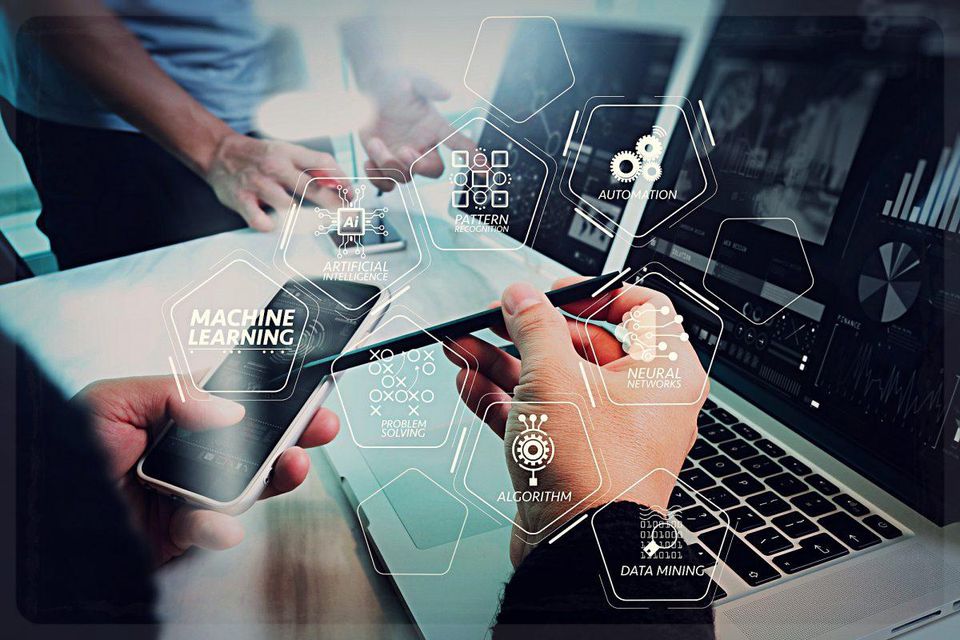
Reimagining Enterprise Decision-Making With Artificial Intelligence
Add Your Heading Text Here
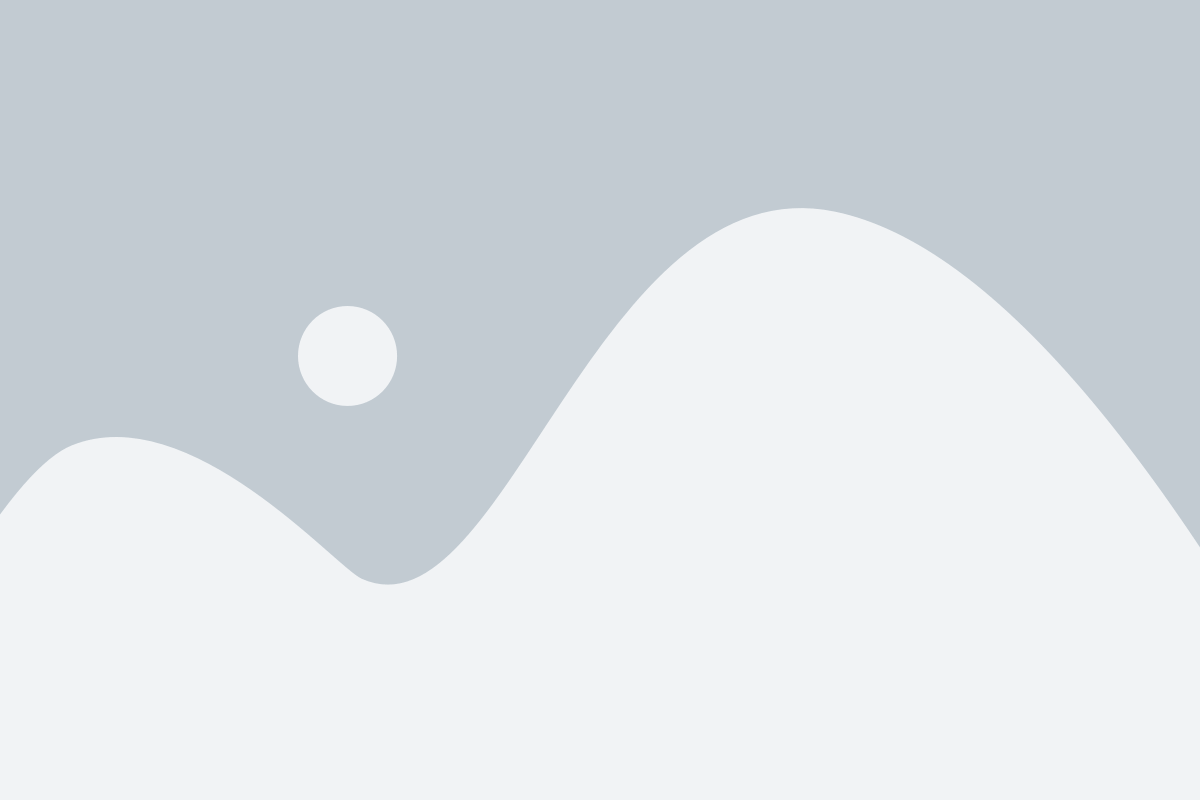
Artificial Intelligence will deliver revolutionary impact on how enterprises make decisions today. In the last few years alone, we have rapidly moved beyond heuristics-based decision-making to analytics-driven decision-support. In the VUCA phase, businesses globally are now pivoting to an AI-led, algorithm-augmented style of decision-making. With huge computing power and ever-increasing data storage and analytics prowess, we are entering a new paradigm, a probable and interesting scenario wherein, Artificial Intelligence will play a huge role in augmenting human intelligence and enabling decision-making with complete autonomy. The big hope is that this new paradigm will not only reduce human biases and errors that are common with heuristic decisions, but also reduce the time involved in making these critical decisions.
Here, I’ll attempt to focus on how we moved from simpler data driven decision-support to AI-powered decisions. The evolution of this technology has been breathtaking to behold and just might provide clues as to what we can expect in the future. Further, I’ll cover a few critical aspects that need to be inculcated by organizations on the AI transformation journey, and provide a few insightful cues that will make this journey exciting and fruitful.
Transformation of Decision-Making: From Analytics to AI
First, let us look at how we got here. Some truly pathbreaking events happened along the way while we were trying to make more accurate business decisions, leading us to reimagine how decisions will be made in the enterprise.
Organizations are Becoming Math Houses
