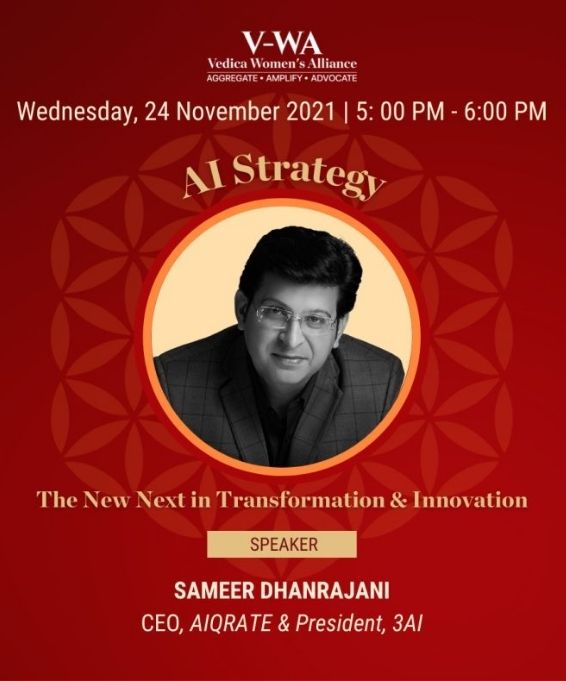
Session on AI Strategy at The Vedica Women’s Alliance (V-WA)
Add Your Heading Text Here
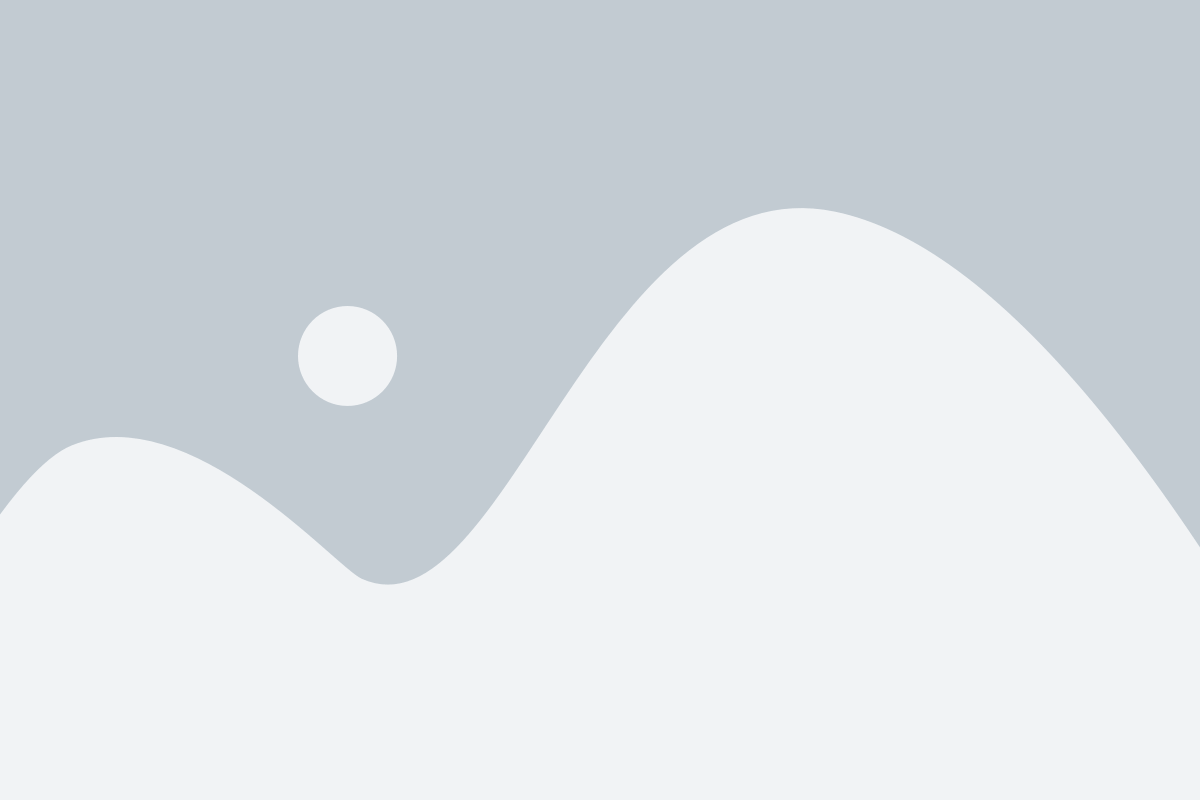
The Vedica Women’s Alliance (V-WA) is hosting a session with Sameer Dhanrajani, Chief Executive Officer, AIQRATE, and President, 3AI – AI & Analytics Association on AI Strategy: The New Next in Transformation & Innovation.
Wedenesday, 24th November 2021 | 5pm – 6pm ISTIn this session, Sameer will demystify the adoption of AI and discuss how organisations can accelerate embracing AI.
Related Posts
AIQRATIONS
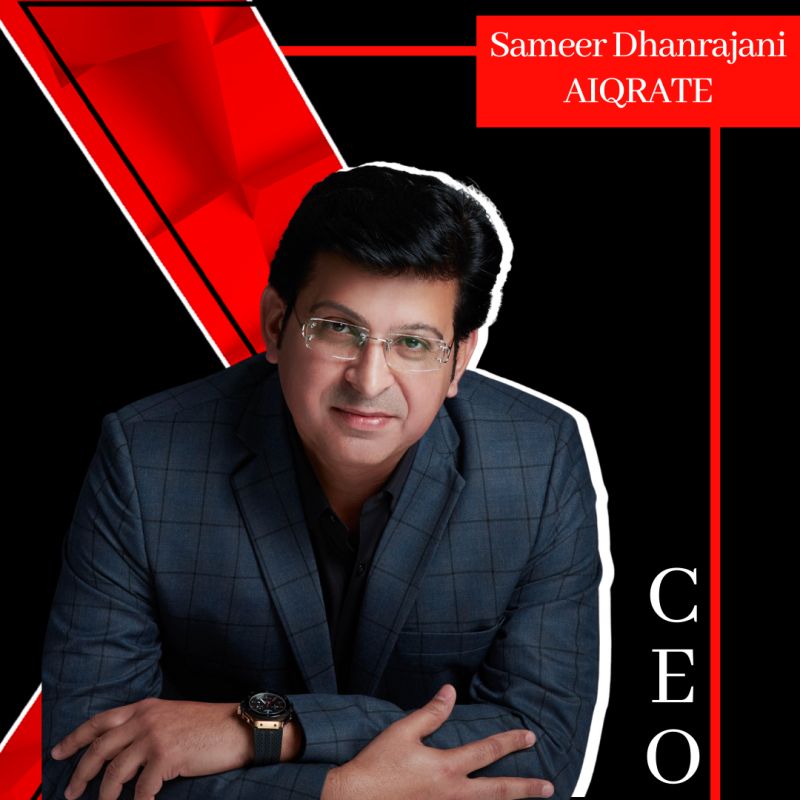
Fifth TEDx speaking engagement at TEDxNMIMSBangalore
Add Your Heading Text Here
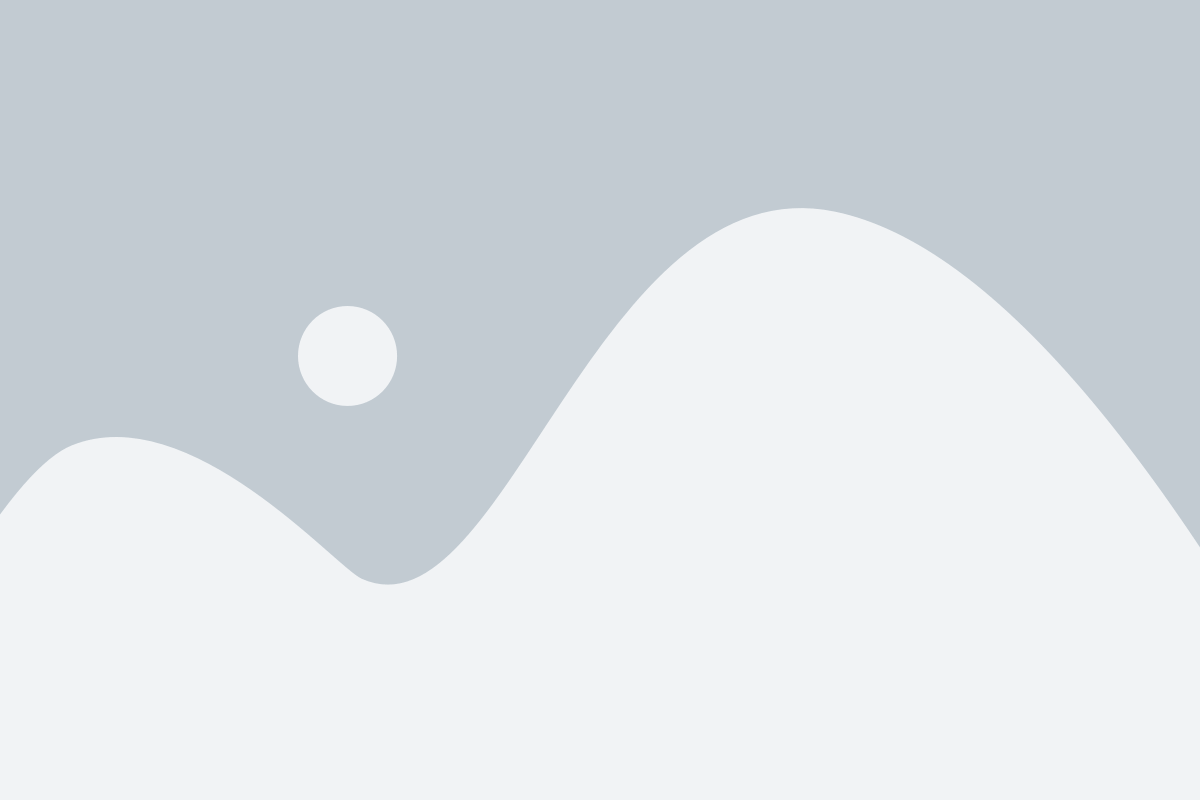
Sameer Dhanrajani, CEO, AIQRATE Advisory & Consulting, is part of speakers line up at TEDxNMIMS Bangalore.. TEDx speaking engagement, his fifth. A marquee platform with a distinguished & ensemble line up of speakers.
