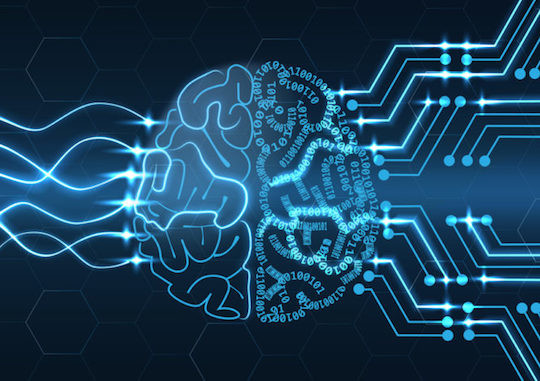
Redesigning exponential technologies landscape with AI & Blockchain fusion
Add Your Heading Text Here
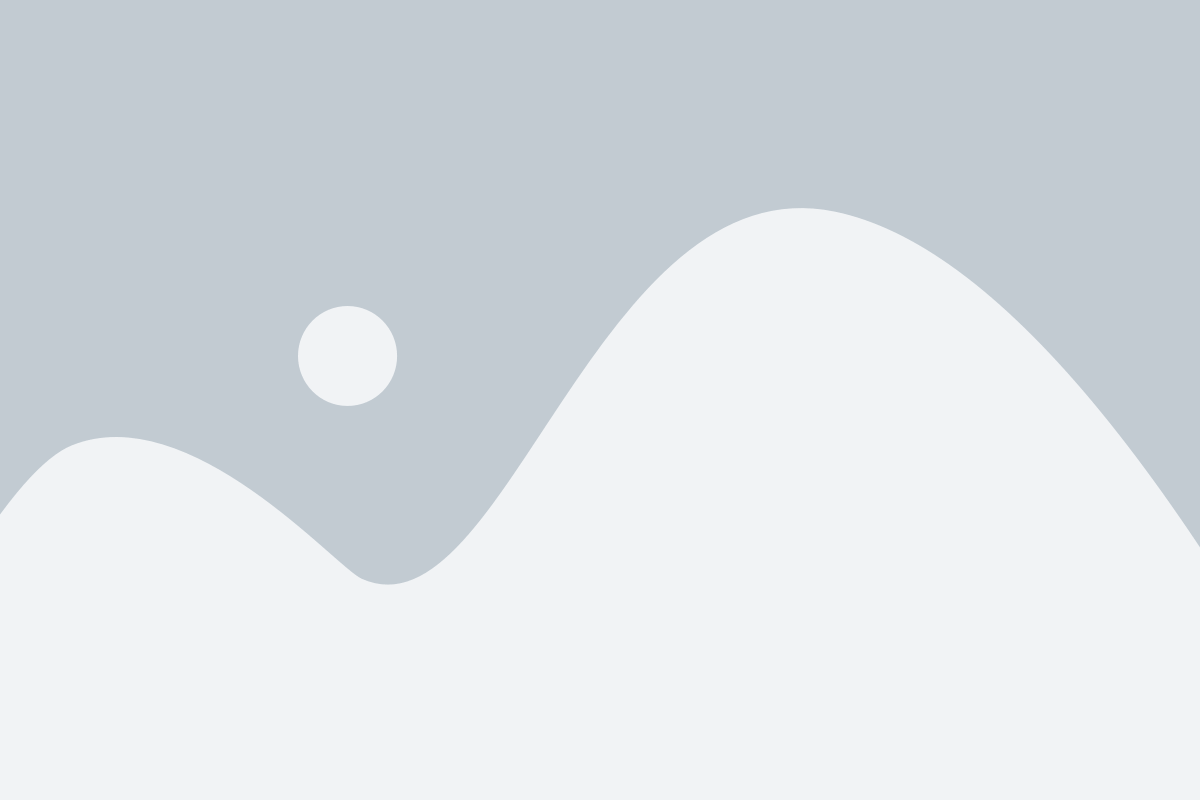
AI and blockchain are two of the prime drivers in the technology space that catalyze the pace of innovation and demonstrating radical shifts across every industry. Each of this technical venture comes with a degree of technical complexity and business implications. Fusion of the two will be able to redesign the entire technical landscape along with a human effect from scratch.
Blockchain has its own limitations, it is a mix of technology-related and culture influence from the financial services sector, but most of them can be conceited by AI in a way or another.
The illustrated points below will be able to give a gist of the potentials that can be realized at the intersection of AI and Blockchain:
Energy consumption in mining: Mining has already proven that it requires tons of energy and is heavy in the economic perspective. AI has mastered in optimizing energy consumption across multiple sectors, similar results can be expected for the blockchain as well. AI can dramatically reduce the costs of maintaining servers and validate potential savings to lower investments in mining hardware.
Federated Learning: Blockchain is growing at a steady pace of 1MB every 10 minutes. Blockchain pruning is a possible solution through AI. A new decentralized learning system such as federated learning, for example, or new data sharing techniques to make the system more efficient.
Security: Concerns still exist on the security system of built-in layers and applications for Blockchain (e.g., the DAO, Bitfinex, etc.). The mileage created by machine learning in the last two years makes AI a solid candidate for the blockchain to guarantee secure applications deployment, especially given the fixed structure of the system.
Blockchain-AI Data gates: Blockchain has proven its ability for record keeping, authentication, and execution while AI drives decisions by assessing/understanding patterns and datasets, ultimately engendering autonomous interaction. The combo (AI and blockchain) will be become a data gate with these several characteristics that will ensure a seamless interaction in the nearest future.
Auditing of AI through blockchain: AI is seen as a black box ( complex set of calculations and algorithms) to distinguish patterns or trends. This makes it a difficult task for the humans to govern the choices taken by the artificial intelligence in yielding results. Accountability of the AI black box is seen as biggest challenge, considering concerns across the community for tampering or the altering happening to the calculations for the given input which eventually reflects in the output generated. This challenge can be easily comprehended by the blockchain innovation. Implementing robust auditing of these calculations utilizing the blockchain is seen as the biggest driver for enhancing the credibility of the business organizations and reinstating trust in the reliability of the information.
Leverage on Artificial Trust: Future roadmap of this fusion can successfully lead into creation of virtual agents that will create new ledger by themselves. Machine to machine interaction will be the new norm reinstating trust in a secure way to share data and coordinate decisions, as well as a robust mechanism to reach a quorum.
Machine performance monitoring and changes: Blockchain miners (companies and individuals) pour an incredible amount of money into specialized hardware components. AI can complement such as machine/equipment monitoring to deploy more efficient systems and do away with the unproductive heavy ones.
Blockchain for better information management: AI has a proven mechanism that runs of an incorporated or centralized database. In such a case, there are always chances for information occurrence of a mishap, i.e. gets lost, altered, or undermined.
Blockchain and artificial intelligence fusion can eliminate the above concern. Under the umbrella of blockchain the data is decentralized and stored within different nodes or systems. This reinstates trust on that your information is safe and unaltered. Most importantly the information is time-stamped and is in the sequence making recuperation less demanding and exact.
Some key challenges on the block: The fusion throws open technical and ethical implications arising from the interaction between these two technologies, such as the need to edit data on a blockchain and most importantly the duo pushing to become data hoarder. Experimentations alone will be able to provide a detailed answer on these lines.
In conclusion blockchain and AI are the two sides of the technology spectrum. One efficiently fosters centralized intelligence while the other promotes decentralized applications in an open-data environment. The fusion of the two will be an intelligent way to amplify positive externalities and advance mankind, most importantly reap the maximum potential for business needs.
Related Posts
AIQRATIONS
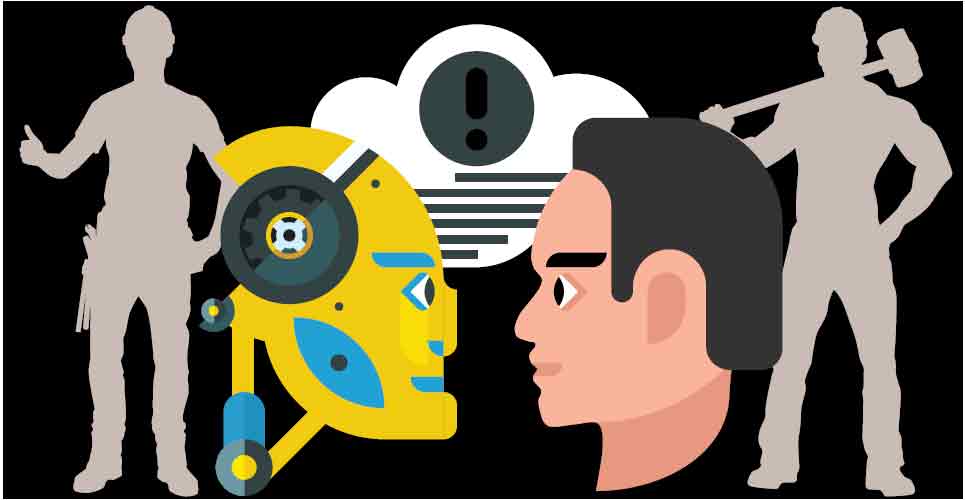
AI for energising SMBs
Add Your Heading Text Here
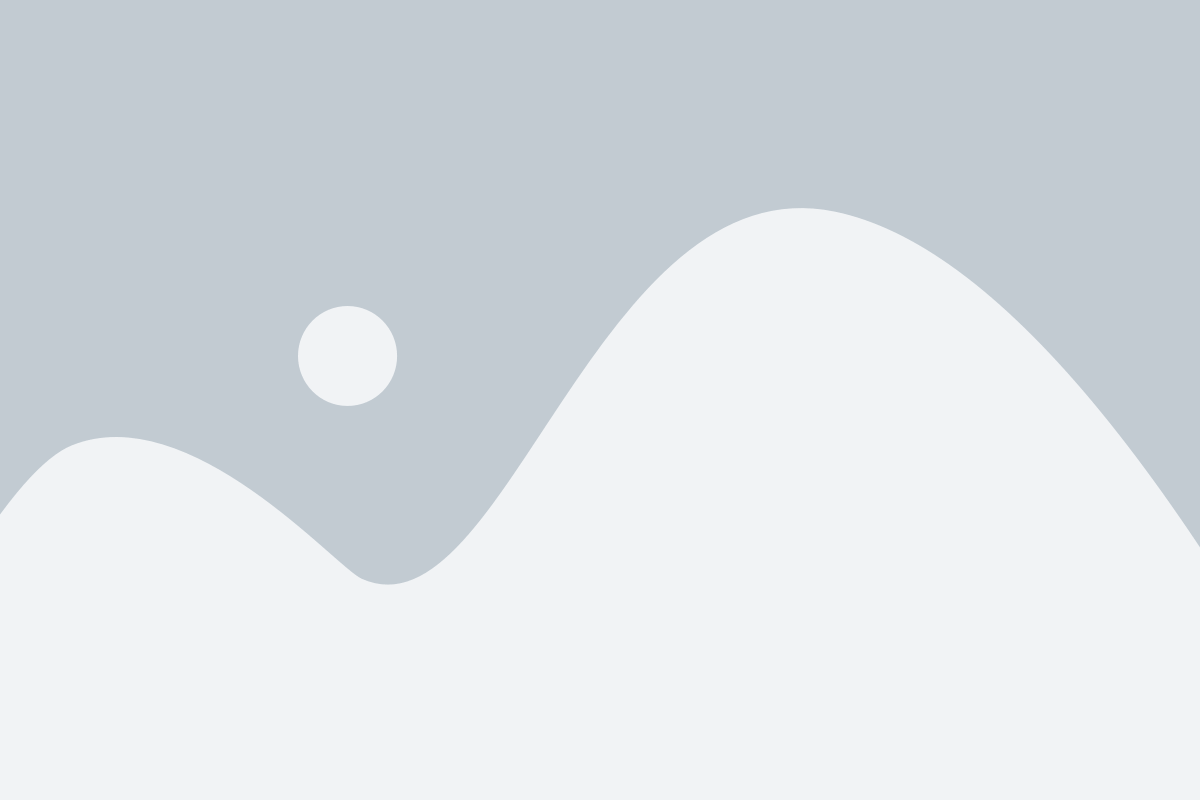
A robust ecosystem for small and medium enterprises is one of the key indicators of economic vibrancy and entrepreneurial energy in a nation. India’s entrepreneurial spirit was given a massive boost after liberalisation in the early 90s. These norms ended the draconian ‘Licence Raj’ that kept the lid on the business aspirations of the average Indian. With improving access to capital, heightened ease of doing business and a galvanised ecosystem that provides mentorship and guidance to fledgling startup founders, the small and medium enterprise (SME) sector has been riding high over the past couple of decades. Now with Artificial Intelligence (AI) in the mix, SMEs will be given another boost – through reduced cost and improved efficiencies in how they run, operate and succeed.