With data deluge and digital detonation, combined with the appreciation of the fact that robust analytical capabilities lead to more informed decisions, we are witnessing AI savvy organizations rapidly maturing into ‘math houses.’ Data science – the ability to extract meaningful insights out of data has become de rigueur. Why? Because we now know that data, when seen in isolation, is inherently dumb. It is the ability to process this data and identify patterns and anomalies – using sophisticated algorithms and ensemble techniques – that makes all the difference. These self-intuitive algorithms are where real value resides – as they define the intelligence required to uncover insights and make smart recommendations. Organizations today are evolving into algorithm factories. There is a real understanding today that by enabling continuous advancement in mathematical algorithms, we can deliver consistent decisions based on prescribed as well as evolving business rules.
It is now an established reality that companies with robust mathematical capabilities possess a huge advantage over those that don’t. Indeed, it’s this math-house orientation that separates companies like Amazon and Google from the ones they leave in their wake, with their ability to understand their customers better, identify anomalies and recognize key patterns.
AI: From Predictive to Prescriptive
We saw a similar evolution in the age of analytics – wherein the science and value veered from descriptive analytics, providing diagnostics of past events to prescriptive analytics, helping see and shape the future. We are seeing a similar evolution in how AI gets leveraged in the enterprise and where its maximum value lies.
In early implementations, it was common to see AI as just a tool to predict and forecast future conditions, while accounting for the dynamism seen in the external environment. Today, AI-enabled decision-making is more prescriptive, with AI providing enterprises not just a look into the future, but also key diagnostics and suggestions on potential decision options and their payoffs. Such evolved applications of AI can help businesses make decisions that can potentially exploit more business opportunities, while averting potential threats much earlier.