The TEDx theme “miniature yet monumental “ is apt in topical times . I will be sharing my perspectives on AI taking center stage in the enterprise decision making process .. the unknown phenomenon yet the biggest one !!
Related Posts
AIQRATIONS
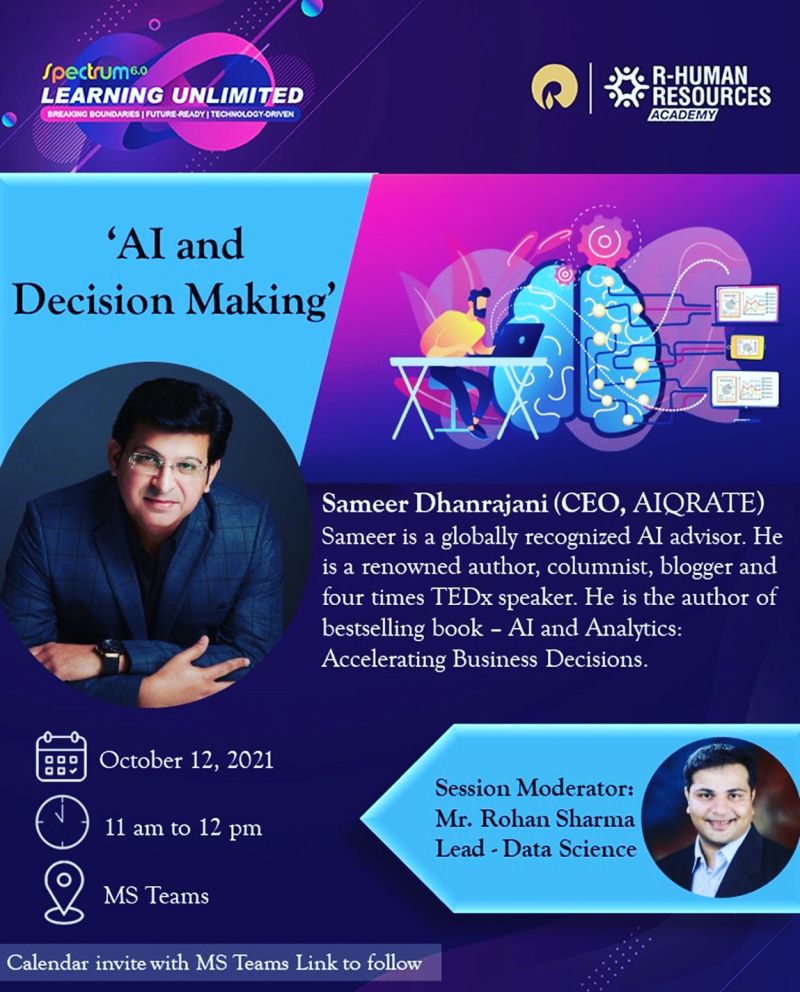
AI & Decision Making session at Spectrum 6.0 Learning Unlimited event at Reliance Industries Limited
Add Your Heading Text Here
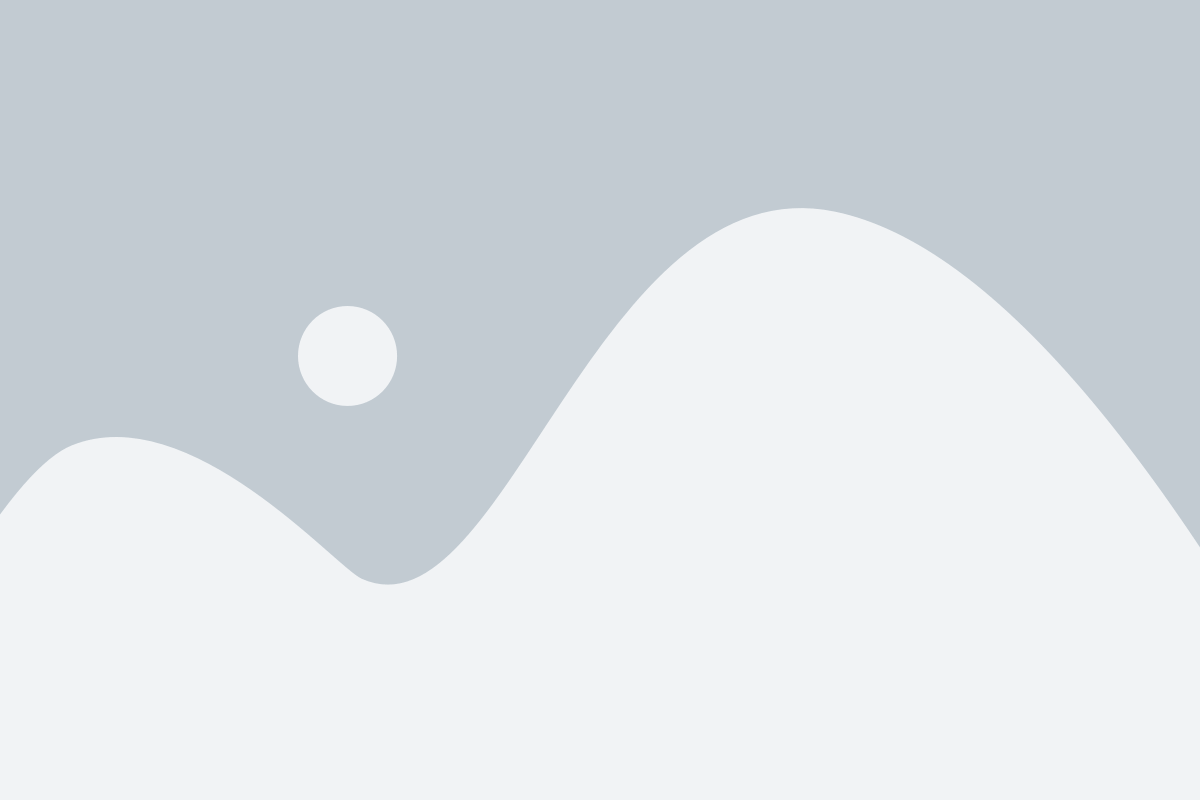
Sameer Dhanrajani, CEO, AIQRATE Advisory & Consulting, presented AI framework & approach on the art of possible & decision making at scale with AI at Spectrum 6.0 Learning Unlimited event at Reliance Industries Ltd on 12th October, 2021. The session was attended by 300+ HR senior leaders & executives. The session carried aspects on reimagining HR business chain with AI and the ensuing impact across employee journey & experience .
