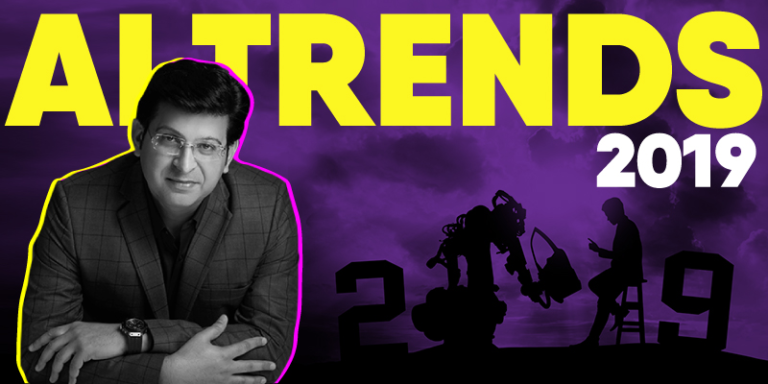
Here are the top 10 AI trends to watch out for in 2019
Add Your Heading Text Here
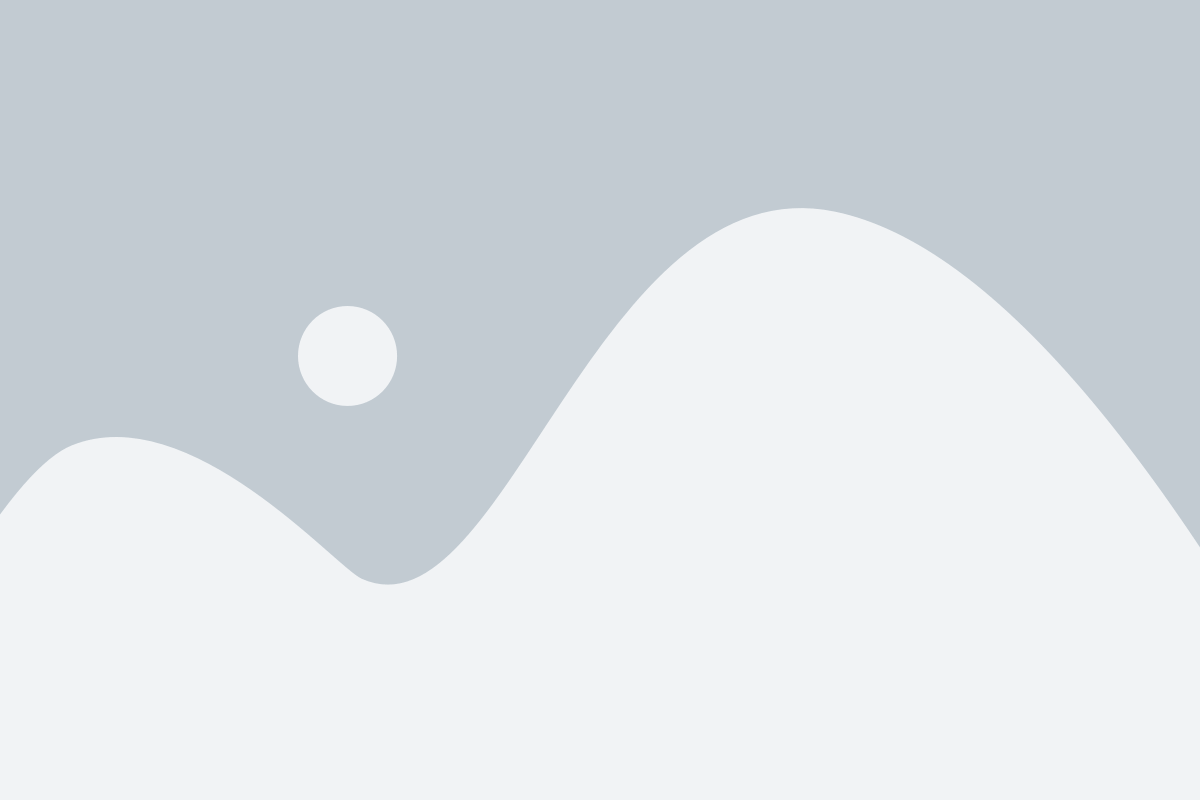
The year 2018 will be remembered as the year that artificial intelligence stopped being on the periphery of business and entered the mainstream realm. With increasing awareness and capability of AI among the numerous stakeholders, including tech buyers, vendors, investors, governments, and academia, I expect AI will go beyond just tinkering and experiments and will become the mainstay in the business arena.