Mr. Algorithm to Drive Decision Making
The culmination of this AI-era advancement would be the introduction of smart algorithms in every walk of life and business. Algorithms will become further mainstream leading to what will be the most sweeping business change since the industrial revolution. Organizations – those that already aren’t – will start developing a suite of algorithmic IP’s that will de-bias most enterprise decisions.
If Mr. Algorithm is going to drive most enterprise decisions of tomorrow, we need to create some checks and balances to ensure that it does not go awry. It is more critical today than ever before that the algorithmic suite developed by enterprises has a strong grounding in ethics and can handle situations appropriately for which explicit training may not have been provided.
How to Enable this AI Era of Change
Ushering into an AI-centric era of decision-making will require organizational transformation from business, cultural and technical standpoints. The following facets will be the enablers of this change:
Developing an Engineering Mindset
Instrumenting AI in the enterprise requires a combination of data scientists and computer scientists. As AI matures in the enterprise, the users, use cases and data will increase exponentially. To deliver impactful AI applications, scale and extensibility is critically important. This is where having an engineering mindset comes in. Imbibing an engineering mindset will help standardize the use of these applications while ensuring that they are scalable and extensible.
Learning, Unlearning, Relearning
The other critical aspect to a culture where AI can thrive is creating an environment supporting continuous unlearning and relearning. AI can succeed if the people developing and operating it are rewarded for continuous experimentation and exploration. And just like AI, people should be encouraged to incorporate feedback loops and learn continuously. As technology matures it’s important that the existing workforce keeps up. For one, it’s critical that the knowledge of algorithm theory, applied math alongside training on AI library and developer tools, is imparted into the workforce – and is continuously updated to reflect new breakthroughs in this space.
Embedding Design-Thinking and Behavioral Science at the Center of this Transformation
Finally, given the nature of AI applications, it’s critical that they are consumed voraciously. User input very often activates the learning cycles of artificial intelligence applications. To ensure high usage of these applications, it’s very important that we put the user at the center while designing these applications. This is where the application of behavioral sciences and human-centered design will deliver impact. By imparting empathy in these applications for the user, we will be able to design better and more useful AI applications.
