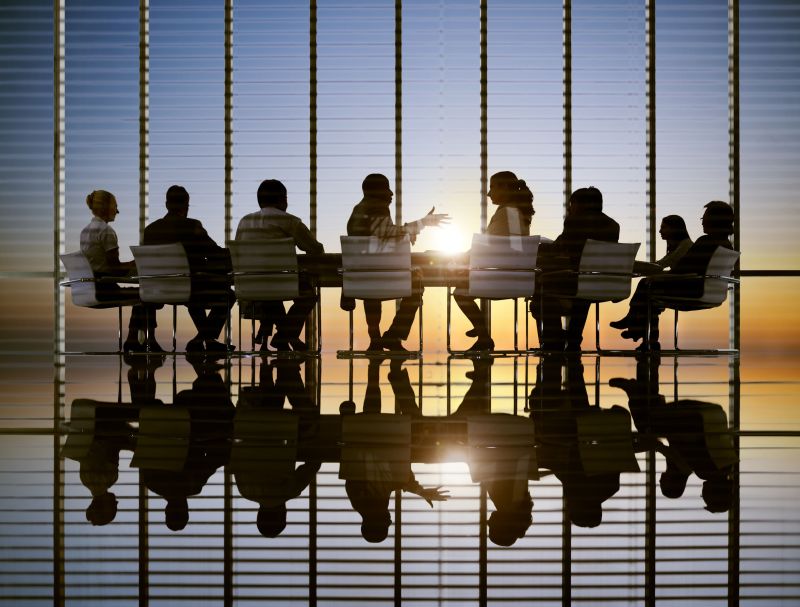
How CXOs are Leveraging AI to Pivot Business Strategy and Operational Models
Add Your Heading Text Here
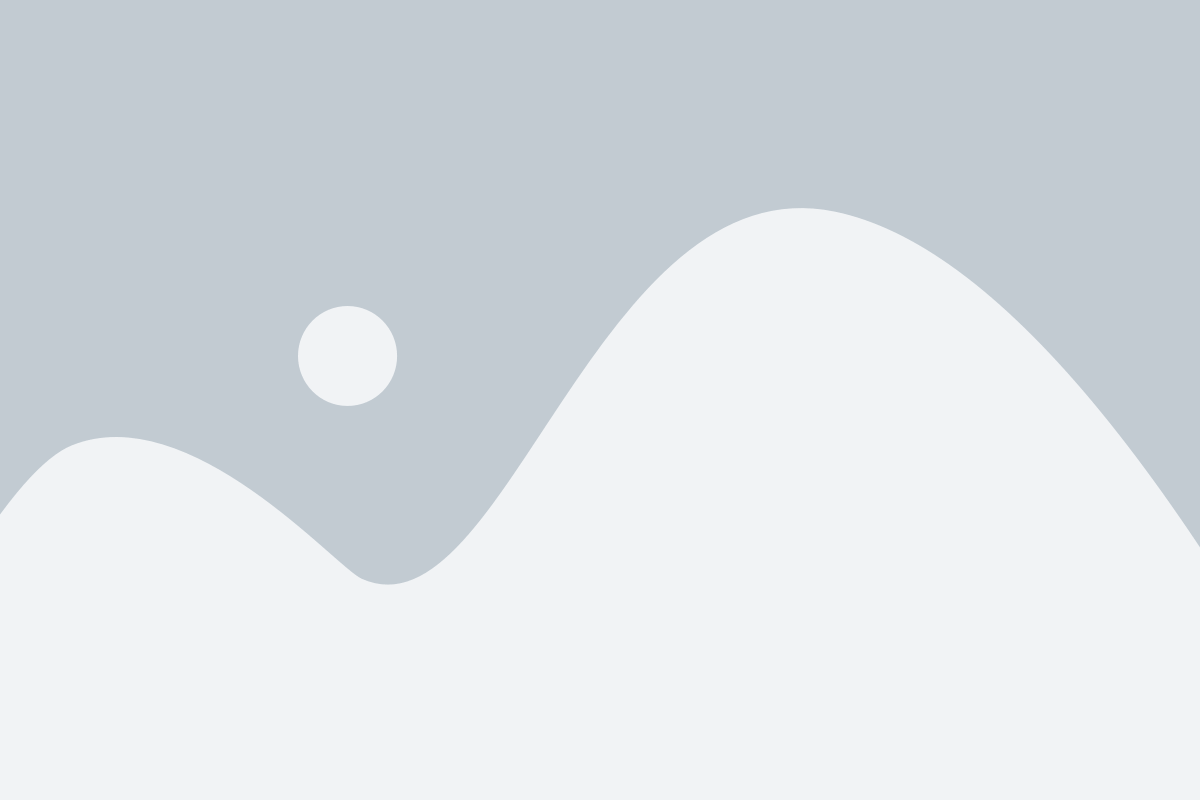
AlphaGo caused a stir by defeating 18-time world champion Lee Sedol in Go, a game thought to be impenetrable by AI for another 10 years. AlphaGo’s success is emblematic of a broader trend: An explosion of data and advances in algorithms have made technology smarter than ever before. Machines can now carry out tasks ranging from recommending movies to diagnosing cancer — independently of, and in many cases better than, humans. In addition to executing well-defined tasks, technology is starting to address broader, more ambiguous problems. It’s not implausible to imagine that one day a “strategist in a box” could autonomously develop and execute a business strategy. We’ve spoken to CXOs and leaders who express such a vision — and companies such as Amazon and Alibaba are already beginning to make it a reality.
Business Processes – Increasing productivity by reducing disruptions
AI algorithms are not natively “intelligent.” They learn inductively by analyzing data. While most leaders are investing in AI talent and have built robust information infrastructures,
As Airbus started to ramp up production of its new A350 aircraft, the company faced a multibillion-euro challenge. The plan was to increase the production rate of that aircraft faster than ever before. To do that, they needed to address issues like responding quickly to disruptions in the factory. Because they will happen. Airbus turned to artificial intelligence. It combined data from past production programs, continuing input from the A350 program, fuzzy matching, and a self-learning algorithm to identify patterns in production problems.
AI led to rectification of about 70% of the production disruptions for Airbus, by matching to solutions used previously — in near real time.
Just as it is enabling speed and efficiency at Airbus, AI capabilities are leading directly to new, better processes and results at other pioneering organizations. Other large companies, such as BP, Infosys, Wells Fargo, and Ping An Insurance, are already solving important business problems with AI. Many others, however, have yet to get started.
Integrated Strategy Machine – The Implementation Scope Augmented AI
The integrated strategy machine is the AI analog of what new factory designs were for electricity. In other words, the increasing intelligence of machines could be wasted unless businesses reshape the way they develop and execute their strategies. No matter how advanced technology is, it needs human partners to enhance competitive advantage. It must be embedded in what we call the integrated strategy machine. An integrated strategy machine is the collection of resources, both technological and human, that act in concert to develop and execute business strategies. It comprises a range of conceptual and analytical operations, including problem definition, signal processing, pattern recognition, abstraction and conceptualization, analysis, and prediction. One of its critical functions is reframing, which is repeatedly redefining the problem to enable deeper insights.
Amazon represents the state-of-the-art in deploying an integrated strategy machine. It has at least 21 data science systems, which include several supply chain optimization systems, an inventory forecasting system, a sales forecasting system, a profit optimization system, a recommendation engine, and many others. These systems are closely intertwined with each other and with human strategists to create an integrated, well-oiled machine. If the sales forecasting system detects that the popularity of an item is increasing, it starts a cascade of changes throughout the system: The inventory forecast is updated, causing the supply chain system to optimize inventory across its warehouses; the recommendation engine pushes the item more, causing sales forecasts to increase; the profit optimization system adjusts pricing, again updating the sales forecast.