With an increasing percentage of these stakeholders professing their commitment to leveraging this technology within their organisations, AI has arrived on the world scene. We are sure to see transformative business value being derived through AI in the coming years. As we come to the close of 2018, let us gaze into the crystal ball to see what 2019 will hold for this game-changing technology:
The rise of topical business applications
Currently, we have a lot of general purposes AI frameworks such as Machine Learning and Deep Learning that are being used by corporations for a plethora of use cases. We will see a further evolution of such technology into niche, topical business applications as the demand for pre-packaged software with lower time-to-value increases. We will see a migration from the traditional AI services paradigm to very specific out-of-the-box applications geared to serve particular use cases. Topical AI applications in this space that serve such use cases will be monumentally useful for furthering the growth of AI, rather than bespoke services that require longer development cycles and may cause bottlenecks that enterprises cannot afford.
The merger of AI, Blockchain, cloud, and IoT
Could a future software stack comprise AI, Blockchain, and IoT running on the cloud? It is not too hard to imagine how these exponential technologies can come together to create great value. IoT devices will largely be the interface with which consumers and other societal stakeholders will interact. Voice-enabled and always connected devices – such as Google Home and Amazon’s Alexa – will augment the customer experience and eventually become the primary point of contact with businesses. AI frameworks such as Speech Recognition and Natural Language Processing will be the translation layer between the sensor on one end and the deciphering technology on the other end. Blockchain-like decentralised databases will act as the immutable core for managing contracts, consumer requests, and transactions between various parties in the supply chain. The cloud will be the mainstay for running these applications, requiring huge computational resources and very high availability.
Focus on business value rather than cost efficiency
2019 will finally be the year that majority of the executive and boardroom conversations around AI will move from reducing headcount and cost efficiency to concrete business value. In 2019, more and more businesses will realise that focusing on AI solutions that reduce cost is a criminal waste of wonderful technology. Ai can be used to identify revenues lost, plug leakages in customer experience, and entirely reinvent business models. I am certain that businesses that focus only on the cost aspect will stand to lose ground to competitors that have a more cogent strategy to take the full advantage of the range of benefits that AI offers.
Development of AI-optimised hardware and software
Ubiquitous and all-pervasive availability of AI will require paradigm shifts in the design of the hardware and software that runs it. In 2019, we will see an explosion of hardware and software designed and optimised to run artificial intelligence. With the increasing size and scale of data fueling AI applications and even more complex algorithms, we will see a huge demand for specialised chipsets that can effectively run AI applications with minimal latency. Investors are showing heavy interest in companies developing GPUs, NPUs, and the like – as demonstrated by Chinese startup Cambricon, which stands valued at a whopping $2.5 billion since its last round of funding this year. End-user hardware such as smart assistants and wearables will also see a massive increase in demand. Traditional software paradigms will also continue to be challenged. Today’s novel frameworks such as TensorFlow will become de rigueur. Architectural components such as edge computing will ensure that higher processing power is more locally available to AI-powered applications.
‘Citizen AI’ to be the new normal
One of the reasons we saw widespread adoption of analytics and data-driven decision-making is because we built applications that democratised the power of data. No longer was data stuck in a remote silo, accessible only to the most sophisticated techies. With tools and technology frameworks we brought data into the mainstream and made it the cornerstone of how enterprises plan and execute strategy. According to Gartner, the number of citizen data scientists will grow five times faster than the number of expert data scientists. In 2019, I expect Citizen AI to gain traction as the new normal. Highly advanced AI-powered development environments that automate functional and non-functional aspects of applications will bring forward to a new class of “citizen application developers”, allowing executives to use AI-driven tools to automatically generate new solutions.
