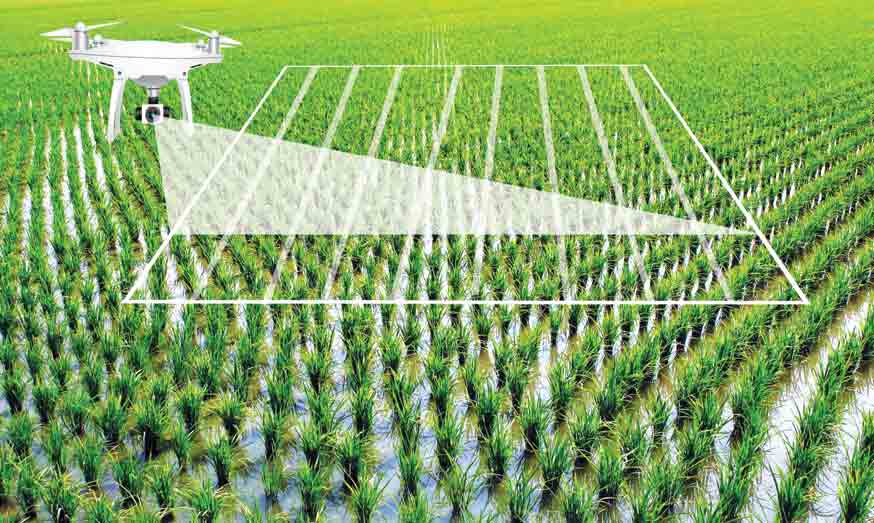
Add Your Heading Text Here
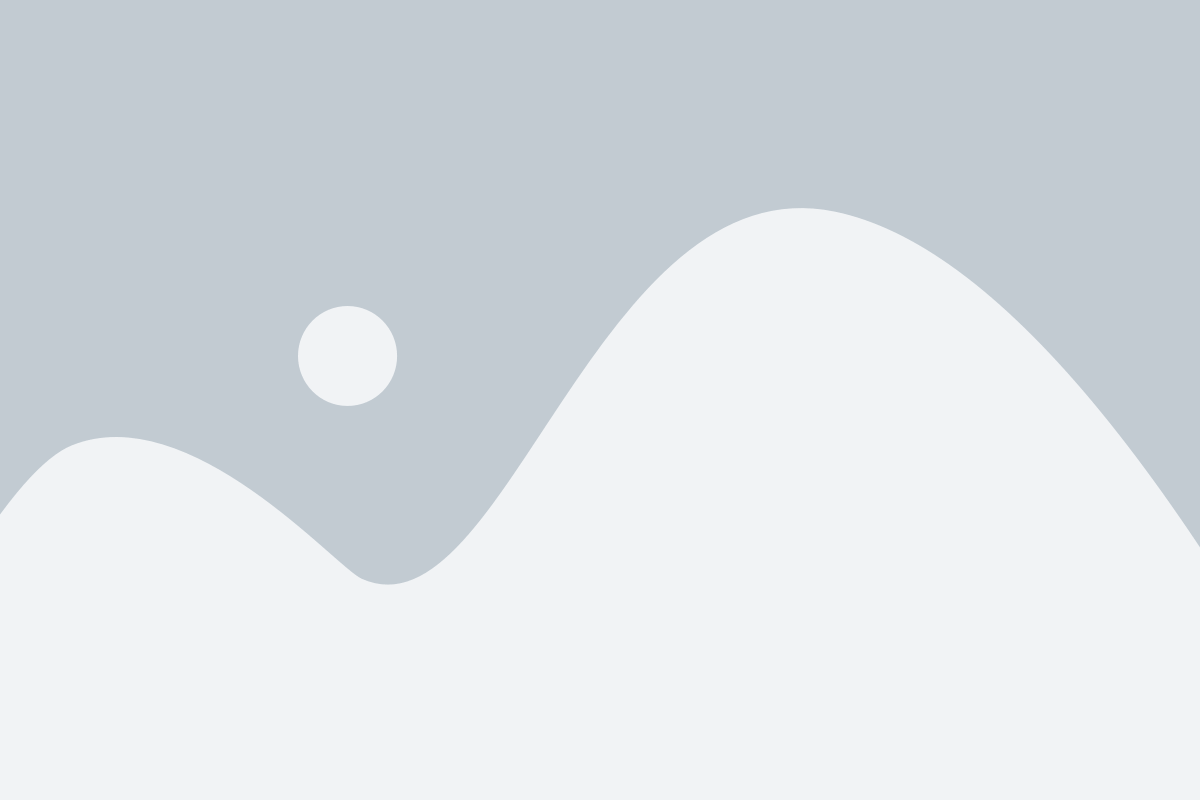
Artificial Intelligence can make a huge impact in the sector by improving operations and productivity
For the many potential application areas where artificial intelligence can deliver breakthrough transformation, unlock efficiencies and augment human life, one of the most impactful areas – at least in the Indian context – will be the value it can bring to the field of agriculture.
