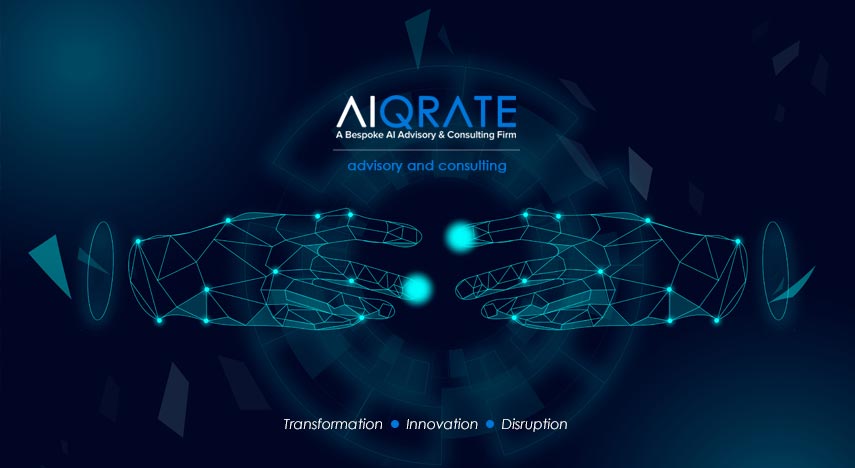
In the AI era, building the next generation of consulting firm: AIQRATE advisory and consulting
Add Your Heading Text Here
– Published in www.xtechalpha.com on 22-November-2019
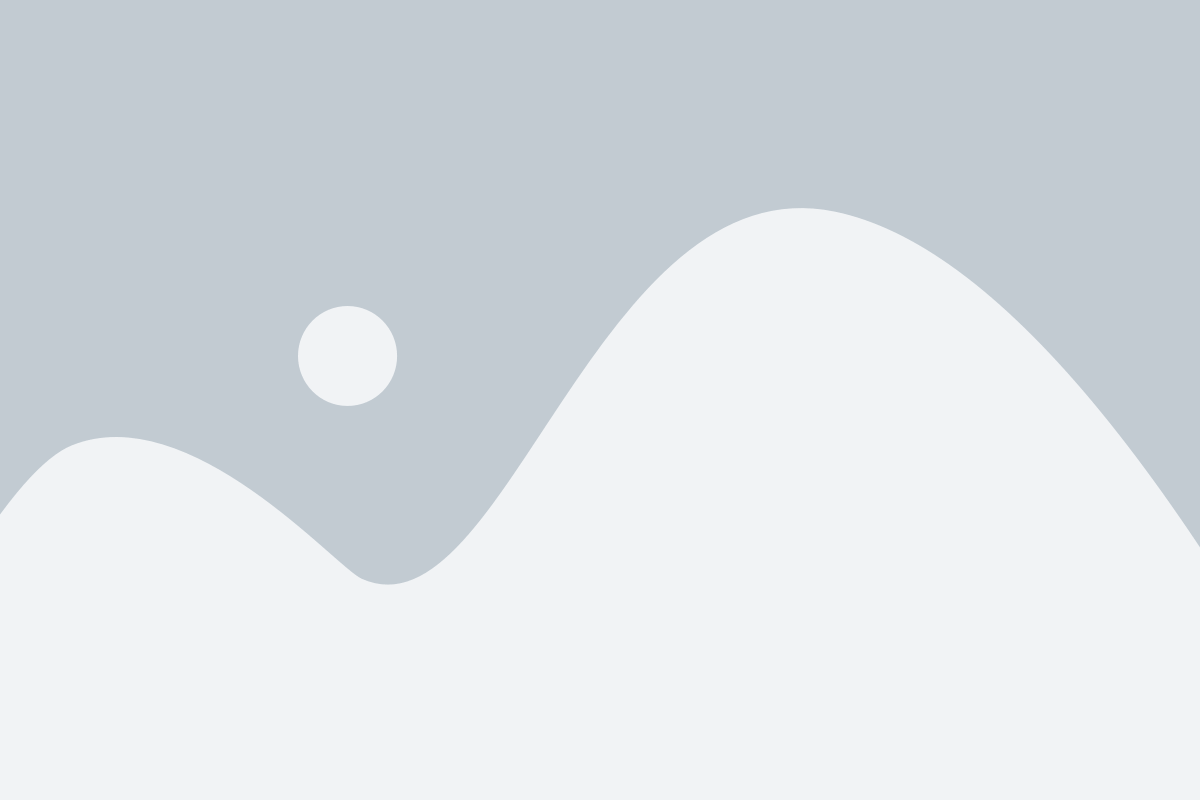
The power of AI is changing business as we know it; When it comes to AI, businesses think ambitiously. Nearly 85% of CXOs believe AI will allow their enterprise to obtain or sustain a competitive advantage in the marketplace. Contrastingly, just one in five enterprises have incorporated AI into their organization and less than 20% of enterprises have an AI strategy.