Manufacturing Operations – An AI assistant on the floor
CXOs at industrial companies expect the largest effect in operations and manufacturing. BP plc, for example, augments human skills with AI in order to improve operations in the field. They have something called the BP well advisor that takes all of the data that’s coming off of the drilling systems and creates advice for the engineers to adjust their drilling parameters to remain in the optimum zone and alerts them to potential operational upsets and risks down the road. They are also trying to automate root-cause failure analysis to where the system trains itself over time and it has the intelligence to rapidly assess and move from description to prediction to prescription.
Customer-facing activities – near real time scoring
Ping An Insurance Co. of China Ltd., the second-largest insurer in China, with a market capitalization of $120 billion, is improving customer service across its insurance and financial services portfolio with AI. For example, it now offers an online loan in three minutes, thanks in part to a customer scoring tool that uses an internally developed AI-based face-recognition capability that is more accurate than humans. The tool has verified more than 300 million faces in various uses and now complements Ping An’s cognitive AI capabilities including voice and imaging recognition.
AI Strategy for Different Operational Models
To make the most of this technology implementation in various business operations in your enterprise, consider the three main ways that businesses can or will use AI:
Assisted intelligence
Now widely available, improves what people and organizations are already doing. For example, Google’s Gmail sorts incoming email into “Primary,” “Social,” and “Promotion” default tabs. The algorithm, trained with data from millions of other users’ emails, makes people more efficient without changing the way they use email or altering the value it provides. Assisted intelligence tends to involve clearly defined, rules-based, repeatable tasks.
Assisted intelligence apps often involve computer models of complex realities that allow businesses to test decisions with less risk. For example, one auto manufacturer has developed a simulation of consumer behavior, incorporating data about the types of trips people make, the ways those affect supply and demand for motor vehicles, and the variations in those patterns for different city topologies, marketing approaches, and vehicle price ranges. The model spells out more than 200,000 variations for the automaker to consider and simulates the potential success of any tested variation, thus assisting in the design of car launches. As the automaker introduces new cars and the simulator incorporates the data on outcomes from each launch, the model’s predictions will become ever more accurate.
Augmented intelligence
Augmented Intelligence, emerging today, enables organizations and people to do things they couldn’t otherwise do. Unlike assisted intelligence, it fundamentally alters the nature of the task, and business models change accordingly.
For example, Netflix uses machine learning algorithms to do something media has never done before: suggest choices customers would probably not have found themselves, based not just on the customer’s patterns of behavior, but on those of the audience at large. A Netflix user, unlike a cable TV pay-per-view customer, can easily switch from one premium video to another without penalty, after just a few minutes. This gives consumers more control over their time. They use it to choose videos more tailored to the way they feel at any given moment. Every time that happens, the system records that observation and adjusts its recommendation list — and it enables Netflix to tailor its next round of videos to user preferences more accurately. This leads to reduced costs and higher profits per movie, and a more enthusiastic audience, which then enables more investments in personalization (and AI).
Autonomous intelligence
Being developed for the future, Autonomous Intelligence creates and deploys machines that act on their own. Very few autonomous intelligence systems — systems that make decisions without direct human involvement or oversight — are in widespread use today. Early examples include automated trading in the stock market (about 75 percent of Nasdaq trading is conducted autonomously) and facial recognition. In some circumstances, algorithms are better than people at identifying other people. Other early examples include robots that dispose of bombs, gather deep-sea data, maintain space stations, and perform other tasks inherently unsafe for people.
As you contemplate the introduction of artificial intelligence, articulate what mix of the three approaches works best for you.
- Are you primarily interested in upgrading your existing processes, reducing costs, and improving productivity? If so, then start with assisted intelligence, probably with a small group of services from a cloud-based provider.
- Do you seek to build your business around something new — responsive and self-driven products, or services and experiences that incorporate AI? Then pursue an augmented intelligence approach, probably with more complex AI applications resident on the cloud.
- Are you developing a genuinely new technology? Most companies will be better off primarily using someone else’s AI platforms, but if you can justify building your own, you may become one of the leaders in your market.
The transition among these forms of AI is not clean-cut; they sit on a continuum. In developing their own AI strategy, many companies begin somewhere between assisted and augmented, while expecting to move toward autonomous eventually.
Related Posts
AIQRATIONS

The most strategic agenda in CEO’s mind – Is the enterprise AI ready ?
Add Your Heading Text Here
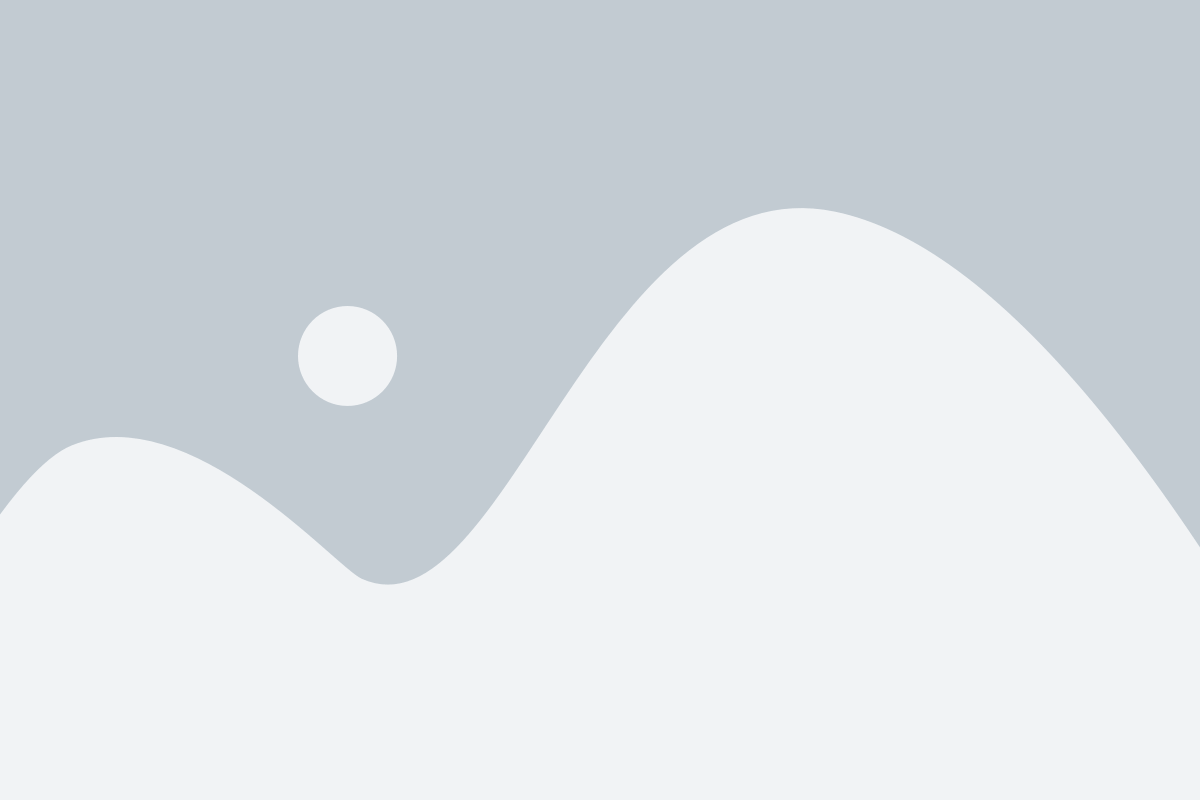
For the larger mass of professionals, the words “artificial intelligence,” or AI, often conjure up images of robots, the sorts of robots that might someday take their jobs. But at the enterprise level, AI means something different. It has enormous power and potential: it can disrupt, innovate, enhance, and in many cases totally transform a business. Forrester Research predicts a 300% increase in AI investment in 2017 from last year, and IDC estimates that the AI market will surge from about $8 billion in 2016 to more than $47 billion in 2020. There’s solid proof that the investment can pay off—if CEO’s can adopt the right strategy. Organizations that deploy AI strategically enjoy advantages ranging from cost reductions and higher productivity to top-line benefits such as increasing revenue and profits, richer customer experiences, and working-capital optimization. The survey shows that the companies winning at AI are also more likely to enjoy broader business success.