Related Posts
AIQRATIONS
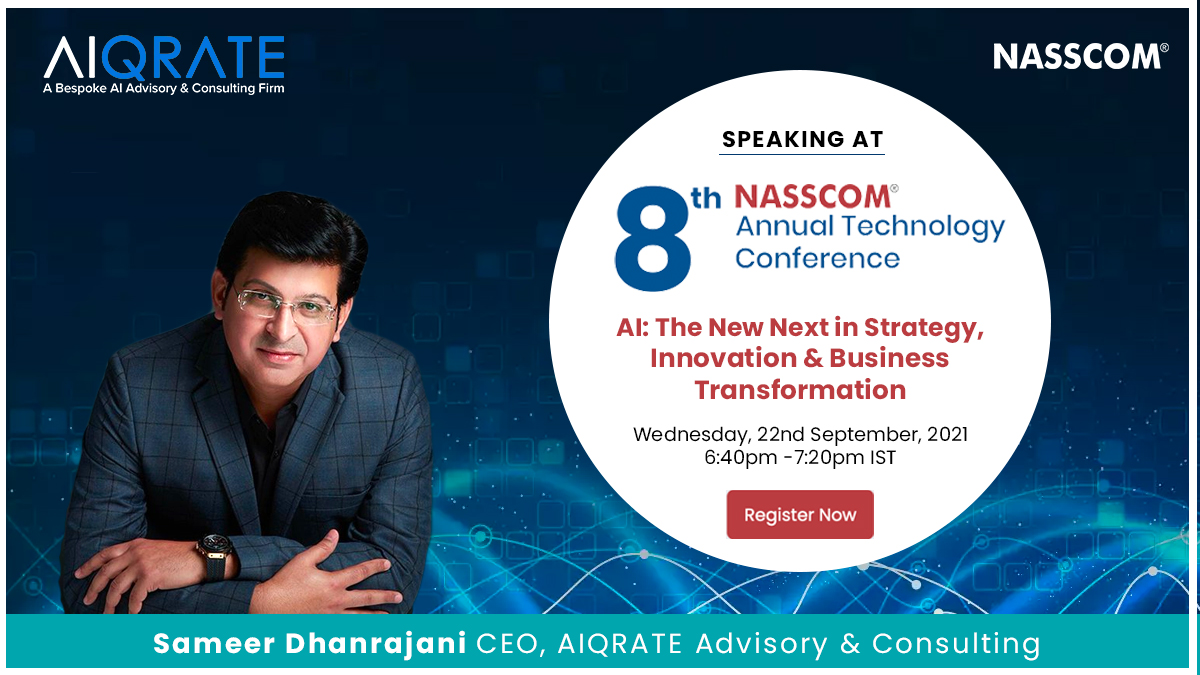
8th NASSCOM Annual Technology Conference 2021
Add Your Heading Text Here
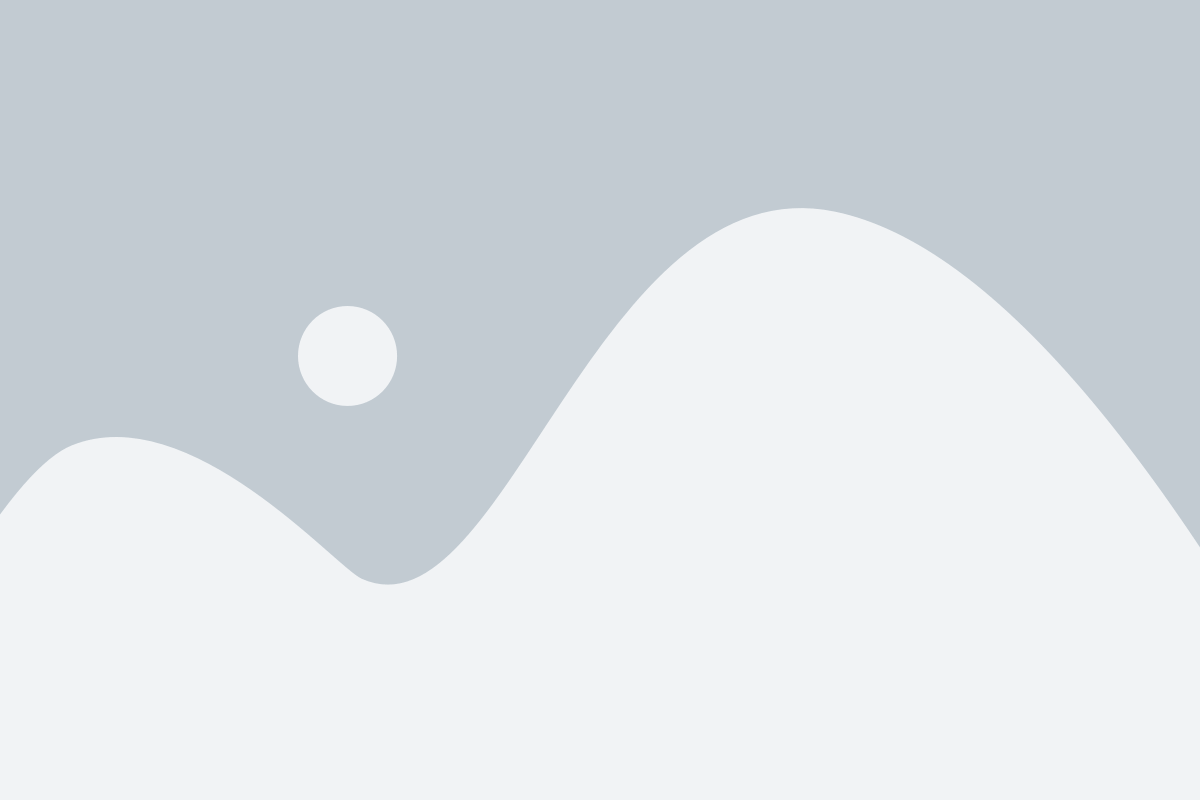
NASSCOM Annual Technology Conference 2021
GO DIGITAL – Thrive in the New Normal
22nd – 24th September, 2021
REGISTER NOW: https://nasscom.in/natc/
The global pandemic has accelerated technology disruption, in addition to shifting buyer behaviors and increasing competition. At NASSCOM Annual Technology Conference 2021, you will get a uniquely informed update on the technology trends that are impacting your business. A guide to thriving and managing high growth, at the same time ensuring seamless delivery, process optimization, efficiencies, customer experience enhancement without compromising security, trust, and ethics.The future of any business is AI. Being AI ready is changing the whole economics of the world, buying behaviour of the consumers and dynamics of the organizations. In this ever evolving world, any organization that is not in sync with velocity and agility of change stands at a huge risk of becoming irrelevant.
Leveraging AI will result in emergence of trifecta of better decision making – Augmented Intelligence, Automate & learn and Incorporate human behaviour. The trifecta of better decision making enabled by AI will compel CXOs to usher new strategic paradigms.
AI: the New Next in strategy, innovation & business transformation
The session delivered by seasoned and proven AI evangelist and business builder Sameer Dhanrajani, CEO, AIQRATE Advisory & Consulting, will compel the participants to cogitate towards developing AI strategies in conjunction with looking at developing frameworks and action plans for leveraging AI capabilities for inculcating Transformation, Innovation and Disruption dynamics within your organizations.