AI is invoking massive shifts in the business value chains of enterprises. And it is redefining what it takes for enterprises to achieve competitive advantage. Yet, even as several enterprises have begun applying AI engagements with impressive results, few have developed full-scale AI strategy that is contextualized and enterprise wide.
A first in its genre, AIQRATE is a global bespoke AI advisory and consulting firm that promises to provide path breaking AI advisory and consulting offerings with unique client engagement models. Started by three experienced leaders in AI arena – Rohan, NK & Sameer have proven consulting, advisory and client facing professional background coupled with deep experience of understanding global AI market landscape, ecosystem, topical trends, scenarios and approaches. AIQRATE attempts to disrupt the management consulting space with holistic coverage of AI advisory and consulting from AI maturity assessment, AI CoE construct & interventions, partner selection to AI business value output.
AIQRATE advisory services and consulting offerings covers seven business segments: Enterprises, GCCs, Startups, SMBs, VC/PE firms, Academic Institutions & Public Sector Institutions and have 50+ proprietary AI playbooks, primers, frameworks, methodologies, toolkits to enable Boards, CXOs and senior leaders with artisanal insights and recommendations coupled with defining and showcasing broad exhibit plans for making enterprises, GCCs, SMBs and Startups AI ready.
Elaborates Sameer Dhanrajani– CEO & Co-founder, AIQRATE
Adding on, Sameer says that
He builds up further on alluding that a focus on strategic building blocks for AI can help enterprises develop penetrating insights, intelligence and recommendations. While “insight” conjures up visions of research, data crunching, and “aha” moments, real strategic intelligence also rests on a seemingly mundane and easy-to-overlook factor: a thorough understanding of how and why an enterprise, its competitors, and others in the industry value chain make money. Absent dumb luck, AI strategy that doesn’t tap directly into such an understanding will underperform.