So How to make your Enterprise AI Ready?
just one quarter of organizations say they are getting significant impact from it. But these leading businesses have taken clear, practical steps to get the results they want. Here are five of their key strategies:
- Core AI Resource Assimilation using Funding or Acquisition
- Gain senior management support
- Focus on process, not function
- Reskill your teams and foster a learning culture
- Shift from system-of-record to system-of-intelligence apps, platforms
- Encourage innovation
Core AI Resource Assimilation using Funding or Acquisition
As per insights from Forbes and Cowen & Company, 81% of IT leaders are currently investing in or planning to invest in Artificial Intelligence (AI). Based on the study, CIOs have a new mandate to integrate AI into IT technology stacks. The study found that 43% are evaluating and doing a Proof of Concept (POC) and 38% are already live and planning to invest more. The following graphic provides an overview of company readiness for machine learning and AI projects.
Through 2020, organization using cognitive ergonomics and system design in new AI projects will achieve long term success four times more often than others
– Gartner
With $1.7 billion invested in AI startups in Q1 2017 alone, and the exponential efficiencies created by this sort of technology, this evolution will happen quicker than many business leaders are prepared for. If you aren’t sure where to start, don’t worry – you’re not alone. The good news is that you still have options:
- You can acquire, or invest in, an innovative technology company applying AI/ML in your market, and gain access to new product and AI/ML talent.
- You can seek to invest as a limited partner in a few early stage AI focused VC firms, gaining immediate access and exposure to vetted early stage innovation, a community of experts and market trends.
- You can set out to build an AI-focused division to optimize your internal processes using AI, and map out how AI can be integrated into your future products. But recruiting in the space is painful and you will need a strong vision and sense of purpose to attract and retain the best.
- You can use outside development-for-hire shops like new entrant Element.ai, who raised over $100M last June, or more traditional consulting firms, to fill the gaps or get the ball rolling.
Process Based Focus Rather than Function Based
One critical element differentiates AI success from AI failure: strategy. AI cannot be implemented piecemeal. It must be part of the organization’s overall business plan, along with aligned resources, structures, and processes. How a company prepares its corporate culture for this transformation is vital to its long-term success. That includes preparing people by having senior management that understands the benefits of AI; fostering the right skills, talent, and training; managing change; and creating an environment with processes that welcome innovation before, during, and after the transition.
The challenge of AI isn’t just the automation of processes—it’s about the up-front process design and governance you put in to manage the automated enterprise. The ability to trace the reasoning path AI technologies use to make decisions is important. This visibility is crucial in financial services, where auditors and regulators require firms to understand the source of a machine’s decision.
Taking down Resistance to change of Upper Management
One of the biggest challenges to digital transformation is resistance to change. The survey found that upper management is the group most strongly opposed to AI implementation. C-suite executives may not have warmed up to it either. There is such a lack of understanding about the benefits which the technology can bring that the C-suite or board members simply don’t want to invest in it, nor do they understand that failing to do so will adversely affect their bottom line and even cause them to go out of business. Regulatory uncertainty about AI, rough experiences with previous technological innovation, and a defensive posture to better protect shareholders, not stakeholders, may be contributing factors.
Pursuing AI without senior management support is difficult. Here the numbers again speak for themselves. The majority of leading AI companies (68%) strongly agree that their senior management understands the benefits AI offers. By contrast, only 7% of laggard firms agree with this view. Curiously, though, the leading group still cites the lack of senior management vision as one of the top two barriers to the adoption of AI.
Reskilling Teams and HR Redeployment
HR and corporate management will need to figure out new jobs for people to do. Redeployment is going to be a huge factor that the better companies will learn how to handle. The question of job losses is a sensitive one, most often played up in news headlines. But AI also creates numerous job opportunities in new and different areas, often enabling employees to learn higher-level skills. In healthcare for example, physicians are learning to work with AI-powered diagnostic tools to avoid mistakes and make better decisions. The question is who owns the data. If HR retains ownership of people data, it continues to have a role. If it loses that, all bets are off.
HR’s other role in an AI future will be to help make decisions about if and when to automate, whether to reskill or redeploy the human workforce, and the moral and ethical aspects of such decisions. Companies which are experimenting with bots and AI with no thought for the implications need to realize that HR should be central to the governance of AI automation.
Given the potential of AI to complement human intelligence, it is vital for top-level executives to be educated about reskilling possibilities. It is in the best interest of companies to train workers who are being moved from jobs that are automated by AI to jobs in which their work is augmented by AI.
The Dawn of System-of-Intelligence Apps & Platforms
Cowen predicts that an Intelligent App Stack will gain rapid adoption in enterprises as IT departments shift from system-of-record to system-of-intelligence apps, platforms, and priorities. The future of enterprise software is being defined by increasingly intelligent applications today, and this will accelerate in the future.
By 2019, AI platform services will cannibalize revenues for 30% of market leading companies -Gartner
Cowen predicts it will be commonplace for enterprise apps to have machine learning algorithms that can provide predictive insights across a broad base of scenarios encompassing a company’s entire value chain. The potential exists for enterprise apps to change selling and buying behaviour, tailoring specific responses based on real-time data to optimize discounting, pricing, proposal and quoting decisions.
The Process of Supporting Innovation
Besides developing capabilities among employees, an organization’s culture and processes must also support new approaches and technologies. Innovation waves take a lot longer because of the human element. You can’t just put posters on the walls and say, ‘Hey, we have become an AI-enabled company, so let’s change the culture.’ The way it works is to identify and drive visible examples of adoption.
Algorithmic trading, image recognition/tagging, and patient data processing are predicted to the top AI uses cases by 2025. Tractica forecasts predictive maintenance and content distribution on social media will be the fourth and fifth highest revenue producing AI uses cases over the next eight years.