Related Posts
AIQRATIONS
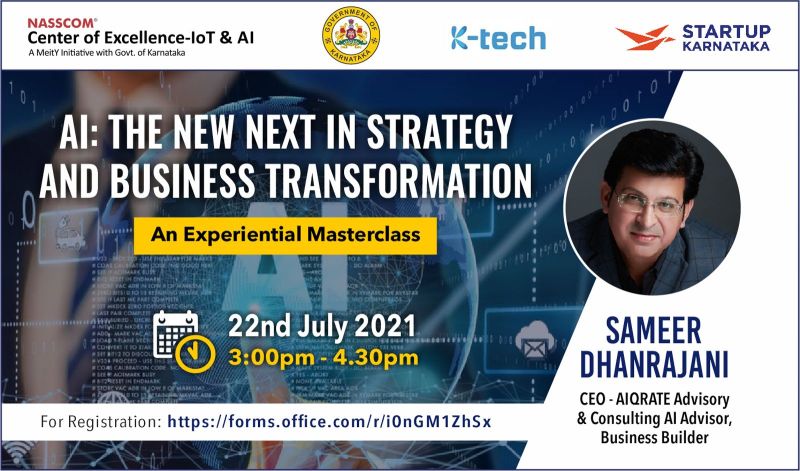
AI Experiential Masterclass – NASSCOM CoE IOT & AI
Add Your Heading Text Here
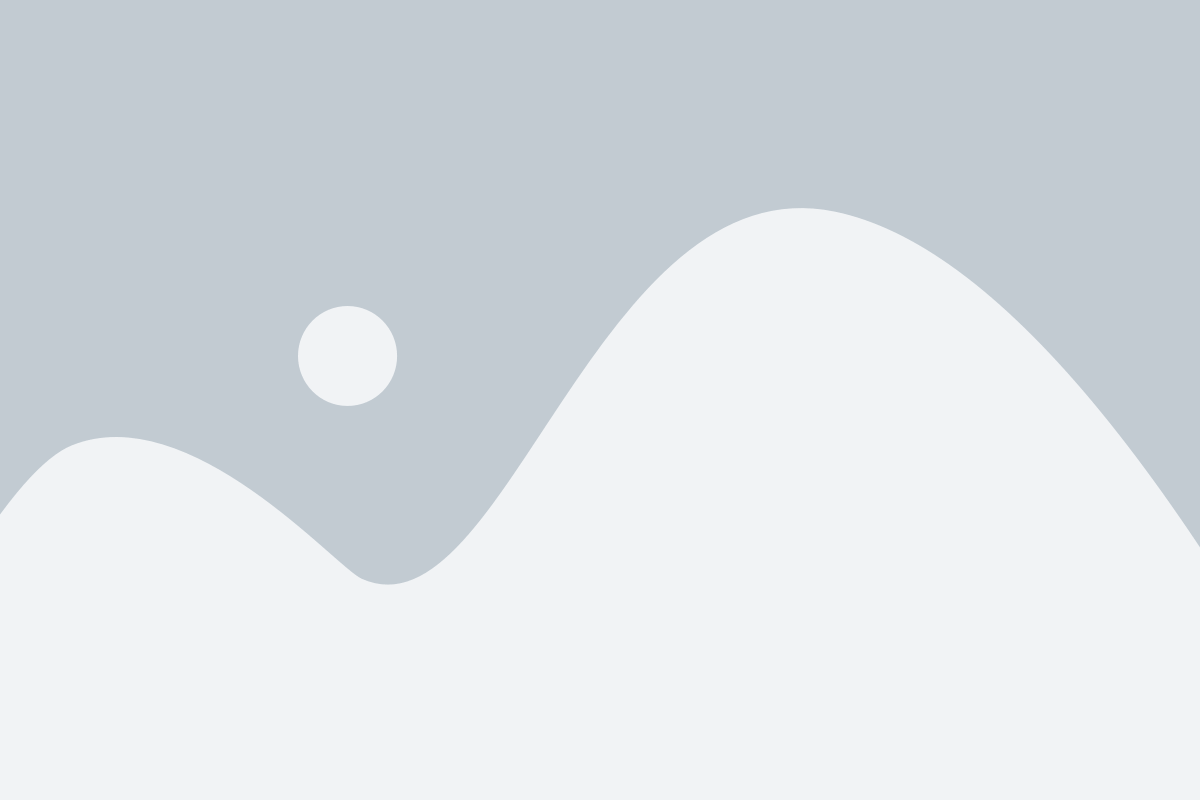
NASSCOM Centre of Excellence IOT & AI in collaboration with Startup Karnataka invited Sameer Dhanrajani, CEO at AIQRATE Advisory & Consulting, for delivering one-of-a-kind customized and experiential masterclass “AI: The New Next in Strategy and Business Transformation“. This was for delegates to learn about the developing AI strategies that can be inculcated to perceive disruption dynamics within organizations.
Masterclass was held on 22nd July, 2021 | 3pm – 4:30pm.
Related Posts
AIQRATIONS
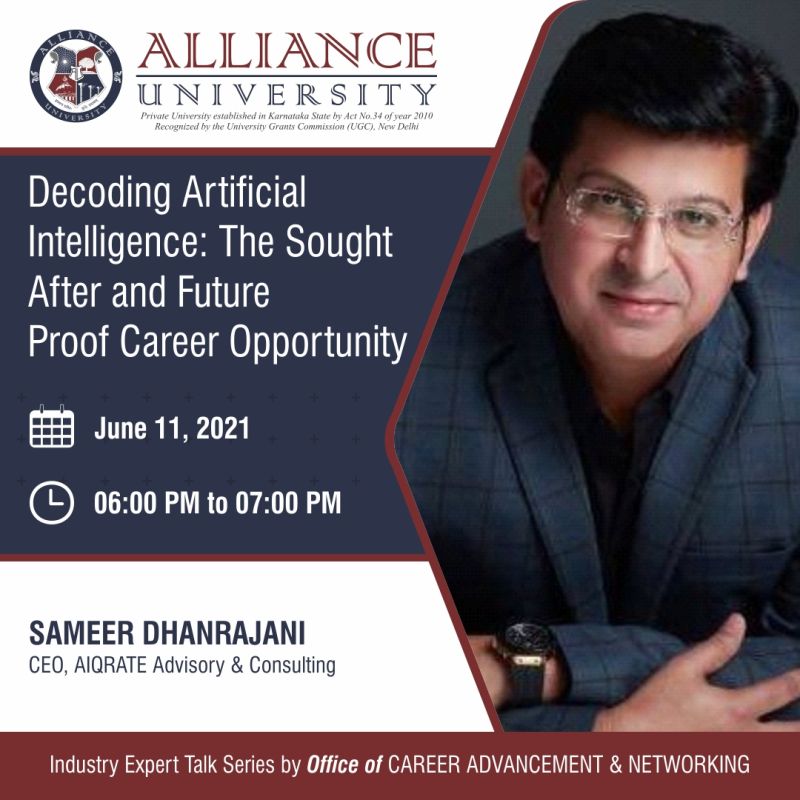
Industry Expert Talk at Alliance University
Add Your Heading Text Here
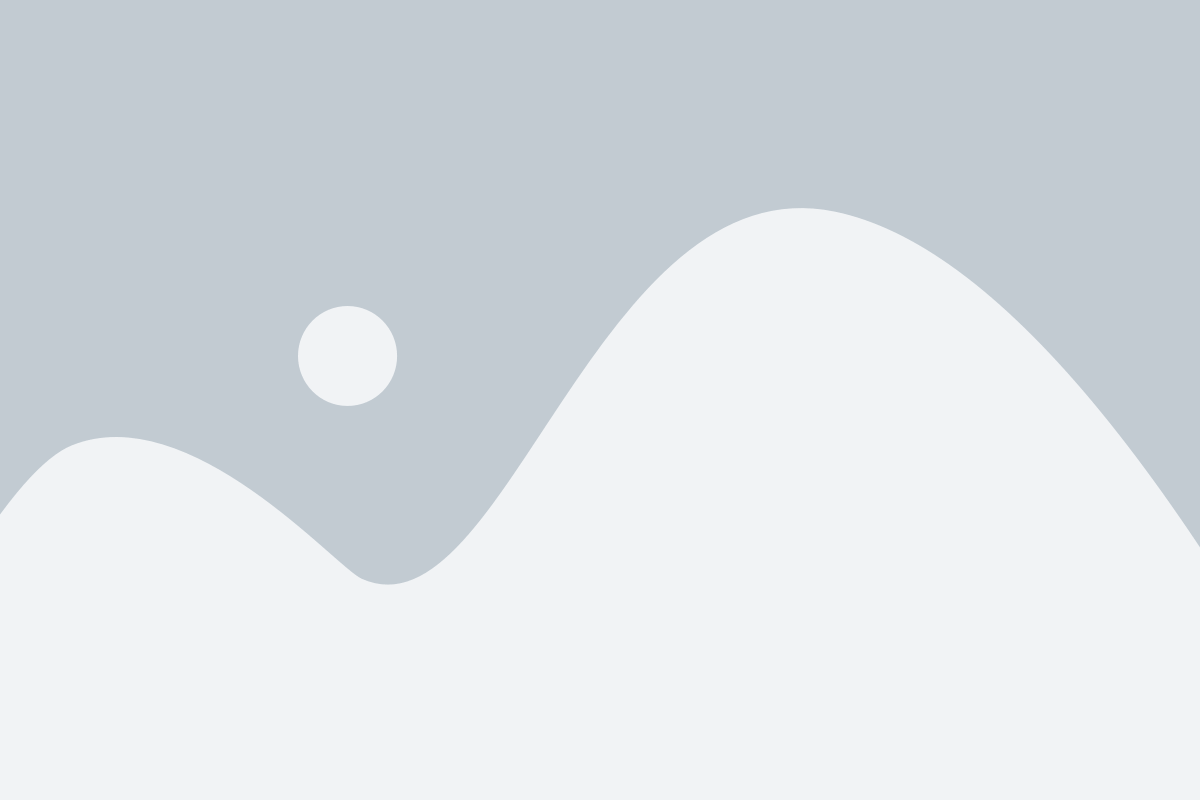
Office of Career Advancement and Networking at Alliance University had organized an Industry Expert Talk with Mr. Sameer Dhanrajani to discuss ‘Decoding Artificial Intelligence: The Sought After and Future Proof Career Opportunity’ on June 11, 2021 at 6:00 PM.