Rohan Nag – Chief Client Officer & Co-founder AIQRATE mentions that
Rohan poses couple of pertinent queries as to “How do successful enterprises curate building blocks of AI strategy? And where do AI powerful strategies come from? In our experience, it’s also possible to load the dice in favor of developing good AI strategies by focusing on the core building blocks that often get overlooked. One is the need to gain strategic alignment, before creating an AI strategy on the essential decisions and the criteria for making them. Another is to ensure that the enterprise is prepared and willing to act on an AI strategy once it is adopted. Too much of what passes for strategy development, we find, consists of hurried efforts that skip one or more of the essentials. The resulting AI strategies are often flawed from the start.”
NK, CTO & Co-founder, AIQRATE elucidates upon the advantages of engaging with AIQRATE
We are at an inflection point where AI will revolutionize the way we do business. The paradigms of customer, products, offerings, services and competition will change dramatically; and being AI ready will become a true differentiator. AIQRATE could be your strategic partner to help you to prepare for what’s next in order to stay relevant.
Related Posts
AIQRATIONS
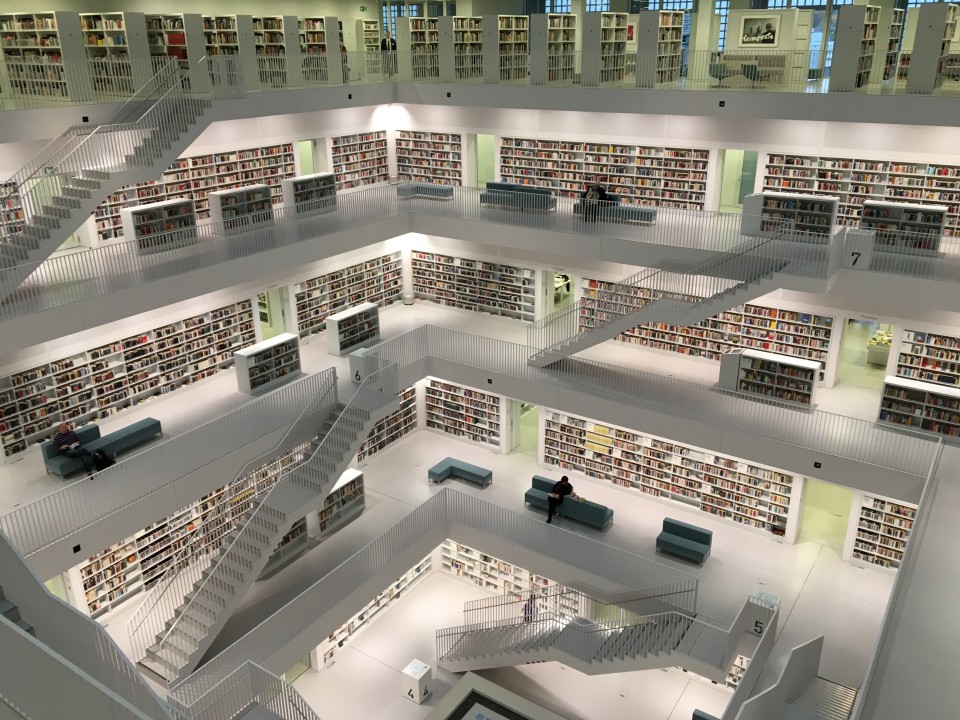
Enterprise Data Engineering Strategy: A must have for New Age organizations
Add Your Heading Text Here
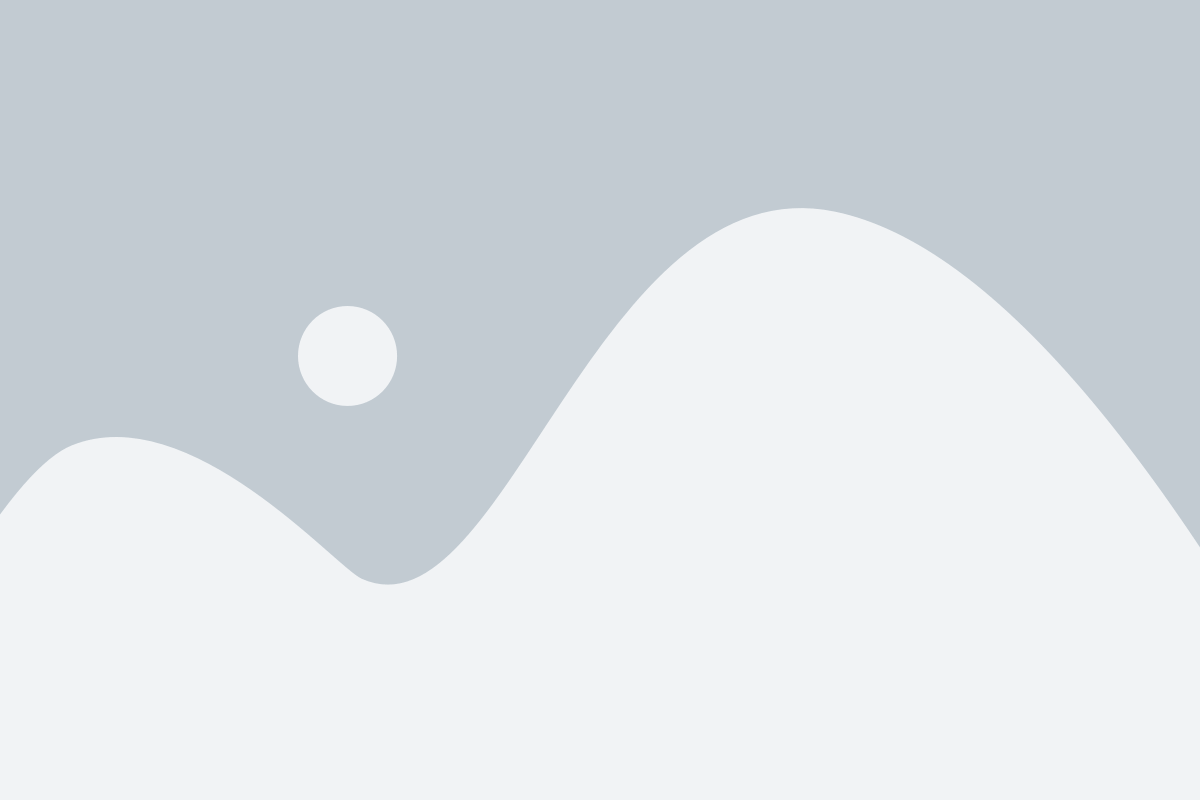
“Enterprise Data Engineering” may sound dated and not so cutting-edge buzzword now. But in this age of several disruptive ideas, concepts and technologies, Enterprise Data Engineering has caught-up with all the necessary advancements and capabilities equally if not better. With organizations becoming more and more keen to be data driven and embrace AI, there is heightened necessity of robust Enterprise Data Engineering Strategy (EDE Strategy). Organizations that do not have efficient EDE Strategy laid out would be ignoring or mismanaging their data asset. Inefficient use of data asset and insights would weaken the competitive edge and their competitors will leap ahead multi-fold in no-time.