Policies to foster and govern AI
Following China’s blockbuster announcement of a National AI Policy in 2017, other countries have rushed to share their take on policy level interventions around AI. I expect to see more countries come forward with their versions of a policy framework for AI – from overarching vision to allaying concerns around ethical breaches. At the same time, countries will also be asked to temper their enthusiasm of widespread data proliferation by releasing their own versions of GDPR-like regulations. For enabling an ecosystem where data can be used to enrich AI algorithms, the public will need to be convinced that this is for the overall good, and they have nothing to fear from potential data misuse and theft.
Speech Recognition will revolutionise NLP
In the last few years, frameworks for Natural Language Understanding (NLU) and Natural Language Generation (NLG) have made huge strides. NLP algorithms are now able to decipher emotions, sarcasm, and figures of speech. Going forward, voice assistants will use data from voice and combine that with deep learning to associate the words spoken with emotions, enriching the overall library that processes speech and text. This will be a revolutionary step forward for fields such as customer service and customer experience where many bots have typically struggled with the customer’s tone of voice and intonation.
The growth of explainable AI
And finally, with numerous decisions powered by AI – and specifically unsupervised learning models – we will see enterprises demand “explainable” AI. In simplified terms, explainable AI helps executives “look under the hood” to understand the “what” and “why” of the decisions and recommendations made by artificial intelligence. Development of explainable AI will be predicated on the need for increased transparency and trust. Explainable AI will be essential to ensure that there is some level of transparency (and potentially, learning) that is gleaned from unsupervised systems.
Convergence of AI and analytics
This is a trend that is a logical consequence of the decisive power of data in business today. In 2019, we will see a merger of analytics and AI – as the one-stop for uncovering and understanding insights from data. With advancements in AI seen so far, the algorithms are more than capable of taking up tasks that involve complex insight generation from multi-source, voluminous data. This convergence of AI and analytics will lead to automation that will improve the speed and accuracy of the decisions that power business planning and strategy. AI-powered forecasting will help deliver faster decisions, with minimal human interventions and create higher cost savings for the business.
Focus on physical and cybersecurity paradigms
Two of the domains ripe for an AI transformation are the fields of physical and cybersecurity. As intrusions into physical and virtual environments become commonplace and threats become hugely pervasive, AI will be a massive boost to how we secure these environments. Advances in fields such as ML-powered anomaly detection will drastically reduce the time required to surface potential intrusions into secure environments. This will enable organisations to better protect user data. When combined with Blockchain, AI will give cybersecurity a huge boost through decentralised, traceable databases containing valuable client and strategic information. On the physical security side, Computer Vision is rapidly gaining currency in the fields of physical intruder detection. Surveillance cameras, originally manned by security guards, will soon be replaced by AI-powered systems that will be able to react faster and more proactively to intruders that pose a threat to physical premises. When you combine that with face recognition, working with a database of known offenders, we will see a quantum drop in the time required to adjudicate and address cases of theft and unauthorised entry by law enforcement agencies.
In summary, the broad directions that I predict AI will take include interventions to make it more embedded, responsible, and explainable; convergence with other exponential technologies such as cloud, Blockchain, and IoT; cybersecurity; a greater proliferation and development of use cases; and great strides in the technology and its supporting infrastructure. Enterprises would do well to adopt this revolutionary technology and ensure a strong availability of talent to conceptualise, develop, and unleash value from AI applications.