In the End, it’s about Transforming Enterprise
AI is part of a much bigger process of re-engineering enterprises. That is the major difference between the sci-fi robots of yesteryear and today’s AI: the technologies of the latter are completely integrated into the fabric of business, allowing private and public-sector organizations to transform themselves and society in profound ways. You don’t have to turn to sci-fi. The story of human/machine collaboration is already playing at an enterprise near
Related Posts
AIQRATIONS
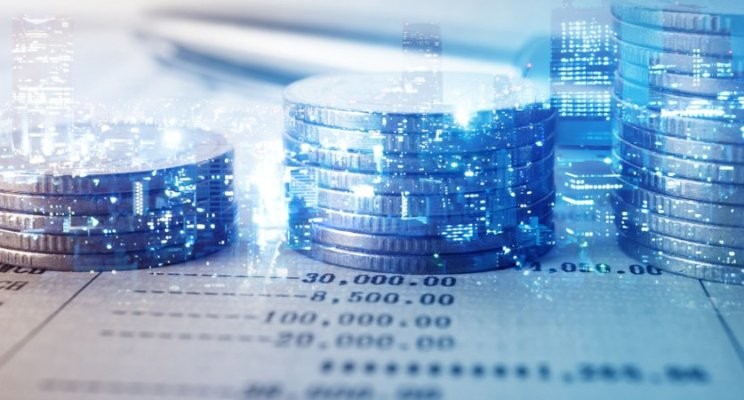
Banking & Financial services rebooted with AI – A perspective for banking professionals
Add Your Heading Text Here
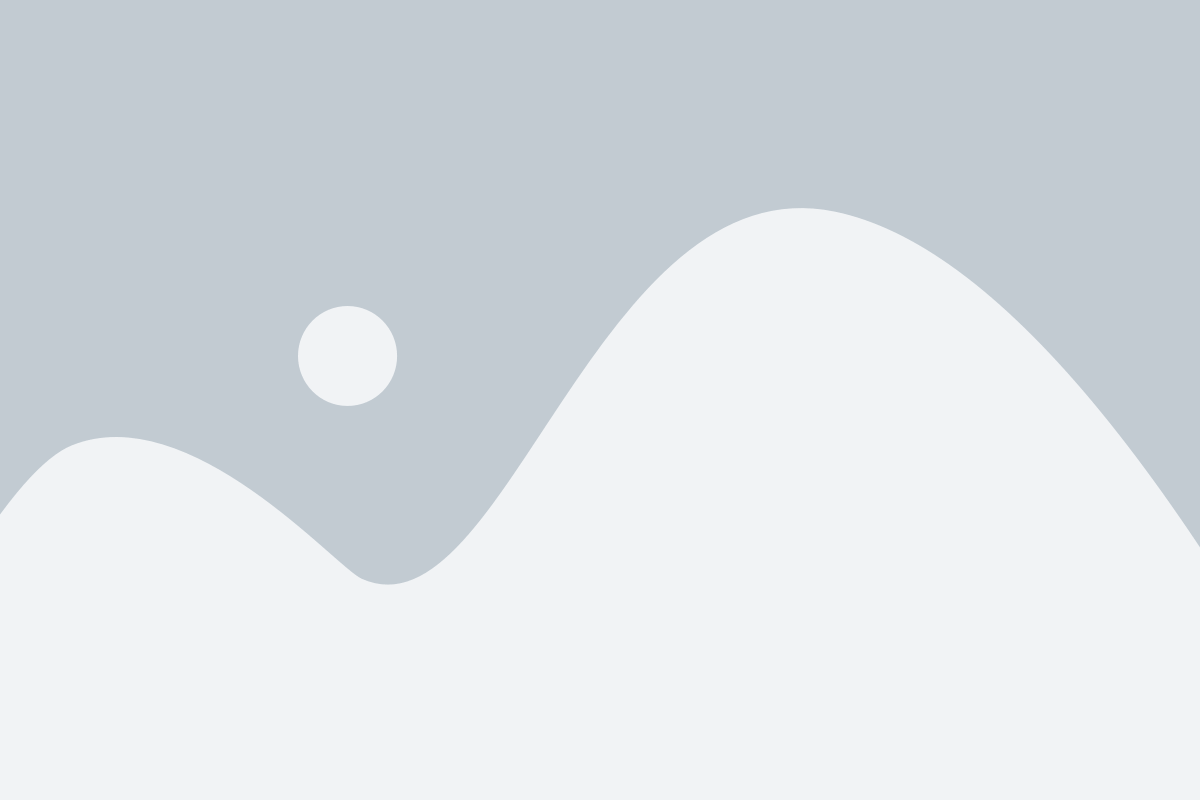
AI today can be described in terms of three application domains: cognitive automation, cognitive engagement and cognitive insight.
- Cognitive automation: In the first AI domain are machine learning (ML), Robotics Process Automation (RPA), natural language processing (NLP) and other cognitive tools to develop deep domain-specific expertise and then automate related tasks.
- Cognitive engagement: At the next level of the AI value tree lies cognitive ‘agents’: systems that employ cognitive technology to engage with people, unlocking the power of unstructured data (industry reports / financial news) leveraging text/image/video understanding, offering a personalized engagement between banks and customers with personalized product offerings and unlocking new revenue streams.
- Cognitive insights: Cognitive Insights refer to the extraction of concepts and relationships from various data streams to generate personalized and relevant answers hidden within a mass of structured and unstructured data. Cognitive Insights allow to detect real time key patterns and relationships from large amount of data across multiple sources to derive deep and actionable insights.
Here are five key applications of artificial intelligence in the Banking industry that will revolutionize the industry in the next 5 years.
AML Pattern Detection
Anti-money laundering (AML) refers to a set of procedures, laws or regulations designed to stop the practice of generating income through illegal actions. In most cases, money launderers hide their actions through a series of steps that make it look like money that came from illegal or unethical sources are earned legitimately.
HSBC has partnered with Silicon Valley-based artificial intelligence startup Ayasdi to automate some of its compliance processes in a bid to become more efficient. The banking group is implementing the company’s AI technology to automate anti money-laundering investigations that have traditionally been conducted by thousands of humans, the bank’s Chief Operating Officer Andy Maguire said in an interview last week.
Chatbots
Chat bots are already being extensively used in the banking industry to revolutionize the customer relationship management at personal level. Bank of America plans to provide customers with a virtual assistant named “Erica” who will use artificial intelligence to make suggestions over mobile phones for improving their financial affairs. Allo, released by Google is another generic realization of chat bots.
The State Bank of India (SBI) on Monday announced SBI Intelligent Assistant (SIA) — a chat assistant aimed to address customer enquiries like a “bank representative” does. Developed by Payjo, an artificial intelligence (AI) banking platform, “SIA” is equipped to handle nearly 10,000 enquiries per second or 864 million in a day — which is nearly 25 per cent of the queries processed by Google each day.
Algorithmic Trading
Plenty of Hedge funds across the globe are using high end systems to deploy artificial intelligence models which learn by taking input from several sources of variation in financial markets and sentiments about the entity to make investment decisions on the fly. Reports claim that more than 70% of the trading today is carried out by automated artificial intelligence systems. Most of these hedge funds follow different strategies for making high frequency trades (HFTs) as soon as they identify a trading opportunity based on the inputs.
A few hedge funds active in AI space are: Two Sigma, PDT Partners, DE Shaw, Winton Capital Management, Ketchum Trading, LLC, Citadel, Voleon, Vatic Labs, Cubist, Point72, Man AHL.
Fraud Detection
Fraud detection is one of the fields which has received massive boost in providing accurate and superior results with the intervention of artificial intelligence. It’s one of the key areas in banking sector where artificial intelligence systems have excelled the most. Starting from the early example of successful implementation of data analysis techniques in the banking industry is the FICO Falcon fraud assessment system, which is based on a neural network shell to deployment of sophisticated deep learning based artificial intelligence systems today, fraud detection has come a long way and is expected to further grow in coming years.
Mastercard announced the acquisition of Brighterion. Brighterion’s portfolio of AI and machine learning technologies provide real-time intelligence from all data sources regardless of type, complexity and volume. Its smart agent technology will be added to Mastercard’s suite of security products already using AI.
Customer Recommendations
Recommendation engines are a key contribution of artificial intelligence in banking sector. It is based on using the data from the past about users and/ or various offerings from a bank like credit card plans, investment strategies, funds, etc. to make the most appropriate recommendation to the user based on their preferences and the users’ history. Recommendation engines have been very successful and a key component in revenue growth accomplished by major banks in recent times.
With Big Data and faster computations, machines coupled with accurate artificial intelligence algorithms are set to play a major role in how recommendations are made in banking sector. For further reading on recommendation engines, you can refer to the complete guide of how recommendation engines work.
JPMorgan, which is spending big on technology as it looks to cut costs and increase efficiency, last year launched a predictive recommendation engine to identify those clients which should issue or sell equity. And now, given the initial success of the engine, it’s being rolled out to other areas.
Strategic Challenges of AI
As with any new endeavor, there are several challenges associated with the development and application of AI solutions.
- Most banks and credit unions are in the early stages of adopting AI technologies. According to a survey conducted by Narrative Science in conjunction with the National Business Research Institute, 32% of financial services executives surveyed confirmed using AI technologies such as predictive analytics, recommendation engines, voice recognition and response.