EDE Strategy being the master plan of enterprise wide data infrastructure, is a foundational component for any meaningful corporate initiative in recent years. “Explosive growth of data” is being identified as a challenge, an opportunity, a new trend, a significant asset, a source of immense insights, etc. Social media, connected devices and detailed transaction logging are generating huge stream of data which simply can’t be ignored. In simple terms, a panoramic view and wholesome control of a data setup is essential to make data a powerhouse for organizational strategic growth.
Global IP traffic annual run rate is projected to reach 3.3 zettabytes by 2021 (TV & Smartphones together will be accounted for more than 60% of this IP traffic) -CISCO
Along with changing landscape of data, associated disciplines are now aligning to the new demands. EDE Strategy ensures that, these alignments are in line with the roadmap and caters to business users and their requirements.
EDE Strategy encompasses multiple strategy elements that are seeking increased attention now-a-days due to multiple advancements in & around them. Let me introduce some of those strategy elements here:
Automated Data Ingestion & Enrichment
Data Ingestion has now become seriously challenging due to variety & number of sources (social media, new devices, IoT, etc.), new types of data (text stream, video, images, voice, etc), volume of data and the increasing demand for immediately consumable data. Data Ingestion & Enrichment are almost coming together in several scenarios. Due to this, Data Ingestion is almost going off the ETL tools and is fast adopting Pythons and Sparks. Streamed data is not just about absorbing the data as it happens at the source. It is also about enabling AI within the ingestion pipeline to perform data enrichment by bringing together needed data sets (internal & external) and making the Ingestion-to-Insight transformation automatically in seconds/minutes.
ML/AI Models and Algorithms
ML/AI infuses smartness in building data objects, tables, views and models to oversee the data flow across data infrastructure. It uses intelligence in identifying data types/keys/join paths, find & fix data quality issues, identify relationships, identify required data sets to be imported, derive insights, etc. So, the advent of ML/AI in data engineering infuses intelligence into learning, adjusting, alerting and recommending by leaving complex tasks & administration to humans.
Cloud Strategy
The most significant shift seen in the digital world recently is the amount of data being generated and transported. Studies say, 90% of the data existing today were generated in just last 2 years. This is going to increase multi-fold in the coming years. The on-prem based infrastructure and provisioning processes aren’t agile enough to scale rapidly on demand. Even if this is managed, the associated overhead of buying, managing and securing the infrastructure becomes highly expensive and error prone. So, it is essential for organizations to opt for highly efficient and intelligent data platforms on cloud. Cloud offers several advantages across cost, speed, scale, performance, reliability and security. It is also maturing away from initial IaaS into newer services and players. But it doesn’t mean organizations simply initiate the cloud migration and get it done at the press of a button. There should be a carefully drafted Cloud strategy & execution roadmap for adopting cloud in alignment to organization requirements and constraints. Data and information on cloud has the potential to give organizations the flexibility, scalability and ability to discover powerful insights. Cloud also enables applying ML/AI for discovering dark data, monetizing opportunities and disruptive business insights.
Data Lake
Among the data-management technologies most significant space is of data lake. Data Lake is not a specific technology but a concept of housing “one source of truth” data for an organization. When implemented, data lakes can hold and process both structured and unstructured data. Though name indicates huge infrastructure, data lakes are less costly to operate if on cloud. It doesn’t require data to be indexed or prepared to fit specific storage requirements. Instead it holds data in their native formats. Data is then accessed, formatted or reconfigured when needed. Though data lakes are easy to initiate due to easily accessible and affordable cloud offerings, it requires careful planning and incremental adoption model for large scale implementations. In addition, the ever-changing data regulatory & compliance standards add to challenges of implementing and managing data lakes.
Master Data Management (MDM)
Though there is ongoing debate on whether MDM is needed where data lake is the central theme. Schema-on-Write, Schema-on-Read, unstructured data, cloud, etc., are the main contention points in these debates. No matter who wins these debates, it is important for us to know more about MDM while discussing on data engineering. Because, MDM is very essential for organizations to serve their customers/clients near real-time and with better efficiency. As Master Data is the key reference for transactions, typically all independent applications maintain them locally. This leads to redundancy, inconsistency and inefficiency when these data are brought together. It becomes a big challenge while integrating and processing these data due to complexity, chances for errors and increased cost. So, it is important to address MDM element in the EDE strategy carefully by considering organization objectives. Multiple models are practiced for implementing MDM like, registry, hybrid, hub, repository, coexistence, consolidation, etc., which would be discussed in my next articles.
Visualization
Visualization is an exercise that helps in understanding the data in a visual context like patterns, trends, relations, etc. This sounds like an external element or a client to the data engineering. Then, why is Virtualization an important element in EDE Strategy?