As we augment decision-making with algorithmic, AI-centered systems and platforms – the big expectation is that they will bring untold efficiencies in terms of cost, alongside improvement in the speed and quality with which decisions get made. It’s time to reimagine and deliver on enterprise decision-making that is increasingly shaped through artificial intelligence. These aspects – how the AI is progressing and how to exploit its potential are of paramount importance to keep in mind for an AI transformation.
Related Posts
AIQRATIONS
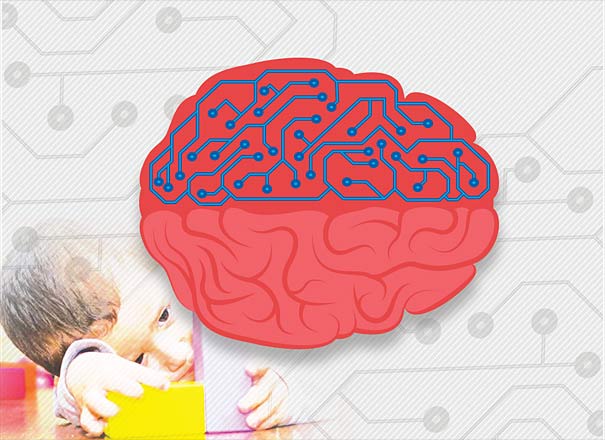
Autism care with AI
Add Your Heading Text Here
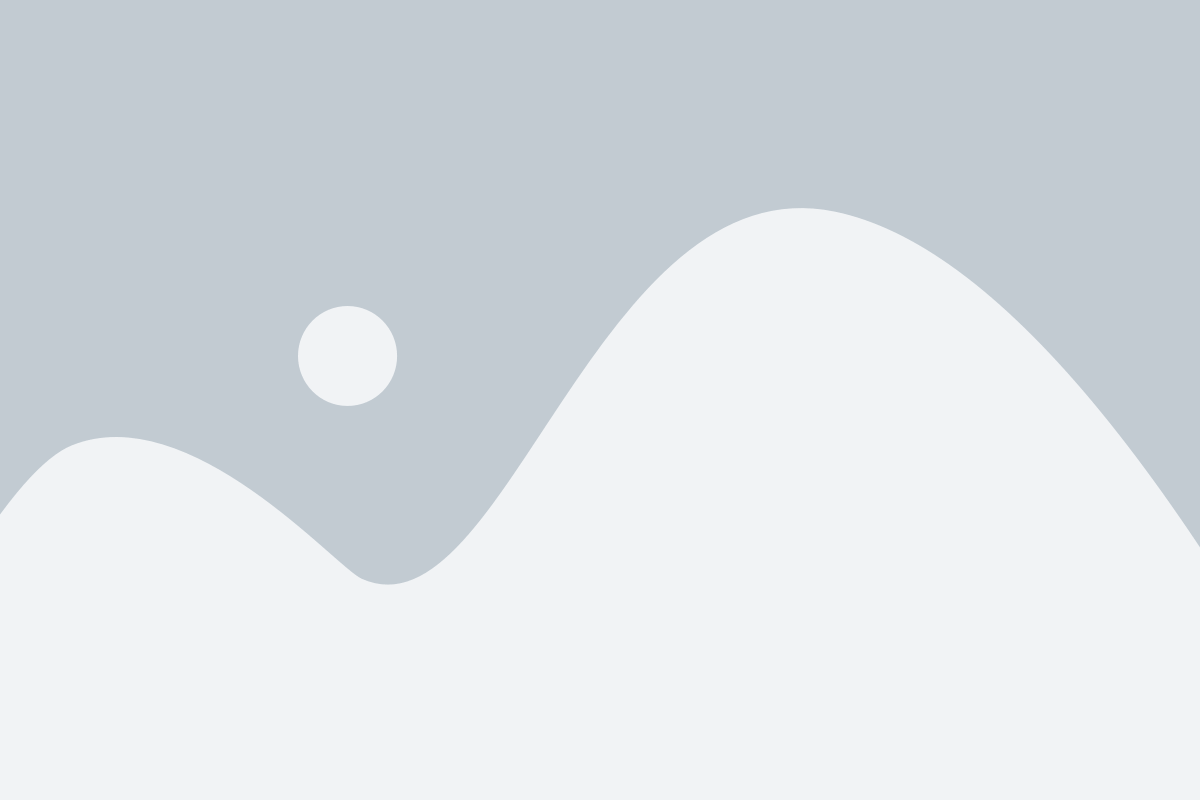
In these columns, I have earlier attempted to highlight the possibilities of multiple game-changing applications in the field of artificial intelligence (AI) that hold the potential to deliver positive benefits to humanity. AI can do much more beyond enabling a transformative business impact. Every technological advancement brings with it an opportunity to deliver positive changes to society. It is no different with AI. With massive increases in computing power and data deluge, it is possible for AI to be a harbinger of change for the society we live in.
A complex problem that we see is Autism Spectrum Disorder. As of 2015, this neurodevelopment disorder is estimated to have affected the lives of 24.8 million people globally. Worse, despite all the advances in medical sciences, there is no conclusive understanding of its causes and cures. While we do see cases where autism gets resolved, we are still unable to point concretely to any medical option that works better than others.
At best, autism can be managed – possibly through a mix of early diagnosis and continuous therapy. Thankfully, we live in an era where the awareness of autism is on the rise and the associated stigma is declining. By harnessing the power of AI to detect and manage cases of autism, we could potentially help those suffering from it lead a fulfilling life of dignity and respect.
While not conclusively proven, it is likely that an early diagnosis of autism is hugely beneficial for managing it in the future. An early detection would also help the people around these children be better attuned to their condition and enable them to set an environment that is conducive to their development.
One such application is applying AI to analyse vocal and behavioural cues exhibited by children. Stephen Sheinkopf — an autism researcher and psychologist at the Brown University Center for the Study of Children at Risk — believes anomalous crying patterns of babies might serve as an early warning sign of autism.