- Also, one of the biggest challenges is finding the right talent. With only slightly more than half of survey respondents (55%) stating they have identified an AI leader within their company, more than half of those have appointed the head of innovation as the leader.
- In some cases, current employees will not be well positioned for the ‘new age of banking.’ In other cases, the transformation of labor caused by the advances of AI will eliminate some positions entirely.
- 12% of the overall group weren’t using AI yet because they felt it was too new, untested or weren’t sure about the security.
- There is no clear internal ownership of testing emerging technologies— only 6% of those surveyed having an innovation leader or an executive dedicated to testing new ideas and processes.
How to make AI Part of Banking Ecosystem
The potential of open banking and artificial intelligence are intertwined, making up the foundation for a new banking ecosystem that will most likely include both financial and non-financial components. By partnering with fintech providers and data analytic professionals, the power of organizational data and insights can be realized. The partnerships and structure decided upon today will determine an organization’s competitive differentiation in the future.
Multiple providers are offering AI-based solutions and, as a result, banks need to navigate between specialist players and AI powerhouses. The goal will not to become more automated and less personalized, but to use technology and customer insights to become a lot more personalized and contextual.
The banking industry is still in the early stages of developing strong AI solutions. While these solutions can impact the cost and revenue structures of financial organizations, the real potential is with how artificial intelligence can improve the customer experience. Singaporean bank DBS had the vision to launch Digi bank, India’s first mobile-only bank. Being paperless and branchless, Digi bank had to rely on emerging technologies like conversational AI to succeed. Digi bank was built with one-fifth of the cost of a regular retail bank and can contain 82% of customer inquiries with bots. Some banks just want to hand off responsibility to the vendor but Digi bank’s approach is to empower the customer with self-service tools. They don’t want to be professional services
There are four key recommendations that experts make to financial services firms who are looking to effectively exploit the value of AI. These are:
- Look to invest, learn and pair up with experts from outside of the industry
- Make use of cognitive computing to make better use of data
- Implement the right mix of platform technologies
- Strive to maintain a human touch.
In conclusion, it is evident that AI is here to stay, and is impacting a large number of industries, Banking is an early adopter of this trend. This trend is likely to grow exponentially in the future. Companies that embrace this trend are likely to be winners
Related Posts
AIQRATIONS
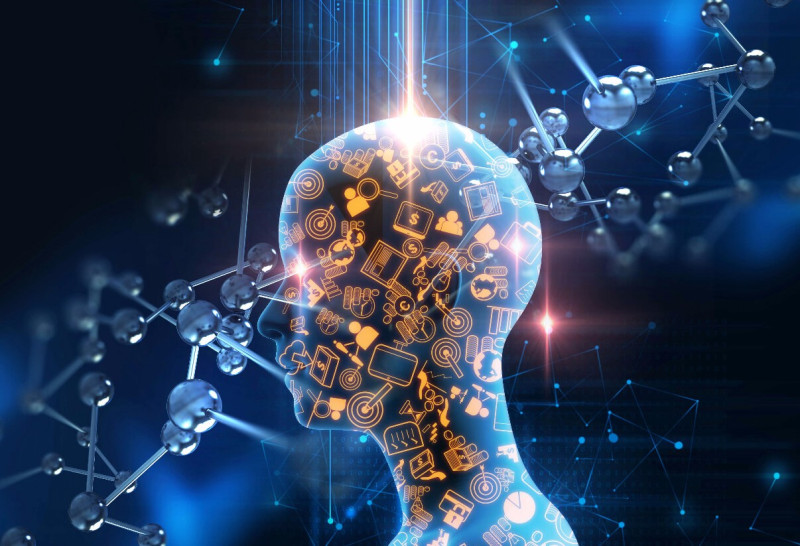
Understanding Burgeoning AI Landscape; A Perspective for PE/VC & Investment Funds
Add Your Heading Text Here
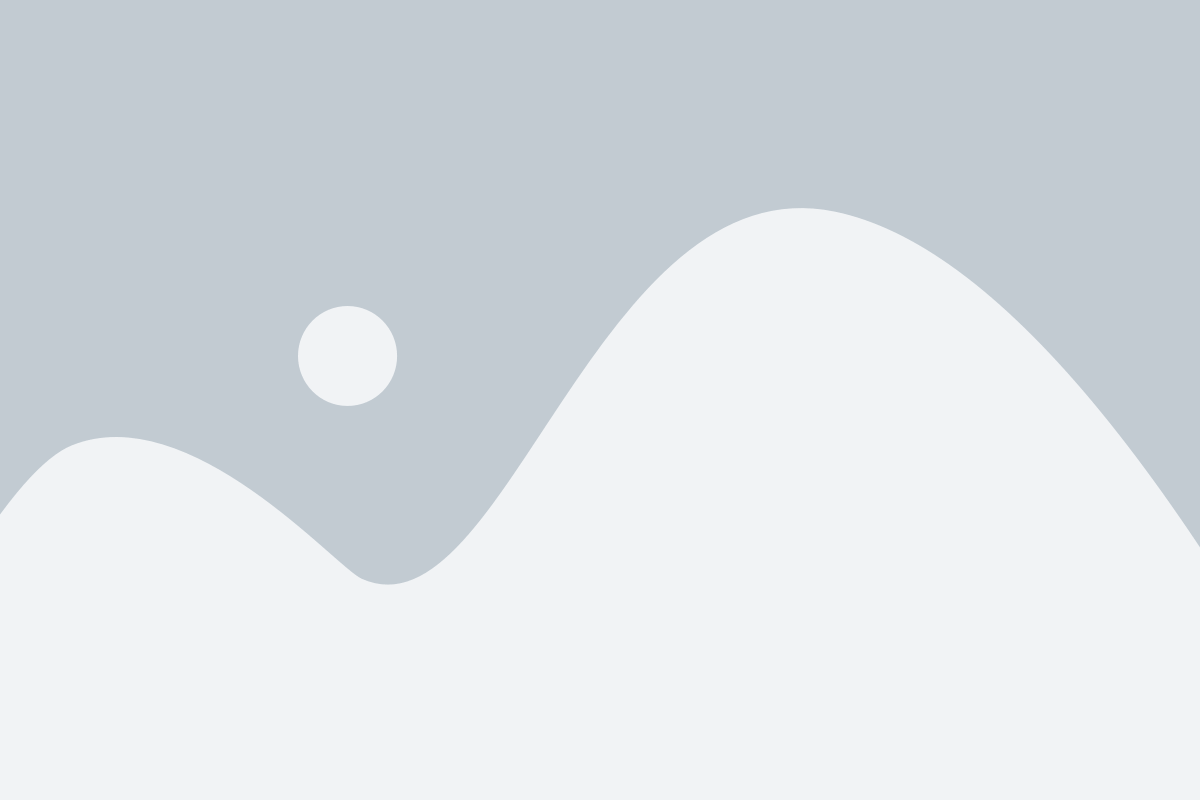
There seems to be a glaring ambiguity as to exactly what artificial intelligence (AI) is, and how the discipline of AI should be categorized. Is AI a form of analytics or is it a totally new discipline that is distinct from analytics? I firmly believe that AI is more closely related to predictive analytics and data sciences than to any other discipline. One might even argue that AI is the next generation of predictive analytics and is born out of sophistication of analytics . Additionally, AI is often utilized in situations where it is necessary to operationalize the analytics process. So, in that sense, AI is also often pushing the envelope of prescriptive, operationalized analytics. It would be a mistake to say that AI is not a form of analytics.
I’ve seen AI applied to some of the most obscure topics you can imagine, ranging from industrial energy usage all the way to finding the right GIFs. Using Artificial Intelligence to improve and create solutions to today’s pressing business and social problems is one of the defining trends of the tech world for me.
So, if you are a PE / VC entity and are looking for investment opportunity in AI space, you will have to understand what kinds of AI companies exist and how this AI practice has evolved from Analytics practice.