There is no doubt that AI will be an important gamechanger for the SME sector. Startups today are much more data-rich than before and understand the value that can be unlocked through intelligent deployment of advanced analytics. They understand that a data-driven understanding of their business landscape will far outweigh heuristic methods in the dynamic environment in which their enterprises operate. Further, with lowering cost of adoption, increased focus on the SME industry by incumbent analytics/AI vendors and partners, we have a perfect storm of sorts for the sector to derive the exponential benefits of this technology. Let us look at the areas where AI can deliver a strong, demonstrable impact on the sector and how such businesses can get started on their AI journey.
Galvanising SME Operations
When run on the right data set, AI can work its magic in providing untold operational benefits to SMEs. The case for AI in the startup sector is much stronger than it is for their larger corporate counterparts. The reasons for that are two-fold. First, startups typically operate on smaller budgets – which means that they need to automate as much as they can to reduce costs associated with a higher headcount.
Secondly, startups by their very nature are extremely nimble, allowing them to experiment rapidly with new, innovative technologies. This twofold advantage means that AI vendors as SMEs need to have a robust strategy in place to work together and uncover the latent advantages offered by this technology. Here are a few areas where AI can specifically help startups galvanise their operations.
• Predictive maintenance: SMEs, especially in the manufacturing segment, can unlock huge benefits in the production process using AI. With sophisticated algorithms monitoring machine health, AI can help reduce the downtime in production schedules by accurately modelling when a critical machine is likely to go down, allowing businesses to better plan demand fulfilment.
• Supply chain and logistics: A major drain on the revenue of nascent businesses is the cost associated with procurement of raw materials and delivery of finished products. By using AI and third-party location data, SMEs can plug this drain by powering faster and leaner delivery schedules. Similarly, demand planning and order fulfilment will get a big boost as SMEs learn how to forecast accurately through machine learning models, thus reducing the waste that entails unused, unsold and unutilised inventory.
• Marketing and sales: Multiple SMEs tend to go under because they take on much large corporations with massive sales and marketing budgets. AI can help these startups level the playing field. By using data from each prospect interactions as well as leveraging emerging breakthroughs in the field of programmatic advertising, AI can help fine-tune the marketing programs of startups and help deliver better ROI on their spend. Similarly, through an improved understanding of their territory, AI can provide laser-guided focus to sales people on which prospects to focus on and what approaches can deliver the best results.
• Customer service: Where large enterprises can afford to outsource customer service operations or even bring them in-house, SMEs do not have these advantages. In today’s environment, customers are shown to be more loyal when provided with a superior customer experience. AI can bridge the gap between customer expectations and the constrained budgets available to provide those. With intelligent assistants, SMEs can navigate common questions and complaints put forth by customers and provide a superior customer service at much lowered costs of delivery.
• Talent acquisition: SMEs often have vastly varying needs for talent. For instance, those that are on a strong growth trajectory need to staff their companies rapidly before the competitive advantage they offer slips. Those that are on a slower curve also need to make sure that they hire candidates with the right mix of experience and attitudinal attributes to ensure the smooth functioning of their business.
AI can help reduce the time taken to identify the right candidates by rapidly screening resumes to identify the best fit for the needs of the business. Further, with the right data and training, AI can also administer relevant tests to candidates and grade their performance, thus reducing the requirement of human intervention and time taken to screen good candidates.
Getting Started
Let us look at some of the key factors that business leaders need to keep in mind as they get started on their AI journey.
• AI starts with data: The first consideration before planning an AI intervention is to understand whether high quality data is available for AI to work its magic. Without the right data sets, even the best algorithms can go awry. It is essential that business leaders ensure that their data repositories are sufficiently rich to get started on the AI journey.
• Identify the right problems: SMEs tend to be inundated with multiple issues of burning importance. It can be very enticing for business leaders to assume that AI is the panacea for all problems. That is not the case. Business leaders need to identify the right problem statements where AI can make a demonstrable impact and prioritise use cases that can be solved through AI. Scan the market for best practices and learn from peers to better understand what AI can do and what measurable benefit you can derive from AI-led interventions
• Set success benchmarks: For AI leaders, it is important to set a marker for the right expectations with business leaders. Hence, for the business to see continuous improvement in the results delivered by AI, it is critical to identify the right set of business metrics and expected performance against each of those.
Artificial Intelligence today has gone well beyond experimentation to now becoming a real game-changer in how businesses operate. AI can bring significant benefits to startups with improved efficiencies and faster operations. SME leaders looking for strong competitive advantages with respect to their peers would do well to harness the power of this technology and infuse it into their key business process to accelerate outcomes and grow their businesses.
Related Posts
AIQRATIONS
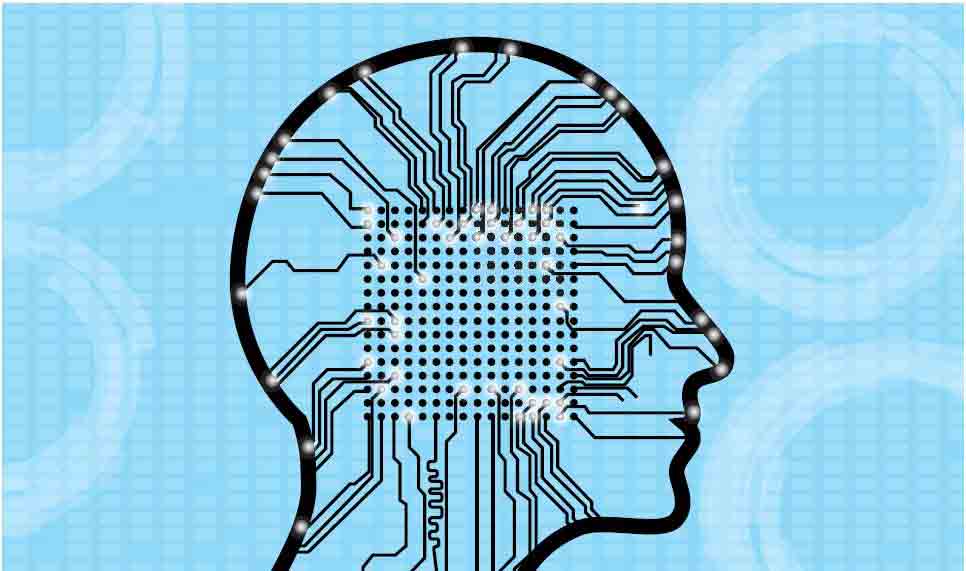
Detecting depression early with AI
Add Your Heading Text Here
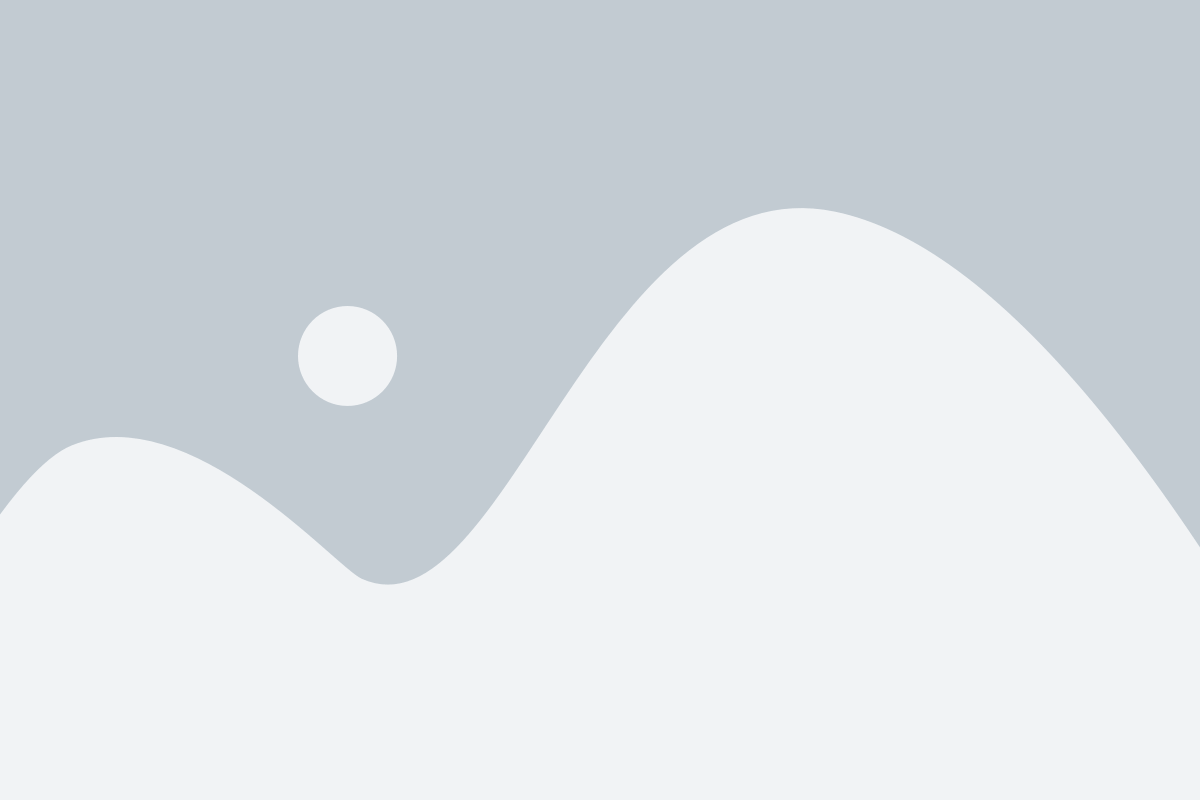
Can artificial intelligence be used in conjunction with mental health practitioners to better discover and address cases of depression and other mental illnesses? We have seen impactful examples of AI being applied to a range of health and wellness use cases, with the potential to help improve the lives of millions of people. Recent advances in this breakthrough technology have also shown the great promise that AI holds in helping individuals and doctors better manage cases and mitigate adverse health impacts of depression and other illnesses that plague the human mind.
Depression is a key problem faced by humans across the world — from milder forms of repetitive depressive mood states to more deeper-seated clinical depression. While we have seen significant improvements in our theoretical understanding of clinical depression, its causes and how to effectively mitigate it, the problem continues to affect millions of people. Psychiatric conditions such as this do not have vaccines or proven preventive medication. So much of the success in helping those with clinical depression hinges on early detection, continuous monitoring and scientific counselling and – in certain cases with a physician’s prescription – medication.
Health Data
At the macro-level, the downside of not managing clinical depression, especially for big populations, can be devastating. According to a study in 2015, it is estimated that approximately 216 million individuals across the globe (3% of total global population) have been affected with clinical depression – with a usual onset in the 20s and 30s. A lack of concerted focus on overall mental health and well-being can lead to outcomes as diverse as high suicide and crime rates to a reduction in national productivity and high levels of substance abuse and addiction.
Today we have a huge repository of health data. By applying machine learning and deep learning algorithms, AI can help individuals and physicians detect early indications of the onset of depression, monitor the progress of patients using medical and other therapeutic options and provide continuous support.
Early Detection
By using AI to understand and highlight speech patterns, use of specific words in text communication and even facial expression, we could possibly improve the speed at which such cases are uncovered. For achieving this, it is important that doctors and machine learning experts work together to identify patterns that have a strong correlation with clinical depression.
A research led by Dr Fei-Fei Li of Stanford University found that using facial and speech recognition data and algorithmic models, it might be possible to detect cases where there is an onset of clinical depression. By leveraging a combination of facial expressions, voice intonation and spoken words, their research was able to diagnose if an individual was suffering from depression with 80% accuracy.
Facebook too has begun piloting its own machine learning algorithm to go through posts to identify linguistic red flags that are indicative of depression. In early testing, the algorithm was said to perform just as well as existing questionnaires that are used to identify depression.
Therapist Interaction
Can AI inform therapists and help them do their job better? With the high and growing number of mental illnesses, the limited number of therapists need AI interventions to help make their job easier while helping improve health outcomes. AI could change the game in the therapy arena by augmenting therapists in identifying subtle signs showcased by patients – such as intonations in voice and facial expressions – which therapists might sometimes miss. Further, AI can also provide guided suggestions to them in providing effective treatment options.
An interesting innovation in this area comes from Ginger. This technology company combines a strong clinician network with machine learning to provide patients with the emotional support that they need at the right time – offering 24/7 availability for cognitive behaviour therapy (CBT), alongside mindfulness and resilience training.
On the back-end, AI is using each patient’s progress to inform its capabilities and make it smarter and more scalable. AI also helps Ginger match its users with a team of three emotional support coaches that it determines to be best suited for the individual.
Continuous Support
The stigma associated with mental illnesses often acts as a bottleneck in uncovering which individuals are suffering from it. Having a non-human companion that can provide human-like compassion can be a possible game changer in helping counsel patients suffering from depression and help them in the rehabilitation process. There are also advantages associated with AI being that counsellor – as it offers higher availability at a much lower cost.