Gone are the days of graphs and charts for human analysis. Especially due to data deluge, fitting so much information in a graph or chart is almost impossible for human-beings. They need help in building meaningful representations by consuming huge amount of data. ML/AI is the answer to this wherein its models/algorithms show patterns and correlations by studying huge data-sets in no-time. So, to enable AI to process data, it is important to arrange and label the data suiting it the best. Hence, Visualization is an important aspect to be considered while constructing an Enterprise wide Data Engineering Strategy.
Conclusion
In this new era of data being the new oil, every interest required is being taken to improve the way data is received, cleansed, enriched, assembled and transformed. EDE Strategy ensures establishing effective deployment/management guidelines and continuously improves them because data environments are living organisms. A solid EDE Strategy is highly essential to cater to the demands of this new age. All organizations must have EDE Strategy for realizing their digital vision. Ignoring to have a well laid Enterprise Data Engineering Strategy is as good as regressing in this competitive world.
Related Posts
AIQRATIONS
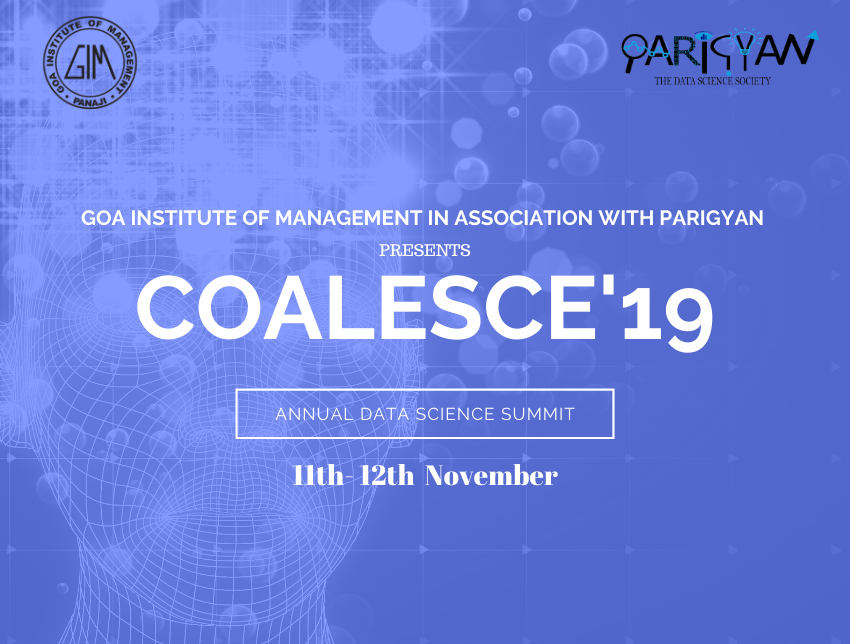
AIQRATE at COALESCE’19 – Goa Institute of Management
Add Your Heading Text Here
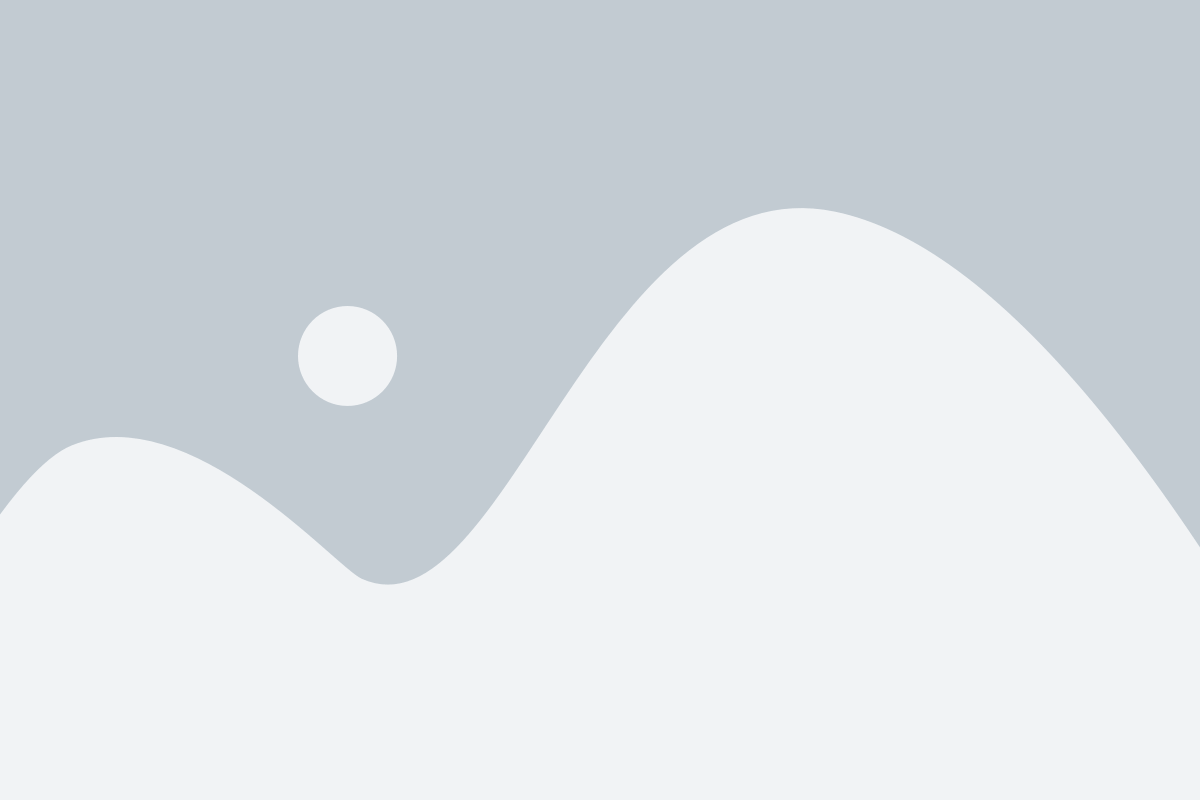
Goa Institute of Management (GIM), organized one of its most anticipated event, the two day long Annual Data Science Summit, Coalesce’19 at its campus in Sanquelim recently where leaders from the world of data science interacted with audiences.