There are three types of AI companies — core, applied, and industry
1. Core AI Companies
Core AI companies develop technology that improves parts of the AI creation or deployment process itself. Here are a few selected parts of that process and a few companies that are innovating in each:
Data scrubbing and cleaning: Trifacta, Paxata, Wealthport, Datalogue
Modeling: Sentient, Petuum, MLJar
Deployment: Yhat, Seldon
These companies all innovate in some specific, industry-agnostic part of the AI pipeline. Some of them are specific tools, while others purport to have an entirely new approach to AI that will revolutionize how it’s done (see Geometric Intelligence circa 2015).
If you’re investing in Core AI companies, you should probably have a good understanding of how this pipeline works. If you’re founding one of these companies, you should probably have experience deploying Machine Learning and AI at scale.
2. Applied AI Companies
A bit on the more specific side, Applied AI start-ups develop technology that helps companies across different industries perform a specific task using AI. As with the above, here are some examples of those applications and a few interesting companies in each:
Analysing and understanding text: Indico, Synapsify, Lexalytics
Analysing and understanding images and videos: Clarifai, Kairos, Imagry, Affectiva, Deepomatic
Bots / Voice: Init.ai, MindMeld
While investors can get away with not having experience in one of these specific applications, founders will likely have done projects involving this stuff in the past.
The implementation of AI in this scenario corresponds to the implementation of predictive analytics. At its core, predictive analytics is, naturally, about predicting something. Who will buy? Will certain equipment break? Which price will maximize profits? Each of these questions can be addressed by following a familiar workflow – First, we identify a metric or state that we want to predict and gather historical information on that metric or state. Next, we gather additional data that we believe could be relevant to predicting our target. Then, we pass the data through one or more algorithms that attempt to find a relationship between the target and the additional data. Through this process, a model is created that produces a prediction if new data is fed to it. If a customer had this profile, how likely would she be to respond? If we priced at this point, how much profit might we expect?
The goals and steps followed within an AI process are the same. Let’s look at two examples:
Take image recognition. First, we identify a bunch of cat pictures. Then, we grab a bunch of non-cat pictures. We pass a deep learning algorithm over the images to learn to accurately predict whether an image is a cat. When provided with a new image, the model will answer with the probability that the image is a cat. Sounds a lot like predictive analytics, doesn’t it?
Let’s now consider natural language processing (NLP). We gather a wide range of statements that have specific meanings we care about. We also gather a wide range of other statements. We run NLP procedures against the data to try to tease out how to tell what is important and how to tell what is being asked. As we feed a new line of text to the process, it will identify what the point of the statement is in probabilistic terms. The NLP process will assign probabilities to various possible interpretations and send those back (think Watson playing jeopardy). This also sounds a lot like predictive analytics.
3. Industry AI Companies
The final category of ML/AI companies apply these techniques to specific business problems in specific verticals. This is undoubtedly the lion’s share of the actual number of companies being founded, and in many ways, represents the true promise of AI — solving actual and immediate problems with new techniques. Here, it’s easier to give companies as examples. The format is always “AI for ________”:
DigitalGenius: AI for customer support
Cylance: AI for cyber threat prevention
X.ai: AI for scheduling meetings
Drive.ai: AI for autonomous vehicles
The implementation of AI in this scenario corresponds to industrialized embedded analytics. A major trend today is to embed analytics into business applications so that the models are utilized in an automated, embedded, prescriptive fashion at the point of a business decision. For example, as a person navigates a web page, models are utilized to predict what offers should appear on the next page. There is no human intervention once the process is in place. The process makes offers until told to stop.
Many applications of AI today also require industrialization. For example, as an image is posted on social media, it is immediately analysed to identify who is present in the image. As I make a statement to Siri or Alexa, it attempts to determine what I said and what the best answer is. While this qualifies as a more advanced application of predictive analytics that moves into embedded, prescriptive, automated processes, it is still very much in line with how industrialized embedded analytics are being used today.
The common theme among these companies is that they take Machine Learning / AI and use it on a specific problem or space. When researching investments like this, investors should look at both the AI itself (if it works well) and the business case (whether it’s compelling). In x.ai’s case, investors need to know if the AI works, but they should also consider whether AI is the best way to solve the scheduling problem, and whether scheduling is a problem worth solving at all. With the other two types of companies, this is rarely a consideration. Founders of these types of companies can often not have AI experience and can even be non-technical (with the right supporting team and CTO, of course).
Final Word
Your journey to a fruitful AI investment will be far easier if you recognize and embrace AI as sophistication of analytics and understand the true categorization, and then task your analysts with leading the charge. Don’t cause confusion and redundancy by considering AI to be something completely different.
Related Posts
AIQRATIONS
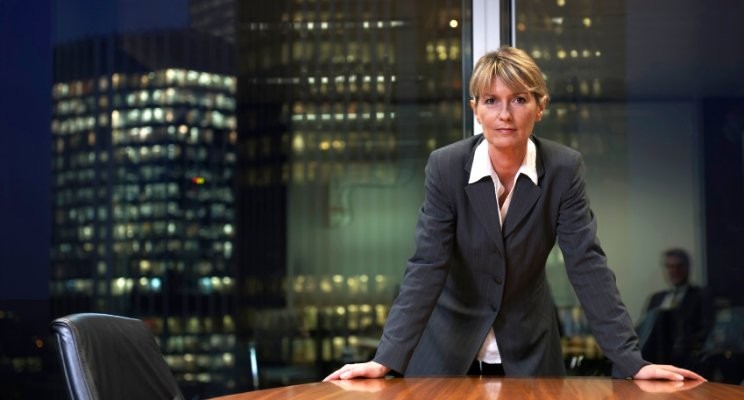
Transformation in marketing redefined by AI – a brief AI Chief Marketing Officer (CMO) primer
Add Your Heading Text Here
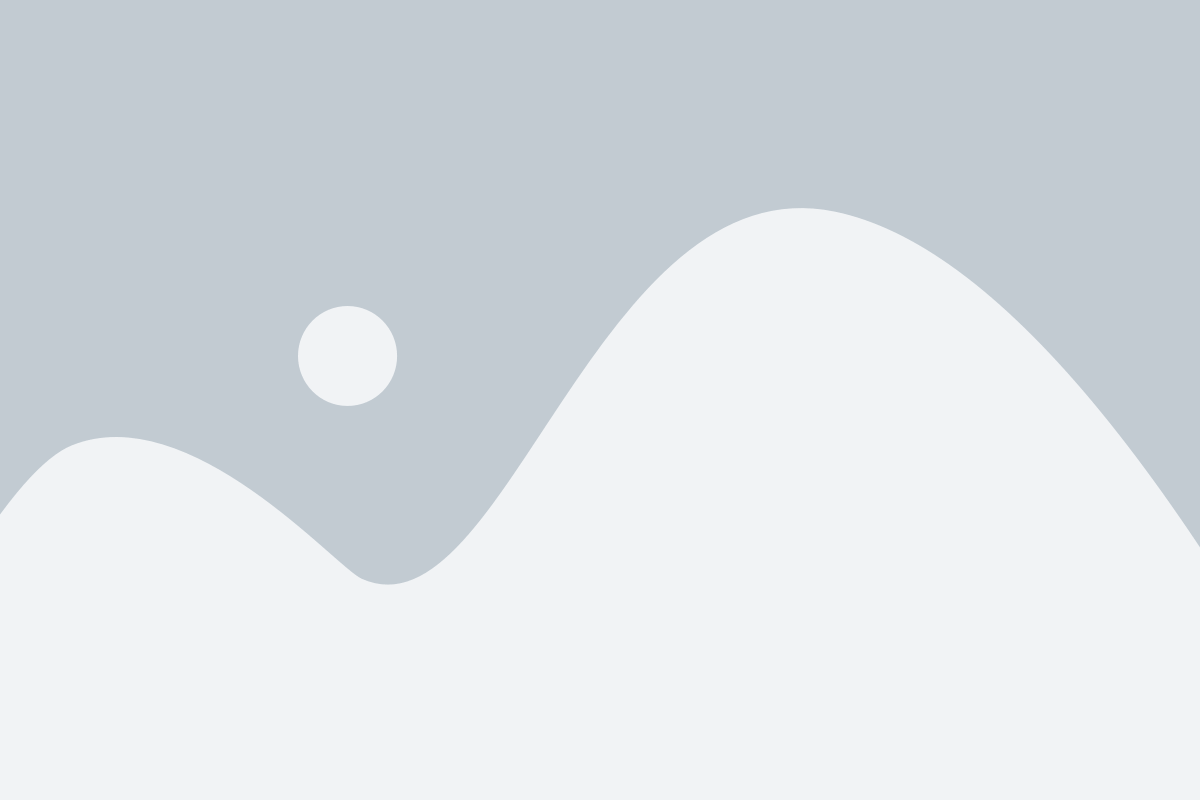
Previous week , I had an opportunity to moderate a fireside chat at NASSCOM Martech conference that carried a theme around changing role for CMO with the advent of AI and I could notice a substantial set of queries during the conference on how AI will redefine marketing. As understandable, each new technology can create fear, uncertainty, and doubt until we understand it better. And AI, with all its hype, fits that bill. But to remain current and relevant, CMOs must quickly understand and apply AI. Here’s a short AI CMO Primer.