Woebot is an excellent example and a bellwether for what AI could accomplish in the future for this use case. Created by Dr Alison Darcy, Woebot is a computer program that can be integrated with Facebook and is aimed at replicating the typical conversation a patient would have with a therapist. The tool asks about a patient’s mood and thoughts and offers evidence-based CBT. Just like a therapist would in a real-life situation, Woebot ‘listens’ actively in a conversation and learns about the patient to offer interactions more tailored to an individual’s unique situation.
Last year, researchers from the Massachusetts Institute of Technology presented a paper detailing a neural-network model that can be run over raw text and audio data to discover speech patterns that could be indicative of depression, without the need for a pre-set questionnaire. This immense breakthrough will hopefully ripple into more similar research, which could help aid those who suffer from cognitive conditions such as dementia.
While the potential for AI in the field of mental health is massive, it is also imperative that we consider the aspect of protecting the privacy and identity of those included in studies pertaining to this domain. Maintaining data security norms is an extremely crucial part of any AI exercise and in this case, it is even more paramount. To protect the sanctity of AI interventions and participating patients providing the data, it is extremely important to address concerns around data and information security first, before addressing any future use cases in this technology.
Related Posts
AIQRATIONS
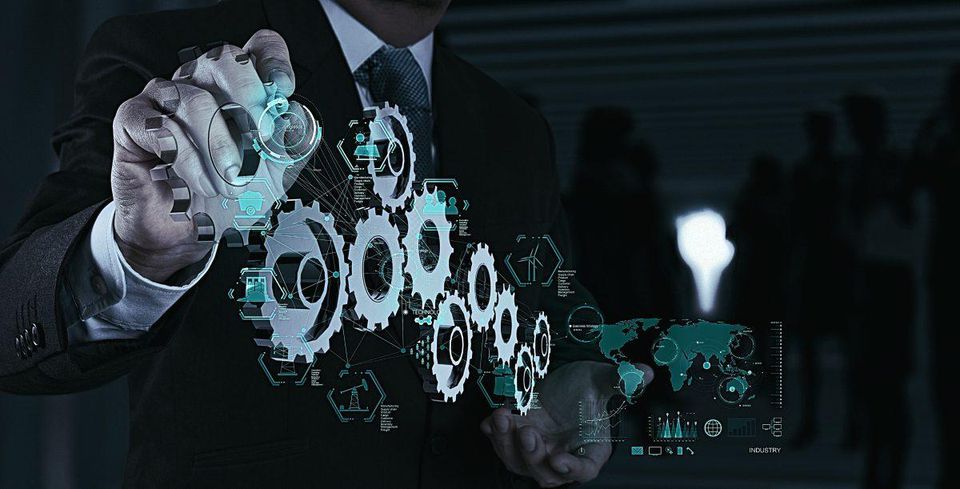
Reimagining Strategic Management Theories And Models With Artificial Intelligence
Add Your Heading Text Here
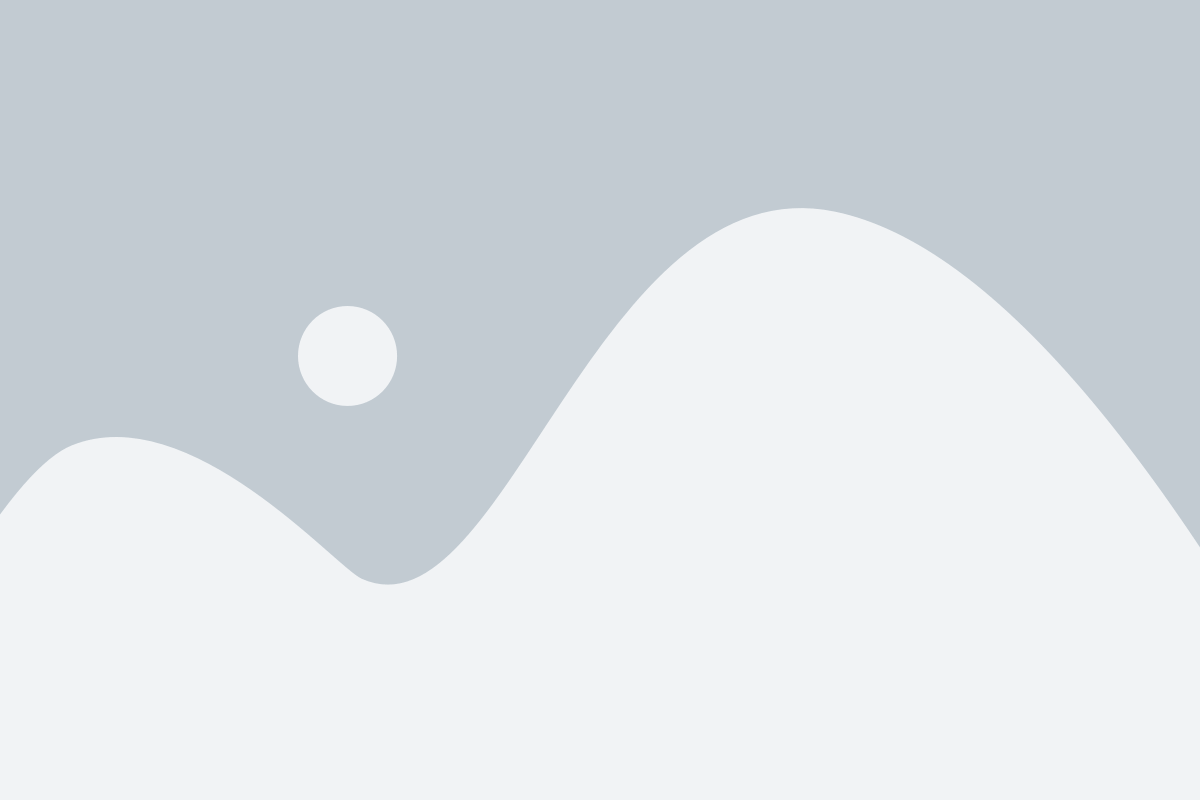
The advent of Artificial Intelligence in the corporate world is disrupting existing business processes and changing the way organizations are run. AI is fast becoming a cornerstone of how businesses manage their bottom line, while opening new revenue streams that could provide a boost to their toplines as well. Given the scale of its impact, there is no doubt that AI will also have a severe impact on the science that governs how organizations are run today.
I am obviously referring to incumbent management theories and models that govern modern organizational management. In classic terms, management theories are frameworks of wisdom which guide the decisions made by organizational leaders that have survived phenomenally well over the period of the modern enterprise. Sure, there have been reasons to fine-tune each one to the realities of each era and industry, but the core construct has been omnipresent through the years.
With AI’s entry into the mainstream of business, management theories may need to be re-evaluated and tweaked appropriately. While the core construct remains powerfully relevant, an injection of the new-age reality of AI will help managers and business leaders apply them in a more contemporary manner on a few theories and models that are being redefined by AI.
Porter’s Five Forces
The theory of the Five Competitive Forces put forth by Michael Porter in 1979 is one of the marquee and evergreen theories in management thought schools. Michael Porter suggests that organizations looking for an understanding of their competitor environment need to consider the impact from five perspectives and work on reducing the risks associated: 1) Threat of new entrants, 2) Threat of Substitutes, 3) Bargaining Power of Customers, 4) Bargaining Power of Suppliers and 5) Intra-industry Rivalry. The construct of this theory is that when businesses need to evaluate the competitiveness (or for that matter, the probability of success) in a business or an industry, they need to keep in consideration these five levers that determine an industry’s attractiveness.
With AI now entering the fray, it is time to reimagine our understanding of Porter’s theory. Specifically, when it comes to the threat of new entrants. Over the years, AI has levelled the playing field as a secret sauce, moving even the most established incumbents from their positions in traditional industries. One must look at how AI is fuelling Amazon’s massive growth – which has hugely disrupted the traditional retail industry. Amazon uses AI in a variety of ways – from identifying the next likely purchase to piloting drone-based deliveries. It was no surprise when Amazon’s announcement last year that it will be entering the healthcare industry led to a tumble in the share price of traditional healthcare companies. AI puts enterprises in a pole position and organizations that harness its’ power correctly stand to gain huge ground over those that do not.
Elton Mayo’s Human Relations Theory
Elton Mayo’s landmark research in the field of organizational productivity comes from his studies in the 1920s at Hawthorne plants in Chicago. In seeking to answer questions around how to improve human productivity, he and his assistants tried tinkering with multiple variables that might have an impact on the quality of the labour force’s work – such as light, duration of breaks and duration of working hours. After all these variables proved inconclusive on how to uplift worker productivity, Mayo finally hit upon his hypothesis i.e. giving attention to employees is what truly resulted in improved performances. Giving your workers a voice in the decision-making process, an experience of greater freedom and autonomy and considering the inherent social needs of people – is the most critical lever in the productivity puzzle.
Enter Artificial Intelligence. With AI taking away much of the scud work involved in managing the varied bureaucracies inherent in organizations, leaders will find a lot more time in managing the performance of its most valued asset – human talent. By simplifying routine and repetitive processes for leadership and the people, we can afford to pay much more attention to the well-being of our human talent, celebrate successes and course-correct flagging performances – with the much-needed human(e) touch.
Total Quality Management (TQM)
Many models and theories surround the overall framework for TQM (Total Quality Management) – a science that owes much of its early evolution to manufacturing techniques originating in Japan. At its very essence, TQM is the science that governs the quality in the manufacturing process. It relates to the adherence of manufactured products with agreed specifications, evolved keeping in mind the needs of the end user. TQM bridges multiple concepts – from customer centricity, lowering the waste in manufacturing processes with a view to increasing the overall quality of the manufacturing output.
The theories surrounding this domain may also be due for a revamp. TQM has long been a data-driven process – relying heavily on a post-mortem understanding of evidence-based decision-making and process improvement. With AI in the picture, organizations can improve predictions around off-specified products earlier, leading to a quantum leap in manufacturing quality. AI is also helping improve the forecasting process, thus reducing the waste created through unused, unsold inventory. Similarly, AI will reduce the overhead associated with identifying anomalous manufacturing conditions and provision for predictive machine maintenance as well to keep up the quality standards in manufacturing activity.
The Future of Organizational Management
The defining case for AI to changing existing models and theories of management boils down to the need for creating a blended workforce comprising both humans and machines. Management science today is largely rooted in building more efficient and agile organizations for humans. In the future, humans and AI will work side-by-side to achieve shared organizational goals. This means that AI will help remove a lot of administrative work that often throttles the productivity of leaders – and allow them to direct their energies towards more complex, judgement driven work that requires them to think creatively. Intelligent machines will soon be considered by the workforce to be ‘colleagues’ and the evolution of management thought needs to account for policies and systems that make the most out of this hybrid workforce.
In conclusion, infusing AI will make business more human centric. Ironic as it may sound, putting AI in charge of the day-to-day, routinized activities will lead to more time for compassionate interactions between humans and unleash human creativity in a huge way. New management theories and models that emerge in the future will hence need to account for the impact of AI – and help organizations and their leaders understand how to navigate this new normal in business.
Related Posts
AIQRATIONS

The New Age Enterprise – Enabled by AI
Add Your Heading Text Here
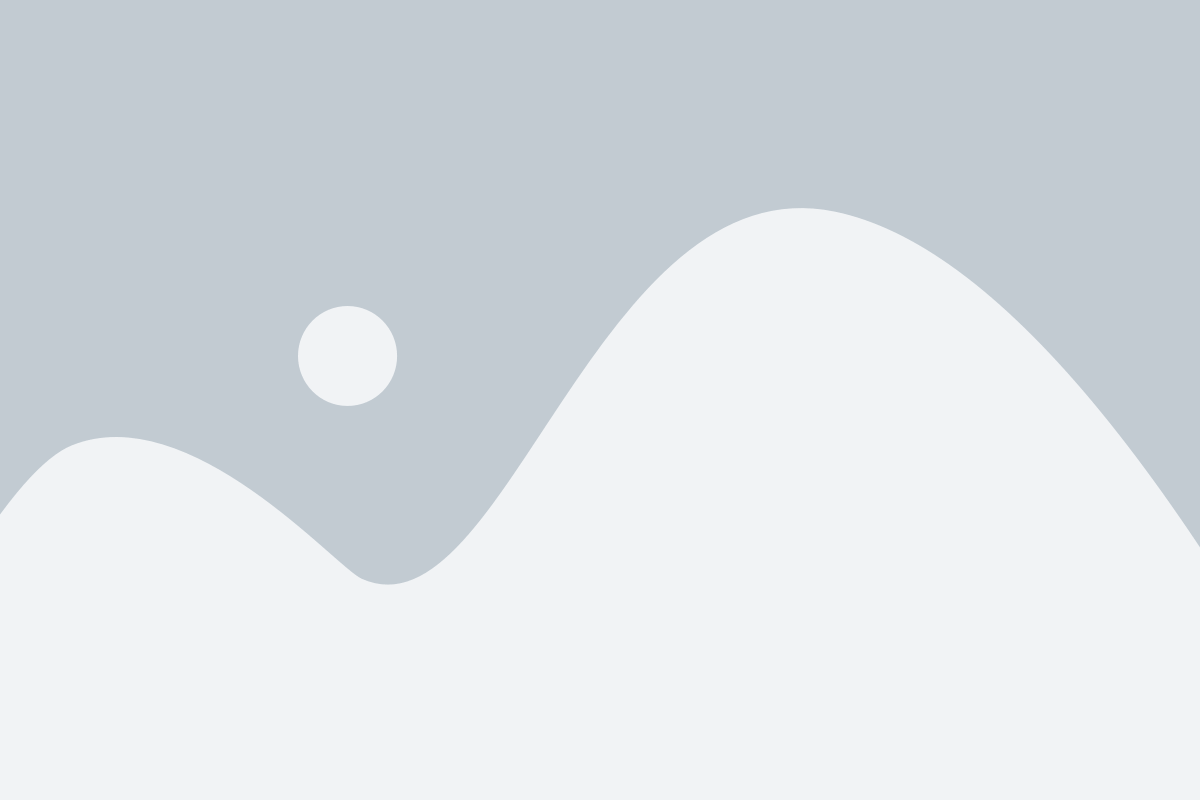
The excitement around artificial intelligence is palpable. It seems that not a day goes by without one of the giants in the industry coming out with a breakthrough application of this technology, or a new nuance is added to the overall body of knowledge. Horizontal and industry-specific use cases of AI abound and there is always something exciting around the corner every single day.