Related Posts
AIQRATIONS
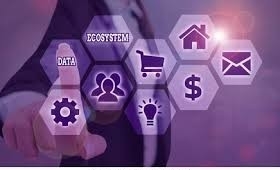
Data Driven Enterprise – Part II: Building an operative data ecosystems strategy
Add Your Heading Text Here
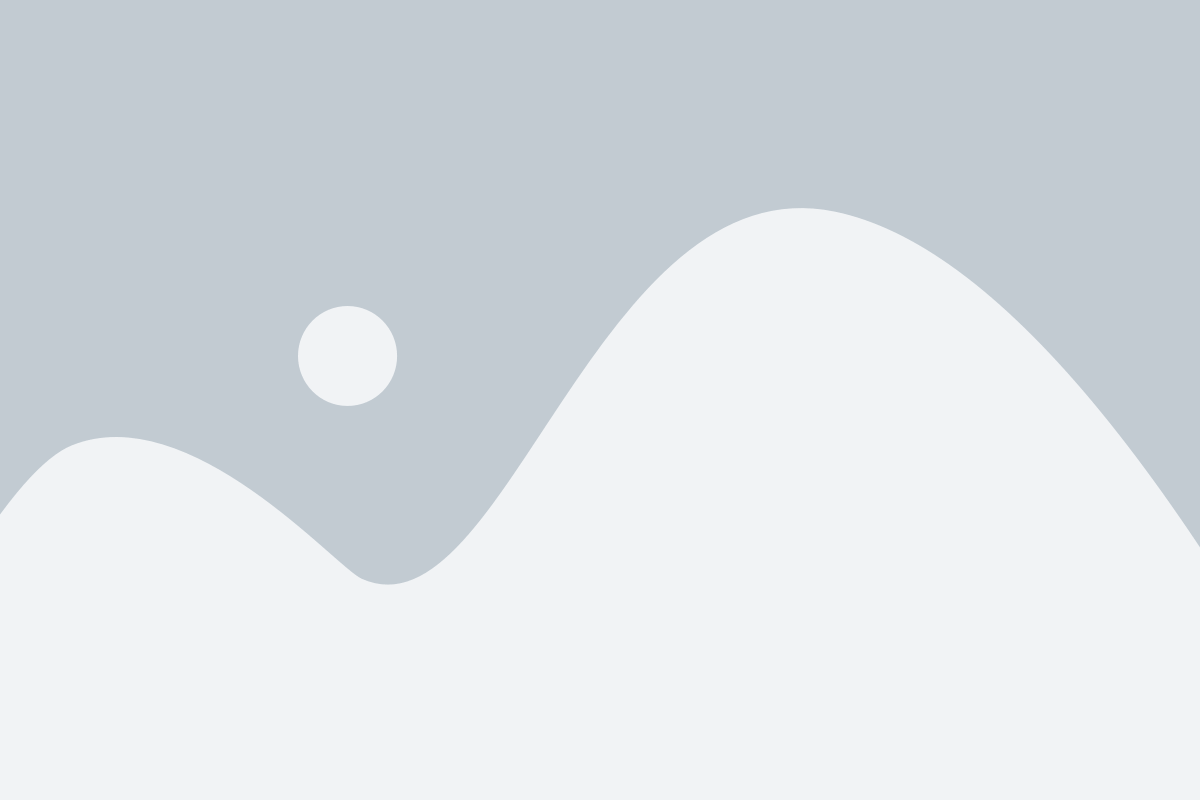
Ecosystems—interconnected sets of services in a single integrated experience—have emerged across a range of industries, from financial services to retail to healthcare. Ecosystems are not limited to a single sector; indeed, many transcend multiple sectors. For traditional incumbents, ecosystems can provide a golden opportunity to increase their influence and fend off potential disruption by faster-moving digital attackers. For example, banks are at risk of losing half of their margins to fintechs, but they have the opportunity to increase margins by a similar amount by orchestrating an ecosystem.
In my experience, many ecosystems focus on the provision of data: exchange, availability, and analysis. Incumbents seeking to excel in these areas must develop the proper data strategy, business model, and architecture.
What is a data ecosystem?
Simply put, a data ecosystem is a platform that combines data from numerous providers and builds value through the usage of processed data. A successful ecosystem balances two priorities:
Building economies of scale by attracting participants through lower barriers to entry. In addition, the ecosystem must generate clear customer benefits and dependencies beyond the core product to establish high exit barriers over the long term.Cultivating a collaboration network that motivates a large number of parties with similar interests (such as app developers) to join forces and pursue similar objectives. One of the key benefits of the ecosystem comes from the participation of multiple categories of players (such as app developers and app users).
What are the data-ecosystem archetypes?
As data ecosystems have evolved, five archetypes have emerged. They vary based on the model for data aggregation, the types of services offered, and the engagement methods of other participants in the ecosystem.
- Data utilities. By aggregating data sets, data utilities provide value-adding tools and services to other businesses. The category includes credit bureaus, consumer-insights firms, and insurance-claim platforms.
- Operations optimization and efficiency centers of excellence. This archetype vertically integrates data within the business and the wider value chain to achieve operational efficiencies. An example is an ecosystem that integrates data from entities across a supply chain to offer greater transparency and management capabilities.
- End-to-end cross-sectorial platforms. By integrating multiple partner activities and data, this archetype provides an end-to-end service to the customers or business through a single platform. Car reselling, testing platforms, and partnership networks with a shared loyalty program exemplify this archetype.
- Marketplace platforms. These platforms offer products and services as a conduit between suppliers and consumers or businesses. Amazon and Alibaba are leading examples.
- B2B infrastructure (platform as a business). This archetype builds a core infrastructure and tech platform on which other companies establish their ecosystem business. Examples of such businesses are data-management platforms and payment-infrastructure providers.
The ingredients for a successful data ecosystem : Data ecosystems have the potential to generate significant value. However, the entry barriers to establishing an ecosystem are typically high, so companies must understand the landscape and potential obstacles. Typically, the hardest pieces to figure out are finding the best business model to generate revenues for the orchestrator and ensuring participation.
If the market already has a large, established player, companies may find it difficult to stake out a position. To choose the right partners, executives need to pinpoint the value they can offer and then select collaborators who complement and support their strategic ambitions. Similarly, companies should look to create a unique value proposition and excellent customer experience to attract both end customers and other collaborators. Working with third parties often requires additional resources, such as negotiating teams supported by legal specialists to negotiate and structure the collaboration with potential partners. Ideally, partnerships should be mutually beneficial arrangements between the ecosystem leader and other participants.
As companies look to enable data pooling and the benefits it can generate, they must be aware of laws regarding competition. Companies that agree to share access to data, technology, and collection methods restrict access for other companies, which could raise anti-competition concerns. Executives must also ensure that they address privacy concerns, which can differ by geography.
Other capabilities and resources are needed to create and build an ecosystem. For example, to find and recruit specialists and tech talent, organizations must create career opportunities and a welcoming environment. Significant investments will also be needed to cover the costs of data-migration projects and ecosystem maintenance.
Ensuring ecosystem participants have access to data
Once a company selects its data-ecosystem archetype, executives should then focus on setting up the right infrastructure to supports its operation. An ecosystem can’t deliver on its promise to participants without ensuring access to data, and that critical element relies on the design of the data architecture. We have identified five questions that incumbents must resolve when setting up their data ecosystem.
How do we exchange data among partners in the ecosystem?
Industry experience shows that standard data-exchange mechanisms among partners, such as cookie handshakes, for example, can be effective. The data exchange typically follows three steps: establishing a secure connection, exchanging data through browsers and clients, and storing results centrally when necessary.
How do we manage identity and access?
Companies can pursue two strategies to select and implement an identity-management system. The more common approach is to centralize identity management through solutions such as Okta, OpenID, or Ping. An emerging approach is to decentralize and federate identity management—for example, by using blockchain ledger mechanisms.
How can we define data domains and storage?
Traditionally, an ecosystem orchestrator would centralize data within each domain. More recent trends in data-asset management favor an open data-mesh architecture . Data mesh challenges conventional centralization of data ownership within one party by using existing definitions and domain assets within each party based on each use case or product. Certain use cases may still require centralized domain definitions with central storage. In addition, global data-governance standards must be defined to ensure interoperability of data assets.
How do we manage access to non-local data assets, and how can we possibly consolidate?
Most use cases can be implemented with periodic data loads through application programming interfaces (APIs). This approach results in a majority of use cases having decentralized data storage. Pursuing this environment requires two enablers: a central API catalog that defines all APIs available to ensure consistency of approach, and strong group governance for data sharing.
How do we scale the ecosystem, given its heterogeneous and loosely coupled nature?
Enabling rapid and decentralized access to data or data outputs is the key to scaling the ecosystem. This objective can be achieved by having robust governance to ensure that all participants of the ecosystem do the following:
- Make their data assets discoverable, addressable, versioned, and trustworthy in terms of accuracy
- Use self-describing semantics and open standards for data exchange
- Support secure exchanges while allowing access at a granular level
The success of a data-ecosystem strategy depends on data availability and digitization, API readiness to enable integration, data privacy and compliance—for example, General Data Protection Regulation (GDPR)—and user access in a distributed setup. This range of attributes requires companies to design their data architecture to check all these boxes.