Related Posts
AIQRATIONS

3 Ways To Human Centric AI
Add Your Heading Text Here
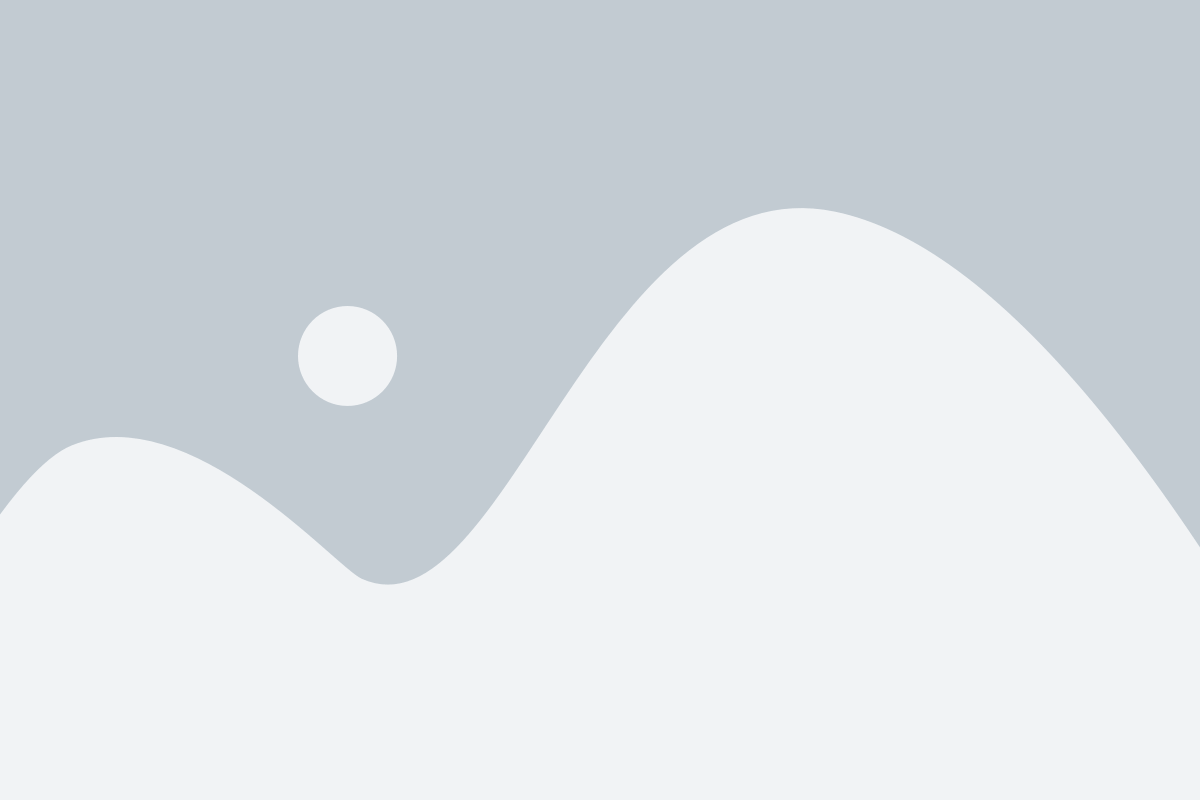
Three Ways to Facilitate a Symbiotic Relationship Between Cognitive Intelligence and Behavioral Sciences
After every conference I speak at about the transformative power of Artificial Intelligence and its potential to unlock untold business value, the one question that often crops up from the audience is.. if AI is expected to perform much of the grunt work in the enterprise world, what scope is there for the so-called ‘human’ qualities?
Is the future of business and technology so deeply intertwined that it leaves virtually no scope in the future for the vagaries of human intelligence and behavior? The answer is quick and simple – absolutely not. Artificial Intelligence, while a great paradigm-shifter in the world of business, is still one of the tools that will be used by humans for making better decisions. At the end of the day, AI will still be developed and used by humans. And maintaining the ‘human element’ in the way it is made, delivered, used and improved will most certainly make it a lot more successful. AI exists to make human life simpler and richer – and hence it is critical that AI practitioners and data scientists adopt a human-centric approach to its development, deployment and adoption. Even the best AI will become quickly redundant without inputs from real humans on how to accelerate strategic decisions and processes.
How do we then build in that ‘human element’ into our Artificial Intelligence tools? This is where humanities-centric subjects of design and behavioral science come into the picture. What is behavioral science? Simply put, it is the study of internal cognitive processes of humans and societies and how these processes manifest into external perceptible and imperceptible actions and interactions. Behavioral science typically stands at a nexus of various subjects – borrowing aspects from sociology, anthropology, psychology and even economics and political science. Its interdisciplinary nature precludes the scale of impact it can have if applied correctly. In technology, and specifically in AI, behavioral science will and should impact how we build, use and interact with technology.