According to Indian Brand Equity Foundation, nearly 58% of India’s population relies on agriculture as their primary source of livelihood. The total export of agricultural commodities for India is expected to hit $38.1 billion in FY18, making the country one of the top 15 exporters globally.
However, agriculture in India is riddled with systemic problems, which AI is well placed to address. First, the traditionally unorganised agriculture sector continues to be difficult to organise due to our vast geography combined with our cultural and linguistic diversity. Second, farmers tend to be severely cash-strapped owing to smaller landholding, leading to lower farm productivity. Due to this poor cash position, capital expenditure associated with mechanisation and technology adoption continues to remain slow – thus precluding the possibility of improving farm productivity. Third, farmers are unable to tap a wider base of knowledge and tend to rely on community-based sources of knowledge. This handicap keeps them insulated from the progress of their peers.
Almost all the other large and small topical problems faced by farmers in India flow from one of these three macro-level problems – a lack of organisation, weaker capital muscle to introduce modernisation initiatives and poorer access to a larger, refined body of knowledge. On the bright side, with improved data collection techniques and easier access to cutting-edge research, enabled with better access to telecommunications infrastructure and improving technology literacy, these endemic problems might finally see an amenable solution; combined with the burgeoning interest of the private sector and thrust by the government for modernising farming.
So, it is time for artificial intelligence to step in and deliver a smarter, leaner and productive agrarian sector. Here are the three novel ideas for improving and augmenting farm productivity output using AI:
Communication in Local Languages
AI-powered technology provides a unique opportunity for agriculturists to break down traditional linguistic barriers. Through the infusion of natural language processing (NLP) and machine learning in agriculture, farmers would be able to communicate and learn from their peers and with civic bodies in their local language.
One such example is the development of ‘Kisan Helplines’. Farmers typically need to reach out to civic bodies for information and guidance but this may get hampered due to the poor availability and limited scalability offered by human-centric contact centres. Step in, chatbot. Chatbots can offer high availability, high scalability and reduce the wait time for farmers in need of advice or warnings. Through multi-lingual chatbots powered by NLP, farmers could get easier access to information that may be extremely critical for their activities.
Further, using an NLP-powered communication exchange, they would be able to connect with peers across States for information. Such a communication platform would help crowdsource information on weather progression and potential pest attacks. It would also help seek reviews and feedback on a multitude of farm procurement – seeds, fertilizers, pesticides and mechanical equipment – as well as trade information on successes and practices. By reducing this communication gap and enabling farmers to organise, they would be empowered to make better decisions from sowing to selling and everything in between.
Improving Farming Productivity
While increasing landholding might be challenging, AI can help make the most out of the land available by improving the yield through farm productivity interventions.
By using AI techniques such as machine vision and deep learning, farmers will be able to make many small tweaks to their farming activity that will ultimately result in sizeable gains. For instance, in the pre-sowing and sowing period, machine vision will help farmers monitor soil health and defects – helping them take corrective action early. During the sowing period, farmers can use AI to predict the near-term weather conditions, which can inform the optimal time to sow their seeds. Prior to the harvest, they could harness the power of machine vision to mitigate the impact of pest attacks. Finally, at the time of harvest, machine vision can help farmers grade their produce and demand the right market price.
One such brilliant example of this in action is Microsoft’s initiative with the International Crop Research Institute for the Semi-Arid Tropics (Icrisat), Hyderabad. Their AI sowing app uses machine learning and Microsoft’s PowerBI platform to predict and inform farmers the optimal date to sow. A feature phone capable of receiving text messages is all they need to receive this information. Early results from the use of this technology suggest a 30% improvement in yield per hectare achieved through 175 farmers, who were part of this programme.
Another example is the work being done by Bengaluru-based startup CropIn. Their suite of solutions captures data from multiple sources to provide real-time actionable insights – around weather information, scheduling and monitoring farm activity, monitoring crop health and estimating size of harvest. AI-enabled solutions such as these have great potential to reduce inefficiencies and uncertainties.
Pricing and Supply Chain
While pricing strategies and supply chain optimisation have reached a high level of maturity in the corporate sphere, there is a huge scope for them to impact the agricultural space in India. Using AI, farmers would be able to sense market demand for their goods and keep a pulse of customer choices and seasonality. Market sensing around consumer demand would help them aggressively price their goods and yield a better return.
AI-powered supply chains, on the other hand, can help improve their bottom line by reducing the costs incurred in managing distributed logistics and a multitude of middlemen. Through technology-powered communication networks and smarter routing, smaller farmers too will be able to organise their route-to-market more efficiently and gain benefits of scale. They would also be able to get their perishable goods to market quicker without the intervention of middlemen – thus reducing wastage and losses. An efficient, synchronous farm-to-table supply chain would help bring down their costs and improve their financial position.
At present, AI is only making its first inroads into agriculture. A multitude of use cases exists beyond these as well – from accurately forecasting commodities in the futures market to speeding up loan approval requests through faster credit scoring. AI for agriculture is not only a huge opportunity for startups and large organisations. It also offers the chance to make a huge impact in a sector crying for outside help to improve its operations and productivity.