As incumbents consider establishing data ecosystems, we recommend they develop a road map that specifically addresses the common challenges. They should then look to define their architecture to ensure that the benefits to participants and themselves come to fruition. The good news is that the data-architecture requirements for ecosystems are not complex. The priority components are identity and access management, a minimum set of tools to manage data and analytics, and central data storage.Truly mentioning , Developing an operative data ecosystem strategy is far more difficult than getting the tech requirements right.
Related Posts
AIQRATIONS
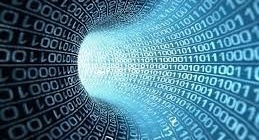
Data Driven Enterprise – Part I: Building an effective Data Strategy for competitive edge
Add Your Heading Text Here
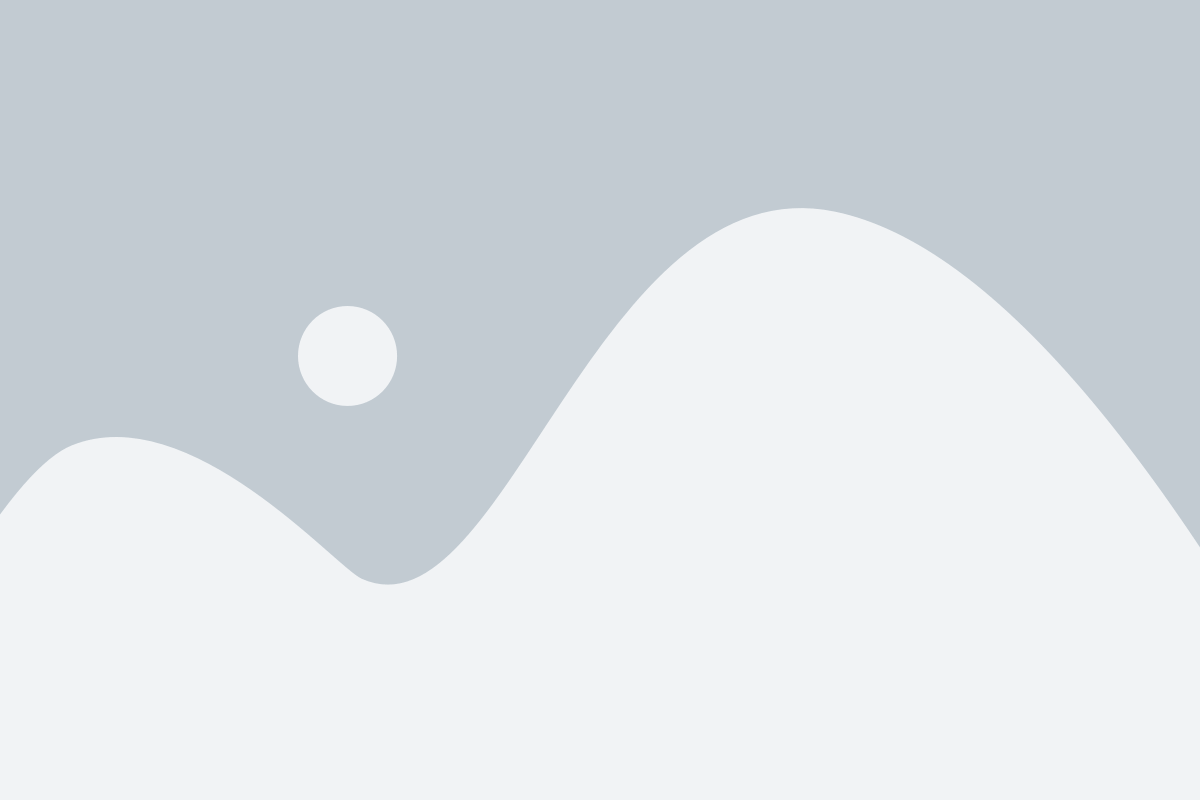
Few Enterprises take full advantage of data generated outside their walls. A well-structured data strategy for using external data can provide a competitive edge. Many enterprises have made great strides in collecting and utilizing data from their own activities. So far, though, comparatively few have realized the full potential of linking internal data with data provided by third parties, vendors, or public data sources. Overlooking such external data is a missed opportunity. Organizations that stay abreast of the expanding external-data ecosystem and successfully integrate a broad spectrum of external data into their operations can outperform other companies by unlocking improvements in growth, productivity, and risk management.
The COVID-19 crisis provides an example of just how relevant external data can be. In a few short months, consumer purchasing habits, activities, and digital behavior changed dramatically, making preexisting consumer research, forecasts, and predictive models obsolete. Moreover, as organizations scrambled to understand these changing patterns, they discovered little of use in their internal data. Meanwhile, a wealth of external data could—and still can—help organizations plan and respond at a granular level. Although external-data sources offer immense potential, they also present several practical challenges. To start, simply gaining a basic understanding of what’s available requires considerable effort, given that the external-data environment is fragmented and expanding quickly. Thousands of data products can be obtained through a multitude of channels—including data brokers, data aggregators, and analytics platforms—and the number grows every day. Analyzing the quality and economic value of data products also can be difficult. Moreover, efficient usage and operationalization of external data may require updates to the organization’s existing data environment, including changes to systems and infrastructure. Companies also need to remain cognizant of privacy concerns and consumer scrutiny when they use some types of external data.
These challenges are considerable but surmountable. This blog series discusses the benefits of tapping external-data sources, illustrated through a variety of examples, and lays out best practices for getting started. These include establishing an external-data strategy team and developing relationships with data brokers and marketplace partners. Company leaders, such as the executive sponsor of a data effort and a chief data and analytics officer, and their data-focused teams should also learn how to rigorously evaluate and test external data before using and operationalizing the data at scale.
External-data success stories: Companies across industries have begun successfully using external data from a variety of sources . The investment community is a pioneer in this space. To predict outcomes and generate investment returns, analysts and data scientists in investment firms have gathered “alternative data” from a variety of licensed and public data sources, many of which draw from the “digital exhaust” of a growing number of technology companies and the public web. Investment firms have established teams that assess hundreds of these data sources and providers and then test their effectiveness in investment decisions.
A broad range of data sources are used, and these inform investment decisions in a variety of ways:
- Investors actively gather job postings, company reviews posted by employees, employee-turnover data from professional networking and career websites, and patent filings to understand company strategy and predict financial performance and organizational growth.
- Analysts use aggregated transaction data from card processors and digital-receipt data to understand the volume of purchases by consumers, both online and offline, and to identify which products are increasing in share. This gives them a better understanding of whether traffic is declining or growing, as well as insights into cross-shopping behaviors.
- Investors study app downloads and digital activity to understand how consumer preferences are changing and how effective an organization’s digital strategy is relative to that of its peers. For instance, app downloads, activity, and rating data can provide a window into the success rates of the myriad of live-streaming exercise offerings that have become available over the last year.
Corporations have also started to explore how they can derive more value from external data . For example, a large insurer transformed its core processes, including underwriting, by expanding its use of external-data sources from a handful to more than 40 in the span of two years. The effort involved was considerable; it required prioritization from senior leadership, dedicated resources, and a systematic approach to testing and applying new data sources. The hard work paid off, increasing the predictive power of core models by more than 20 percent and dramatically reducing application complexity by allowing the insurer to eliminate many of the questions it typically included on customer applications.
Three steps to creating value with external data:
Use of external data has the potential to be game changing across a variety of business functions and sectors. The journey toward successfully using external data has three key steps.
1. Establish a dedicated team for external-data sourcing
To get started, organizations should establish a dedicated data-sourcing team. Per our understanding at AIQRATE , a key role on this team is a dedicated data scout or strategist who partners with the data-analytics team and business functions to identify operational, cost, and growth improvements that could be powered by external data. This person also would be responsible for building excitement around what can be made possible through the use of external data, planning the use cases to focus on, identifying and prioritizing data sources for investigation, and measuring the value generated through use of external data. Ideal candidates for this role are individuals who have served as analytics translators and who have experience in deploying analytics use cases and in working with technology, business, and analytics profiles.
The other team members, who should be drawn from across functions, would include purchasing experts, data engineers, data scientists and analysts, technology experts, and data-review-board members . These team members typically spend only part of their time supporting the data-sourcing effort. For example, the data analysts and data scientists may already be supporting data cleaning and modeling for a specific use case and help the sourcing work stream by applying the external data to assess its value. The purchasing expert, already well versed in managing contracts, will build specialization on data-specific licensing approaches to support those efforts.