However, with the keen interest from global leaders of multinational corporations, the conversation is shifting towards having a strategic agenda for AI in the enterprise. Business heads are less interested in topical experiments and minuscule productivity gains made in the short term. They are more keen to understand the impact of AI in their areas of work from a long-term standpoint. Perhaps the most important question that they want to see answered is – what will my new AI-enabled enterprise look like?
The question is as strategic as it is pertinent. For business leaders, the most important issues are – improving shareholder returns and ensuring a productive workforce – as part of running a sustainable, future-ready business. Artificial intelligence may be the breakout technology of our time, but business leaders are more occupied with trying to understand just how this technology can usher in a new era of their business, how it is expected to upend existing business value chains, unlock new revenue streams, and deliver improved efficiencies in cost outlays. In this article, let us try to answer these questions.
AI is Disrupting Existing Value Chains
Ever since Michael Porter first expounded on the concept in his best-selling book, Competitive Advantage: Creating and Sustaining Superior Performance, the concept of the value chain has gained great currency in the minds of business leaders globally. The idea behind the value chain was to map out the interlinkages between the primary activities that work together to conceptualize and bring a product / service to market (R&D, manufacturing, supply chain, marketing, etc.), as well as the role played by support activities performed by other internal functions (finance, HR, IT etc.). Strategy leaders globally leverage the concept of value chains to improve business planning, identify new possibilities for improving business efficiency and exploit potential areas for new growth.
Now with AI entering the fray, we might see new vistas in the existing value chains of multinational corporations. For instance:
- Manufacturing is becoming heavily augmented by artificial intelligence and robotics. We are seeing these technologies getting a stronger foothold across processes requiring increasing sophistication. Business leaders need to now seriously consider workforce planning for a labor force that consists both human and artificial workers at their manufacturing units. Due attention should also be paid in ensuring that both coexist in a symbiotic and complementary manner.
- Logistics and Delivery are two other areas where we are seeing a steady growth in the use of artificial intelligence. Demand planning and fulfilment through AI has already reached a high level of sophistication at most retailers. Now Amazon – which handles some of the largest and most complex logistics networks in the world – is in advanced stages of bringing in unmanned aerial vehicles (drones) for deliveries through their Amazon Prime Air program. Business leaders expect outcomes to range from increased customer satisfaction (through faster deliveries) and reduction in costs for the delivery process.
- Marketing and Sales are constantly on the forefront for some of the most exciting inventions in AI. One of the most recent and evolved applications of AI is Reactful. A tool developed for eCommerce properties, Reactful helps drive better customer conversions by analyzing the clickstream and digital footprints of people who are on web properties and persuades them into making a purchase. Business leaders need to explore new ideas such as this that can help drive meaningful engagement and top line growth through these new AI-powered tools.
AI is Enabling New Revenue Streams
The second way business leaders are thinking strategically around AI is for its potential to unlock new sources of revenue. Earlier, functions such as internal IT were seen as a cost center. In today’s world, due to the cost and competitive pressure, areas of the business which were traditionally considered to be cost centers are require to reinvent themselves into revenue and profit centers. The expectation from AI is no different. There is a need to justify the investments made in this technology – and find a way for it to unlock new streams of revenue in traditional organizations. Here are two key ways in which business leaders can monetize AI:
- Indirect Monetization is one of the forms of leveraging AI to unlock new revenue streams. It involves embedding AI into traditional business processes with a focus on driving increased revenue. We hear of multiple companies from Amazon to Google that use AI-powered recommendation engines to drive incremental revenue through intelligent recommendations and smarter bundling. The action item for business leaders is to engage stakeholders across the enterprise to identify areas where AI can be deeply ingrained within tech properties to drive incremental revenue.
- Direct Monetization involves directly adding AI as a feature to existing offerings. Examples abound in this area – from Salesforce bringing in Einstein into their platform as an AI-centric service to cloud infrastructure providers such as Amazon and Microsoft adding AI capabilities into their cloud offerings. Business leaders should brainstorm about how AI augments their core value proposition and how it can be added into their existing product stack.
AI is Bringing Improved Efficiencies
The third critical intervention for a new AI-enabled enterprise is bringing to the fore a more cost-effective business. Numerous topical and early-stage experiments with AI have brought interesting success for reducing the total cost of doing business. Now is the time to create a strategic roadmap for these efficiency-led interventions and quantitatively measure their impact to business. Some food for thought for business leaders include:
- Supply Chain Optimization is an area that is ripe for AI-led disruption. With increasing varieties of products and categories and new virtual retailers arriving on the scene, there is a need for companies to reduce their outlay on the network that procures and delivers goods to consumers. One example of AI augmenting the supply chain function comes from Evertracker – a Hamburg-based startup. By leveraging IOT sensors and AI, they help their customers identify weaknesses such as delays and possible shortages early, basing their analysis on internal and external data. Business leaders should scout for solutions such as these that rely on data to identify possible tweaks in the supply chain network that can unlock savings for their enterprises.
- Human Resources is another area where AI-centric solutions can be extremely valuable to drive down the turnaround time for talent acquisition. One such solution is developed by Recualizer – which reduces the need for HR staff to scan through each job application individually. With this tool, talent acquisition teams need to first determine the framework conditions for a job on offer, while leaving the creation of assessment tasks to the artificial intelligence system. The system then communicates the evaluation results and recommends the most suitable candidates for further interview rounds. Business leaders should identify such game-changing solutions that can make their recruitment much more streamlined – especially if they receive a high number of applications.
- The Customer Experience arena also throws up very exciting AI use cases. We have now gone well beyond just bots answering frequently asked questions. Today, AI-enabled systems can also provide personalized guidance to customers that can help organizations level-up on their customer experience, while maintaining a lower cost of delivering that experience. Booking.com is a case in point. Their chatbot helps customers identify interesting activities and events that they can avail of at their travel destinations. Business leaders should explore such applications that provide the double advantage of improving customer experience, while maintaining strong bottom-line performance.
The possibilities for the new AI-enabled enterprises are as exciting as they are varied. The ideas shared in this article are by no means exhaustive, but hopefully seed in interesting ideas for powering improved business performance. Strategy leaders and business heads need to consider how their AI-led businesses can help disrupt their existing value chains for the better, and unlock new ideas for improving bottom-line and top-line performance. This will usher in a new era of the enterprise, enabled by AI.
Related Posts
AIQRATIONS
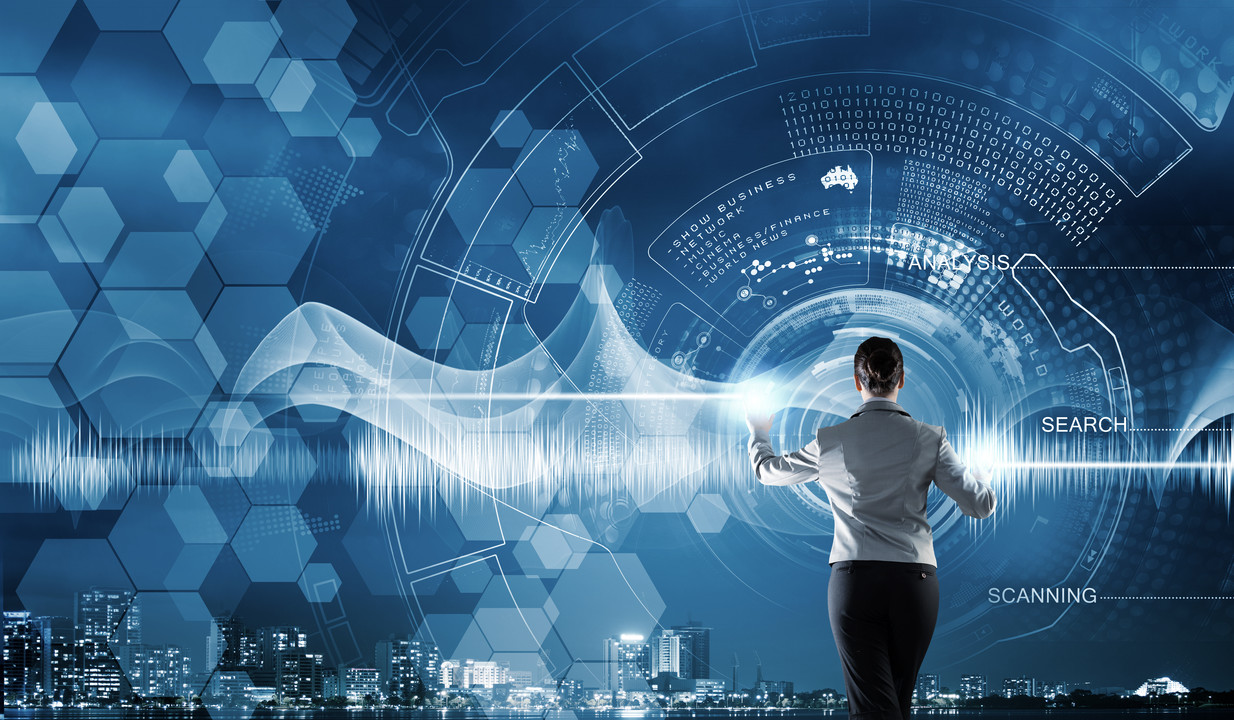
Top 10 Exponential Technologies Trends – 2019
Add Your Heading Text Here
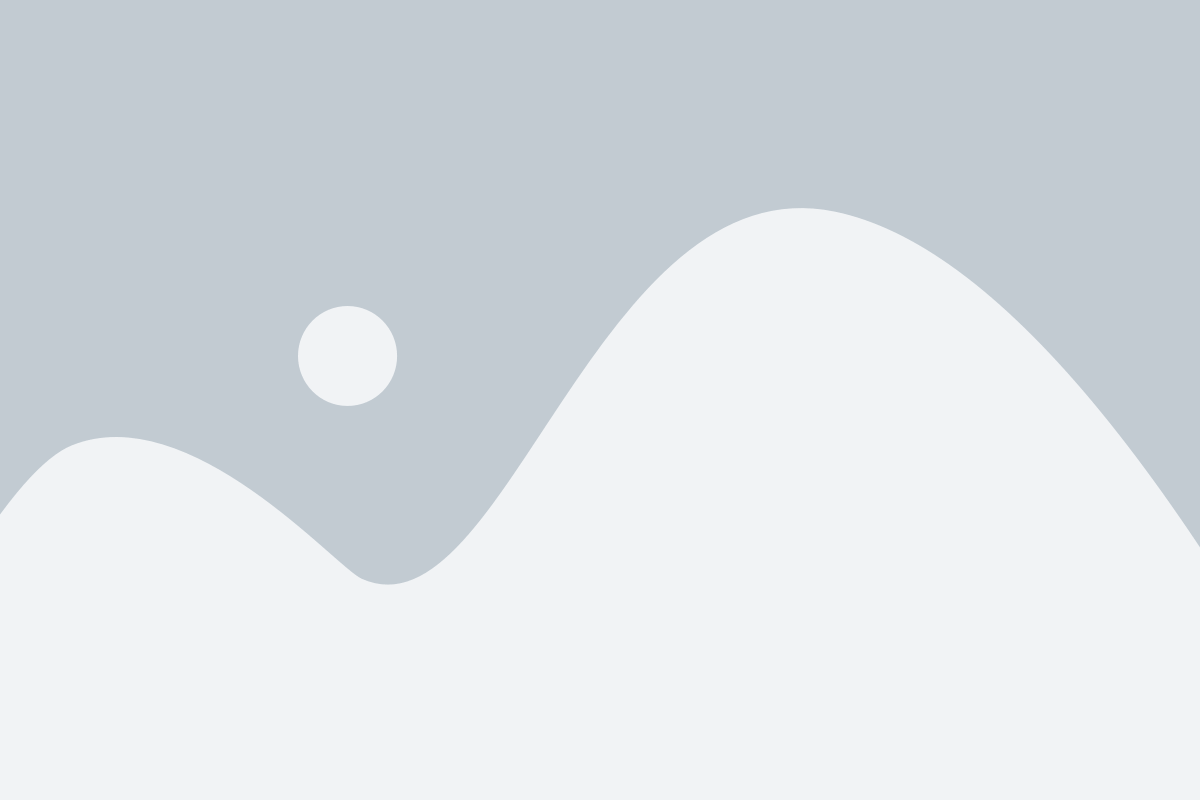
Across the world of technology, we are seeing the proliferation of new age developments across software and hardware – titled “Exponential Technologies”. The term refers to a wide range of recent technology breakthroughs – Artificial Intelligence, Internet of Things, Cloud Computing, Augmented and Virtual Reality, Blockchain and the allied. They are collectively referred to as ‘exponential’ considering the humungous potential value that they could possibly add to business. As these technologies continue to mature in their development and adoption, the world is gaining a more concrete insight into the worth of these technologies and their use cases. 2019 will most certainly be the year where these technologies will go mainstream – and deliver exponential value to their proponents. With high investor interest (and money) riding on these new age technologies, I am confident that in 2019, there will be a high uptake in their commercialization. Here are the top10 trends that I foresee in 2019 in exponential technologies :
1. Blockchain Beyond the Hype
In 2018, there was no doubt a lot of excitement and buzz as technology vendors and investors started investigating blockchain and cryptocurrency. In 2019, expect blockchain to move beyond the hype and enter the mainstream. Gartner estimates that blockchain applications will create $3.1 trillion in business value by 2030. Over 2018, several tech-savvy businesses started their own experiments with blockchain in areas such as supply chain, which is ripe for a blockchain-powered disruption. Within blockchain, I foresee:
Increased collaboration between businesses and tech vendors to unlock the power of blockchain across multiple use cases. Given its immutable and decentralized nature, blockchain will be invaluable in sectors such as manufacturing, defense and financial services – and we will see innovative use cases coming out of these domains
Within blockchain, smart contracts specifically will gain immense traction. The business value of smart contracts is remarkably clear – they drastically reduce the time and effort for routine but lengthy paperwork processes, while maintaining the sanctity through a blockchain network
Due to the numerous crypto frauds seen uncovered in the last year, more and more sovereign governments will push legislation to regulate and establish clear rules around blockchain and cryptocurrency. I have no doubts that this will have a net positive impact – as it will demonstrably improve the consumer confidence and enterprise adoption for these technologies by laying down a clear legal framework for their use
2. 3rd Platform Technology to Accelerate Digital Transformation
A combination of social, mobile, data-driven decision-making and cloud infrastructure and processing is commonly referred to today as 3rd platform technology. In 2019, there will be no stopping the juggernaut of internal IT departments moving ever faster towards digital technology.