Can I put off AI until later?
The answer is no! AI is here. Waiting to deal with it could put you well behind the curve. Leading businesses are already either using AI to profound effect, or actively planning for it.
- Amazon, the company that wants to eat everyone’s lunch, is already driving a third of its business from a AI-powered function: its recommended purchases.
- In a June 2016 report, Weber Shandwick found that 68% of CMOs report their company is “planning for business in the AI era” with 55% of CMOs expecting AI to have a “greater impact on marketing and communications than social media ever had.”
To wait is to get left behind. And as you’ll see later, getting started doesn’t have to be painful or costly.
What is AI, machine learning, and cognitive intelligence?
Academic experts might hate my explanation, but differentiating between AI, machine learning, and cognitive intelligence from a practical CMO perspective isn’t necessary. I use AI as an umbrella term to refers to software that carries out a task which normally requires human intuition—including learning and problem solving.
AI can be thought of as a set of repeatable steps and, while AI doesn’t technically replicate free-will and decision making, it does map out these steps and use computer processing speed to make its way through them to come to an outcome—like how a person would. It can do this much faster, and taking into account far more relevant data than a human would.
Is AI ready for marketing now?
AI has come at the right time, along with the explosion of Big Data. In essence, with access to an incredible amount of data, it’s never been more important for organizations to make sense of it and leverage important pieces out of the noise.
With the exponential growth of cheap, fast, scalable, and interconnected computing and storage in the cloud, the horsepower and data to efficiently run AI algorithms is now within everyone’s reach.
But, that being said, it is also sadly true that there’s one very simple reason why progress towards full automation and AI marketing is relatively sluggish – because most machines aren’t actually learning anything. All of these platforms that exist today, there’s no machine learning. And if it is, their machine learning is, ‘Did someone open an email? Yes, give them a point. That’s not real machine learning. Which is a problem, because effective automation is fast becoming a prerequisite of effective marketing. From chatbots to real-time contextual geographic marketing, modern marketing solutions demand insight-driven automation to deploy the right message quickly, at scale.
marketing automation (especially AI marketing) will have to eventually free marketers from manual work which comprises ‘98% of their eight hours a day’, empowering them to spend their time more productively tackling the creative jobs that machines aren’t well suited to. This requires three key problems AI marketing providers need to solve:
1. The creation of effective, scalable machine learning which can optimize a campaign without human input.
2. Ensuring that decision-making system’s logic is transparent and easily comprehensible by marketers seeking to analyze and augment those automated insights.
3. Designing a prescriptive system which can not only predict future actions – but understand why the user would make those actions.
How can AI be applied to marketing?
AI has the potential to revolutionize customer engagement, customer service, and marketing automation. It can enhance the way we communicate with new, current, and inactive customers, and automate admin functions at the backend. In other words, it can help make marketing operations more efficient and effective.
AI can far more accurately predict next best action, by churning through (in real-time) all relevant data about the customers – purchases, interactions, social media posts, email exchanges – and then learn from the results and do it on a scale not previously possible.
For example, let’s say you have a few million customers and want to communicate with them as if you know them very well, providing everyone the right offer at the right time. AI can enable this level of personalization at a scale of millions of individuals, and in near real time.
In essence, AI can save marketers time and bring companies far closer to their customers, without worrying about IT, data lakes, data quality, or hiring armies of data scientists.
Do I need to become an AI expert?
The short answer is no. AI systems shouldn’t require you to become a mathematician. With AI system, you’ll be able to focus on the results not the process of churning through of thousands, millions, or trillions of data points to arrive at the insights you need about your customers.
How much will it cost?
Surprisingly, AI systems can reduce costs and eliminate waste. AI systems can significantly reduce the requirement for data engineers and data scientists, or the need to depend on IT teams.
And AI can take wasted effort out of the system by providing a deeper understanding of what your customers want and how to interact with them effectively.
How do I get started?
First, start exploring today. Read, talk to people, and evaluate first hand. Select a contained, but impactful area business problem. A subset of your customer loyalty system could make a great initial project. Loyal customers should be the life blood of most companies, but often are underserved as it’s difficult to pull together and analyze all relevant data in a timely manner. This is a perfect fit for AI because there’s typically a lot more known data for AI to analyze about current customers, as compared to prospects. And it’s a project where you can start seeing high-impact results in weeks—perhaps even new revenue from customers who were previously inactive.