Throughout the process of finding and using external data, companies must keep in mind privacy concerns and consumer scrutiny, making data-review roles essential peripheral team members. Data reviewers, who typically include legal, risk, and business leaders, should thoroughly vet new consumer data sets—for example, financial transactions, employment data, and cell-phone data indicating when and where people have entered retail locations. The vetting process should ensure that all data were collected with appropriate permissions and will be used in a way that abides by relevant data-privacy laws and passes muster with consumer.This team will need a budget to procure small exploratory data sets, establish relationships with data marketplaces (such as by purchasing trial licenses), and pay for technology requirements (such as expanded data storage).
2. Develop relationships with data marketplaces and aggregators
While online searches may appear to be an easy way for data-sourcing teams to find individual data sets, that approach is not necessarily the most effective. It generally leads to a series of time-consuming vendor-by-vendor discussions and negotiations. The process of developing relationships with a vendor, procuring sample data, and negotiating trial agreements often takes months. A more effective strategy involves using data-marketplace and -aggregation platforms that specialize in building relationships with hundreds of data sources, often in specific data domains—for example, consumer, real-estate, government, or company data. These relationships can give organizations ready access to the broader data ecosystem through an intuitive search-oriented platform, allowing organizations to rapidly test dozens or even hundreds of data sets under the auspices of a single contract and negotiation. Since these external-data distributors have already profiled many data sources, they can be valuable thought partners and can often save an external-data team significant time. When needed, these data distributors can also help identify valuable data products and act as the broker to procure the data.
Once the team has identified a potential data set, the team’s data engineers should work directly with business stakeholders and data scientists to evaluate the data and determine the degree to which the data will improve business outcomes. To do so, data teams establish evaluation criteria, assessing data across a variety of factors to determine whether the data set has the necessary characteristics for delivering valuable insights . Data assessments should include an examination of quality indicators, such as fill rates, coverage, bias, and profiling metrics, within the context of the use case. For example, a transaction data provider may claim to have hundreds of millions of transactions that help illuminate consumer trends. However, if the data include only transactions made by millennial consumers, the data set will not be useful to a company seeking to understand broader, generation-agnostic consumer trends.
3. Prepare the data architecture for new external-data streams
Generating a positive return on investment from external data calls for up-front planning, a flexible data architecture, and ongoing quality-assurance testing.Up-front planning starts with an assessment of the existing data environment to determine how it can support ingestion, storage, integration, governance, and use of the data. The assessment covers issues such as how frequently the data come in, the amount of data, how data must be secured, and how external data will be integrated with internal data. This will provide insights about any necessary modifications to the data architecture.
Modifications should be designed to ensure that the data architecture is flexible enough to support the integration of a continuous “conveyor belt” of incoming data from a variety of data sources—for example, by enabling application-programming-interface (API) calls from external sources along with entity-resolution capabilities to intelligently link the external data to internal data. In other cases, it may require tooling to support large-scale data ingestion, querying, and analysis. Data architecture and underlying systems can be updated over time as needs mature and evolve.The final process in this step is ensuring an appropriate and consistent level of quality by constantly monitoring the data used. This involves examining data regularly against the established quality framework to identify whether the source data have changed and to understand the drivers of any changes (for example, schema updates, expansion of data products, change in underlying data sources). If the changes are significant, algorithmic models leveraging the data may need to be retrained or even rebuilt.
Minimizing risk and creating value with external data will require a unique mix of creative problem solving, organizational capability building, and laser-focused execution. That said, business leaders who demonstrate the achievements possible with external data can capture the imagination of the broader leadership team and build excitement for scaling beyond early pilots and tests. An effective route is to begin with a small team that is focused on using external data to solve a well-defined problem and then use that success to generate momentum for expanding external-data efforts across the organization.
Related Posts
AIQRATIONS
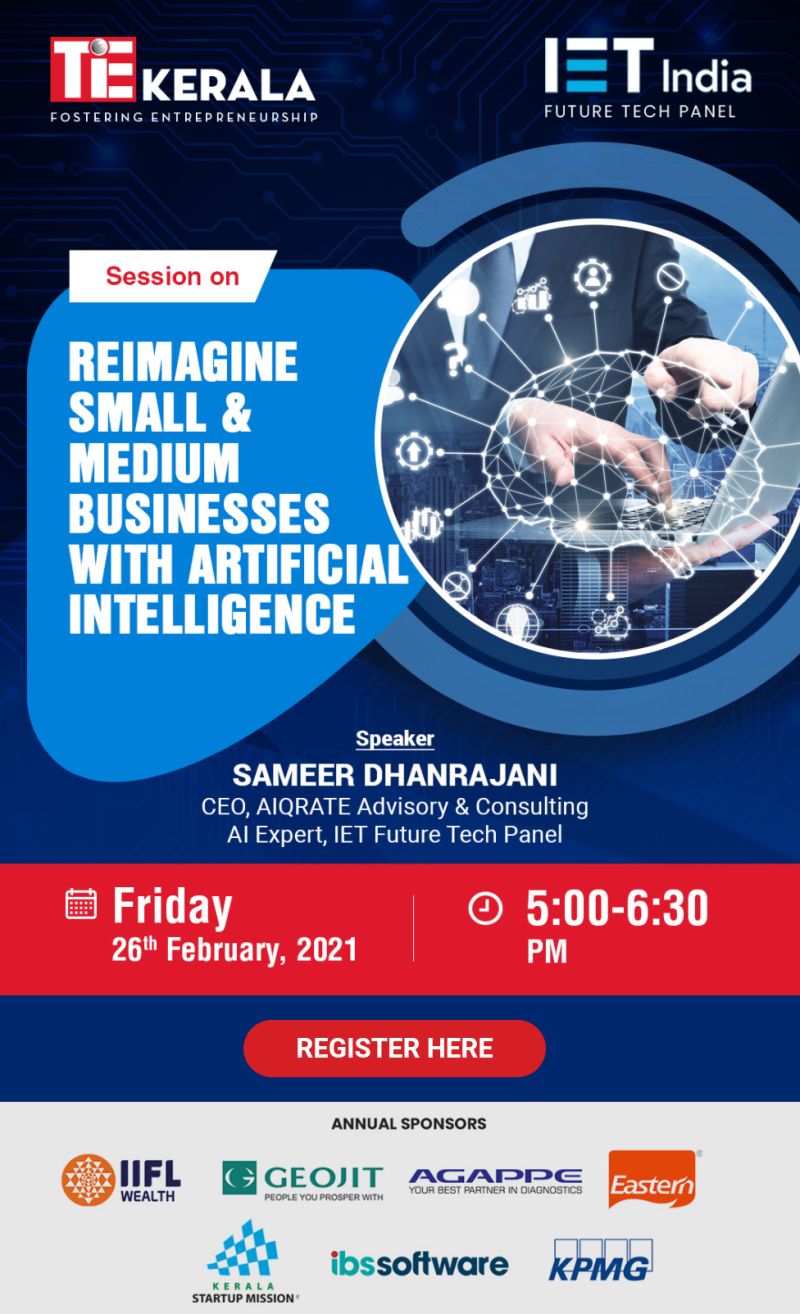
TiE Kerala – IET India : Webinar on ‘Reimagine Small & Medium Businesses with Artificial Intelligence
Add Your Heading Text Here
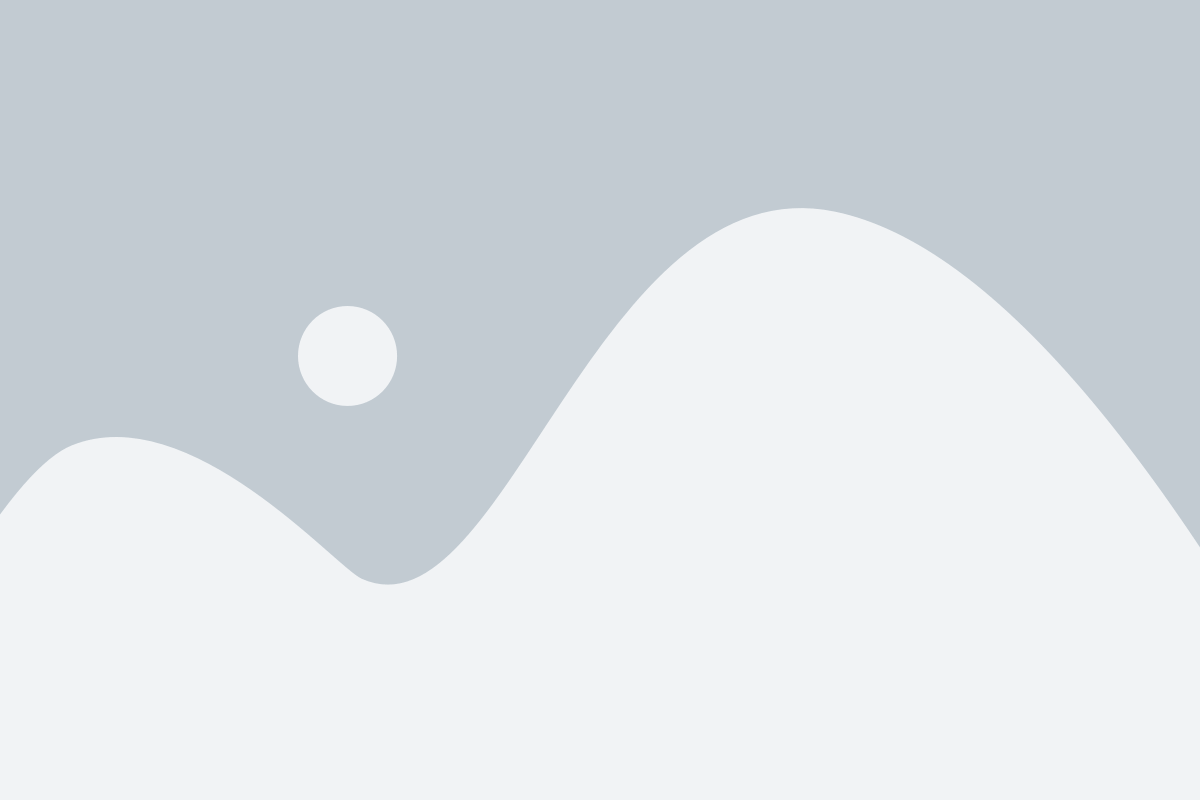
TiE Kerala in partnership with IET India is organising a Webinar on ‘Reimagine SMB’s with AI for Scalability & Competitive advantage’ on Friday, 26th February 2021 between 5:00 to 6:30PM.