Coalesce (Powered by TVS Credit) saw prominent industry leaders and experts in the field of Data Science and Analytics, delivered informative talks and shared insights on how the industry is embracing innovation as conversations grows around data analytics.
Sameer Dhanrajani, Former Chief Strategy Officer at Fractal Analytics and CEO & Co-Founder of a new venture, AIQRATE advisory & consulting delivered an electrifying session on Artificial Intelligence: The next normal in strategy and business transformation. He spoke about the direct correlation between Data and AI leading to a transformation in decision making and problem-solving.
Avik Sarkar, Head of the Data Analytics Cell of Niti Aayog spoke about the role of Big Data in Public Policies & Governance, emphasizing on how Big Data had been used in different government programs with success.
Shailendra Kumar, Vice president at SAP, addressed the audience nd shared pointers on Making Money Out of Data, and how one could create value for an enterprise using data, predictive analytics and advanced analytics. After hearing his enlightening thoughts, the podium was taken by Moumita Sarker speaking on the topic Forecasting Monsters: What hinders the usage of Forecasting techniques in business decisions. She expedited details on different techniques and scenarios where one could easily build a forecasting model that would solve the growth story.
A panel discussion on the topic: Does Analytics act as an enabler in current business models? saw panellists Srinidhi Shama Rao, Senior Partner with The Math Company, Amit Kurhekar, Global Technology Manager, Aparana Gupta and Sameer Dhanrajani, CEO of AIQRATE share insights in a session moderated by Prof. Hemant Padhiari.