According to a research by IDC, it is expected that by 2023, 75% of all IT spending will be on such 3rd platform technology, with over 90% of all enterprises building “digital native” IT environments
Further advanced technologies such as distributed cloud, hyperagile app technologies and architectures, AI at the edge and AI-powered voice UIs will be central to how enterprises enable digital transformation using 3rd platform technologies.
This expansion in demand for 3rd platform technologies will be the outcome on increasing pressures on internal IT to become profit centers and unlocking new sources of revenue for the parent enterprise. Using easily scalable and replicable digital frameworks, early adopter IT departments would be able to commercialize this technologies to their competitors while giving their businesses critical competitive advantage
3. Quantum Computing to Come of Age
Quantum computing is a non-traditional form of computing operating on the quantum state of subatomic particles and representing information as elements denoted through quantum bits. The unmitigated rise in the development and permeation of quantum computing is the third key trend that I see for 2019. It is estimated that by 2023, 20% of organizations will carve out budgets for quantum computing projects, as opposed to less than 1% today.
With heavier software paradigms such as Internet of Things, Artificial Intelligence and blockchain achieving mainstream status, there will be large scale demand for quantum computing to come out of the shadows of academia and into business. Quantum computing will move well beyond a buzzword and will be part of multiple projects at an experimental scale at corporations.
Quantum Computing will succeed where traditional computing has failed, providing parallel execution and exponential scalability. Such systems will take on problems too complex for a traditional approach or where the latency for traditional algorithms would be untenable
Business leaders across multiple industries – automotive, financial, insurance, pharmaceuticals, military and research organizations – will see massive gains through the advancements in Quantum Computing .
4.Acceleration in the Pervasiveness of the Internet of Things
While Internet of Things has demonstrably hit mainstream status across industries such as consumer goods and retail, and use cases such as supply chain and logistics, we will see further acceleration in its adoption in 2019
IOT-enabled hardware devices will proliferate nearly all walks of human life. Devices from sensors, wearables, smart assistants and wearables will be a feature in everyday life for most individuals in the developed world and will be a key focus for powering digital transformation
With increasing demand for IOT-powered devices across use cases will definitively bring endpoint security into focus for enterprises. As IOT devices become the first frontier for communication with consumers through highly sensorized environments, we will see a rapid escalation in the adoption of endpoint security practices and software
To support this deep network of the Internet of Things will require an immediate focus on rapidly enabling 5G connectivity in 2019. Not having a robust underlying infrastructure to support IOT will be disastrous for businesses and individuals who will be highly reliant on it for their day-to-day activity.
5. Convergence of AI, Blockchain, Cloud and IO
Could a future software stack comprise AI, Blockchain and IOT running on the cloud? It is not too hard to imagine how these exponential technologies can come together to create great value. In 2019, I expect that we will see a strong spread of use cases that effectively combine these technologies.
Internet of Things devices will largely be the interface with which consumers and other societal stakeholder will interact. Voice-enabled and always connected devices – such as Google Home and Amazon’s Alexa will augment the customer experience and eventually become the primary point of contact with businesses
Artificial Intelligence frameworks such as Speech Recognition and Natural Language Processing are making huge advances. These will be the translation layer between the sensor on one end and the deciphering technology on the other end
Blockchain-like decentralized databases will act as the immutable core for managing contracts, consumer requests and transactions between various parties in the supply chain
Cloud will be the mainstay for running these applications requiring huge computational resources and very high availability. I expect more cloud vendors to come forward (Amazon and Google for instance already have) with specialized cloud frameworks to handle the torrent of requests that these type of applications would require.
6.New UI/UX Interfaces to Emerge on the Scene
To unlock and harness the true value of exponential technology it is incumbent that we do not rely only on existing paradigms of end-user interfaces such as web and mobile. We need to reinvent new paradigms and explore game changing new interfaces that will help usher better customer and user experiences.
Conversational platforms – ones which are primarily activated through voice and voice-recognition AI will conduct numerous exchanges on behalf of customers. Already we are seeing rapid adoption of conversational interfaces such as Google Home, Amazon Alexa and Apple’s Siri. These will only grow and prominence and entire CX use cases will be centered around these platforms
Virtual Reality (VR), Augmented Reality (AR) and Mixed Reality (MR) will be increasingly leveraged across a vast selection of topical use cases. Incorporating these alongside traditional interfaces will be crucial to delivering the future of an immersive user experience. According to Gartner, we will shift from thinking about individual devices and fragmented user interface (UI) technologies to a multichannel and multimodal experience.
These immersive experience-led interfaces such as VR and AR will become increasingly popular, with 70% of enterprises experimenting with such technology for consumer and enterprise use and 25% of organizations deploying it into production.
7.Edge Computing to become an Enterprise Mandate
Simply put, edge computing is a computing topology in which information processing, and content collection and delivery, are placed closer to these endpoints. For reducing the latency running AI algorithms and eventual response times, edge computing will become an enterprise mandate for use cases involving a convergence of IOT and AI.
In 2019, adoption of edge computing will be driven by the need to keep the processing power close to endpoints as opposed to a centralized cloud server. Having said that, edge computing will not necessitate the creation of a new architecture. Cloud and edge computing will complement each other. Cloud services will be charged with centralized service execution, not only on centralized servers, but also across distributed servers on-premises and on-the-edge devices themselves.
Five years down the line expect to see specialized AI chips, supporting greater processing power, storage and other advanced capabilities. They will be incorporated into a wider array of edge devices. Not too far into the future, we will see 40% of organizations’ cloud deployments include an element of edge computing and 25% of endpoint devices and systems will execute AI algorithms.
We will see more intelligent and empowered edge computing devices as well. According to Gartner, storage, computing and advanced AI and analytics capabilities will expand the capabilities of edge devices through 2028.
8. DevOps Augmented by AI
Despite almost universal acceptance of the DevOps framework across global enterprises, adoption has been patchy and slow. This is due to numerous reasons, ranging from a distributed toolset and a paucity of expert practitioners. However with the emergence of AI, we will see an increased process automation between software development and deployment, accelerating the enablement of DevOps
AI-powered QA suites will increase the automation quotient in the DevOps process. Given the advancements seen in automation, AI will rapidly intervene in the QA process across unit testing, regression testing, functional testing and user acceptance testing.
DevSecOps will combine the power of DevOps and AI in the field of information security. A centralized logging architecture recording suspicious activity and threats combined with ML-based anomaly detection techniques will empower developers to accurately pinpoint potential threats to their system and secure it for the future.
AI will also break the cultural barriers that typically exist between developer and operations teams. . AI-powered systems will enable DevOps teams to have a single, unified view into system issues across a complex toolchain while improving the collective knowledge of anomalies detected and the pathways for redressal.
9.Autonomous Things on the Rise:
At present, we are seeing experiments at an advanced level in the field of autonomous things. Autonomous things comprise whole gamut of unmanned objects – from drones, cars and robots. In 2019, I expect there to be a steady rise in the adoption and appreciation of this area of technology
Autonomous things of today are largely centered around the current paradigm of basic automation and rigid if-else programming rules. The next revolution in the field of autonomous things will be by exploiting the power of AI to exhibit more advanced, proactive and multi-threaded behaviors
Demand for autonomous things will continue to grow, specifically for autonomous vehicles. According to a Gartner survey, by 2021, 10% of new vehicles will have autonomous driving capability, compared to less than 1% in 2017.
Robotics and drones powered by AI will be able to address more complex use cases bringing in further efficiencies to incumbent businesses in the field of logistics delivery, warehouse management and manufacturing
10. AI to Disrupt Cybersecurity
Finally, the last key trend in the exponential technologies space for 2019 pertains to cybersecurity. While this is a remarkably advanced field, we will see continued growth and evolution of cybersecurity in combination with artificial intelligence
Using anomaly detection and machine learning, AI will hugely disrupt the field of cyber security. Security practitioners will be empowered to identify intrusions and malafide behavior faster using automated, always-on algorithms to constantly survey the secured network for wrongful activity and address concerns before they break-ins occur
AI can be quickly training over a massive data set of cyber security, network, and even physical information. Cyber security vendors will soon roll out AI-enabled solutions that will learn at an abstract level to detect and block abnormal behavior, even when this behavior does not fit within a known pattern. I expect that in 2019 companies will incorporate ML into every category of cybersecurity products.