I see primarily three key areas where the symbiosis of AI-led cognitive intelligence systems and behavioral science can unlock massive value for enterprises that marry these two starkly different, but extremely complementary fields of study:
Appeal to the Non-Conscious
We have known for nearly a century now that a large majority of human biases, inferences, preferences and reactions are largely controlled by the dark recesses of our non-conscious brain. For technologists to build successful AI products, that are widely adopted and used they need to reach out inside the non-conscious parts of the human brain and orchestrate responses from there.
In AI technology specifically, user adoption is often the difference between make and break for products. Numerous AI products are mostly informed by the data they gather from human actions and their preferences. This data feeds the algorithms running in the background and makes them more sophisticated to better understand their human overlords. To that end, AI products need to have a strong underpinning in behavioral science, so that they can appeal to the non-conscious and improve adoption.
Take for example the work done by Nir Eyal for his book, ‘Hooked: How to Build Habit Forming Products.’ In the book, Eyal writes about multiple ways in which human subjects get applied to technology development. One of them is the Hook canvas – a loop comprising triggers, actions, rewards and investments – which are the cornerstone features of every addictive software you’ve used – from Facebook to Instagram, Snapchat and YouTube. Another is the idea of using the trinity of emotion, features and incentives – extremely relevant ideas to anyone working on building AI products. Another example comes from Worxogo – an Indian startup that employs behavioral design, neuroscience in tandem with predictive analytics to enhance employee performance through nudges to the non-conscious.
Build with Humans
Not only is AI built to serve humans, it is also built by humans. To that end, it becomes extremely important to consider what emotional triggers help define what we build and how we build it. Again, behavioral science practitioners have a key role to play in order to engage empathy in defining the requirements and going about the development of AI. Learnings from behavioral science can bring to light immeasurably important interventions for how we manage and lead teams, collaborate between a team and across multiple teams – all while maintaining a high level of motivation by appealing to a higher sense of purpose. It is worth examining how something as simple as empathy can be extremely valuable in how we build software. For instance, with improved self-awareness and empathy, developers can feel an intrinsic desire to write cleaner code while maintaining proper documentation. Also, given that AI is largely deployed using the DevOps methodology – empathy can be the difference between whether we can build a trust-based bridge between how we build, deploy and automate releases faster.
Beyond the ‘how’ of AI development, behavioral science can also contribute meaningfully to the ‘what’. Currently a lot of concern around AI is related to ethics – will AI lead to loss of meaningful work for humans? What data privacy issues can rear their head when we deploy large-scale data capture systems to improve our algorithms? We need to move the dial from apathy to empathy in the process of conceptualizing software – and knowledge of behavioral science will undoubtedly help AI practitioners develop more responsible AI.
Artificial Emotional Intelligence
The third key application of behavioral science – and possibly the most game-changing of the lot is – how can we apply behavioral science to make our systems more ‘human’? Is it possible to add a dash of EQ to these high IQ systems?
I certainly think there is a huge scope for developing AI that has a strong human bent. Consider the applications we are building today with AI and robotics – companions for the elderly, coaching apps for autistic children, even something as comparatively mundane is chatbots for customer service. Behavioral science holds the key to achieving the holy grail of how we can better balance the human-machine equation, by infusing human qualities into artificial systems.
To enable this, it is important to know who we are building for and what are their intrinsic and non-conscious needs. Behavioral science holds the clues that can complement AI’s ability to eliminate biases, while serving the emotional needs of humans. For example, StressSense tracks when people are highly stressed and helps them avoid anxious situations. This kind of breakthrough research can help in multiple AI applications, teaching them how to behave with humans, while ensuring a strong impact.
As technology providers and businesses work together to build transformational artificial intelligence systems and data science teams, it is very important to consider the human element. These teams would do well to develop a better understanding of whom the AI is built for and how it is used – through techniques offered by behavioral science. Balancing the human-machine equation and powering a complementary relationship between AI systems and the people who use them necessitates an infusion of behavioral science into the process. Ultimately, for AI to succeed, we need both – the foresight of technology as well as the insight of humans.