Coalesce ’19 also included a 2-day workshop organized in association with Sankhya on Artificial Intelligence & Machine Learning which witnessed a Datathon Crypto sponsored by TVS credit where about 240 teams participated and the top 10 teams from different B-schools visited the campus for the final round.
– By NewsExperts.in
Related Posts
AIQRATIONS
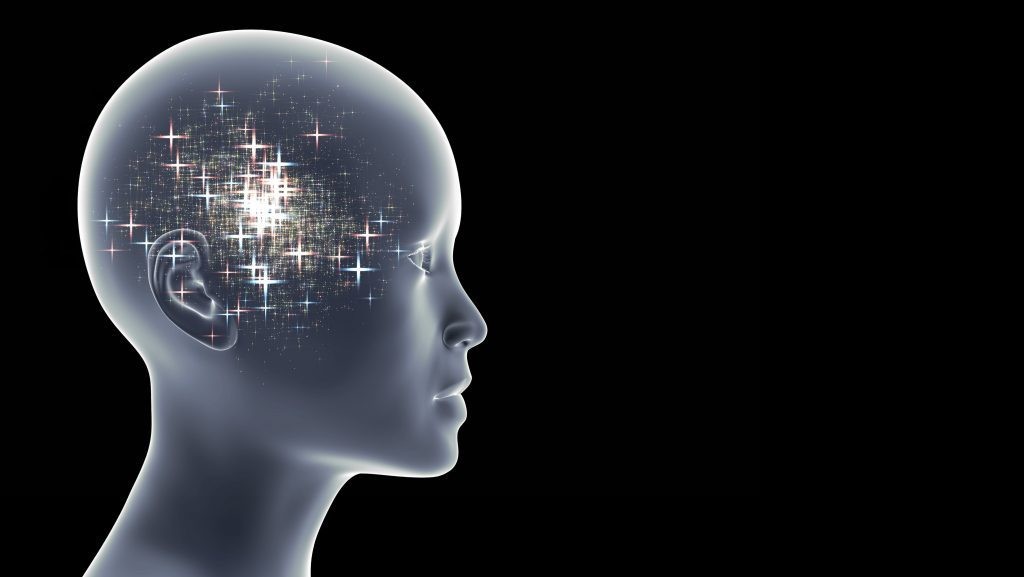
The ‘Dark’ side of AI: Algorithm Bias, influenced decision making.. Defining AI Ethics Strategy
Add Your Heading Text Here
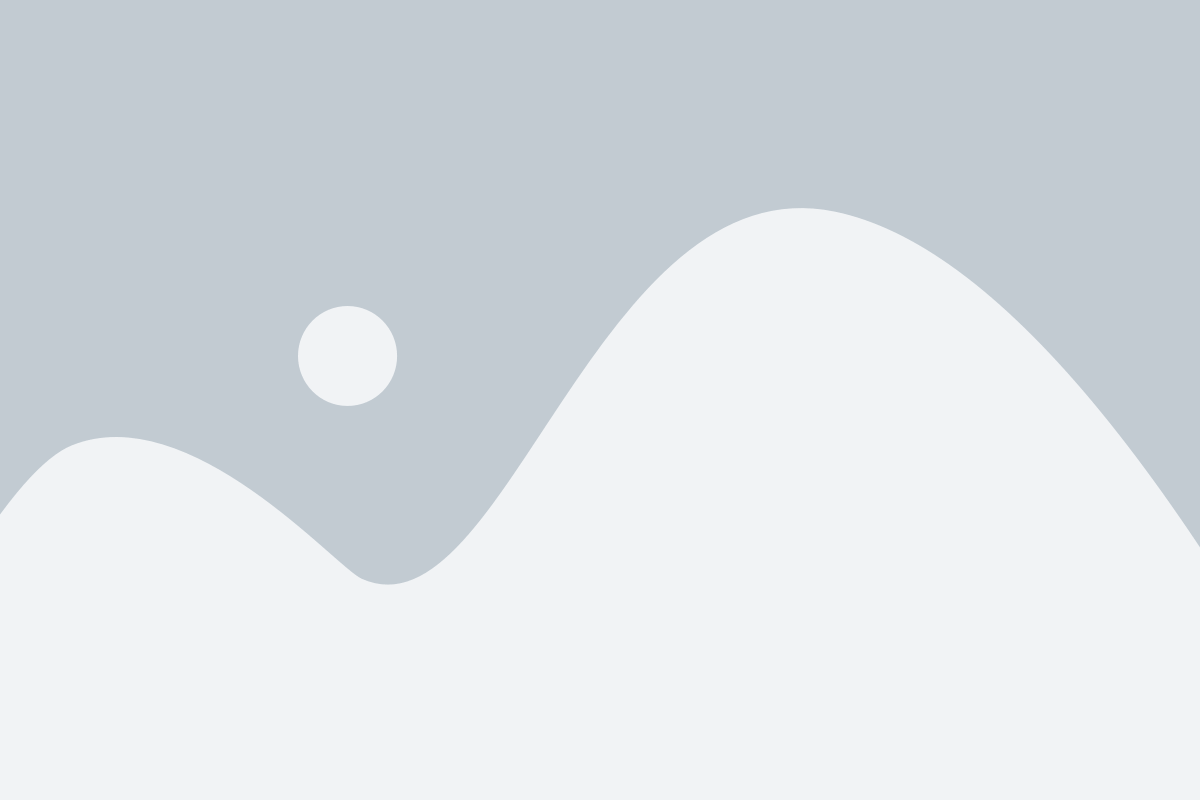
According to a 2019 report from the Centre for the Governance of AI at the University of Oxford, 82% of Americans believe that robots and AI should be carefully managed. Concerns cited ranged from how AI is used in surveillance and in spreading fake content online (known as deep fakes when they include doctored video images and audio generated with help from AI) to cyber attacks, infringements on data privacy, hiring bias, autonomous vehicles, and drones that don’t require a human controller.
What happens when injustices are propagated not by individuals or organizations but by a collection of machines? Lately, there’s been increased attention on the downsides of artificial intelligence and the harms it may produce in our society, from unequitable access to opportunities to the escalation of polarization in our communities. Not surprisingly, there’s been a corresponding rise in discussion around how to regulate AI.