By extension, we will see a fight between good AI and bad AI in the domain of cybersecurity. There are genuine fears that the next generation of attacks will not be carried out by human hackers but pieces of code designed to rapidly infiltrate a secure environment. Countering that with so-called ‘good AI’ will be crucial in undermining the impact these fast-paced attacks can have
Related Posts
AIQRATIONS
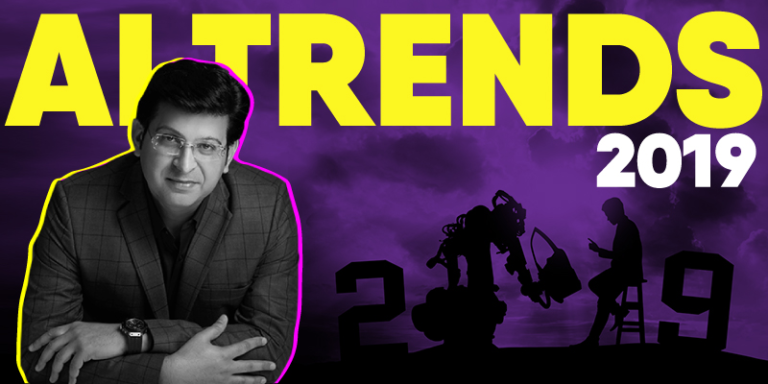
Here are the top 10 AI trends to watch out for in 2019
Add Your Heading Text Here
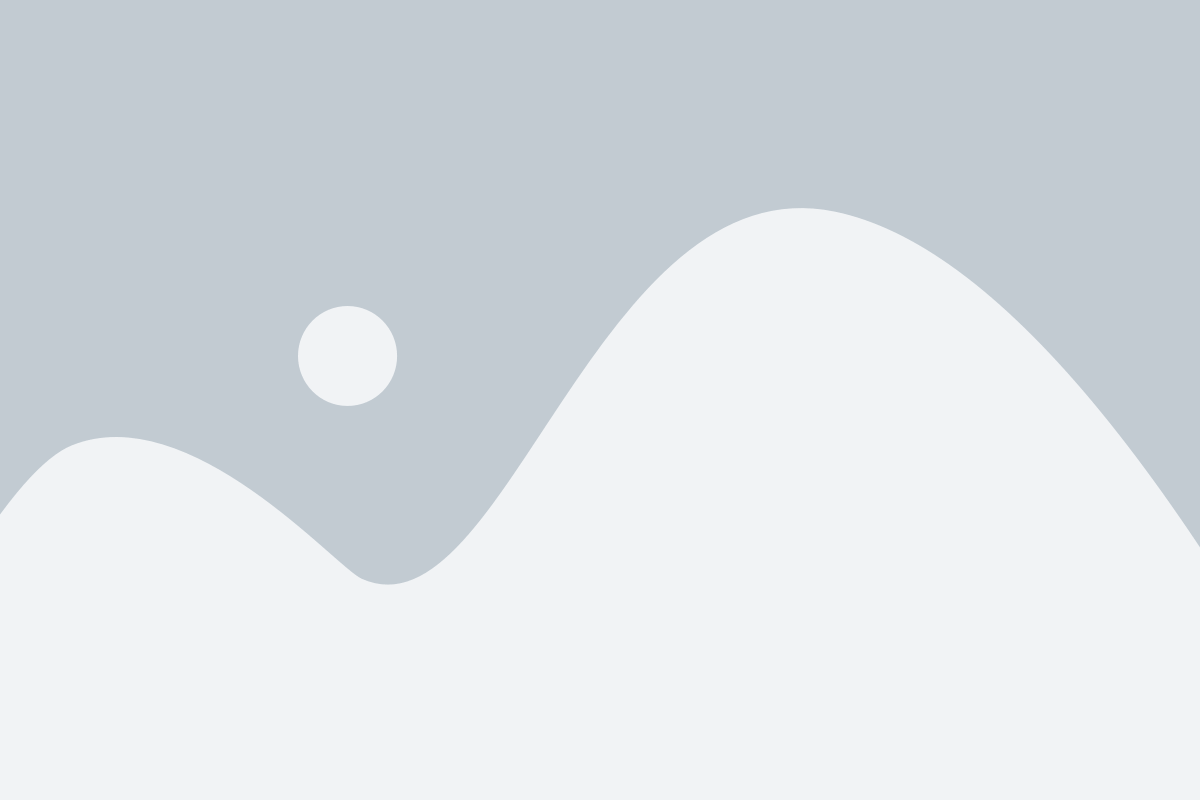
The year 2018 will be remembered as the year that artificial intelligence stopped being on the periphery of business and entered the mainstream realm. With increasing awareness and capability of AI among the numerous stakeholders, including tech buyers, vendors, investors, governments, and academia, I expect AI will go beyond just tinkering and experiments and will become the mainstay in the business arena.
With an increasing percentage of these stakeholders professing their commitment to leveraging this technology within their organisations, AI has arrived on the world scene. We are sure to see transformative business value being derived through AI in the coming years. As we come to the close of 2018, let us gaze into the crystal ball to see what 2019 will hold for this game-changing technology:
The rise of topical business applications
Currently, we have a lot of general purposes AI frameworks such as Machine Learning and Deep Learning that are being used by corporations for a plethora of use cases. We will see a further evolution of such technology into niche, topical business applications as the demand for pre-packaged software with lower time-to-value increases. We will see a migration from the traditional AI services paradigm to very specific out-of-the-box applications geared to serve particular use cases. Topical AI applications in this space that serve such use cases will be monumentally useful for furthering the growth of AI, rather than bespoke services that require longer development cycles and may cause bottlenecks that enterprises cannot afford.
The merger of AI, Blockchain, cloud, and IoT
Could a future software stack comprise AI, Blockchain, and IoT running on the cloud? It is not too hard to imagine how these exponential technologies can come together to create great value. IoT devices will largely be the interface with which consumers and other societal stakeholders will interact. Voice-enabled and always connected devices – such as Google Home and Amazon’s Alexa – will augment the customer experience and eventually become the primary point of contact with businesses. AI frameworks such as Speech Recognition and Natural Language Processing will be the translation layer between the sensor on one end and the deciphering technology on the other end. Blockchain-like decentralised databases will act as the immutable core for managing contracts, consumer requests, and transactions between various parties in the supply chain. The cloud will be the mainstay for running these applications, requiring huge computational resources and very high availability.
Focus on business value rather than cost efficiency
2019 will finally be the year that majority of the executive and boardroom conversations around AI will move from reducing headcount and cost efficiency to concrete business value. In 2019, more and more businesses will realise that focusing on AI solutions that reduce cost is a criminal waste of wonderful technology. Ai can be used to identify revenues lost, plug leakages in customer experience, and entirely reinvent business models. I am certain that businesses that focus only on the cost aspect will stand to lose ground to competitors that have a more cogent strategy to take the full advantage of the range of benefits that AI offers.
Development of AI-optimised hardware and software
Ubiquitous and all-pervasive availability of AI will require paradigm shifts in the design of the hardware and software that runs it. In 2019, we will see an explosion of hardware and software designed and optimised to run artificial intelligence. With the increasing size and scale of data fueling AI applications and even more complex algorithms, we will see a huge demand for specialised chipsets that can effectively run AI applications with minimal latency. Investors are showing heavy interest in companies developing GPUs, NPUs, and the like – as demonstrated by Chinese startup Cambricon, which stands valued at a whopping $2.5 billion since its last round of funding this year. End-user hardware such as smart assistants and wearables will also see a massive increase in demand. Traditional software paradigms will also continue to be challenged. Today’s novel frameworks such as TensorFlow will become de rigueur. Architectural components such as edge computing will ensure that higher processing power is more locally available to AI-powered applications.
‘Citizen AI’ to be the new normal
One of the reasons we saw widespread adoption of analytics and data-driven decision-making is because we built applications that democratised the power of data. No longer was data stuck in a remote silo, accessible only to the most sophisticated techies. With tools and technology frameworks we brought data into the mainstream and made it the cornerstone of how enterprises plan and execute strategy. According to Gartner, the number of citizen data scientists will grow five times faster than the number of expert data scientists. In 2019, I expect Citizen AI to gain traction as the new normal. Highly advanced AI-powered development environments that automate functional and non-functional aspects of applications will bring forward to a new class of “citizen application developers”, allowing executives to use AI-driven tools to automatically generate new solutions.
Policies to foster and govern AI
Following China’s blockbuster announcement of a National AI Policy in 2017, other countries have rushed to share their take on policy level interventions around AI. I expect to see more countries come forward with their versions of a policy framework for AI – from overarching vision to allaying concerns around ethical breaches. At the same time, countries will also be asked to temper their enthusiasm of widespread data proliferation by releasing their own versions of GDPR-like regulations. For enabling an ecosystem where data can be used to enrich AI algorithms, the public will need to be convinced that this is for the overall good, and they have nothing to fear from potential data misuse and theft.
Speech Recognition will revolutionise NLP
In the last few years, frameworks for Natural Language Understanding (NLU) and Natural Language Generation (NLG) have made huge strides. NLP algorithms are now able to decipher emotions, sarcasm, and figures of speech. Going forward, voice assistants will use data from voice and combine that with deep learning to associate the words spoken with emotions, enriching the overall library that processes speech and text. This will be a revolutionary step forward for fields such as customer service and customer experience where many bots have typically struggled with the customer’s tone of voice and intonation.
The growth of explainable AI
And finally, with numerous decisions powered by AI – and specifically unsupervised learning models – we will see enterprises demand “explainable” AI. In simplified terms, explainable AI helps executives “look under the hood” to understand the “what” and “why” of the decisions and recommendations made by artificial intelligence. Development of explainable AI will be predicated on the need for increased transparency and trust. Explainable AI will be essential to ensure that there is some level of transparency (and potentially, learning) that is gleaned from unsupervised systems.
Convergence of AI and analytics
This is a trend that is a logical consequence of the decisive power of data in business today. In 2019, we will see a merger of analytics and AI – as the one-stop for uncovering and understanding insights from data. With advancements in AI seen so far, the algorithms are more than capable of taking up tasks that involve complex insight generation from multi-source, voluminous data. This convergence of AI and analytics will lead to automation that will improve the speed and accuracy of the decisions that power business planning and strategy. AI-powered forecasting will help deliver faster decisions, with minimal human interventions and create higher cost savings for the business.
Focus on physical and cybersecurity paradigms
Two of the domains ripe for an AI transformation are the fields of physical and cybersecurity. As intrusions into physical and virtual environments become commonplace and threats become hugely pervasive, AI will be a massive boost to how we secure these environments. Advances in fields such as ML-powered anomaly detection will drastically reduce the time required to surface potential intrusions into secure environments. This will enable organisations to better protect user data. When combined with Blockchain, AI will give cybersecurity a huge boost through decentralised, traceable databases containing valuable client and strategic information. On the physical security side, Computer Vision is rapidly gaining currency in the fields of physical intruder detection. Surveillance cameras, originally manned by security guards, will soon be replaced by AI-powered systems that will be able to react faster and more proactively to intruders that pose a threat to physical premises. When you combine that with face recognition, working with a database of known offenders, we will see a quantum drop in the time required to adjudicate and address cases of theft and unauthorised entry by law enforcement agencies.
In summary, the broad directions that I predict AI will take include interventions to make it more embedded, responsible, and explainable; convergence with other exponential technologies such as cloud, Blockchain, and IoT; cybersecurity; a greater proliferation and development of use cases; and great strides in the technology and its supporting infrastructure. Enterprises would do well to adopt this revolutionary technology and ensure a strong availability of talent to conceptualise, develop, and unleash value from AI applications.
Related Posts
AIQRATIONS

3 Ways To Human Centric AI
Add Your Heading Text Here
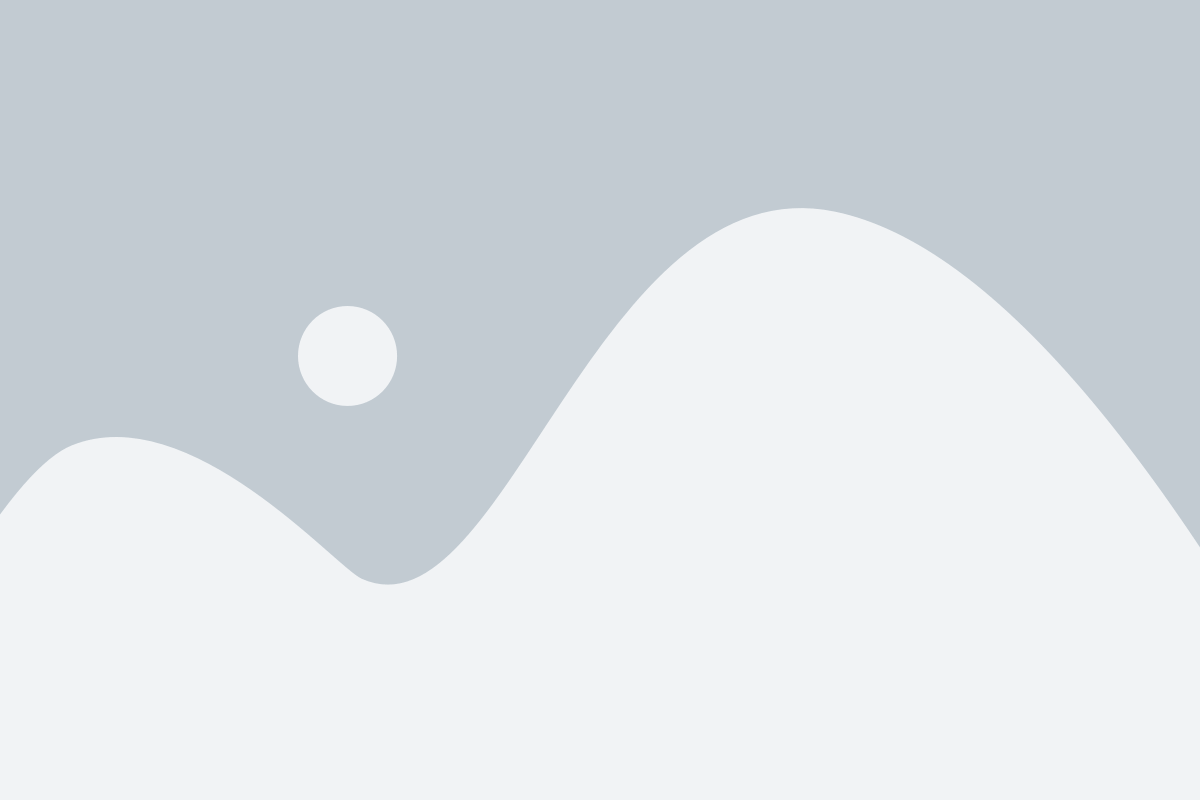
Three Ways to Facilitate a Symbiotic Relationship Between Cognitive Intelligence and Behavioral Sciences
After every conference I speak at about the transformative power of Artificial Intelligence and its potential to unlock untold business value, the one question that often crops up from the audience is.. if AI is expected to perform much of the grunt work in the enterprise world, what scope is there for the so-called ‘human’ qualities?