Related Posts
AIQRATIONS
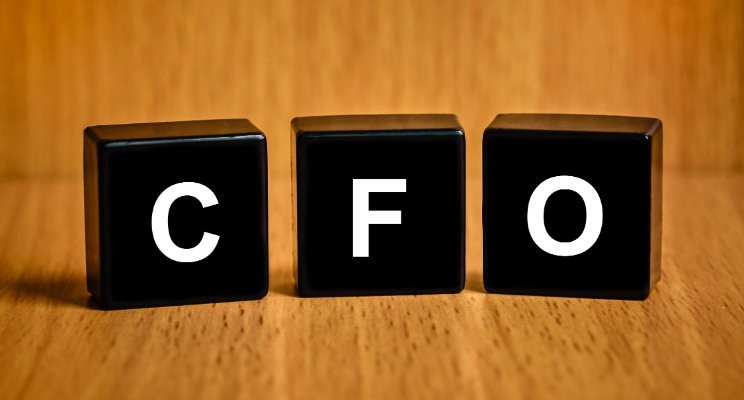
Financial transformation accomplished by AI – a perspective for Chief Financial Officer (CFO)
Add Your Heading Text Here
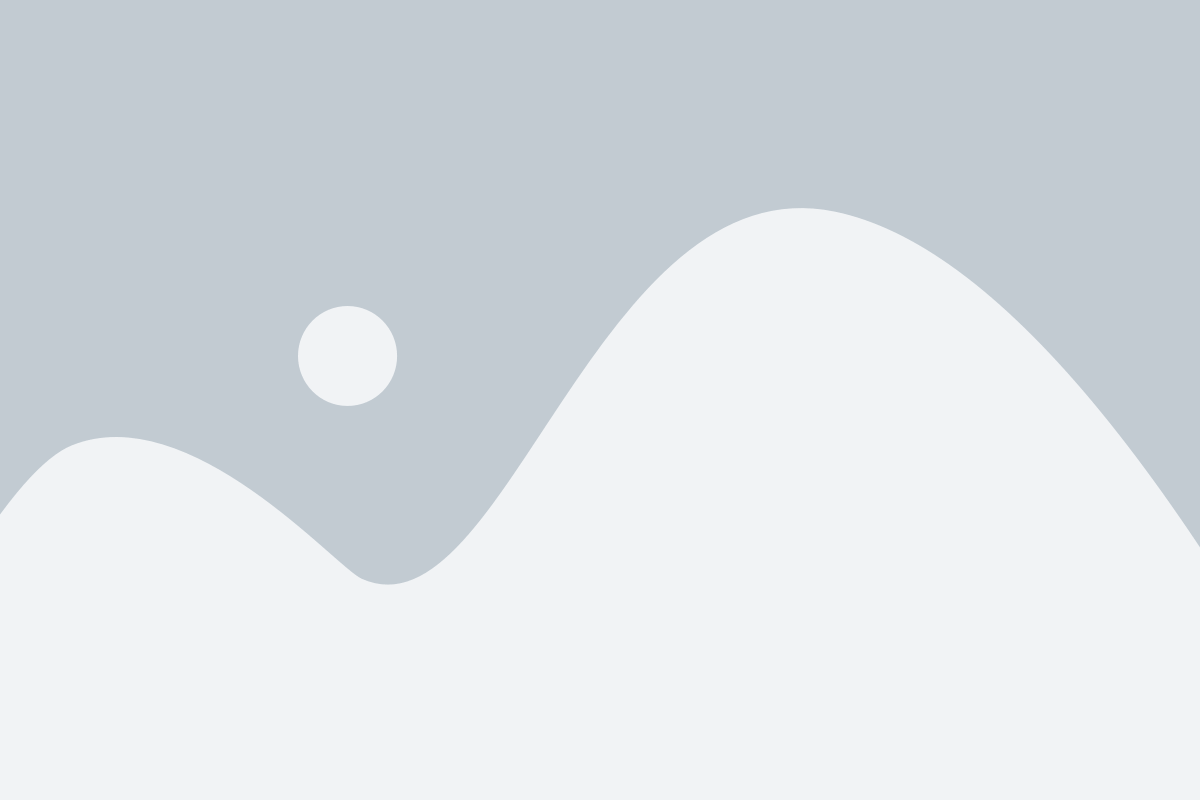
For organizations, transforming finance and accounting function via adoption of topical technology means improving how they pre-empt red flags around the financial transactions within the organizations.
In fact, prudent finance and accounting operability represents the single biggest challenge firms have to deliver on their priorities, according to a survey by Econsultancy.
What’s more, 45% of respondents indicated that embedding analytics &AI relevant as possible in the finance and accounting function is their key focus.
CFOs around the world are not asking if digital disruption will occur, but instead, what it means for their function. So the question asked in this article, is how can CFO’s leverage digital transformation wave using AI to advance their organization’s competitive position and improve performance of their function ?
CFO of Tomorrow
With business around the world undergoing digital transformation, the roles of the c-suite is also changing. Perhaps most significantly, the role of the chief financial officer is moving from simply counting pennies to being a major driver of change within companies.
In the past, the role of the CFO was all about getting the numbers. That was 90 per cent of their time, but that is changing and now it’s about understanding the source of the number, understanding what created the number, and understanding the business drivers behind the number. A CFO then needs to try to make sense of the business drivers, and be able to present to the board what the outlook for the organization is, what the costs are, and what actions need to be taken.
This means that the CFO has to have a solid handle on data and analytics, and once they have that in their arsenal, they can become a strategic adviser to the business and they are able to tell the CEO things like what impact customer satisfaction has on the business.
I want to further highlight a few use cases showing how disruptive technologies such as artificial intelligence (AI) and machine learning will be used in the office of the CFO to increase productivity, simplify processes, and support decision-making, and aid in digital finance evolution:
Digital chatbots : Digital assistants for CFOs could impact analytics and the way they handle them. Today, almost everybody in Financial Planning & Analysis (FP&A) receives countless calls asking for information like, “What was our revenue in Q3 last year for this product? What has our growth been over the last three years for this line of business?”
Smart assistants like Amazon’s Alexa and Apple’s Siri can already answer questions on weather forecasts, stock quotes, and so forth, but what if they could provide the latest financial results and give decision makers instant access to information? A CFO could have a conversation with his or her ERP system using a digital assistant to get an immediate response or a clarifying question, without having to open a dashboard or dig into a database.
Risk assessments: When we assess commercial proposals for our services projects, we evaluate each project individually based on the customer characteristics – maturity, industry, size, current system landscape, and so on – as well as the complexity of the products to be implemented. To qualify this assessment, we depend on managers who have previously worked on similar projects. That can limit us to the individual perspective of those managers.
Machine learning could give finance teams and executives the power to access decades’ worth of projects, around the world, at the touch of a button. In levering these insights, teams could then develop a better-informed risk assessment, mapping the project against a much larger database of historical projects.
Invoice clearing: In finance departments today, accounts receivable or treasury clerks can often be challenged in clearing invoice payments, as customers often combine invoices in one payment, pay incorrect amounts, or forget to include invoice numbers with their payments. To clear the invoice, the employee then has two options: manually add up various invoices that could possibly match the payment amount, or reach out to the customer to clarify. In the case of short payment, the employee either has to ask for approvals to accept the short payment or request the remaining amount from the customer.
What if an intelligent system could help streamline this process by suggesting invoices in real time that might match the paid amount and, based on established thresholds, automatically clear the short payments or automatically generate a delta invoice?
Expense-claim auditing: Expense-claim auditing is another routine, transactional finance task. Finance teams are tasked with ensuring that receipts are genuine, match claimed amounts, and are in line with company policy. While state-of-the-art travel-and-expense solutions can simplify the process, a manual audit still needs to be performed.
Machine learning and AI technologies could improve this process, auditing 100% of all claims, and sending only questionable claims to a manager for approval. The machine could read receipts – regardless of language – to ensure that they are genuine, and match them against the policy.
Accruals: Artificial intelligence and machine learning also offer promise when it comes to determining bonus accruals. Today, teams have a myriad of factors to consider when determining bonus accruals. CFO teams look at current headcount salaries and bonus plans, and try to forecast all KPIs in compensation plans. From there, teams try to calculate the most accurate accrual (likely adding a buffer, to be safe). However, oftentimes, accuracy ends of being a matter of luck more than anything else.
By applying machine learning to these calculations, predictive analytics could serve as a valuable tool to generate unbiased accrual figures, leaving finance teams more time during closing periods for other activities that require human review and judgment.
Customer Journey: This is an area where the CFO is ideally placed to play a greater role in contributing to company growth and profits. His perspective on new customer acquisition, retention activities, customer development and predictive customer behavior models is crucial.
AI is what’s making all of this possible. With his new 360° vision and customer knowledge, the CFO can become a strategic business leader. Via AI and the breaking down of old company silos, the customer journey becomes everyone’s concern. And customer engagement wins its rightful place at the heart of business strategy.
The overall impact on jobs in finance: As these advanced technologies continue to penetrate the finance function, a new crop of skills are rising to the forefront when it comes to hiring finance talent. Routine, transactional roles will become less prevalent, while the need for strategic thinkers with cross-functional knowledge and technology prowess will be critical. Additionally, while transactional tasks will be fewer, digital transformation will require additional finance resources to be developed and supported, creating an opportunity to redefine processes and roles.
CFO’s, like everyone else, will have to adopt AI
In the future, it will be the companies that can harness AI that will set themselves apart. They will become fully digital businesses. Forward-thinking CFOs will help this happen. Because, by making AI accessible company-wide, they now have the power to unleash infinite company value.