AI has already shown itself very publicly to be capable of bad biases — which can lead to unfair decisions based on attributes that are protected by law. There can be bias in the data inputs, which can be poorly selected, outdated, or skewed in ways that embody our own historical societal prejudices. Most deployed AI systems do not yet embed methods to put data sets to a fairness test or otherwise compensate for problems in the raw material.
There also can be bias in the algorithms themselves and in what features they deem important (or not). For example, companies may vary their product prices based on information about shopping behaviors. If this information ends up being directly correlated to gender or race, then AI is making decisions that could result in a PR nightmare, not to mention legal trouble. As these AI systems scale in use, they amplify any unfairness in them. The decisions these systems output, and which people then comply with, can eventually propagate to the point that biases become global truth.
The unrest on bringing AI Ethics
Of course, individual companies are also weighing in on what kinds of ethical frameworks they will operate under. Microsoft president Brad Smith has written about the need for public regulation and corporate responsibility around facial recognition technology. Google established an AI ethics advisory council board. Earlier this year, Amazon started a collaboration with the National Science While we have yet to reach certain conclusions around tech regulations, the last three years have seen a sharp increase in forums and channels to discuss governance. In the U.S., the Obama administration issued a report in 2016 on preparing for the future of artificial intelligence after holding public workshops examining AI, law, and governance; AI technology, safety, and control; and even the social and economic impacts of AI. The Institute of Electrical and Electronics Engineers (IEEE), an engineering, computing, and technology professional organization that establishes standards for maximizing the reliability of products, put together a crowdsourced global treatise on ethics of autonomous and intelligent systems. In the academic world, the MIT Media Lab and Harvard University established a $27 million initiative on ethics and governance of AI, Stanford is amid a 100-year study of AI, and Carnegie Mellon University established a centre to explore AI ethics.
Corporations are forming their own consortiums to join the conversation. The Partnership on AI to Benefit People and Society was founded by a group of AI researchers representing six of the world’s largest technology companies: Apple, Amazon, DeepMind/Google, Facebook, IBM, and Microsoft. It was established to frame best practices for AI, including constructs for fair, transparent, and accountable AI. It now has more than 80 partner companies. Foundation to fund research to accelerate fairness in AI — although some immediately questioned the potential conflict of interest of having research funded by such a giant player in the field.
Are data regulations around the corner?
There is a need to develop a global perspective on AI ethics, Different societies around the world have very different perspectives on privacy and ethics. Within Europe, for example, U.K. citizens are willing to tolerate video camera monitoring on London’s central High Street, perhaps because of IRA bombings of the past, while Germans are much more privacy oriented, influenced by the former intrusions of East German Stasi spies , in China, the public is tolerant of AI-driven applications like facial recognition and social credit scores at least in part because social order is a key tenet of Confucian moral philosophy. Microsoft’s AI ethics research project involves ethnographic analysis of different cultures, gathered through close observation of behaviours, and advice from external academics such as Erin Meyer of INSEAD. Eventually, we could foresee that there will be a collection of policies about how to use AI and related technologies. Some have already emerged, from avoiding algorithmic bias to model transparency to specific applications like predictive policing.
The longer take is that although AI standards are not top of the line sought after work, they are critical for making AI not only more useful but also safe for consumer use. Given that AI is still young but quickly being embedded into every application that impacts our lives, we could envisage an array of AI ethics guidelines by several countries for AI that are expected to lead to mid- and long-term policy recommendations on AI-related challenges and opportunities.
Chief AI ethical officer on the cards?
As businesses pour resources into designing the next generation of tools and products powered by AI, people are not inclined to assume that these companies will automatically step up to the ethical and legal responsibilities if these systems go awry.
The time when enterprises could simply ask the world to trust artificial intelligence and AI-powered products is long gone. Trust around AI requires fairness, transparency, and accountability. But even AI researchers can’t agree on a single definition of fairness: There’s always a question of who is in the affected groups and what metrics should be used to evaluate, for instance, the impact of bias within the algorithms.
Since organizations have not figured out how to stem the tide of “bad” AI, their next best step is to be a contributor to the conversation. Denying that bad AI exists or fleeing from the discussion isn’t going to make the problem go away. Identifying CXOs who are willing to join in on the dialogue and finding individuals willing to help establish standards are the actions that organizations should be thinking about today. There comes the aspect of Chief AI ethical officer to evangelize, educate, ensure that enterprises are made aware of AI ethics and are bought into it.
When done correctly, AI can offer immeasurable good. It can provide educational interventions to maximize learning in underserved communities, improve health care based on its access to our personal data, and help people do their jobs better and more efficiently. Now is not the time to hinder progress. Instead, it’s the time for enterprises to make a concerted effort to ensure that the design and deployment of AI are fair, transparent, and accountable for all stakeholders — and to be a part of shaping the coming standards and regulations that will make AI work for all