Is the future of business and technology so deeply intertwined that it leaves virtually no scope in the future for the vagaries of human intelligence and behavior? The answer is quick and simple – absolutely not. Artificial Intelligence, while a great paradigm-shifter in the world of business, is still one of the tools that will be used by humans for making better decisions. At the end of the day, AI will still be developed and used by humans. And maintaining the ‘human element’ in the way it is made, delivered, used and improved will most certainly make it a lot more successful. AI exists to make human life simpler and richer – and hence it is critical that AI practitioners and data scientists adopt a human-centric approach to its development, deployment and adoption. Even the best AI will become quickly redundant without inputs from real humans on how to accelerate strategic decisions and processes.
How do we then build in that ‘human element’ into our Artificial Intelligence tools? This is where humanities-centric subjects of design and behavioral science come into the picture. What is behavioral science? Simply put, it is the study of internal cognitive processes of humans and societies and how these processes manifest into external perceptible and imperceptible actions and interactions. Behavioral science typically stands at a nexus of various subjects – borrowing aspects from sociology, anthropology, psychology and even economics and political science. Its interdisciplinary nature precludes the scale of impact it can have if applied correctly. In technology, and specifically in AI, behavioral science will and should impact how we build, use and interact with technology.
I see primarily three key areas where the symbiosis of AI-led cognitive intelligence systems and behavioral science can unlock massive value for enterprises that marry these two starkly different, but extremely complementary fields of study:
Appeal to the Non-Conscious
We have known for nearly a century now that a large majority of human biases, inferences, preferences and reactions are largely controlled by the dark recesses of our non-conscious brain. For technologists to build successful AI products, that are widely adopted and used they need to reach out inside the non-conscious parts of the human brain and orchestrate responses from there.
In AI technology specifically, user adoption is often the difference between make and break for products. Numerous AI products are mostly informed by the data they gather from human actions and their preferences. This data feeds the algorithms running in the background and makes them more sophisticated to better understand their human overlords. To that end, AI products need to have a strong underpinning in behavioral science, so that they can appeal to the non-conscious and improve adoption.
Take for example the work done by Nir Eyal for his book, ‘Hooked: How to Build Habit Forming Products.’ In the book, Eyal writes about multiple ways in which human subjects get applied to technology development. One of them is the Hook canvas – a loop comprising triggers, actions, rewards and investments – which are the cornerstone features of every addictive software you’ve used – from Facebook to Instagram, Snapchat and YouTube. Another is the idea of using the trinity of emotion, features and incentives – extremely relevant ideas to anyone working on building AI products. Another example comes from Worxogo – an Indian startup that employs behavioral design, neuroscience in tandem with predictive analytics to enhance employee performance through nudges to the non-conscious.
Build with Humans
Not only is AI built to serve humans, it is also built by humans. To that end, it becomes extremely important to consider what emotional triggers help define what we build and how we build it. Again, behavioral science practitioners have a key role to play in order to engage empathy in defining the requirements and going about the development of AI. Learnings from behavioral science can bring to light immeasurably important interventions for how we manage and lead teams, collaborate between a team and across multiple teams – all while maintaining a high level of motivation by appealing to a higher sense of purpose. It is worth examining how something as simple as empathy can be extremely valuable in how we build software. For instance, with improved self-awareness and empathy, developers can feel an intrinsic desire to write cleaner code while maintaining proper documentation. Also, given that AI is largely deployed using the DevOps methodology – empathy can be the difference between whether we can build a trust-based bridge between how we build, deploy and automate releases faster.
Beyond the ‘how’ of AI development, behavioral science can also contribute meaningfully to the ‘what’. Currently a lot of concern around AI is related to ethics – will AI lead to loss of meaningful work for humans? What data privacy issues can rear their head when we deploy large-scale data capture systems to improve our algorithms? We need to move the dial from apathy to empathy in the process of conceptualizing software – and knowledge of behavioral science will undoubtedly help AI practitioners develop more responsible AI.
Artificial Emotional Intelligence
The third key application of behavioral science – and possibly the most game-changing of the lot is – how can we apply behavioral science to make our systems more ‘human’? Is it possible to add a dash of EQ to these high IQ systems?
I certainly think there is a huge scope for developing AI that has a strong human bent. Consider the applications we are building today with AI and robotics – companions for the elderly, coaching apps for autistic children, even something as comparatively mundane is chatbots for customer service. Behavioral science holds the key to achieving the holy grail of how we can better balance the human-machine equation, by infusing human qualities into artificial systems.
To enable this, it is important to know who we are building for and what are their intrinsic and non-conscious needs. Behavioral science holds the clues that can complement AI’s ability to eliminate biases, while serving the emotional needs of humans. For example, StressSense tracks when people are highly stressed and helps them avoid anxious situations. This kind of breakthrough research can help in multiple AI applications, teaching them how to behave with humans, while ensuring a strong impact.
As technology providers and businesses work together to build transformational artificial intelligence systems and data science teams, it is very important to consider the human element. These teams would do well to develop a better understanding of whom the AI is built for and how it is used – through techniques offered by behavioral science. Balancing the human-machine equation and powering a complementary relationship between AI systems and the people who use them necessitates an infusion of behavioral science into the process. Ultimately, for AI to succeed, we need both – the foresight of technology as well as the insight of humans.
Related Posts
AIQRATIONS
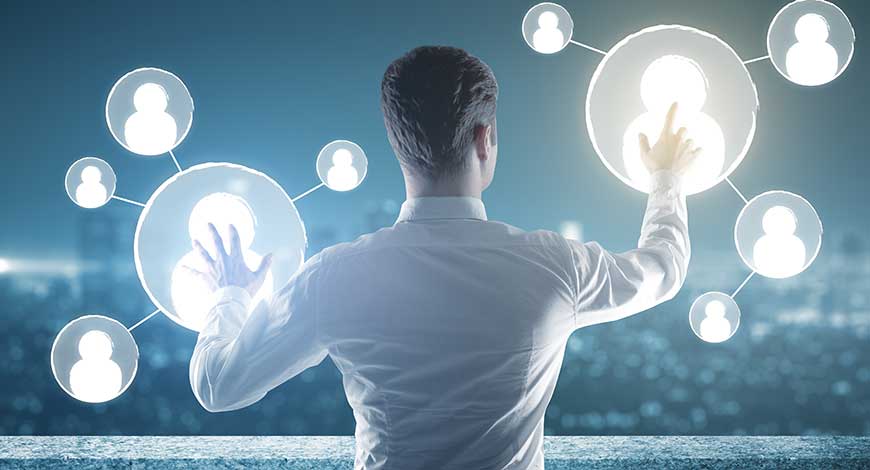
Reimagining Executive Education Programs In The Industry 4.0 Era
Add Your Heading Text Here
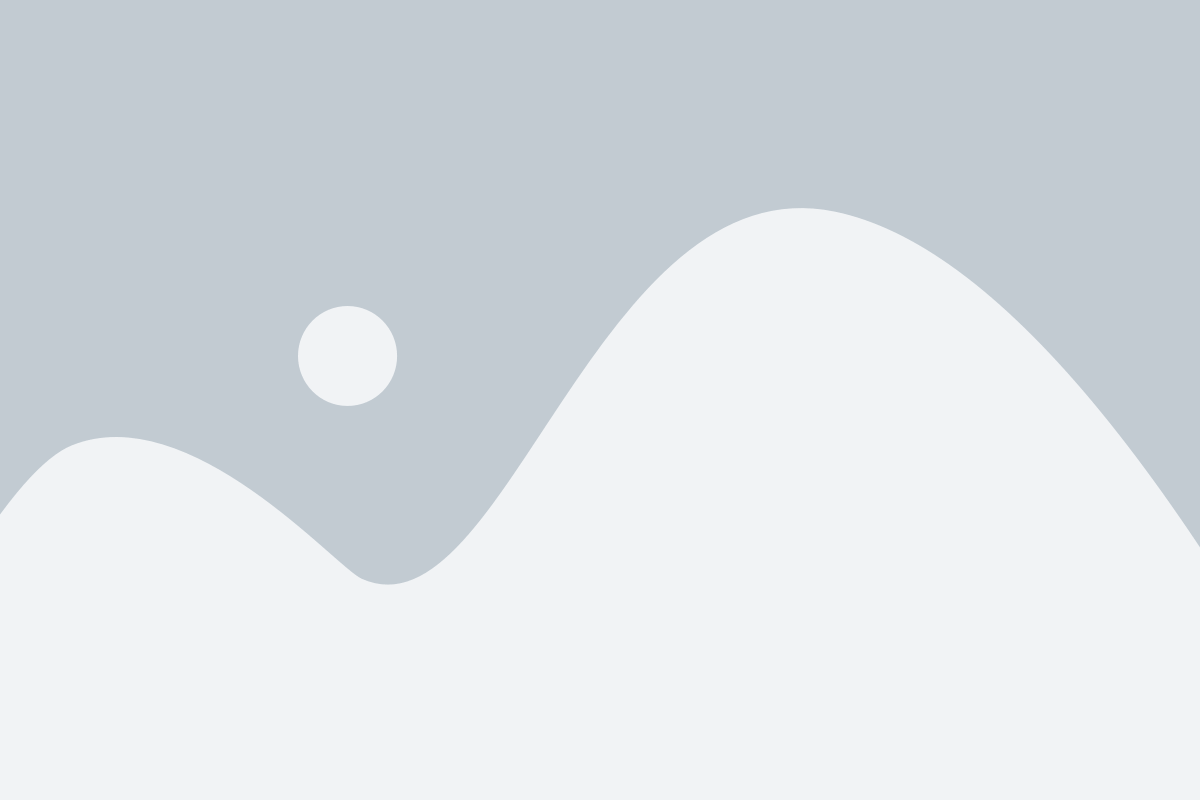
The traditional archetype of learning and employment – where students went to university and learned a skill that served them for the entirety of the careers – is rapidly evolving and changing. Today, we are in the age of continuous learning – where there is an inherent expectation on members of the employed workforce to constantly upgrade their knowledge and soft and hard skills. Earlier, executive education used to be a reserve of a privileged few high performers at large organizations, who showed great promise and rapidly rose through the ranks. Now, continuous learning across all the segments of the workforce is increasingly the new normal – almost to the point where it is the mandate for organizations that wish to grow and succeed in the business sphere. There is an expectation now that even the rank-and-file of the organization devote time to learning, unlearning & relearning and apply newfound ideas and techniques into their area of business.
What has been the driver behind this change? Why has upskilling and reskilling become the norm for the contemporary corporate career? A few important reasons underpin this change. The nature of the business today is extremely dynamic. Business environment and competitive landscape are changing faster than ever, with technology becoming the mainstay of the modern business. Tech-enabled startups are moving in and challenging traditional incumbents across industries. These changes are made a further complex with emergent ideas and changing paradigms of organizational management and leadership. In today’s fast-moving world of business, it is a critical priority for executives to keep their organizations nimble, proactive and armed with every arrow in their quiver, to ensure the continued success of their firms
Executive education is an important medium to achieve this goal and helps bridge the skill gap that is almost certain to rise when industries and organizations face structural headwinds. However, for executive education to live up to the promise and deliver value to employees and their organizations, we need to re-look at the programs itself. We need to ensure that the coursework and curriculum are topical, contextualized and relevant, whilst being personalized to the needs of the organization and its professionals. Here are a few perspectives on how executive education can be adapted for the industry4.0 era:
Expand the Scope of Executive Education and the Courseware
As we dismantle the traditional paradigms of work and education, we also need to rewire our traditional understanding of what an executive education comprises. For years, corporations relied on top-tier management schools and universities to facilitate the essential leadership training for their workforce. In today’s world, rewiring an understanding of leadership is just not going to cut it. Executive education programs need to add more in terms of practical, on-the-job skills, that will help employees perform better and remain relevant to the needs of the business.