Neurological cues present in the acoustic features of cries — pitch, energy and resonance — might hold the key to early detection. Combining vocal, behavioural and physiological data, we might be able to piece together a pathway to early detection. That’s where AI and machine learning can add really great value, in integrating these disparate pieces of information that might otherwise be hard to make sense of.
An excellent example of this in action is Chatterbaby — conceptualised and developed by Ariana Anderson, a computational neuropsychologist at UCLA. Earlier, functionality enabled identification of potential causes for why a baby is crying by monitoring crying patterns. In addition, Chatterbaby is also attempting to identify if there are discernible differences in the crying patterns of autistic children and neurotypical ones — ultimately aiming to isolate the characteristics of each group to detect autism early.
Early ‘Testing’
Researchers in the UK and Italy have turned to AI for developing what might be the world’s first ‘test’ for autism. In their study, they leveraged AI to compare the protein levels in the blood samples of two groups of children — one group comprising 38 children diagnosed with autism, and the other with 31 children without the diagnosis. Their findings helped develop an algorithm that could test autism — with a 90% accuracy for which children have autistic spectrum disorder and an 87% accuracy for which children do not have it.
Another example is through tracking changes in brain function of six-month-old babies, which researchers from UNC Chapel Hill and Washington University’s School of Medicine believe can help early detection. They recently published a paper wherein they examined the brain scans of 59 high-risk babies to understand the connections and interactions between different regions of the brain. Post this, they analysed the brain scans of the 11 babies that were eventually diagnosed with autism.
By combining the data with AI and deep learning, they developed an algorithm able to detect the possibility of autism with an accuracy of 9 out of 11.
Chatbots and Virtual Assistants
Across studies, we see children with ASD have high levels of comfort with computers due to their predictable and logical nature. Autistic children can perceive humans to be emotional and unpredictable but computer-based systems (even those with human expression) to be rational and non-judgmental.
Systems such as chatbots and social robots can help capture and track the progress of autistic children, continuously monitor their social behaviours and make quick, informal assessments in school and at home. The big promise of these systems is allowing autistic children the opportunity to navigate social interactions, unfamiliar environments while aiding them to reach the developmental goals usually set for neurotypical children.
An example of this is an app called Companion, featuring a virtual assistant named Abby. Abby helps identify interests and needs of autistic children and provides support throughout the day. Beyond this app, Identifier identifies talents of autistic children through interactive game-play; eventual results are compiled onto a dashboard detailing the skills and shortcomings of the child.
A Strict No-No
While AI can bring these benefits, it is also important to ensure that these systems are designed with empathy for the autistic population. For instance, the use of bright, jarring colours is an absolute no-no for the design of such applications. Secondly, virtual agents should be enabled to speak in simple language, without the use of idioms, euphemisms or figures of speech to help such people understand instructions much better.
For a problem that has no apparent cause or cure, AI could be a breakthrough in improving the quality of life of those who suffer from autism and the people around them. By using AI for early diagnosis, disease management and people enablement, we would be able to help bring dignity to the lives of affected people.