Sameer Dhanrajani, CEO of AIQRATE – a bespoke global AI advisory & consulting firm will lead the session.
REGISTER NOW: https://hub.tie.org/e/tie-kerala–iet-india-joint-session-on-reimagine-smb-s-with-ai-for-scalability–competitive-advantage
About the topic:
AI adoption is not just restricted to large enterprises , it is also an excellent lever to achieve tremendous growth for SMBs as well. There is a belief that AI requires considerable investment. Fortunately, for SMBs on a budget, one doesn’t need to break the bank to start incorporating AI into their operations. By picking the low hanging fruits of the business and leveraging quick to deploy AI interventions, one can efficiently harness the capabilities of AI and improve performance metrics across different business functions. However, To be AI ready, SMBs need to strategize and align their AI initiatives with their strategic and operational business goals : revenue enhancement, cost/bottom-line optimization , operational efficiencies , customer acquisition.
SMB’s focused on manufacturing sector are reshaping itself with AI to achieve new models: addictive manufacturing , smart factory , federated production , personalization, connected value networks . This is a critical point for a sector where the possibilities for innovation, transformation through AI comes with new learnings and human capital challenges . The inexorable shift from simple digitization (third industrial revolution ) to innovation based on AI of industry 4.0 has brought about exciting opportunities that policy makers , business leaders and senior executives must pay attention to . Whilst , economic impairment and stunted growth have been deterrents , adoption and adaption of AI is no longer an option . In order to stay relevant in this highly competitive and fast changing business environment , it is imperative for SMB’s to respond quickly to changing customer demands and maximize new market opportunities.
The session anchored by Sameer will showcase on the AI led application areas , AI adoption framework and will provide use cases from different industry segments on leveraging AI to drive growth , scalability and above all, competitive advantage.
Related Posts
AIQRATIONS
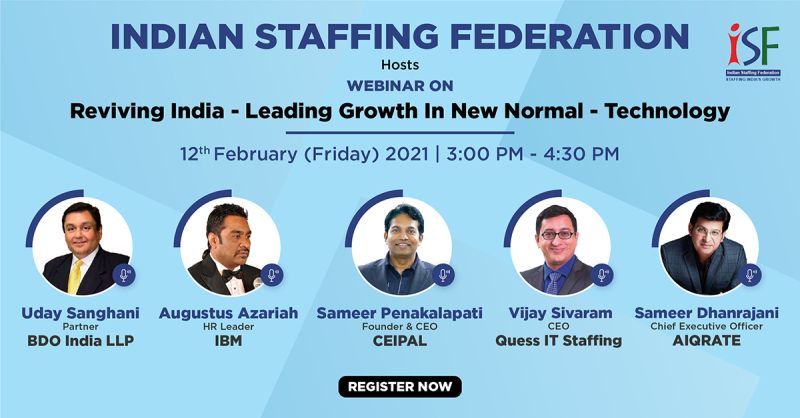
Indian Staffing Federation (ISF) Industry series on Reviving India: How is Technology Leading Growth in New Normal?
Add Your Heading Text Here
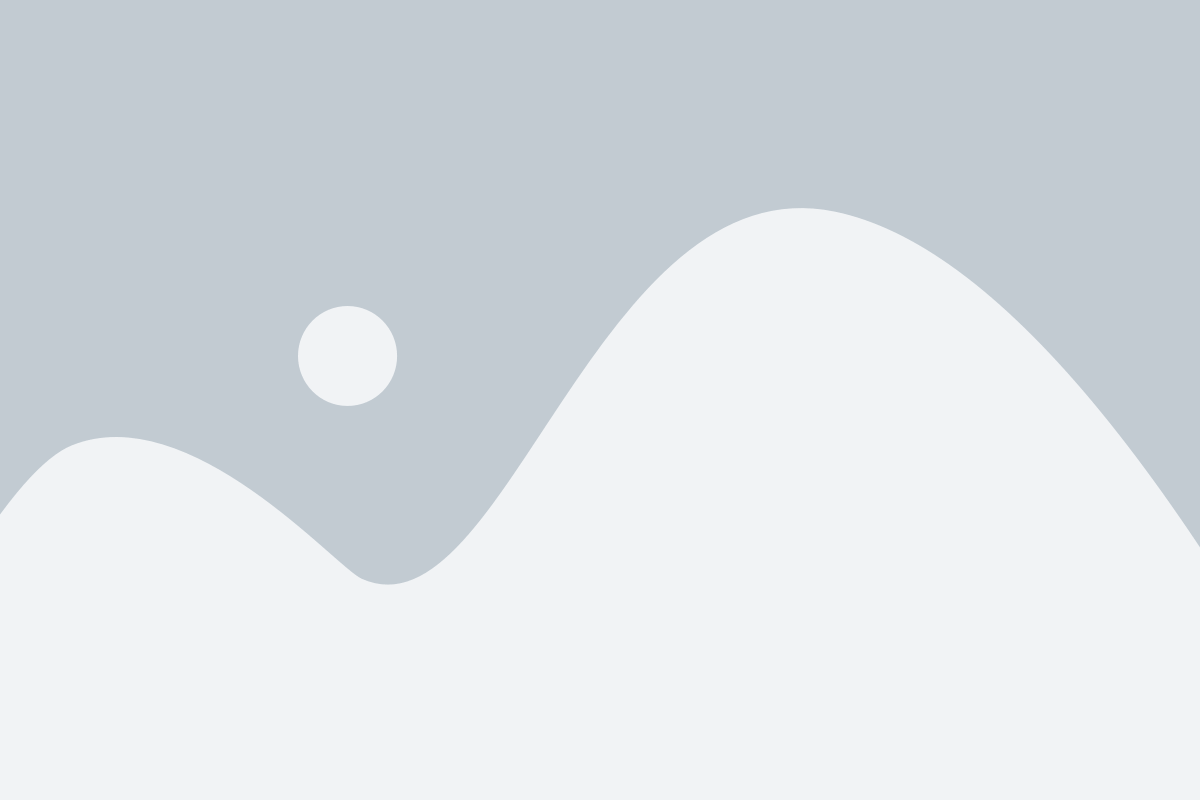
Indian Staffing Federation (ISF) brings its flagship Industry series “Reviving India: How is Technology Leading Growth in New Normal?” on Feb 12, 2021 03:00 PM IST.
About :
The webinar will bring focus on trends and technological changes that are in the anvil of impacting business decisions in the new normal.
Businesses witnessed major disruption in the last eleven months as we witnessed a wave of change towards digitisation, smart transformation – bringing many industries forward with their technological investments. The discussions will cover the way technology has started changing our environment with impact of Digitisation, Internet of Things, Gig Workforce, Artificial Intelligence, Machine Learning among others.
Subject Matter Experts
– Augustus Azariah, HR Leader, IBM India Pvt Ltd
– Uday Sanghani, Partner, BDO India LLP
– Sameer Dhanrajani, CEO, AIQRATE Advisory & Consulting
– Sameer Penakalapati, CEO & Founder, Ceipal
Session Chair
Vijay Sivaram, CEO, Quess IT Staffing
REGISTER NOW: https://zoom.us/webinar/register/9016069279809/WN_2KgmBvGiTVW2wdOaqhuUrA