There is now a strong case to expand the scope of executive education beyond traditional B-schools and include even MOOC-based education – which is provided by a plethora of websites today. Coursera, Udemy, LinkedIn Learning – to name a few – provide very tactical, hands-on understanding of essential, practical skills that the workforce can put to use right away, while also facilitating the career change aspirations that employees may have. Organizations need to seek out these MOOC-based providers to augment the executive education curriculum in a way that increases its scope and reach among employees.
These programs could very well help employees refresh their skill sets. For instance, such programs could help coders become well-rounded full-stack developers. Similarly, those with data engineering skills could be moved into areas such as high-performance analytics or artificial intelligence. Team leads could be educated formally in the tools and techniques associated with product management. For mapping current employee skills with the contemporary requirements of the business, MOOCs can be a critical intervention to incrementally upskill employees in their domain of work.
Incorporating the importance of shorter, tactical courses
Whilst there is no doubt about the value provided by a long-form one-year executive education program, companies also need to consider the benefits of short-term tactical coursework. Corporations need to augment their training programs with shorter, time-boxed courseware that can deliver instant impact for the organization.
There are two reasons why this is important. Firstly, given the speed at which technology and business mature, it may not always make sense to put someone in a one-year program and wait for the delivery of associated results. In such circumstances, short form courses help deliver faster time-to-value – with employees able to deliver results in weeks, rather than months. Secondly, shorter-term courses also help reduce some of the inherent barriers people have towards learning. Shortening the learning cycle, putting it to use immediately and seeing real-life results, can help employees see instant benefits of the lifelong learning paradigm and break down mental barriers to learning.
Co-create multiple, personalized career pathways
The key word here is ‘personalized’. We need to move away from the old thinking of one-size-fits-all training to deliver more tailored, fit-for-purpose and relevant executive education to employees. To start, organizations need to develop skill maps and assessments – to identify where the workforce is today in terms of the required skill sets and where they are expected to be. Once this is performed, L&D teams can help create personalized learning journey-maps for their employees – based on the career interests and aspirations of employees. For instance, for some employees, it may make sense to provide a refresher and upskilling in their current areas of work and for others, it may make sense to reskill them in new areas of the business. Either way, developing a personalized training regimen for the executive education of the employees will deliver better results and help them excel in their field and improve the efficacy of learning programs too.
Related Posts
AIQRATIONS
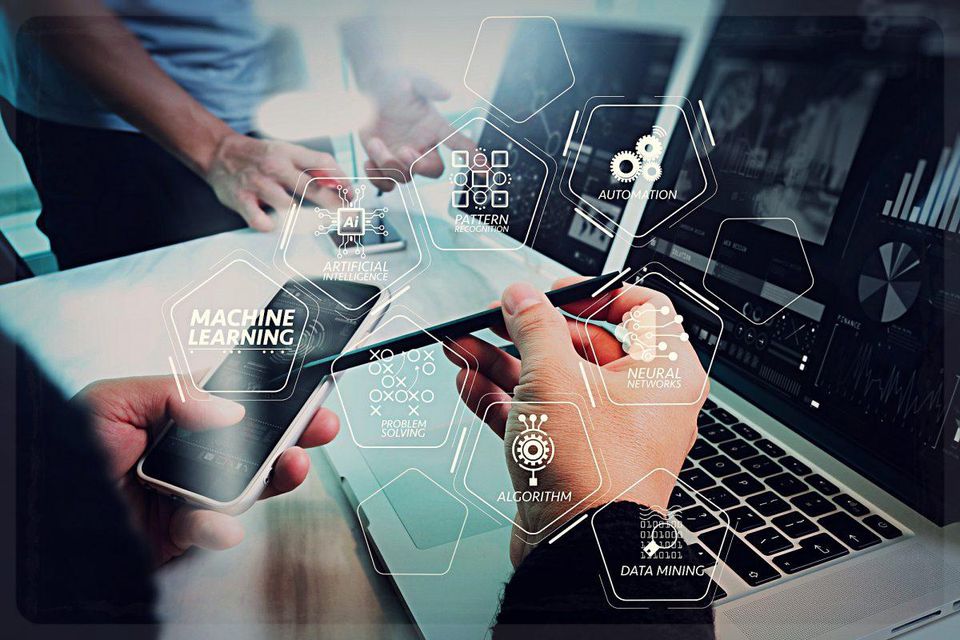
Reimagining Enterprise Decision-Making With Artificial Intelligence
Add Your Heading Text Here
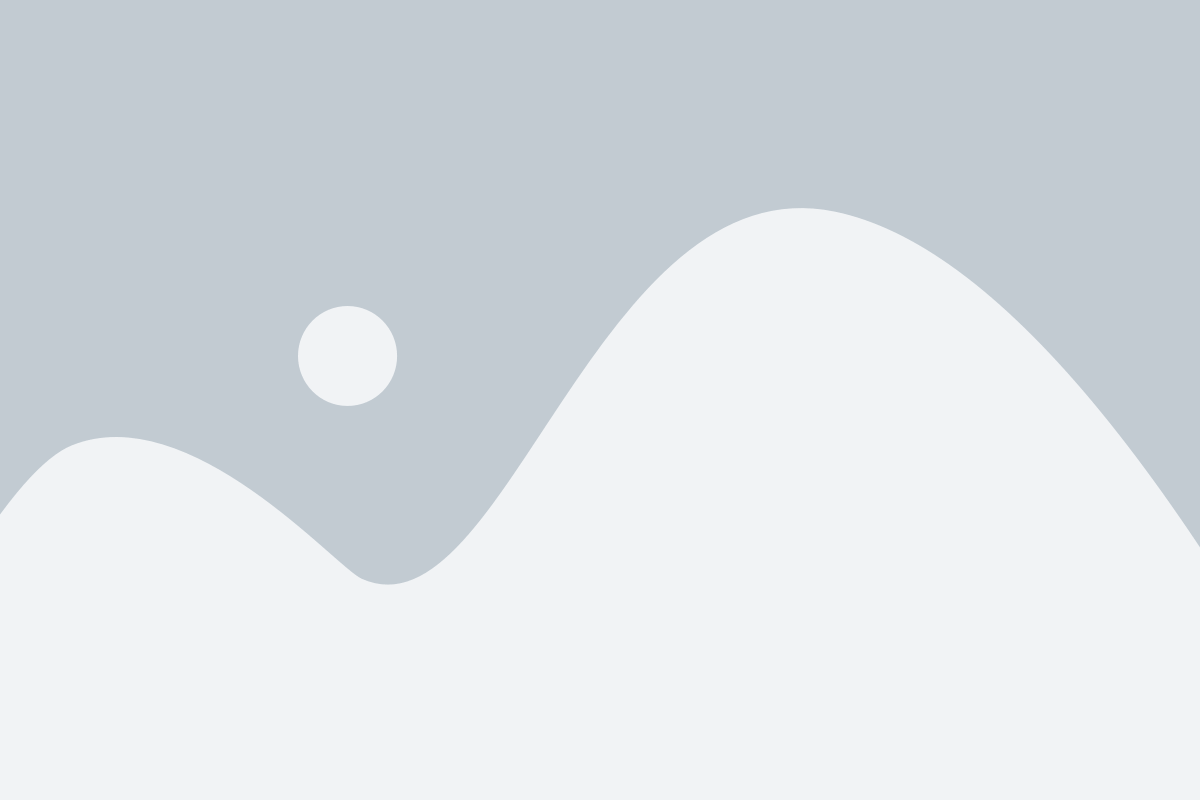
Artificial Intelligence will deliver revolutionary impact on how enterprises make decisions today. In the last few years alone, we have rapidly moved beyond heuristics-based decision-making to analytics-driven decision-support. In the VUCA phase, businesses globally are now pivoting to an AI-led, algorithm-augmented style of decision-making. With huge computing power and ever-increasing data storage and analytics prowess, we are entering a new paradigm, a probable and interesting scenario wherein, Artificial Intelligence will play a huge role in augmenting human intelligence and enabling decision-making with complete autonomy. The big hope is that this new paradigm will not only reduce human biases and errors that are common with heuristic decisions, but also reduce the time involved in making these critical decisions.
Here, I’ll attempt to focus on how we moved from simpler data driven decision-support to AI-powered decisions. The evolution of this technology has been breathtaking to behold and just might provide clues as to what we can expect in the future. Further, I’ll cover a few critical aspects that need to be inculcated by organizations on the AI transformation journey, and provide a few insightful cues that will make this journey exciting and fruitful.
Transformation of Decision-Making: From Analytics to AI
First, let us look at how we got here. Some truly pathbreaking events happened along the way while we were trying to make more accurate business decisions, leading us to reimagine how decisions will be made in the enterprise.
Organizations are Becoming Math Houses
With data deluge and digital detonation, combined with the appreciation of the fact that robust analytical capabilities lead to more informed decisions, we are witnessing AI savvy organizations rapidly maturing into ‘math houses.’ Data science – the ability to extract meaningful insights out of data has become de rigueur. Why? Because we now know that data, when seen in isolation, is inherently dumb. It is the ability to process this data and identify patterns and anomalies – using sophisticated algorithms and ensemble techniques – that makes all the difference. These self-intuitive algorithms are where real value resides – as they define the intelligence required to uncover insights and make smart recommendations. Organizations today are evolving into algorithm factories. There is a real understanding today that by enabling continuous advancement in mathematical algorithms, we can deliver consistent decisions based on prescribed as well as evolving business rules.
It is now an established reality that companies with robust mathematical capabilities possess a huge advantage over those that don’t. Indeed, it’s this math-house orientation that separates companies like Amazon and Google from the ones they leave in their wake, with their ability to understand their customers better, identify anomalies and recognize key patterns.
AI: From Predictive to Prescriptive
We saw a similar evolution in the age of analytics – wherein the science and value veered from descriptive analytics, providing diagnostics of past events to prescriptive analytics, helping see and shape the future. We are seeing a similar evolution in how AI gets leveraged in the enterprise and where its maximum value lies.
In early implementations, it was common to see AI as just a tool to predict and forecast future conditions, while accounting for the dynamism seen in the external environment. Today, AI-enabled decision-making is more prescriptive, with AI providing enterprises not just a look into the future, but also key diagnostics and suggestions on potential decision options and their payoffs. Such evolved applications of AI can help businesses make decisions that can potentially exploit more business opportunities, while averting potential threats much earlier.
Mr. Algorithm to Drive Decision Making
The culmination of this AI-era advancement would be the introduction of smart algorithms in every walk of life and business. Algorithms will become further mainstream leading to what will be the most sweeping business change since the industrial revolution. Organizations – those that already aren’t – will start developing a suite of algorithmic IP’s that will de-bias most enterprise decisions.
If Mr. Algorithm is going to drive most enterprise decisions of tomorrow, we need to create some checks and balances to ensure that it does not go awry. It is more critical today than ever before that the algorithmic suite developed by enterprises has a strong grounding in ethics and can handle situations appropriately for which explicit training may not have been provided.
How to Enable this AI Era of Change
Ushering into an AI-centric era of decision-making will require organizational transformation from business, cultural and technical standpoints. The following facets will be the enablers of this change:
Developing an Engineering Mindset
Instrumenting AI in the enterprise requires a combination of data scientists and computer scientists. As AI matures in the enterprise, the users, use cases and data will increase exponentially. To deliver impactful AI applications, scale and extensibility is critically important. This is where having an engineering mindset comes in. Imbibing an engineering mindset will help standardize the use of these applications while ensuring that they are scalable and extensible.
Learning, Unlearning, Relearning
The other critical aspect to a culture where AI can thrive is creating an environment supporting continuous unlearning and relearning. AI can succeed if the people developing and operating it are rewarded for continuous experimentation and exploration. And just like AI, people should be encouraged to incorporate feedback loops and learn continuously. As technology matures it’s important that the existing workforce keeps up. For one, it’s critical that the knowledge of algorithm theory, applied math alongside training on AI library and developer tools, is imparted into the workforce – and is continuously updated to reflect new breakthroughs in this space.
Embedding Design-Thinking and Behavioral Science at the Center of this Transformation
Finally, given the nature of AI applications, it’s critical that they are consumed voraciously. User input very often activates the learning cycles of artificial intelligence applications. To ensure high usage of these applications, it’s very important that we put the user at the center while designing these applications. This is where the application of behavioral sciences and human-centered design will deliver impact. By imparting empathy in these applications for the user, we will be able to design better and more useful AI applications.
As we augment decision-making with algorithmic, AI-centered systems and platforms – the big expectation is that they will bring untold efficiencies in terms of cost, alongside improvement in the speed and quality with which decisions get made. It’s time to reimagine and deliver on enterprise decision-making that is increasingly shaped through artificial intelligence. These aspects – how the AI is progressing and how to exploit its potential are of paramount importance to keep in mind for an AI transformation.