Related Posts
AIQRATIONS
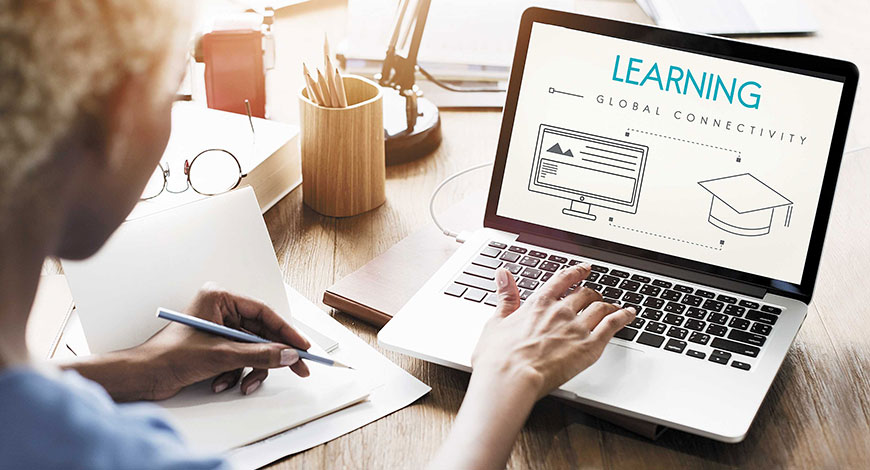
Promoting AI Research In India
Add Your Heading Text Here
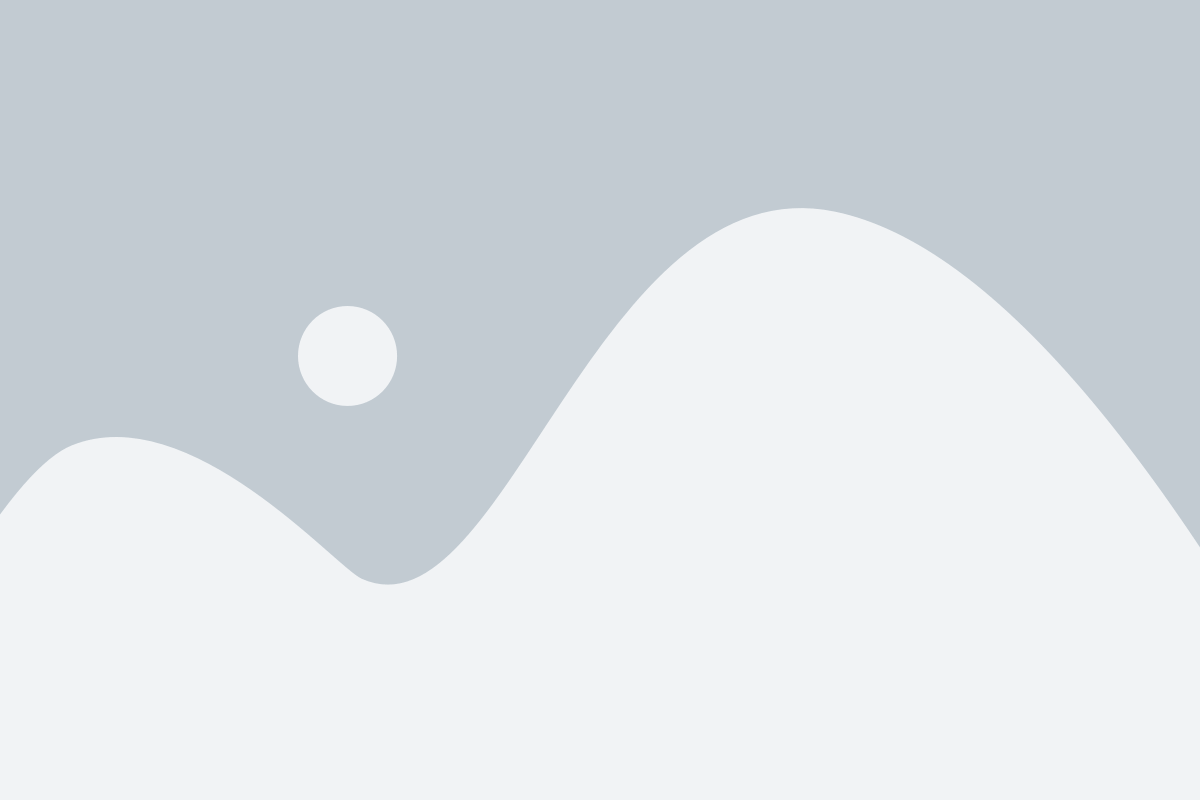
In the academic, industry forums and conferences, India is being positioned as a potential superpower in the field of AI on the world stage. It is our collective vision to see India as the premier destination of AI in the foreseeable future. There is seemingly a lot of work to be done if we are to overtake our formidable competitors and eventually the current leaders in the space of AI – the US and China. But no intervention is perhaps as urgent as the need to promote natively developed AI research and intellectual property within our academic institutions, universities and corporate enterprises.
China spends 2.07% of its GDP on core research and development. In India, that number is a meagre 0.6%. Given this, it should not be surprising that China produced 4.5 times the number of citable research documents in the field of AI between 2010 and 2016. In terms of citations, the H-index of papers published in India (100) lags well behind China (195) and the US (413) – for the same period. At this juncture, it is evident that India’s contribution to the overall body of knowledge of Artificial Intelligence has been both quantitatively and qualitatively disappointing.
The contribution from Indian corporate enterprises has also been found to be wanting. For the period between 2001 and 2016, corporates contributed to merely 14.42% of the AI research done in India, with almost 70% of that being done by foreign multinationals doing business in India – the likes of Microsoft, Google, IBM and others.
There is a definite need for both academia and Indian industry to step up and contribute to India’s vibrancy in the domain of artificial intelligence. Here are 5 critical interventions needed urgently to take our research capabilities to the next level:
Foster a collaborative approach to IP development
There is often a pattern of multiple research projects happening in silos – through collaboration between like-minded researchers from the same field of science. Artificial Intelligence on the other is an interdisciplinary subject. It essentially requires researchers from different walks of life – data engineers, machine learning scientists and those conversant with real-life challenges that AI can solve – to come together to solve common problems and add to the overall body of knowledge.
Universities need to quickly recognize that and build research capabilities in AI in an interdisciplinary, collaborative manner. The good thing is, universities by their very nature tend to host brilliant minds from multiple fields, but it is imperative that these minds be brought together for real, cutting-edge research to be published in the field of AI
Boost Funding for Research at Universities
Whilst India does not lag in the number of STEM graduates, our research capability is still inadequate. This is partly because of a lack of funding for research. For millennials, the allure of a corporate job or opting for entrepreneurship is far too high due to the compensation and benefits on offer.
It is critical that local and national governments intervene to help academic research in the field of AI be a viable career option for those who are interested in research. By expanding the budget allocated towards research and development, more researchers would be able to tap into public grants to expand the research in AI.
Promote Corporate Funding and Empanelment
The onus of funding AI research cannot only fall on public institutions. Private Indian corporations would also be a huge beneficiary of locally developed AI competency. It is critical that we continue the work in building the bridge between academia and corporations to fund and promote AI research.
Several large companies, as well as startups, recognize the need for indigenously developed research in Artificial Intelligence. Corporate empanelment programs take multiple forms – from the low touch speaker arrangements to corporations helping setup topical centers of excellence at universities in a technology area that is key to their business success. More arrangements of the latter form are necessary, in addition to individual research grants and scholarships that corporations provide.
Release government datasets for AI algorithm development
Activating the development and learning for AI algorithms requires access to a lot of data. Here again, the government should step in to provide the relevant data sets to researchers working on complex, India-specific problems.
In multiple circumstances, the government holds a treasure trove of data which can be hugely beneficial for the learning cycle of algorithms and promoting their development. The government should take a positive stand on sharing data sets, all the while keeping in mind data security measures and privacy rights of citizens.
Two-Pronged Approach – Core AI as well as Applications-Led IP Development
Research in AI needs to be supported at two levels. First, the development of patented core AI algorithms that will have broad, cross-industry applicability. The second is the development of intellectual property that is topical to industry or sector-specific problems.
India suffers from numerous topical problems that need an AI-led solution – from providing health care services to our burgeoning population, support for those engaged in the agricultural sector and provision of basic public infrastructure – roads, hospitals, schools and sanitation facilities. In addition to developing core mathematical capability, significant value can be unlocked through by developing expertise around these large, complex problems in AI, with solutions that can be applied to help countries facing similar problems.
Building core R&D capabilities and more IPs in Artificial Intelligence is key to cementing India’s position and competitiveness in this space. Building strong capabilities in AI is now a mandate for most of the world’s most powerful countries and it is imperative that India does not fall by the wayside. The good news is that NITI Aayog, MEITY, NASSCOM and others are already taking concrete steps to engage the stakeholder community – researchers, educational and corporate institutions – for AI research. The foundational aspects – an inclination towards STEM streams and an orientation towards key subject areas among the current students is there as well. It is time we harness their wisdom and knowledge to catapult India to the forefront in the field of AI.